- Ruhr-Universität Bochum
Scientific output
Publications
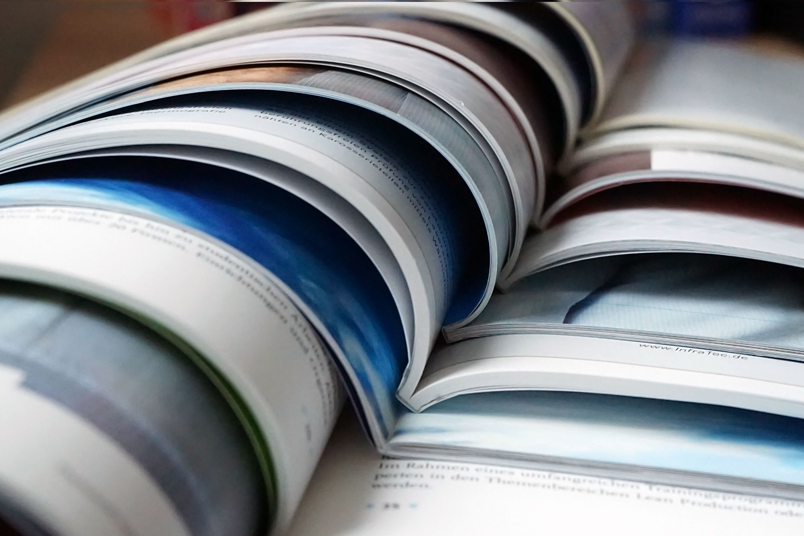
Below, you can either scroll through the complete list of our annually published research in peer-reviewed journals or search for a specific author or keyword via the free text search.
- 2024 • 7852
Testing equivalence of multinomial distributions — A constrained bootstrap approach
Bastian, P. and Dette, H. and Koletzko, L.
STATISTICS AND PROBABILITY LETTERS. Volume: 206 (2024) - 2024 • 7851
Comparing regression curves: an L 1-point of view
Bastian, P. and Dette, H. and Koletzko, L. and Möllenhoff, K.
ANNALS OF THE INSTITUTE OF STATISTICAL MATHEMATICS. Volume: 76 (2024) - 2024 • 7850
Investigating the inflow into a granular bed using a locally resolved method
Brömmer, M. and Scharnowski, M. and Illana Mahiques, E. and Wirtz, S. and Scherer, V.
PARTICUOLOGY. Volume: 85 (2024) - 2024 • 7849
Ni-Alloyed Copper Iodide Thin Films: Microstructural Features and Functional Performance
Dethloff, C. and Thieme, K. and Selle, S. and Seifert, M. and Vogt, S. and Splith, D. and Botti, S. and Grundmann, M. and Lorenz, M.
PHYSICA STATUS SOLIDI (B) BASIC RESEARCH. Volume: (2024) - 2024 • 7848
Statistical inference for function-on-function linear regression
Dette, H. and Tang, J.
BERNOULLI. Volume: 30 (2024) - 2024 • 7847
Phase formation and electrical properties of reactively sputtered Fe1−x O thin films
Evertz, S. and Nicolin, N. and Cheng, N. and Primetzhofer, D. and Best, J.P. and Dehm, G.
JOURNAL OF PHYSICS D: APPLIED PHYSICS. Volume: 57 (2024) - 2024 • 7846
Prediction of high- Tc superconductivity in ternary actinium beryllium hydrides at low pressure
Gao, K. and Cui, W. and Shi, J. and Durajski, A. P. and Hao, J. and Botti, S. and Marques, M. A. L. and Li, Y.
PHYSICAL REVIEW B. Volume: 109 (2024) - 2024 • 7845
Prediction of high- Tc superconductivity in ternary actinium beryllium hydrides at low pressure
Gao, K. and Cui, W. and Shi, J. and Durajski, A. P. and Hao, J. and Botti, S. and Marques, M. A. L. and Li, Y.
PHYSICAL REVIEW B. Volume: 109 (2024) - 2024 • 7844
Comparing two IBM implementations for the simulation of uniform packed beds
Gorges, C. and Brömmer, M. and Velten, C. and Wirtz, S. and Mahiques, E.I. and Scherer, V. and Zähringer, K. and van Wachem, B.
PARTICUOLOGY. Volume: 86 (2024) - 2024 • 7843
pH-Dependent photocatalytic performance of faceted BiOBr semiconductor particles in degradation of dyes
Guo, Y. and Siretanu, I. and Mugele, F. and Mul, G. and Mei, B.
MOLECULAR CATALYSIS. Volume: 553 (2024) - 2024 • 7842
pH-Dependent photocatalytic performance of faceted BiOBr semiconductor particles in degradation of dyes
Guo, Y. and Siretanu, I. and Mugele, F. and Mul, G. and Mei, B.
MOLECULAR CATALYSIS. Volume: 553 (2024) - 2024 • 7841
Influence of pre-existing configurations of dislocations on the initial pop-in load during nanoindentation in a CrCoNi medium-entropy alloy
Habiyaremye, F. and Guitton, A. and Chen, X. and Richeton, T. and Berbenni, S. and Schäfer, F. and Laplanche, G. and Maloufi, N.
PHILOSOPHICAL MAGAZINE. Volume: 104 (2024) - 2024 • 7840
Spatially resolved investigation of flame particle interaction in a two dimensional model packed bed
Khodsiani, M. and Namdar, R. and Varnik, F. and Beyrau, F. and Fond, B.
PARTICUOLOGY. Volume: 85 (2024) - 2024 • 7839
On the inherent strength of Cr23C6 with the complex face-centered cubic D84 structure
Kishida, K. and Ito, M. and Inui, H. and Heilmaier, M. and Eggeler, G.
ACTA MATERIALIA. Volume: 263 (2024) - 2024 • 7838
Numerical study of convective heat transfer in static arrangements of particles with arbitrary shapes: A monolithic hybrid lattice Boltzmann-finite difference-phase field solver
Namdar, R. and Khodsiani, M. and Safari, H. and Neeraj, T. and Hosseini, S.A. and Beyrau, F. and Fond, B. and Thévenin, D. and Varnik, F.
PARTICUOLOGY. Volume: 85 (2024) - 2024 • 7837
Investigating the platinum electrode surface during Kolbe electrolysis of acetic acid
Olde Nordkamp, M. and Ashraf, T. and Altomare, M. and Borca, A. C. and Ghigna, P. and Priamushko, T. and Cherevko, S. and Saveleva, V. A. and Atzori, C. and Minguzzi, A. and He, X. and Mul, G. and Mei, B.
SURFACES AND INTERFACES. Volume: 44 (2024) - 2024 • 7836
Investigating the platinum electrode surface during Kolbe electrolysis of acetic acid
Olde Nordkamp, M. and Ashraf, T. and Altomare, M. and Borca, A. C. and Ghigna, P. and Priamushko, T. and Cherevko, S. and Saveleva, V. A. and Atzori, C. and Minguzzi, A. and He, X. and Mul, G. and Mei, B.
SURFACES AND INTERFACES. Volume: 44 (2024) - 2024 • 7835
A simulation study for a cost-effective PET-like detector system intended to track particles in granular assemblies
Oppotsch, J. and Athanassiadis, A. and Fritsch, M. and Heinsius, F.-H. and Held, T. and Hilse, N. and Scherer, V. and Steinke, M. and Wiedner, U.
PARTICUOLOGY. Volume: 84 (2024) - 2024 • 7834
Misfit and the mechanism of high temperature and low stress creep of Ni-base single crystal superalloys
Parsa, A.B. and Bürger, D. and Pollock, T.M. and Eggeler, G.
ACTA MATERIALIA. Volume: 264 (2024) - 2024 • 7833
Tunnel lining segments with enhanced bearing capacity using hybrid concrete concepts
Petraroia, D. N. and Plückelmann, S. and Mark, P. and Breitenbücher, R.
TUNNELLING AND UNDERGROUND SPACE TECHNOLOGY. Volume: 143 (2024) - 2024 • 7832
The catalytic effect of iron oxide phases on the conversion of cellulose-derived chars in diluted O2 and CO2
Pflieger, C. and Eckhard, T. and Böttger, J. and Schulwitz, J. and Herrendorf, T. and Schmidt, S. and Salamon, S. and Landers, J. and Wende, H. and Kleist, W. and Muhler, M. and Cerciello, F.
APPLIED ENERGY. Volume: 353 (2024) - 2024 • 7831
Sputter-Deposited La–Co–Mn–O Nanocolumns as Stable Electrocatalyst for the Oxygen Evolution Reaction
Piotrowiak, T. H. and Krysiak, O. A. and Suhr, E. and Zhang, J. and Zehl, R. and Kostka, A. and Schuhmann, W. and Ludwig, A.
SMALL STRUCTURES. Volume: (2024) - 2024 • 7830
Sputter-Deposited La–Co–Mn–O Nanocolumns as Stable Electrocatalyst for the Oxygen Evolution Reaction
Piotrowiak, T. H. and Krysiak, O. A. and Suhr, E. and Zhang, J. and Zehl, R. and Kostka, A. and Schuhmann, W. and Ludwig, A.
SMALL STRUCTURES. Volume: (2024) - 2024 • 7829
Convective drying of wood chips: Accelerating coupled DEM-CFD simulations with parametrized reduced single particle models
Reineking, L. and Fischer, J. and Mjalled, A. and Illana, E. and Wirtz, S. and Scherer, V. and Mönnigmann, M.
PARTICUOLOGY. Volume: 84 (2024) - 2024 • 7828
Non-collinear magnetic atomic cluster expansion for iron
Rinaldi, M. and Mrovec, M. and Bochkarev, A. and Lysogorskiy, Y. and Drautz, R.
NPJ COMPUTATIONAL MATERIALS. Volume: 10 (2024) - 2024 • 7827
Effect of stacking fault energy on the thickness and density of annealing twins in recrystallized FCC medium and high-entropy alloys
Schneider, M. and Couzinié, J. and Shalabi, A. and Ibrahimkhel, F. and Ferrari, A. and Körmann, F. and Laplanche, G.
SCRIPTA MATERIALIA. Volume: 240 (2024) - 2024 • 7826
Mode Transition Induced by Gas Heating Along the Discharge Channel in Capacitively Coupled Atmospheric Pressure Micro Plasma Jets
Schulenberg, D. A. and Vass, M. and Klich, M. and Donkó, Z. and Klotz, J. and Bibinov, N. and Mussenbrock, T. and Schulze, J.
PLASMA CHEMISTRY AND PLASMA PROCESSING. Volume: (2024) - 2024 • 7825
Extending the variational quantum eigensolver to finite temperatures
Selisko, J. and Amsler, M. and Hammerschmidt, T. and Drautz, R. and Eckl, T.
QUANTUM SCIENCE AND TECHNOLOGY. Volume: 9 (2024) - 2024 • 7824
Parametrization protocol and refinement strategies for accurate and transferable analytic bond-order potentials: Application to Re
Subramanyam, A. P. A. and Jenke, J. and Ladines, A. N. and Drautz, R. and Hammerschmidt, T.
PHYSICAL REVIEW MATERIALS. Volume: 8 (2024) - 2024 • 7823
Effect of Deformation on the Magnetic Properties of C + N Austenitic Steel
Torres-Mejía, L. G. and Paredes-Gil, K. and Parra Vargas, C. A. and Lentz, J. and Weber, S. and Mujica-Roncery, L.
METALLURGICAL AND MATERIALS TRANSACTIONS A: PHYSICAL METALLURGY AND MATERIALS SCIENCE. Volume: 55 (2024) - 2024 • 7822
Influence of the catalyst precursor for cobalt on activated carbon applied in ammonia decomposition
Winter, F. L. and Diehl, P. and Telaar, P. and Watermann, C. M. and Kaluza, S. and Muhler, M. and Apfel, U. and Zeidler-Fandrich, B.
CATALYSIS TODAY. Volume: (2024) - 2024 • 7821
Combinatorial Screening of Electronic and Geometric Effects in Compositionally Complex Solid Solutions Toward a Rational Design of Electrocatalysts
Zerdoumi, R. and Savan, A. and Amalraj, M. and Tetteh, E. B. and Lourens, F. and Krysiak, O. A. and Junqueira, J. R. C. and Ludwig, A. and Schuhmann, W.
ADVANCED ENERGY MATERIALS. Volume: 14 (2024) - 2023 • 7820
A Unifying Perspective of Common Motifs That Occur across Disparate Classes of Materials Harboring Displacive Phase Transitions
Grünebohm, A. and Hütten, A. and Böhmer, A. E. and Frenzel, J. and Eremin, I. and Drautz, R. and Ennen, I. and Caron, L. and Kuschel, T. and Lechermann, F. and Anselmetti, D. and Dahm, T. and Weber, F. and Rossnagel, K. and Schierning, G.
ADVANCED ENERGY MATERIALS. Volume: 13 (2023) - 2023 • 7819
High nitrogen steels produced by PBF-LB/M - Process strategy and properties for ceramic additivated metal powders
Radtke, F. and Becker, L. and Herzog, S. and Lentz, J. and Weber, S. and Broeckmann, C.
EURO POWDER METALLURGY 2023 CONGRESS AND EXHIBITION, PM 2023. Volume: (2023) - 2023 • 7818
Facile Solid-State Synthesis of Supported PtNi and PtCo Bimetallic Nanoparticles for the Oxygen Reduction Reaction
Gunnarson, A. and De Bellis, J. and Imhof, T. and Pfänder, N. and Ledendecker, M. and Schüth, F.
CHEMISTRY OF MATERIALS. Volume: 35 (2023) - 2023 • 7817
Introduction to Materials Informatics
Rajan, K. and Behler, J. and Pickard, C.J.
MATERIALS ADVANCES. Volume: (2023) - 2023 • 7816
Significantly boosted activity for styrene oxidation through simultaneous regulation of porosity and copper sites in microporous metal-organic framework Cu-BTC
Guo, P. and Zhang, S. and Cheng, H. and Zeng, X. and Wang, H. and Fischer, R.A. and Muhler, M.
CATALYSIS SCIENCE AND TECHNOLOGY. Volume: (2023) - 2023 • 7815
Hardening effect of diffusible hydrogen on BCC Fe-based model alloys by in situ backside hydrogen charging
Rao, J. and Lee, S. and Dehm, G. and Duarte, M. J.
MATERIALS AND DESIGN. Volume: 232 (2023) - 2023 • 7814
Band alignment at the strontium germanate interface with silicon
Rauch, T. and Marton, P. and Botti, S. and Hlinka, J.
PHYSICAL REVIEW B. Volume: 107 (2023) - 2023 • 7813
Low-Temperature Plasma Nitriding of Martensitic and Austenitic Steels to Increase Tribocorrosion Resistance∗ [Niedertemperaturplasmanitrieren martensitischer und austenitischer Stähle zur Erhöhung der Tribokorrosionsbeständigkeit]
Hahn, I. and Siebert, S. and Paschke, H. and Brückner, T. and Weber, S.
HTM - JOURNAL OF HEAT TREATMENT AND MATERIALS. Volume: 78 (2023) - 2023 • 7812
Computational modeling of fiber orientation during 3D-concrete-printing
Reinold, J. and Gudžulić, V. and Meschke, G.
COMPUTATIONAL MECHANICS. Volume: (2023) - 2023 • 7811
Dynamical Franz-Keldysh Effect in Diamond in the Deep Ultraviolet Probed by Transient Absorption and Dispersion Spectroscopy Using a Miniature Beamline
Reislöhner, J. and Chen, X. and Kim, D. and Botti, S. and Pfeiffer, A. N.
PHYSICAL REVIEW LETTERS. Volume: 131 (2023) - 2023 • 7810
Numerical and experimental studies on crack nucleation and propagation in thin films
Harandi, A. and Rezaei, S. and Karimi Aghda, S. and Du, C. and Brepols, T. and Dehm, G. and Schneider, J. M. and Reese, S.
INTERNATIONAL JOURNAL OF MECHANICAL SCIENCES. Volume: 258 (2023) - 2023 • 7809
The dynamical Franz-Keldysh effect in the deep ultraviolet probed by transient absorption and dispersion of diamond
Reislöhner, J. and Chen, X. and Kim, D. and Botti, S. and Pfeiffer, A. N.
2023 CONFERENCE ON LASERS AND ELECTRO-OPTICS EUROPE AND EUROPEAN QUANTUM ELECTRONICS CONFERENCE, CLEO/EUROPE-EQEC 2023. Volume: (2023) - 2023 • 7808
Silver Thin-Film Electrodes Grown by Low-Temperature Plasma-Enhanced Spatial Atomic Layer Deposition at Atmospheric Pressure
Hasselmann, T. and Misimi, B. and Boysen, N. and Zanders, D. and Wree, J. and Rogalla, D. and Haeger, T. and Zimmermann, F. and Brinkmann, K. O. and Schädler, S. and Theirich, D. and Heiderhoff, R. and Devi, A. and Riedl, T.
ADVANCED MATERIALS TECHNOLOGIES. Volume: 8 (2023) - 2023 • 7807
Fertigteilüberbauten für Brücken aus Hochfestem Beton – schneller, schlanker und nachhaltiger
Haveresch, K. and Maurer, R. and Breitenbücher, R.
BETON- UND STAHLBETONBAU. Volume: (2023) - 2023 • 7806
Elastic waveform inversion in the frequency domain for an application in mechanized tunneling
Riedel, C. and Musayev, K. and Baitsch, M. and Hackl, K.
TUNNELLING AND UNDERGROUND SPACE TECHNOLOGY. Volume: 137 (2023) - 2023 • 7805
Influence of chemical composition on coarsening kinetics of coherent L12 precipitates in FCC complex concentrated alloys
Rieger, T. and Joubert, J. and Poulain, R. and Sauvage, X. and Paccou, E. and Perrière, L. and Guillot, I. and Dirras, G. and Laplanche, G. and Laurent-Brocq, M. and Couzinié, J.
JOURNAL OF ALLOYS AND COMPOUNDS. Volume: 967 (2023) - 2023 • 7804
A simple and efficient lagrange multiplier based mixed finite element for gradient damage
Riesselmann, J. and Balzani, D.
COMPUTERS AND STRUCTURES. Volume: 281 (2023) - 2023 • 7803
Description of a new concept for the development of adapted hot-work tool steels for laser-powder bed fusion
Röttger, A. and Boes, J. and Großwendt, F. and Weber, S.
ADDITIVE MANUFACTURING. Volume: 61 (2023) - 2023 • 7802
Machine learning transferable atomic forces for large systems from underconverged molecular fragments
Herbold, M. and Behler, J.
PHYSICAL CHEMISTRY CHEMICAL PHYSICS. Volume: 25 (2023) - 2023 • 7801
Effects of laser-structured and APS flash-coated CoNiCrAlY bondcoats on the furnace cycle lifetime of double-layered Y2O3-stabilized ZrO2/MgAl2O4 abradable coatings
Heyl, H. and Mack, D.E. and Tandler, M. and Schrüfer, S. and Vaßen, R.
SURFACE AND COATINGS TECHNOLOGY. Volume: 464 (2023) - 2023 • 7800
Stability and Failure Mechanisms of Al2O3|Al Bilayer Coatings Exposed to 300 Bar Hydrogen at 673 K
Hieke, S. W. and Frank, A. and Duarte, M. J. and Gopalan, H. and Patil, P. and Wetegrove, M. and Rohloff, M. and Kruth, A. and Pistidda, C. and Dornheim, M. and Taube, K. and Dehm, G. and Scheu, C.
ADVANCED ENGINEERING MATERIALS. Volume: (2023) - 2023 • 7799
Determination of acoustic phonon anharmonicities via second-order Raman scattering in CuI
Hildebrandt, R. and Seifert, M. and George, J. and Blaurock, S. and Botti, S. and Krautscheid, H. and Grundmann, M. and Sturm, C.
NEW JOURNAL OF PHYSICS. Volume: 25 (2023) - 2023 • 7798
Modeling of minimal systems based on ATP-Zn coordination for chemically fueled self-assembly
Rossi, E. and Ferrarini, A. and Sulpizi, M.
PHYSICAL CHEMISTRY CHEMICAL PHYSICS. Volume: 25 (2023) - 2023 • 7797
Storing MEMS Interfaces Without Electrical Auxiliary Energy for Long-Time Monitoring
Hoffmann, M. and Schmitt, P. and Wittemeier, S. and Schaller, F. and Shaporin, A. and Stockel, C. and Geneis, V. and Forke, R. and Hedayat, C. and Hilleringmann, U. and Kuhn, H. and Zimmermann, S.
PROCEEDINGS OF THE IEEE INTERNATIONAL CONFERENCE ON MICRO ELECTRO MECHANICAL SYSTEMS (MEMS). Volume: 2023-January (2023) - 2023 • 7796
Transfer learning on large datasets for the accurate prediction of material properties
Hoffmann, N. and Schmidt, J. and Botti, S. and Marques, M. A. L.
DIGITAL DISCOVERY. Volume: 2 (2023) - 2023 • 7795
Increasing the O2 Resistance of the [FeFe]-Hydrogenase CbA5H through Enhanced Protein Flexibility
Rutz, A. and Das, C.K. and Fasano, A. and Jaenecke, J. and Yadav, S. and Apfel, U.-P. and Engelbrecht, V. and Fourmond, V. and Léger, C. and Schäfer, L.V. and Happe, T.
ACS CATALYSIS. Volume: 13 (2023) - 2023 • 7794
Semiconductor Lasers
Hofmann, M.R. and Koch, S.W.
SPRINGER HANDBOOKS. Volume: (2023) - 2023 • 7793
Adaptive geometric multigrid for the mixed finite cell formulation of Stokes and Navier–Stokes equations
Saberi and Meschke, G. and Vogel, A.
INTERNATIONAL JOURNAL FOR NUMERICAL METHODS IN FLUIDS. Volume: (2023) - 2023 • 7792
Water Management as a Key Parameter for Scaling up a CO2 Electrolyzer [Wassermanagement als Schlüsselparameter für die Skalierung eines CO2-Elektrolyseurs]
Hoof, L. and Pellumbi, K. and Heuser, S. and Siegmund, D. and junge Puring, K. and Apfel, U.-P.
CHEMIE-INGENIEUR-TECHNIK. Volume: (2023) - 2023 • 7791
The Transformation of Inorganic to Organic Carbonates: Chasing for Reaction Pathways in Mechanochemistry
Sander, M. and Fabig, S. and Borchardt, L.
CHEMISTRY - A EUROPEAN JOURNAL. Volume: 29 (2023) - 2023 • 7790
The role of incoherent twin boundaries on the plasticity of Cu micropillars
Hosseinabadi, R. and Brognara, A. and Kirchlechner, C. and Best, J. P. and Dehm, G.
MATERIALS AND DESIGN. Volume: 232 (2023) - 2023 • 7789
Descriptor for slip-induced crack blunting in refractory ceramics
Sangiovanni, D. G. and Kraych, A. and Mrovec, M. and Salamania, J. and Odén, M. and Tasnádi, F. and Abrikosov, I. A.
PHYSICAL REVIEW MATERIALS. Volume: 7 (2023) - 2023 • 7788
Quantitative tests revealing hydrogen-enhanced dislocation motion in α-iron
Huang, L. and Chen, D. and Xie, D. and Li, S. and Zhang, Y. and Zhu, T. and Raabe, D. and Ma, E. and Li, J. and Shan, Z.
NATURE MATERIALS. Volume: (2023) - 2023 • 7787
Influence of deformation degree at cold drawing on structure-properties relationship of a Fe-Ni-Cr superalloy
Huang, S. and Hu, J. and Li, X. and Liu, J. and Liu, K. and Qin, H. and Wang, H.
JOURNAL OF ALLOYS AND COMPOUNDS. Volume: 930 (2023) - 2023 • 7786
Understanding the protective ability of the native oxide on an Fe-13 at% Cr alloy at the atomic scale: A combined atom probe and electron microscopy study
Sasidhar, K.N. and Khanchandani, H. and Zhang, S. and da Silva, A.K. and Scheu, C. and Gault, B. and Ponge, D. and Raabe, D.
CORROSION SCIENCE. Volume: 211 (2023) - 2023 • 7785
Triflate vs Acetonitrile: Understanding the Iron(II)-Based Coordination Chemistry of Tri(quinolin-8-yl)amine
Hüppe, H.M. and Iffland-Mühlhaus, L. and Heck, J. and Eilers, M. and Gildenast, H. and Schönfeld, S. and Dürrmann, A. and Hoffmann, A. and Weber, B. and Apfel, U.-P. and Herres-Pawlis, S.
INORGANIC CHEMISTRY. Volume: 62 (2023) - 2023 • 7784
Effect of substrate temperature on in-situ precipitation during laser powder bed fusion of Fe-TiB2 high modulus steel
Hussain, S. and Tönnißen, N. and Soares Barreto, E. and Gärtner, E. and Kostka, A. and Springer, H. and Uhlenwinkel, V. and Ellendt, N.
VIRTUAL AND PHYSICAL PROTOTYPING. Volume: 18 (2023) - 2023 • 7783
Fatigue behavior and crack opening tests under tensile stress on HPSFRC: Experimental and numerical investigations
Schäfer, N. and Gudžulić, V. and Breitenbücher, R. and Meschke, G.
CURRENT PERSPECTIVES AND NEW DIRECTIONS IN MECHANICS, MODELLING AND DESIGN OF STRUCTURAL SYSTEMS - PROCEEDINGS OF THE 8TH INTERNATIONAL CONFERENCE ON STRUCTURAL ENGINEERING, MECHANICS AND COMPUTATION, 2022. Volume: (2023) - 2023 • 7782
Atomic cluster expansion for a general-purpose interatomic potential of magnesium
Ibrahim, E. and Lysogorskiy, Y. and Mrovec, M. and Drautz, R.
PHYSICAL REVIEW MATERIALS. Volume: 7 (2023) - 2023 • 7781
Locally Resolved Simulation of Gas Mixing and Combustion Inside Static and Moving Particle Assemblies
Illana Mahiques, E. and Brömmer, M. and Wirtz, S. and Scherer, V.
CHEMICAL ENGINEERING AND TECHNOLOGY. Volume: (2023) - 2023 • 7780
Compressible cementitious composite materials: Multiscale modeling and experimental investigation
Iskhakov, T. and Timothy, J. J. and Plückelmann, S. and Breitenbücher, R. and Meschke, G.
CEMENT AND CONCRETE COMPOSITES. Volume: 142 (2023) - 2023 • 7779
Towards active learning: A stopping criterion for the sequential sampling of grain boundary degrees of freedom
Schmalofski, T. and Kroll, M. and Dette, H. and Janisch, R.
MATERIALIA. Volume: 31 (2023) - 2023 • 7778
Determination of factory locations for distributed additive manufacturing, considering pollution, resilience and costs
Schmidt, C. and Finsterwalder, F. and Griesbaum, R. and Sehrt, J.T.
CIRP JOURNAL OF MANUFACTURING SCIENCE AND TECHNOLOGY. Volume: 43 (2023) - 2023 • 7777
Machine-Learning-Assisted Determination of the Global Zero-Temperature Phase Diagram of Materials
Schmidt, J. and Hoffmann, N. and Wang, H. and Borlido, P. and Carriço, P. J. M. A. and Cerqueira, T. F. T. and Botti, S. and Marques, M. A. L.
ADVANCED MATERIALS. Volume: 35 (2023) - 2023 • 7776
Machine learning guided high-throughput search of non-oxide garnets
Schmidt, J. and Wang, H. and Schmidt, G. and Marques, M. A. L.
NPJ COMPUTATIONAL MATERIALS. Volume: 9 (2023) - 2023 • 7775
X-radiography front tracking gradient furnace for directional solidification of bulk Al-alloys
Jafarizadeh-Koohbanani, A. and Steinbach, S. and Drescher, J. and Frenzel, J. and Kargl, F.
REVIEW OF SCIENTIFIC INSTRUMENTS. Volume: 94 (2023) - 2023 • 7774
Enhancement of CO2RR product formation on Cu-ZnO-based electrodes by varying ink formulation and post-treatment methods
Jaster, T. and Albers, S. and Leonhard, A. and Kräenbring, M.-A. and Lohmann, H. and Zeidler-Fandrich, B. and Özcan, F. and Segets, D. and Apfel, U.-P.
JPHYS ENERGY. Volume: 5 (2023) - 2023 • 7773
Tethering zwitterionic polymer coatings to mediated glucose biosensor enzyme electrodes can decrease sensor foreign body response yet retain sensor sensitivity to glucose
Jayakumar, K. and Lielpetere, A. and Domingo-Lopez, D.A. and Levey, R.E. and Duffy, G.P. and Schuhmann, W. and Leech, D.
BIOSENSORS AND BIOELECTRONICS. Volume: 219 (2023) - 2023 • 7772
Local strength of bainitic and ferritic HSLA steel constituents understood using correlative electron microscopy and microcompression testing
Jentner, R.M. and Scholl, S. and Srivastava, K. and Best, J.P. and Kirchlechner, C. and Dehm, G.
MATERIALS AND DESIGN. Volume: 236 (2023) - 2023 • 7771
Unsupervised clustering of nanoindentation data for microstructural reconstruction: Challenges in phase discrimination
Jentner, R.M. and Srivastava, K. and Scholl, S. and Gallardo-Basile, F.J. and Best, J.P. and Kirchlechner, C. and Dehm, G.
MATERIALIA. Volume: 28 (2023) - 2023 • 7770
Automated classification of granular bainite and polygonal ferrite by electron backscatter diffraction verified through local structural and mechanical analyses
Jentner, R.M. and Tsai, S.P. and Welle, A. and Scholl, S. and Srivastava, K. and Best, J.P. and Kirchlechner, C. and Dehm, G.
JOURNAL OF MATERIALS RESEARCH. Volume: 38 (2023) - 2023 • 7769
In Pursuit of Next Generation N-Heterocyclic Carbene-Stabilized Copper and Silver Precursors for Metalorganic Chemical Vapor Deposition and Atomic Layer Deposition Processes
Selvakumar, I. and Boysen, N. and Bürger, M. and Devi, A.
CHEMISTRY (SWITZERLAND). Volume: 5 (2023) - 2023 • 7768
Interface formation evolution of the hot-forged copper-(Cr)diamond composite and its thermal conductivity
Jia, S.Q. and Bolzoni, L. and Li, T. and Yang, F.
JOURNAL OF ALLOYS AND COMPOUNDS. Volume: 943 (2023) - 2023 • 7767
3D phase-field simulations to machine-learn 3D information from 2D micrographs
Jiang, Y. and Ali, M.A. and Roslyakova, I. and Bürger, D. and Eggeler, G. and Steinbach, I.
MODELLING AND SIMULATION IN MATERIALS SCIENCE AND ENGINEERING. Volume: 31 (2023) - 2023 • 7766
Coherent twin-oriented Al3Sc-based precipitates in Al matrix
Shanmugam, S. and Peterlechner, M. and Iskandar, M.R. and Saikia, U. and Kulitckii, V. and Lipińska-Chwałek, M. and Mayer, J. and Rösner, H. and Hickel, T. and Divinski, S.V. and Wilde, G.
SCRIPTA MATERIALIA. Volume: 229 (2023) - 2023 • 7765
Evaluation of major factors influencing the TBC topcoat formation in axial suspension plasma spraying (SPS)
Joeris, J. and Tiwari, A. and Brinckmann, S. and Kurze, F. and Guillon, O. and Vaßen, R.
INTERNATIONAL JOURNAL OF APPLIED CERAMIC TECHNOLOGY. Volume: 20 (2023) - 2023 • 7764
Elementary molecular processes that govern the chemical stimulation of a polyurethane-based shape memory polymer
Shen, Y. and Dumlu, H. and Eggeler, G. and Neuking, K.
POLYMER. Volume: 280 (2023) - 2023 • 7763
Oscillating combustion of different fuel types for NOX reduction in grate furnaces and coal burners
Jolibois, N. and Gehrmann, H.-J. and Aleksandrov, K. and Hauser, M. and Stapf, D. and Jäger, B. and Wirtz, S. and Scherer, V. and Pollmeier, G. and Danz, P. and Matthes, J. and Vogelbacher, M. and Waibel, P.
FUEL. Volume: 340 (2023) - 2023 • 7762
Cyclic deformation behavior of an equiatomic CrFeNi multi-principal element alloy
Sisodia, S. and Rajkowski, M. and Laplanche, G. and Chauhan, A.
INTERNATIONAL JOURNAL OF FATIGUE. Volume: 174 (2023) - 2023 • 7761
Site occupancies in a chemically complex σ-phase from the high-entropy Cr–Mn–Fe–Co–Ni system
Joubert, J. and Kalchev, Y. and Fantin, A. and Crivello, J. and Zehl, R. and Elkaim, E. and Laplanche, G.
ACTA MATERIALIA. Volume: 259 (2023) - 2023 • 7760
Simultaneous Anodic and Cathodic Formate Production in a Paired Electrolyzer by CO2 Reduction and Glycerol Oxidation
Junqueira, J.R.C. and Das, D. and Cathrin Brix, A. and Dieckhöfer, S. and Weidner, J. and Wang, X. and Shi, J. and Schuhmann, W.
CHEMSUSCHEM. Volume: (2023) - 2023 • 7759
Local structure and magnetic properties of a nanocrystalline Mn-rich Cantor alloy thin film down to the atomic scale
Smekhova, A. and Kuzmin, A. and Siemensmeyer, K. and Luo, C. and Taylor, J. and Thakur, S. and Radu, F. and Weschke, E. and Buzanich, A.G. and Xiao, B. and Savan, A. and Yusenko, K.V. and Ludwig, Al.
NANO RESEARCH. Volume: (2023) - 2023 • 7758
Combined ab initio and experimental screening of phase stabilities in the Ce-Fe-Ti- X system (X=3d and 4d metals)
Sözen, H.I. and Ener, S. and Maccari, F. and Fayyazi, B. and Gutfleisch, O. and Neugebauer, J. and Hickel, T.
PHYSICAL REVIEW MATERIALS. Volume: 7 (2023) - 2023 • 7757
Persistent current-carrying state of charge quasiparticles in an np ribbon featuring a single Dirac cone
Kadigrobov, A. M. and Eremin, I. M.
PHYSICAL REVIEW B. Volume: 108 (2023) - 2023 • 7756
Probing porosity in metals by electrical conductivity: Nanoscale experiments and multiscale simulations
Kaiser, T. and Dehm, G. and Kirchlechner, C. and Menzel, A. and Bishara, H.
EUROPEAN JOURNAL OF MECHANICS, A/SOLIDS. Volume: 97 (2023) - 2023 • 7755
Nanoconfinement Allows a Less Active Cascade Catalyst to Produce More C2+ Products in Electrochemical CO2 Reduction
Somerville, S.V. and O’Mara, P.B. and Benedetti, T.M. and Cheong, S. and Schuhmann, W. and Tilley, R.D. and Gooding, J.J.
JOURNAL OF PHYSICAL CHEMISTRY C. Volume: 127 (2023) - 2023 • 7754
Microstructure and Mechanical Properties of a Ni-Based Superalloy Thin Film Investigated by Micropillar Compression
Kalchev, Y. and Zehl, R. and Piotrowiak, T. and Kostka, A. and Naujoks, D. and Pfetzing-Micklich, J. and Ludwig, A.
METALLURGICAL AND MATERIALS TRANSACTIONS A: PHYSICAL METALLURGY AND MATERIALS SCIENCE. Volume: 54 (2023) - 2023 • 7753
Fundamentals of Green Steel Production: On the Role of Gas Pressure During Hydrogen Reduction of Iron Ores
Souza Filho, I.R. and Ma, Y. and Raabe, D. and Springer, H.
JOM. Volume: (2023) - 2023 • 7752
Green laser powder bed fusion based fabrication and rate-dependent mechanical properties of copper lattices
Kang, S. and Gainov, R. and Heußen, D. and Bieler, S. and Sun, Z. and Weinberg, K. and Dehm, G. and Ramachandramoorthy, R.
MATERIALS AND DESIGN. Volume: 231 (2023) - 2023 • 7751
First-principles insight in structure-property relationships of hexagonal Si and Ge polytypes
Keller, M. and Belabbes, A. and Furthmüller, J. and Bechstedt, F. and Botti, S.
PHYSICAL REVIEW MATERIALS. Volume: 7 (2023) - 2023 • 7750
Disordering complexion transition of grain boundaries in bcc metals: Insights from atomistic simulations
Starikov, S. and Abbass, A. and Drautz, R. and Mrovec, M.
ACTA MATERIALIA. Volume: 261 (2023) - 2023 • 7749
Atomistic simulations of pipe diffusion in bcc transition metals
Starikov, S. and Jamebozorgi, V. and Smirnova, D. and Drautz, R. and Mrovec, M.
ACTA MATERIALIA. Volume: 260 (2023) - 2023 • 7748
Quantum phase transitions in non-Hermitian PT-symmetric transverse-field Ising spin chains
Starkov, G. A. and Fistul, M. V. and Eremin, I. M.
ANNALS OF PHYSICS. Volume: 456 (2023) - 2023 • 7747
Schrieffer-Wolff transformation for non-Hermitian systems: Application for PT -symmetric circuit QED
Starkov, G. A. and Fistul, M. V. and Eremin, I. M.
PHYSICAL REVIEW B. Volume: 108 (2023) - 2023 • 7746
An artificial neural network for surrogate modeling of stress fields in viscoplastic polycrystalline materials
Khorrami, M.S. and Mianroodi, J.R. and Siboni, N.H. and Goyal, P. and Svendsen, B. and Benner, P. and Raabe, D.
NPJ COMPUTATIONAL MATERIALS. Volume: 9 (2023) - 2023 • 7745
Direct-Patterning ZnO Deposition by Atomic-Layer Additive Manufacturing Using a Safe and Economical Precursor
Stefanovic, S. and Gheshlaghi, N. and Zanders, D. and Kundrata, I. and Zhao, B. and Barr, M. K. S. and Halik, M. and Devi, A. and Bachmann, J.
SMALL. Volume: 19 (2023) - 2023 • 7744
Improving the Defect Tolerance of PBF-LB/M Processed 316L Steel by Increasing the Nitrogen Content
Stern, F. and Becker, L. and Cui, C. and Tenkamp, J. and Uhlenwinkel, V. and Steinbacher, M. and Boes, J. and Lentz, J. and Fechte-Heinen, R. and Weber, S. and Walther, F.
ADVANCED ENGINEERING MATERIALS. Volume: 25 (2023) - 2023 • 7743
Geometric Deep Learning for Molecular Crystal Structure Prediction
Kilgour, M. and Rogal, J. and Tuckerman, M.
JOURNAL OF CHEMICAL THEORY AND COMPUTATION. Volume: (2023) - 2023 • 7742
Influence of nitrogen content on the corrosion fatigue behavior of additively manufactured AISI 316L stainless steel in chloride solution
Stern, F. and Becker, L. and Tenkamp, J. and Boes, J. and Lentz, J. and Weber, S. and Walther, F.
INTERNATIONAL JOURNAL OF FATIGUE. Volume: 172 (2023) - 2023 • 7741
DEF of high-performance concrete with rapid, non-standard heat-treatment
Stindt, J. and Kempf, L. and Forman, P. and Breitenbücher, R. and Mark, P.
CASE STUDIES IN CONSTRUCTION MATERIALS. Volume: 19 (2023) - 2023 • 7740
Reorganization energy in a polybromide ionic liquid measured by scanning electrochemical cell microscopy
Kim, M. and Tetteh, E.B. and Savan, A. and Xiao, B. and Ludwig, Al. and Schuhmann, W. and Chung, T.D.
THE JOURNAL OF CHEMICAL PHYSICS. Volume: 158 (2023) - 2023 • 7739
Determination of the statistical distribution of drag and lift coefficients of refuse derived fuel by computer vision
Streier, R. and Wirtz, S. and Aleksandrov, K. and Gehrmann, H.-J. and Stapf, D. and Zhang, M. and Vogelbacher, M. and Matthes, J. and Scherer, V.
FUEL. Volume: 338 (2023) - 2023 • 7738
Light Olefins from Acetylene under Pressurized Conditions
Agbaba, O. and Trotuş, I.-T. and Schmidt, W. and Schüth, F.
INDUSTRIAL AND ENGINEERING CHEMISTRY RESEARCH. Volume: 62 (2023) - 2023 • 7737
On the sliding wear and solid particle erosion behaviour of HVOF-sprayed CoNiCrAlY coatings and NiCrCoTi substrates in dependence of the oxidation dwell time at 900 °C
Kiryc, M. and Kurumlu, D. and Eggeler, G. and Vaßen, R. and Marginean, G.
SURFACE AND COATINGS TECHNOLOGY. Volume: 453 (2023) - 2023 • 7736
Microstates and defects of incoherent Σ3 [111] twin boundaries in aluminum
Ahmad, S. and Brink, T. and Liebscher, C.H. and Dehm, G.
ACTA MATERIALIA. Volume: 243 (2023) - 2023 • 7735
Room-temperature deformation of single crystals of the sigma-phase compound FeCr with the tetragonal D8b structure investigated by micropillar compression
Kishida, K. and Okutani, M. and Suzuki, H. and Inui, H. and Heilmaier, M. and Raabe, D.
ACTA MATERIALIA. Volume: 249 (2023) - 2023 • 7734
Data regarding the experimental findings compared with CALPHAD calculations of the AlMo0.5NbTa0.5TiZr refractory high entropy superalloy
Suárez Ocaño, P. and Agudo Jácome, L. and Lopez-Galilea, I. and Kamachali, R.D. and Fries, S.G.
DATA IN BRIEF. Volume: 46 (2023) - 2023 • 7733
Interstitial Segregation has the Potential to Mitigate Liquid Metal Embrittlement in Iron
Ahmadian, A. and Scheiber, D. and Zhou, X. and Gault, B. and Romaner, L. and Kamachali, R. D. and Ecker, W. and Dehm, G. and Liebscher, C. H.
ADVANCED MATERIALS. Volume: 35 (2023) - 2023 • 7732
Influence of cooling rate on the microstructure and room temperature mechanical properties in the refractory AlMo0.5NbTa0.5TiZr superalloy
Suárez Ocaño, P. and Manzoni, A. and Lopez-Galilea, I. and Ruttert, B. and Laplanche, G. and Agudo Jácome, L.
JOURNAL OF ALLOYS AND COMPOUNDS. Volume: 949 (2023) - 2023 • 7731
Competition of density waves and superconductivity in twisted tungsten diselenide
Klebl, L. and Fischer, A. and Classen, L. and Scherer, M.M. and Kennes, D.M.
PHYSICAL REVIEW RESEARCH. Volume: 5 (2023) - 2023 • 7730
Coherency loss marking the onset of degradation in high temperature creep of superalloys: Phase-field simulation coupled to strain gradient crystal plasticity
Ali, M.A. and Shchyglo, O. and Stricker, M. and Steinbach, I.
COMPUTATIONAL MATERIALS SCIENCE. Volume: 220 (2023) - 2023 • 7729
Accurate Fourth-Generation Machine Learning Potentials by Electrostatic Embedding
Ko, T.W. and Finkler, J.A. and Goedecker, S. and Behler, J.
JOURNAL OF CHEMICAL THEORY AND COMPUTATION. Volume: 19 (2023) - 2023 • 7728
Multimodal characterization of carbon electrodes' thermal activation for vanadium redox flow batteries
Köble, K. and Jaugstetter, M. and Schilling, M. and Braig, M. and Diemant, T. and Tschulik, K. and Zeis, R.
JOURNAL OF POWER SOURCES. Volume: 569 (2023) - 2023 • 7727
Aerobic oxidative lactonization of diols at room temperature over defective titanium-based oxides in water
Tang, D. and Shen, Z. and Lechler, S. and Lu, G. and Yao, L. and Hu, Y. and Huang, X. and Muhler, M. and Zhao, G. and Peng, B.
JOURNAL OF CATALYSIS. Volume: 418 (2023) - 2023 • 7726
Influence of refractory and platinum group metals on the microstructure and thermo-physical properties of Co-Al-W-based superalloys
Köbrich, M. and Förner, A. and Volz, N. and Zenk, C.H. and Fries, S.G. and Göken, M. and Neumeier, S.
JOURNAL OF ALLOYS AND COMPOUNDS. Volume: 947 (2023) - 2023 • 7725
Becken aus Beton in Entwässerungsanlagen nach ZTV-ING
Alonso Junghanns, M.T. and Breitenbücher, R. and Günther, M. and Haist, M. and Haus, J.
BETON- UND STAHLBETONBAU. Volume: 118 (2023) - 2023 • 7724
Evolving microstructures in relaxed continuum damage mechanics for the modeling of strain softening
Köhler, M. and Balzani, D.
JOURNAL OF THE MECHANICS AND PHYSICS OF SOLIDS. Volume: 173 (2023) - 2023 • 7723
On the role of potassium in Prussian blue analogue-derived Mn-Co catalysts applied in the CO hydrogenation to higher alcohols
Telaar, P. and Diehl, P. and Herrendorf, T. and Schaefer, S. and Kleist, W. and Muhler, M.
CATALYSIS SCIENCE AND TECHNOLOGY. Volume: 13 (2023) - 2023 • 7722
Influence of Support Material on the Structural Evolution of Copper during Electrochemical CO2 Reduction
Koh, E.S. and Geiger, S. and Gunnarson, A. and Imhof, T. and Meyer, G.M. and Paciok, P. and Etzold, B.J.M. and Rose, M. and Schüth, F. and Ledendecker, M.
CHEMELECTROCHEM. Volume: 10 (2023) - 2023 • 7721
Application of Prussian Blue Analogue-Derived Mn−Co Catalysts in the Hydrogenation of CO to Higher Alcohols
Telaar, P. and Schmidt, S. and Diehl, P. and Schwiderowski, P. and Muhler, M.
CHEMCATCHEM. Volume: 15 (2023) - 2023 • 7720
Fast Li-ion Storage and Dynamics in TiO2 Nanoparticle Clusters Probed by Smart Scanning Electrochemical Cell Microscopy
Tetteh, E.B. and Valavanis, D. and Daviddi, E. and Xu, X. and Santana Santos, C. and Ventosa, E. and Martín-Yerga, D. and Schuhmann, W. and Unwin, P.R.
ANGEWANDTE CHEMIE - INTERNATIONAL EDITION. Volume: 62 (2023) - 2023 • 7719
Semantic segmentation for fully automated macrofouling analysis on coatings after field exposure
Krause, L.M.K. and Manderfeld, E. and Gnutt, P. and Vogler, L. and Wassick, A. and Richard, K. and Rudolph, M. and Hunsucker, K.Z. and Swain, G.W. and Rosenhahn, B. and Rosenhahn, A.
BIOFOULING. Volume: 39 (2023) - 2023 • 7718
Stabilization of alkaline 5-HMF electrolytes via Cannizzaro reaction for the electrochemical oxidation to FDCA
Krebs, M.L. and Bodach, A. and Wang, C. and Schüth, F.
GREEN CHEMISTRY. Volume: 25 (2023) - 2023 • 7717
Comparison of the performance of a microwave plasma torch and a gliding arc plasma for hydrogen production via methane pyrolysis
Kreuznacht, S. and Purcel, M. and Böddeker, S. and Awakowicz, P. and Xia, W. and Muhler, M. and Böke, M. and Keudell, A. v.
PLASMA PROCESSES AND POLYMERS. Volume: 20 (2023) - 2023 • 7716
Local Maxima in Martensite Start Temperatures in the Transition Region between Lath and Plate Martensite in Fe-Ni Alloys
Thome, P. and Schneider, M. and Yardley, V.A. and Payton, E.J. and Eggeler, G.
MATERIALS. Volume: 16 (2023) - 2023 • 7715
Segregation-enhanced grain boundary embrittlement of recrystallised tungsten evidenced by site-specific microcantilever fracture
Tian, C. and Ma, Y. and Ghafarollahi, A. and Patil, P. and Dehm, G. and Bitzek, E. and Rasinski, M. and Best, J. P.
ACTA MATERIALIA. Volume: 259 (2023) - 2023 • 7714
TWO-SAMPLE TESTS FOR RELEVANT DIFFERENCES IN THE EIGENFUNCTIONS OF COVARIANCE OPERATORS
Aue, A. and Dette, H. and Rice, G.
STATISTICA SINICA. Volume: 33 (2023) - 2023 • 7713
Optical properties of Agx Cu1- x I alloy thin films
Krüger, E. and Seifert, M. and Gottschalch, V. and Krautscheid, H. and Schnohr, C. S. and Botti, S. and Grundmann, M. and Sturm, C.
AIP ADVANCES. Volume: 13 (2023) - 2023 • 7712
Damage Evolution and Microstructural Fracture Mechanisms Related to Volume Fraction and Martensite Distribution on Dual-Phase Steels
Avendaño-Rodríguez, D.F. and Rodriguez-Baracaldo, R. and Weber, S. and Mujica-Roncery, L.
STEEL RESEARCH INTERNATIONAL. Volume: (2023) - 2023 • 7711
The Rapid Mechanochemical Synthesis of Microporous Covalent Triazine Networks: Elucidating the Role of Chlorinated Linkers by a Solvent-Free Approach
Krusenbaum, A. and Kraus, F.J.L. and Hutsch, S. and Grätz, S. and Höfler, M.V. and Gutmann, T. and Borchardt, L.
ADVANCED SUSTAINABLE SYSTEMS. Volume: (2023) - 2023 • 7710
Substrate-Gated Transformation of a Pre-Catalyst into an Iron-Hydride Intermediate [(NO)2(CO)Fe(μ-H)Fe(CO)(NO)2]− for Catalytic Dehydrogenation of Dimethylamine Borane
Tseng, Y.-T. and Pelmenschikov, V. and Iffland-Mühlhaus, L. and Calabrese, D. and Chang, Y.-C. and Laun, K. and Pao, C.-W. and Sergueev, I. and Yoda, Y. and Liaw, W.-F. and Chen, C.-H. and Hsu, I.-J. and Apfel, U.-P. and Caserta, G. and Lauterbach, L. and Lu, T.-T.
INORGANIC CHEMISTRY. Volume: 62 (2023) - 2023 • 7709
Unveiling colloidal nanoparticle properties and interactions at a single entity level
Azimzadeh Sani, M. and Tschulik, K.
CURRENT OPINION IN ELECTROCHEMISTRY. Volume: 37 (2023) - 2023 • 7708
Chemical evolution of polycrystalline cementite (Fe3C) during single-pass sliding wear: An investigation by surface spectroscopy
Tsybenko, H. and Prabhakar, J.M. and Rohwerder, M. and Dehm, G. and Brinckmann, S.
ACTA MATERIALIA. Volume: 245 (2023) - 2023 • 7707
Phase-Field Study of the History-Effect of Remelted Microstructures on Nucleation During Additive Manufacturing of Ni-Based Superalloys
Uddagiri, M. and Shchyglo, O. and Steinbach, I. and Wahlmann, B. and Koerner, C.
METALLURGICAL AND MATERIALS TRANSACTIONS A: PHYSICAL METALLURGY AND MATERIALS SCIENCE. Volume: 54 (2023) - 2023 • 7706
Ultrafast Surface-Specific Spectroscopy of Water at a Photoexcited TiO2 Model Water-Splitting Photocatalyst
Backus, E. H. G. and Hosseinpour, S. and Ramanan, C. and Sun, S. and Schlegel, S. J. and Zelenka, M. and Jia, X. and Gebhard, M. and Devi, A. and Wang, H. I. and Bonn, M.
ANGEWANDTE CHEMIE - INTERNATIONAL EDITION. Volume: (2023) - 2023 • 7705
Chemo-mechanical modeling of smooth muscle cell activation for the simulation of arterial walls under changing blood pressure
Uhlmann, K. and Balzani, D.
BIOMECHANICS AND MODELING IN MECHANOBIOLOGY. Volume: (2023) - 2023 • 7704
The Regioselective Solid-State Photo-Mechanochemical Synthesis of Nanographenes with UV light
Baier, D.M. and Spula, C. and Fanenstich, S. and Grätz, S. and Borchardt, L.
ANGEWANDTE CHEMIE - INTERNATIONAL EDITION. Volume: (2023) - 2023 • 7703
Electrooxidation of the Glycerol Derivative Solketal over Cu−Co Hydroxycarbonates to Enable the Synthesis of Glyceric Acid
Kumari, B. and Braun, M. and Cychy, S. and Sanjuán, I. and Behrendt, G. and Behrens, M. and Muhler, M. and Andronescu, C.
CHEMELECTROCHEM. Volume: (2023) - 2023 • 7702
The Mechanochemical Fries Rearrangement: Manipulating Isomer Ratios in the Synthesis of p-Hydroxyacetophenone at Different Scales**
Baier, D.M. and Rensch, T. and Bergheim, K. and Pietryga, V. and Grätz, S. and Borchardt, L.
CHEMISTRY - A EUROPEAN JOURNAL. Volume: (2023) - 2023 • 7701
Phase-Field Simulation of Texture Evolution in Magmatic Rocks
Kundin, J. and Steinbach, I. and Chakraborty, S.
JOURNAL OF GEOPHYSICAL RESEARCH: SOLID EARTH. Volume: 128 (2023) - 2023 • 7700
Crack healing mechanisms in atmospheric plasma sprayed Yb-silicate coatings during post-process heat treatment
Bakan, E. and Vaßen, R.
JOURNAL OF THE EUROPEAN CERAMIC SOCIETY. Volume: 43 (2023) - 2023 • 7699
Off-stoichiometric softening and polytypic transformations in the plastic deformation of the C14 Fe2Nb Laves phase
Ladines, A.N. and Drautz, R. and Hammerschmidt, T.
ACTA MATERIALIA. Volume: 260 (2023) - 2023 • 7698
Metastable to stable phase transformation in atmospheric plasma sprayed Yb-silicate coating during post-heat treatment
Bakan, E. and Sohn, Y.J. and Vaßen, R.
SCRIPTA MATERIALIA. Volume: 225 (2023) - 2023 • 7697
Crystal Plane-Related Oxygen-Evolution Activity of Single Hexagonal Co3O4 Spinel Particles
Varhade, S. and Tetteh, E.B. and Saddeler, S. and Schumacher, S. and Aiyappa, H.B. and Bendt, G. and Schulz, S. and Andronescu, C. and Schuhmann, W.
CHEMISTRY - A EUROPEAN JOURNAL. Volume: 29 (2023) - 2023 • 7696
Atmospheric Plasma Spraying of Different MgO/Al2O3 Feedstocks
Vaßen, R. and Kalfhaus, T. and Vorkötter, C. and Sohn, Y.J. and Conze, S. and Berger, L.-M.
JOURNAL OF THERMAL SPRAY TECHNOLOGY. Volume: 32 (2023) - 2023 • 7695
A review of the metastable omega phase in beta titanium alloys: the phase transformation mechanisms and its effect on mechanical properties
Ballor, J. and Li, T. and Prima, F. and Boehlert, C. J. and Devaraj, A.
INTERNATIONAL MATERIALS REVIEWS. Volume: 68 (2023) - 2023 • 7694
Author Correction: Dual phase patterning during a congruent grain boundary phase transition in elemental copper (Nature Communications, (2022), 13, 1, (3331), 10.1038/s41467-022-30922-3)
Langenohl, L. and Brink, T. and Freitas, R. and Frolov, T. and Dehm, G. and Liebscher, C.H.
NATURE COMMUNICATIONS. Volume: 14 (2023) - 2023 • 7693
Stochastic modelling of continuous glass-fibre reinforced plastics–considering material uncertainty in microscale simulations
Vinot, M. and Liebold, C. and Usta, T. and Holzapfel, M. and Toso, N. and Voggenreiter, H.
JOURNAL OF COMPOSITE MATERIALS. Volume: 57 (2023) - 2023 • 7692
Atomic-resolution observations of silver segregation in a [111] tilt grain boundary in copper
Langenohl, L. and Brink, T. and Richter, G. and Dehm, G. and Liebscher, C. H.
PHYSICAL REVIEW B. Volume: 107 (2023) - 2023 • 7691
Differentiating between Acidic and Basic Surface Hydroxyls on Metal Oxides by Fluoride Substitution: A Case Study on Blue TiO2 from Laser Defect Engineering
Lau, K. and Niemann, F. and Abdiaziz, K. and Heidelmann, M. and Yang, Y. and Tong, Y. and Fechtelkord, M. and Schmidt, T.C. and Schnegg, A. and Campen, R.K. and Peng, B. and Muhler, M. and Reichenberger, S. and Barcikowski, S.
ANGEWANDTE CHEMIE - INTERNATIONAL EDITION. Volume: 62 (2023) - 2023 • 7690
Light-driven urea oxidation for a wearable artificial kidney
Vollenbroek, J.C. and Rodriguez, A.P. and Mei, B.T. and Mul, G. and Verhaar, M.C. and Odijk, M. and Gerritsen, K.G.F.
CATALYSIS TODAY. Volume: 419 (2023) - 2023 • 7689
Microscale Combinatorial Libraries for the Discovery of High-Entropy Materials
Banko, L. and Tetteh, E.B. and Kostka, A. and Piotrowiak, T.H. and Krysiak, O.A. and Hagemann, U. and Andronescu, C. and Schuhmann, W. and Ludwig, A.
ADVANCED MATERIALS. Volume: (2023) - 2023 • 7688
A Novel Hierarchical Recursive Nonsingular Terminal Sliding Mode Control for Inverted Pendulum
Le, H. D. and Nestorović, T.
ACTUATORS. Volume: 12 (2023) - 2023 • 7687
Oxide- and Silicate-Water Interfaces and Their Roles in Technology and the Environment
Bañuelos, J.L. and Borguet, E. and Brown, G.E. and Cygan, R.T. and Deyoreo, J.J. and Dove, P.M. and Gaigeot, M.-P. and Geiger, F.M. and Gibbs, J.M. and Grassian, V.H. and Ilgen, A.G. and Jun, Y.-S. and Kabengi, N. and Katz, L. and Kubicki, J.D. and Lützenkirchen, J. and Putnis, C.V. and Remsing, R.C. and Rosso, K.M. and Rother, G. and Sulpizi, M. and Villalobos, M. and Zhang, H.
CHEMICAL REVIEWS. Volume: 123 (2023) - 2023 • 7686
Adaptive Proportional Integral Derivative Nonsingular Dual Terminal Sliding Mode Control for Robotic Manipulators
Le, H. D. and Nestorović, T.
DYNAMICS. Volume: 3 (2023) - 2023 • 7685
Effects of stacking fault energy and temperature on grain boundary strengthening, intrinsic lattice strength and deformation mechanisms in CrMnFeCoNi high-entropy alloys with different Cr/Ni ratios
Wagner, C. and Laplanche, G.
ACTA MATERIALIA. Volume: 244 (2023) - 2023 • 7684
Design, Simulation, and Characterization of MEMS-Based Slot Waveguides
Barowski, J. and Schmitt, L. and Kother, K. and Hoffmann, M.
IEEE TRANSACTIONS ON MICROWAVE THEORY AND TECHNIQUES. Volume: (2023) - 2023 • 7683
Electronic correlations and superconducting instability in La3Ni2 O7 under high pressure
Lechermann, F. and Gondolf, J. and Bötzel, S. and Eremin, I. M.
PHYSICAL REVIEW B. Volume: 108 (2023) - 2023 • 7682
Effect of grain size on critical twinning stress and work hardening behavior in the equiatomic CrMnFeCoNi high-entropy alloy
Wagner, C. and Laplanche, G.
INTERNATIONAL JOURNAL OF PLASTICITY. Volume: 166 (2023) - 2023 • 7681
Blind competition on the numerical simulation of continuous shallow steel-fiber reinforced concrete beams failing in bending
Barros, J. and Sanz, B. and Filho, M. and Figueiredo, F. and Kabele, P. and Yu, R.C. and Meschke, G. and Planas, J. and Cunha, V. and Caggiano, A. and Ozyurt, N. and Gouveia, V. and van den Bos, A. and Poveda, E. and Gal, E. and Cervenka, J. and Neu, G.E. and Rossi, P. and Dias-da-Costa, D. and Juhasz, P.K. and Cendon, D. and Ruiz, G. and Valente, T.
STRUCTURAL CONCRETE. Volume: (2023) - 2023 • 7680
Electronic stripe patterns near the fermi level of tetragonal Fe(Se,S)
Walker, M. and Scott, K. and Boyle, T.J. and Byland, J.K. and Bötzel, S. and Zhao, Z. and Day, R.P. and Zhdanovich, S. and Gorovikov, S. and Pedersen, T.M. and Klavins, P. and Damascelli, A. and Eremin, I.M. and Gozar, A. and Taufour, V. and da Silva Neto, E.H.
NPJ QUANTUM MATERIALS. Volume: 8 (2023) - 2023 • 7679
Thermal and Chemical Evolution of an Archean Collision Zone: Insights from P-T-t History of Mafic Granulites from the Coorg Block, S. India
Basak, S. and Hasenstab, E. and Bhowmik, S. K. and Gerdes, A. and Dasgupta, S. and Münker, C. and Kumar, Gr. R. and Chakraborty, S.
JOURNAL OF PETROLOGY. Volume: 64 (2023) - 2023 • 7678
Evidence of Sulfur Non-Innocence in [CoII(dithiacyclam)]2+-Mediated Catalytic Oxygen Reduction Reactions
Battistella, B. and Iffland-Mühlhaus, L. and Schütze, M. and Cula, B. and Kuhlmann, U. and Dau, H. and Hildebrandt, P. and Lohmiller, T. and Mebs, S. and Apfel, U.-P. and Ray, K.
ANGEWANDTE CHEMIE - INTERNATIONAL EDITION. Volume: 62 (2023) - 2023 • 7677
Symmetry-based computational search for novel binary and ternary 2D materials
Wang, H. and Schmidt, J. and Marques, M. A. L. and Wirtz, L. and Romero, A. H.
2D MATERIALS. Volume: 10 (2023) - 2023 • 7676
Ultrashort-Pulsed-Laser Annealing of Amorphous Atomic-Layer-Deposited MoS2 Films
Becher, M. J. M. J. and Jagosz, J. and Neubieser, R. and Wree, J. and Devi, A. and Michel, M. and Bock, C. and Gurevich, E. L. and Ostendorf, A.
ADVANCED ENGINEERING MATERIALS. Volume: 25 (2023) - 2023 • 7675
Two-phase model for the excavation analysis in partially saturated soft soils using the particle finite element method
Leon Bal, A.R. and Meschke, G.
INTERNATIONAL JOURNAL FOR NUMERICAL AND ANALYTICAL METHODS IN GEOMECHANICS. Volume: 47 (2023) - 2023 • 7674
Promoting hydrogen evolution reaction with a sulfonic proton relay
Wang, N. and Zhang, X.-P. and Han, J. and Lei, H. and Zhang, Q. and Zhang, H. and Zhang, W. and Apfel, U.-P. and Cao, R.
CHINESE JOURNAL OF CATALYSIS. Volume: 45 (2023) - 2023 • 7673
Wiring of bilirubin oxidases with redox polymers on gas diffusion electrodes for increased stability of self-powered biofuel cells-based glucose sensing
Becker, J.M. and Lielpetere, A. and Szczesny, J. and Bichon, S. and Gounel, S. and Mano, N. and Schuhmann, W.
BIOELECTROCHEMISTRY. Volume: 149 (2023) - 2023 • 7672
A comparative study of in-situ alloying in laser powder bed fusion for the stainless steel X2CrNiMoN20-10-3
Becker, L. and Lentz, J. and Benito, S. and Cui, C. and Ellendt, N. and Fechte-Heinen, R. and Weber, S.
JOURNAL OF MATERIALS PROCESSING TECHNOLOGY. Volume: 318 (2023) - 2023 • 7671
Local measurement of geometrically necessary dislocation densities and their strengthening effect in ultra-high deformed pearlite
Li, Y. and Goto, S. and Kostka, A. and Herbig, M.
MATERIALS CHARACTERIZATION. Volume: 203 (2023) - 2023 • 7670
Influence of spin fluctuations on structural phase transitions of iron
Wang, N. and Hammerschmidt, T. and Hickel, T. and Rogal, J. and Drautz, R.
PHYSICAL REVIEW B. Volume: 107 (2023) - 2023 • 7669
A new approach to the powder metallurgical production of high nitrogen steels by integrated diffusion alloying in a hot isostatic press
Becker, L. and Radtke, F. and Lentz, J. and Herzog, S. and Broeckmann, C. and Weber, S.
MATERIALS LETTERS. Volume: 352 (2023) - 2023 • 7668
Local measurement of geometrically necessary dislocation densities and their strengthening effect in ultra-high deformed pearlite
Li, Y. and Goto, S. and Kostka, A. and Herbig, M.
MATERIALS CHARACTERIZATION. Volume: 203 (2023) - 2023 • 7667
In Vivo Assembly of Photosystem I-Hydrogenase Chimera for In Vitro PhotoH2 Production
Wang, P. and Frank, A. and Appel, J. and Boehm, M. and Strabel, N. and Nowaczyk, M.M. and Schuhmann, W. and Conzuelo, F. and Gutekunst, K.
ADVANCED ENERGY MATERIALS. Volume: 13 (2023) - 2023 • 7666
Influence of annealing time on the microstructure and properties of additively manufactured X2CrNiMoN25–7–4 duplex stainless steel: Experiment and simulation
Becker, L. and Boes, J. and Lentz, J. and Cui, C. and Steinbacher, M. and Li, Y. and Fechte-Heinen, R. and Theisen, W. and Weber, S.
MATERIALIA. Volume: 28 (2023) - 2023 • 7665
Ductile 2-GPa steels with hierarchical substructure
Li, Y. and Yuan, G. and Li, L. and Kang, J. and Yan, F. and Du, P. and Raabe, D. and Wang, G.
SCIENCE. Volume: 379 (2023) - 2023 • 7664
A Novel Electrode for Value-Generating Anode Reactions in Water Electrolyzers at Industrial Current Densities
Wang, C. and Wu, Y. and Bodach, A. and Krebs, M.L. and Schuhmann, W. and Schüth, F.
ANGEWANDTE CHEMIE - INTERNATIONAL EDITION. Volume: 62 (2023) - 2023 • 7663
A biomimetic assembly of folded photosystem I monolayers for an improved light utilization in biophotovoltaic devices
Wang, P. and Frank, A. and Zhao, F. and Nowaczyk, M.M. and Conzuelo, F. and Schuhmann, W.
BIOELECTROCHEMISTRY. Volume: 149 (2023) - 2023 • 7662
Atomic cluster expansion for Pt–Rh catalysts: From ab initio to the simulation of nanoclusters in few steps
Liang, Y. and Mrovec, M. and Lysogorskiy, Y. and Vega-Paredes, M. and Scheu, C. and Drautz, R.
JOURNAL OF MATERIALS RESEARCH. Volume: 38 (2023) - 2023 • 7661
Ag-induced Phase Transition of Bi2O3 Nanofibers for Enhanced Energy Conversion Efficiency towards Formate in CO2 Electroreduction
Wang, X. and He, W. and Shi, J. and Junqueira, J.R.C. and Zhang, J. and Dieckhöfer, S. and Seisel, S. and Das, D. and Schuhmann, W.
CHEMISTRY - AN ASIAN JOURNAL. Volume: 18 (2023) - 2023 • 7660
Cross-Linkable Polymer-Based Multi-layers for Protecting Electrochemical Glucose Biosensors against Uric Acid, Ascorbic Acid, and Biofouling Interferences
Lielpetere, A. and Jayakumar, K. and Leech, D. and Schuhmann, W.
ACS SENSORS. Volume: 8 (2023) - 2023 • 7659
Scrutinizing Intrinsic Oxygen Reduction Reaction Activity of a Fe−N−C Catalyst via Scanning Electrochemical Cell Microscopy
Limani, N. and Batsa Tetteh, E. and Kim, M. and Quast, T. and Scorsone, E. and Jousselme, B. and Schuhmann, W. and Cornut, R.
CHEMELECTROCHEM. Volume: 10 (2023) - 2023 • 7658
Assessing the Environmental Impact of Atomic Layer Deposition (ALD) Processes and Pathways to Lower It
Weber, M. and Boysen, N. and Graniel, O. and Sekkat, A. and Dussarrat, C. and Wiff, P. and Devi, A. and Muñoz-Rojas, D.
ACS MATERIALS AU. Volume: 3 (2023) - 2023 • 7657
Erratum: Band lineup at hexagonal SixGe1-x/SiyGe1-y alloy interfaces (Physical Review B (2022) 106 (085303) DOI: 10.1103/PhysRevB.106.085303)
Belabbes, A. and Botti, S. and Bechstedt, F.
PHYSICAL REVIEW B. Volume: 107 (2023) - 2023 • 7656
Polarization dynamics in spin-VCSELs with integrated surface grating for high birefringence splitting
Lindemann, M. and Jung, N. and Manrique-Nieto, N. and Pusch, T. and Michalzik, R. and Gerhardt, N. C. and Hofmann, M. R.
ELECTRONICS LETTERS. Volume: 59 (2023) - 2023 • 7655
Modifizierte Prüfverfahren zur baustellengerechten Bestimmung der Klebrigkeit von Frischbetonen
Weiß, M. and Przondziono, R. and Breitenbücher, R.
BETON- UND STAHLBETONBAU. Volume: 118 (2023) - 2023 • 7654
Fe2O3-graphitic carbon nitride nanocomposites analyzed by XPS
Benedet, M. and Barreca, D. and Rizzi, G. A. and Maccato, C. and Wree, J. and Devi, A. and Gasparotto, A.
SURFACE SCIENCE SPECTRA. Volume: 30 (2023) - 2023 • 7653
Local enhancement of electron heating and neutral species generation in radio-frequency micro-atmospheric pressure plasma jets: the effects of structured electrode topologies
Liu, Y. and Vass, M. and Hübner, G. and Schulenberg, D. and Hemke, T. and Bischoff, L. and Chur, S. and Steuer, D. and Golda, J. and Böke, M. and Schulze, J. and Korolov, I. and Mussenbrock, T.
PLASMA SOURCES SCIENCE AND TECHNOLOGY. Volume: 32 (2023) - 2023 • 7652
Preventing Hydrogen Embrittlement: The Role of Barrier Coatings for the Hydrogen Economy
Wetegrove, M. and Duarte, M. J. and Taube, K. and Rohloff, M. and Gopalan, H. and Scheu, C. and Dehm, G. and Kruth, A.
HYDROGEN (SWITZERLAND). Volume: 4 (2023) - 2023 • 7651
Advances in photo-assisted seawater splitting promoted by green iron oxide-carbon nitride photoelectrocatalysts
Benedet, M. and Rizzi, G. A. and Lebedev, O. I. and Roddatis, V. and Sada, C. and Wree, J. and Devi, A. and Maccato, C. and Gasparotto, A. and Barreca, D.
JOURNAL OF MATERIALS CHEMISTRY A. Volume: 11 (2023) - 2023 • 7650
The martensitic transition pathway in steel
Liu, T. and Liang, L. and Raabe, D. and Dai, L.
JOURNAL OF MATERIALS SCIENCE AND TECHNOLOGY. Volume: 134 (2023) - 2023 • 7649
Antiferromagnetic order and its interplay with superconductivity in CaK(Fe 1 - X Mnx)4As4
Wilde, J.M. and Sapkota, A. and Ding, Q.-P. and Xu, M. and Tian, W. and Bud'Ko, S.L. and Furukawa, Y. and Kreyssig, A. and Canfield, P.C.
JOURNAL OF PHYSICS CONDENSED MATTER. Volume: 35 (2023) - 2023 • 7648
Statistical characterization of segregation-driven inhomogeneities in metallic microstructures employing fast first-order variograms
Benito, S. and Egels, G. and Hartmaier, A. and Weber, S.
MATERIALS TODAY COMMUNICATIONS. Volume: 34 (2023) - 2023 • 7647
Finite strain crystal plasticity-phase field modeling of twin, dislocation, and grain boundary interaction in hexagonal materials
Liu, C. and Roters, F. and Raabe, D.
ACTA MATERIALIA. Volume: 242 (2023) - 2023 • 7646
CVD Grown Tungsten Oxide for Low Temperature Hydrogen Sensing: Tuning Surface Characteristics via Materials Processing for Sensing Applications
Wilken, M. and Ciftyürek, E. and Cwik, S. and Mai, L. and Mallick, B. and Rogalla, D. and Schierbaum, K. and Devi, A.
SMALL. Volume: 19 (2023) - 2023 • 7645
A New Approach to the Optimization of the Austenite Stability of Metastable Austenitic Stainless Steels
Berger, A. and Egels, G. and Fussik, R. and Benito, S.M. and Weber, S.
JOURNAL OF MATERIALS ENGINEERING AND PERFORMANCE. Volume: (2023) - 2023 • 7644
Considerably Increased Dynamics of CO-Water Complexes over CO and Water Alone
Bertram, C. and Zaum, C. and Fang, W. and Michaelides, A. and Morgenstern, K.
NANO LETTERS. Volume: 23 (2023) - 2023 • 7643
Super-Solidus Hot Isostatic Pressing Heat Treatments for Advanced Single Crystal Ni-Base Superalloys
Lopez-Galilea, I. and Hecker, L. and Epishin, A. and Bürger, D. and Ruttert, B. and Thome, P. and Weber, S. and Theisen, W.
METALLURGICAL AND MATERIALS TRANSACTIONS A: PHYSICAL METALLURGY AND MATERIALS SCIENCE. Volume: 54 (2023) - 2023 • 7642
Bridging the Gap: Assessing Material Performance of Laboratory Specimens and Concrete Structures
Lozano-Valcarcel, J. M. and Ov, D. and Kränkel, T. and Gehlen, C. and Breitenbücher, R.
MATERIALS. Volume: 16 (2023) - 2023 • 7641
Durability of Concrete: Assessing the Performance Achieved in the Laboratory and on the Construction Site
Lozano-Valcarcel, J. M. and Ov, D. and Kränkel, T. and Gehlen, C. and Breitenbücher, R.
LECTURE NOTES IN CIVIL ENGINEERING. Volume: 349 LNCE (2023) - 2023 • 7640
Cooperative deformation mechanisms in a fatigued CoCrNi multi-principal element alloy: A case of low stacking fault energy
Lu, K. and Chauhan, A. and Litvinov, D. and Schneider, M. and Laplanche, G. and Aktaa, J.
JOURNAL OF THE MECHANICS AND PHYSICS OF SOLIDS. Volume: 180 (2023) - 2023 • 7639
A novel microwall sliding test uncovering the origin of grain refined tribolayers
Xia, W. and Patil, P.P. and Liu, C. and Dehm, G. and Brinckmann, S.
ACTA MATERIALIA. Volume: 246 (2023) - 2023 • 7638
Advancing strength and counteracting embrittlement by displacive transformation in heterogeneous high-entropy alloys containing sigma phase
Lu, W. and Guo, W. and Wang, Z. and Li, J. and An, F. and Dehm, G. and Raabe, D. and Liebscher, C.H. and Li, Z.
ACTA MATERIALIA. Volume: 246 (2023) - 2023 • 7637
Complementary spectroscopic and electrochemical analysis of the sealing of micropores in hexamethyldisilazane plasma polymer films by Al2O3 atomic layer deposition
Xie, X. and Zanders, D. and Preischel, F. and de los Arcos, T. and Devi, A. and Grundmeier, G.
SURFACE AND INTERFACE ANALYSIS. Volume: 55 (2023) - 2023 • 7636
Role of Ag segregation on microscale strengthening and slip transmission in an asymmetric Σ5 copper grain boundary
Bhat, M. K. and Sukumar, P. T. and Langenohl, L. and Best, J. P. and Dehm, G.
ACTA MATERIALIA. Volume: 255 (2023) - 2023 • 7635
Linking Composition, Structure and Thickness of CoOOH layers to Oxygen Evolution Reaction Activity by Correlative Microscopy
Luan, C. and Angona, J. and Bala Krishnan, A. and Corva, M. and Hosseini, P. and Heidelmann, M. and Hagemann, U. and Batsa Tetteh, E. and Schuhmann, W. and Tschulik, K. and Li, T.
ANGEWANDTE CHEMIE - INTERNATIONAL EDITION. Volume: 62 (2023) - 2023 • 7634
Atomic-Scale Insights into Morphological, Structural, and Compositional Evolution of CoOOH during Oxygen Evolution Reaction
Luan, C. and Corva, M. and Hagemann, U. and Wang, H. and Heidelmann, M. and Tschulik, K. and Li, T.
ACS CATALYSIS. Volume: 13 (2023) - 2023 • 7633
Transfer learning based physics-informed neural networks for solving inverse problems in engineering structures under different loading scenarios
Xu, C. and Cao, B.T. and Yuan, Y. and Meschke, G.
COMPUTER METHODS IN APPLIED MECHANICS AND ENGINEERING. Volume: 405 (2023) - 2023 • 7632
Corrigendum to “Data Mining in Urology: Understanding Real-world Treatment Pathways for Lower Urinary Tract Systems via Exploration of Big Data” [Eur Urol Focus 2022;8:391–3] (European Urology Focus (2022) 8(2) (391–393), (S2405456922000736), (10.1016/j.euf.2022.03.019))
Binder, N. and Dette, H. and Franz, J. and Zöller, D. and Suarez-Ibarrola, R. and Gratzke, C. and Binder, H. and Miernik, A.
EUROPEAN UROLOGY FOCUS. Volume: 9 (2023) - 2023 • 7631
Decoupling the electrical resistivity contribution of grain boundaries in dilute Fe-alloyed Cu thin films
Bishara, H. and Langenohl, L. and Zhou, X. and Gault, B. and Best, J.P. and Dehm, G.
SCRIPTA MATERIALIA. Volume: 230 (2023) - 2023 • 7630
Selectivity of Laser-Induced versus IET-Induced Carbene Formation from Methoxydiazofluorene on Ag(111)
Lucht, K. and Ulrich, I. and Sander, W. and Morgenstern, K.
JOURNAL OF PHYSICAL CHEMISTRY C. Volume: 127 (2023) - 2023 • 7629
An experimental study of proton implantation in olivine
Bissbort, T. and Jiang, Q. and Becker, H. and Foteinou, V. and Chakraborty, S.
PHYSICS AND CHEMISTRY OF MINERALS. Volume: 50 (2023) - 2023 • 7628
A generic compact and stochastic model for non-filamentary analog resistive switching devices
Yarragolla, S. and Hemke, T. and Mussenbrock, T.
2023 12TH INTERNATIONAL CONFERENCE ON MODERN CIRCUITS AND SYSTEMS TECHNOLOGIES, MOCAST 2023 - PROCEEDINGS. Volume: (2023) - 2023 • 7627
Interactions Between Flow Fields Induced by Surface Dielectric Barrier Discharge Arrays
Böddecker, A. and Passmann, M. and Wilczek, S. and Schücke, L. and Korolov, I. and Skoda, R. and Mussenbrock, T. and Gibson, A. R. and Awakowicz, P.
PLASMA CHEMISTRY AND PLASMA PROCESSING. Volume: 43 (2023) - 2023 • 7626
Active learning strategies for atomic cluster expansion models
Lysogorskiy, Y. and Bochkarev, A. and Mrovec, M. and Drautz, R.
PHYSICAL REVIEW MATERIALS. Volume: 7 (2023) - 2023 • 7625
Reducing Iron Oxide with Ammonia: A Sustainable Path to Green Steel
Ma, Y. and Bae, J.W. and Kim, S.-H. and Jovičević-Klug, M. and Li, K. and Vogel, D. and Ponge, D. and Rohwerder, M. and Gault, B. and Raabe, D.
ADVANCED SCIENCE. Volume: (2023) - 2023 • 7624
Green coal substitutes for boilers through hydrothermal carbonization of biomass: pyrolysis and combustion behavior
Böttger, J. and Eckhard, T. and Pflieger, C. and Senneca, O. and Muhler, M. and Cerciello, F.
FUEL. Volume: 344 (2023) - 2023 • 7623
Ensemble averages of ab initio optical, transport, and thermoelectric properties of hexagonal SixGe1-x alloys
Borlido, P. and Bechstedt, F. and Botti, S. and Rödl, C.
PHYSICAL REVIEW MATERIALS. Volume: 7 (2023) - 2023 • 7622
Carbon monoxide separation: past, present and future
Ma, X. and Albertsma, J. and Gabriels, D. and Horst, R. and Polat, S. and Snoeks, C. and Kapteijn, F. and Eral, H.B. and Vermaas, D.A. and Mei, B. and de Beer, S. and van der Veen, M.A.
CHEMICAL SOCIETY REVIEWS. Volume: (2023) - 2023 • 7621
Liquid injection field desorption/ionization as a powerful tool to characterize volatile, labile, and reactive metal–organic complexes
Boysen, N. and Devi, A.
EUROPEAN JOURNAL OF MASS SPECTROMETRY. Volume: 29 (2023) - 2023 • 7620
The impact of plasma enhancement on the deposition of carbon-containing zirconia films by metalorganic chemical vapor deposition
Maaß, P. A. and Bedarev, V. and Chauvet, L. and Prenzel, M. and Glauber, J. and Devi, A. and Böke, M. and von Keudell, A.
PLASMA PROCESSES AND POLYMERS. Volume: 20 (2023) - 2023 • 7619
Surface modifications of aluminium and aluminium oxide induced by a treatment with a He-plasma jet and plasma electrolytic oxidation
Bracht, V. and Gembus, J.-L. and Bibinov, N. and Awakowicz, P.
JOURNAL OF PHYSICS D: APPLIED PHYSICS. Volume: 56 (2023) - 2023 • 7618
Microstructure and physical properties of dual-phase soft magnetic Fe-Co-Ti-Ge alloys
Zhang, X. and Han, L. and Dehm, G. and Liebscher, C.H.
JOURNAL OF ALLOYS AND COMPOUNDS. Volume: 945 (2023) - 2023 • 7617
The behavior of cutting discs for mechanized tunneling under cyclic loading conditions
Brackmann, L. and Röttger, A. and Treppmann, M. and Weber, S.
TUNNELLING AND UNDERGROUND SPACE TECHNOLOGY. Volume: 137 (2023) - 2023 • 7616
Simulation of Reacting, Moving Granular Assemblies of Thermally Thick Particles by Discrete Element Method/Computational Fluid Dynamics
Mahiques, E.I. and Brömmer, M. and Wirtz, S. and van Wachem, B. and Scherer, V.
CHEMICAL ENGINEERING AND TECHNOLOGY. Volume: (2023) - 2023 • 7615
Single-entity Electrochemistry Unveils Dynamic Transformation during Tandem Catalysis of Cu2O and Co3O4 for Converting NO3− to NH3
Zhang, J. and He, W. and Quast, T. and Junqueira, J.R.C. and Saddeler, S. and Schulz, S. and Schuhmann, W.
ANGEWANDTE CHEMIE - INTERNATIONAL EDITION. Volume: 62 (2023) - 2023 • 7614
Thermal shock resistance of additively manufactured gas-permeable SiO2 ceramic structures for HIP-quench applications
Brackmann, L. and Liu, X. and Ruttert, B. and Weber, S.
JOURNAL OF THE EUROPEAN CERAMIC SOCIETY. Volume: 43 (2023) - 2023 • 7613
Advance Reconnaissance and Optimal Monitoring
Mahmoud, E. and Düllmann, J. and Heußne, L. and Hölter, R. and Lamert, A. and Miro, S. and Möller, T. and Musayev, K. and Riedel, C. and Schindler, S. and Trapp, M. and Alber, M. and Baitsch, M. and Friederich, W. and Hackl, K. and König, M. and Mark, P. and Nestorovic, T.
INTERACTION MODELING IN MECHANIZED TUNNELING. Volume: (2023) - 2023 • 7612
Electrochemical Oxidation of 5-Hydroxymethylfurfural on CeO2-Modified Co3O4 with Regulated Intermediate Adsorption and Promoted Charge Transfer
Zhao, G. and Hai, G. and Zhou, P. and Liu, Z. and Zhang, Y. and Peng, B. and Xia, W. and Huang, X. and Wang, G.
ADVANCED FUNCTIONAL MATERIALS. Volume: 33 (2023) - 2023 • 7611
Cobalt nickel boride as electrocatalyst for the oxidation of alcohols in alkaline media
Braun, M. and Chatwani, M. and Kumar, P. and Hao, Y. and Sanjuán, I. and Apostoleri, A.-A. and Brix, A.C. and Morales, D.M. and Hagemann, U. and Heidelmann, M. and Masa, J. and Schuhmann, W. and Andronescu, C.
JPHYS ENERGY. Volume: 5 (2023) - 2023 • 7610
Terahertz Sources and Receivers: From the Past to the Future
Makhlouf, S. and Cojocari, O. and Hofmann, M. and Nagatsuma, T. and Preu, S. and Weimann, N. and Hubers, H. and Stohr, A.
IEEE JOURNAL OF MICROWAVES. Volume: 3 (2023) - 2023 • 7609
Atomic motifs govern the decoration of grain boundaries by interstitial solutes
Zhou, X. and Ahmadian, A. and Gault, B. and Ophus, C. and Liebscher, C. H. and Dehm, G. and Raabe, D.
NATURE COMMUNICATIONS. Volume: 14 (2023) - 2023 • 7608
Visible light-induced surface grafting polymerization of perfluoropolyether brushes as marine low fouling materials
Manderfeld, E. and Balasubramaniam, A. and Özcan, O. and Anderson, C. and Finlay, J.A. and Clare, A.S. and Hunsucker, K. and Swain, G.W. and Rosenhahn, A.
POLYMER CHEMISTRY. Volume: 14 (2023) - 2023 • 7607
Effect of Pore Formation on Redox-Driven Phase Transformation
Zhou, X. and Bai, Y. and El-Zoka, A.A. and Kim, S.-H. and Ma, Y. and Liebscher, C.H. and Gault, B. and Mianroodi, J.R. and Dehm, G. and Raabe, D.
PHYSICAL REVIEW LETTERS. Volume: 13 (2023) - 2023 • 7606
Universality of grain boundary phases in fcc metals: Case study on high-angle [111] symmetric tilt grain boundaries
Brink, T. and Langenohl, L. and Bishara, H. and Dehm, G.
PHYSICAL REVIEW B. Volume: 107 (2023) - 2023 • 7605
Statistical inference for high-dimensional panel functional time series
Zhou, Z. and Dette, H.
JOURNAL OF THE ROYAL STATISTICAL SOCIETY. SERIES B: STATISTICAL METHODOLOGY. Volume: 85 (2023) - 2023 • 7604
A portmanteau-type test for detecting serial correlation in locally stationary functional time series
Bücher, A. and Dette, H. and Heinrichs, F.
STATISTICAL INFERENCE FOR STOCHASTIC PROCESSES. Volume: (2023) - 2023 • 7603
Concepts of Heterogeneously Catalyzed Liquid-Phase Oxidation of Cyclohexene with tert-Butyl Hydroperoxide, Hydrogen Peroxide and Molecular Oxygen
Büker, J. and Muhler, M. and Peng, B.
CHEMCATCHEM. Volume: 15 (2023) - 2023 • 7602
Exploring the Depths of Corrosion: A Novel GE-XANES Technique for Investigating Compositionally Complex Alloys
Cakir, C.T. and Piotrowiak, T. and Reinholz, U. and Ludwig, Al. and Emmerling, F. and Streli, C. and Guilherme Buzanich, A. and Radtke, M.
ANALYTICAL CHEMISTRY. Volume: 95 (2023) - 2023 • 7601
Effect of stiff substrates on enhancing the fracture resistance of Barium Titanate thin films
Mathews, N. G. and Lambai, A. and Mohanty, G. and Venkataramani, N. and Dehm, G. and Jaya, B. N.
MATERIALS AND DESIGN. Volume: 235 (2023) - 2023 • 7600
How does inherited zircon survive in partially molten mantle: Insights on modes of magma transport in the mantle from nanoscale melt-crystal interaction experiments
Cambeses, A. and Chakraborty, S. and Jöns, N. and Montero, P. and Bea, F.
EARTH AND PLANETARY SCIENCE LETTERS. Volume: 601 (2023) - 2023 • 7599
Electronic and Optical Properties of Alkaline Earth Metal Fluoride Crystals with the Inclusion of Many-Body Effects: A Comparative Study on Rutile MgF2 and Cubic SrF2
Cappellini, G. and Furthmüller, J. and Bechstedt, F. and Botti, S.
SYMMETRY. Volume: 15 (2023) - 2023 • 7598
Scalable Synthesis of Multi-Metal Electrocatalyst Powders and Electrodes and their Application for Oxygen Evolution and Water Splitting
Cechanaviciute, I.A. and Antony, R.P. and Krysiak, O.A. and Quast, T. and Dieckhöfer, S. and Saddeler, S. and Telaar, P. and Chen, Y.-T. and Muhler, M. and Schuhmann, W.
ANGEWANDTE CHEMIE - INTERNATIONAL EDITION. Volume: 62 (2023) - 2023 • 7597
Quantification of electronic and magnetoelastic mechanisms of first-order magnetic phase transitions from first principles: application to caloric effects in La(Fe x Si 1 − x )13
Mendive Tapia, E. and Patrick, C.E. and Hickel, T. and Neugebauer, J. and Staunton, J.B.
JPHYS ENERGY. Volume: 5 (2023) - 2023 • 7596
How Coherent and Semi-Coherent Interfaces Govern Dislocation Nucleation in Lamellar TiAl Alloys
Chauniyal, A. and Janisch, R.
ADVANCED ENGINEERING MATERIALS. Volume: 25 (2023) - 2023 • 7595
Non-oxidative dehydrogenation of methanol to formaldehyde over supported GaOx-based catalysts
Merko, M. and Delsing, S. and Busser, G.Wilma and Muhler, M.
JOURNAL OF CATALYSIS. Volume: 427 (2023) - 2023 • 7594
Analysis of the impact of carbon dissolution and energy transport on the flow in the hearth of an ironmaking blast furnace by transient CFD simulations
Merten, H. and Wirtz, S. and Bartusch, H. and Kaymak, Y. and Hojda, S. and Lin, R. and Scherer, V.
THERMAL SCIENCE AND ENGINEERING PROGRESS. Volume: 39 (2023) - 2023 • 7593
Accelerating Non-Empirical Structure Determination of Ziegler-Natta Catalysts with a High-Dimensional Neural Network Potential
Chikuma, H. and Takasao, G. and Wada, T. and Chammingkwan, P. and Behler, J. and Taniike, T.
JOURNAL OF PHYSICAL CHEMISTRY C. Volume: (2023) - 2023 • 7592
Computational modeling of FRC: From 3D printing to robust design
Meschke, G. and Neu, G. and Gudžulić, V. and Reinold, J. and Iskhakov, T.
CURRENT PERSPECTIVES AND NEW DIRECTIONS IN MECHANICS, MODELLING AND DESIGN OF STRUCTURAL SYSTEMS - PROCEEDINGS OF THE 8TH INTERNATIONAL CONFERENCE ON STRUCTURAL ENGINEERING, MECHANICS AND COMPUTATION, 2022. Volume: (2023) - 2023 • 7591
Combined modeling and experimental characterization of Mn segregation and spinodal decomposition along dislocation lines in Fe–Mn alloys
Mianroodi, J.R. and Shanthraj, P. and Kwiatkowski da Silva, A. and Svendsen, B. and Raabe, D.
ACTA MATERIALIA. Volume: 251 (2023) - 2023 • 7590
Special issue in memory of Prof. Konstantin B. Efetov: Editorial foreword
Chubukov, A. V. and Eremin, I. M. and Galitski, V. and Pepin, C.
ANNALS OF PHYSICS. Volume: 456 (2023) - 2023 • 7589
Synthesis, properties and catalytic performance of the novel, pseudo-spinel, multicomponent transition-metal selenides
Mikuła, A. and Dąbrowa, J. and Kubowicz, M. and Cieślak, J. and Lach, W. and Kożusznik, M. and Smialkowski, M. and Apfel, U.-P.
JOURNAL OF MATERIALS CHEMISTRY A. Volume: 11 (2023) - 2023 • 7588
Special issue in memory of Prof. Konstantin B. Efetov: Editorial foreword
Chubukov, A. V. and Eremin, I. M. and Galitski, V. and Pepin, C.
ANNALS OF PHYSICS. Volume: 456 (2023) - 2023 • 7587
Tailoring the electrocatalytic activity of multicomponent (Co,Fe,Ni)9S8−xSex pentlandite solid electrodes
Mikuła, A. and Kubowicz, M. and Mazurków, J. and Mars, K. and Smialkowski, M. and Apfel, U.-P. and Radecka, M.
JOURNAL OF MATERIALS CHEMISTRY A. Volume: (2023) - 2023 • 7586
Why standard electrokinetic analysis often fails for nanostructured electrodes - Reviewing inhomogeneous electroactivity
Cignoni, P. and Blanc, N. and Tschulik, K.
CURRENT OPINION IN ELECTROCHEMISTRY. Volume: 38 (2023) - 2023 • 7585
Isopropanol electro-oxidation on Pt-Ru-Ir: A journey from model thin-film libraries towards real electrocatalysts
Minichová, M. and Van Pham, C. and Xiao, B. and Savan, A. and Hutzler, A. and Körner, A. and Khalakhan, I. and Rodríguez, M.G. and Mangoufis-Giasin, I. and Briega-Martos, V. and Kormányos, A. and Katsounaros, I. and Mayrhofer, K.J.J. and Ludwig, Al. and Thiele, S. and Cherevko, S.
ELECTROCHIMICA ACTA. Volume: 444 (2023) - 2023 • 7584
Unusual crossover from Bardeen-Cooper-Schrieffer to Bose-Einstein-condensate superconductivity in iron chalcogenides
Mizukami, Y. and Haze, M. and Tanaka, O. and Matsuura, K. and Sano, D. and Böker, J. and Eremin, I. and Kasahara, S. and Matsuda, Y. and Shibauchi, T.
COMMUNICATIONS PHYSICS. Volume: 6 (2023) - 2023 • 7583
Accurate OCT-based diffuse adult-type glioma WHO grade 4 tissue classification using comprehensible texture feature analysis
Möller, J. and Popanda, E. and Aydın, N.H. and Welp, H. and Tischoff, I. and Brenner, C. and Schmieder, K. and Hofmann, M.R. and Miller, D.
BIOMEDICAL SIGNAL PROCESSING AND CONTROL. Volume: (2023) - 2023 • 7582
Enhanced activity and coke resistivity of NiCoFe nanoalloy catalyst in CO2 reforming of methane
Das, S. and Tillmann, L. and Xia, W. and Muhler, M.
JOURNAL OF THE INDIAN CHEMICAL SOCIETY. Volume: 100 (2023) - 2023 • 7581
The Influence of the Reaction Conditions on the Photocatalytic Gas-Phase Conversion of Methanol with Water Vapor over Pt/SrTiO3 in a Continuously Operated Flow Reactor
Deitermann, M. and Haver, Y. and Mei, B. T. and Muhler, M.
ADVANCED SUSTAINABLE SYSTEMS. Volume: (2023) - 2023 • 7580
The Influence of the Reaction Conditions on the Photocatalytic Gas-Phase Conversion of Methanol with Water Vapor over Pt/SrTiO3 in a Continuously Operated Flow Reactor
Deitermann, M. and Haver, Y. and Mei, B. T. and Muhler, M.
ADVANCED SUSTAINABLE SYSTEMS. Volume: (2023) - 2023 • 7579
Impact of in Situ Heat Treatment Effects during Laser-Based Powder Bed Fusion of 1.3343 High-Speed Steel with Preheating Temperatures up to 700 °C
Moritz, S. and Schwanekamp, T. and Reuber, M. and Lentz, J. and Boes, J. and Weber, S.
STEEL RESEARCH INTERNATIONAL. Volume: (2023) - 2023 • 7578
The Influence of the Reaction Conditions on the Photocatalytic Gas-Phase Conversion of Methanol with Water Vapor over Pt/SrTiO3 in a Continuously Operated Flow Reactor
Deitermann, M. and Haver, Y. and Mei, B. T. and Muhler, M.
ADVANCED SUSTAINABLE SYSTEMS. Volume: (2023) - 2023 • 7577
PECVD and PEALD on polymer substrates (part I): Fundamentals and analysis of plasma activation and thin film growth
de los Arcos, T. and Awakowicz, P. and Benedikt, J. and Biskup, B. and Böke, M. and Boysen, N. and Buschhaus, R. and Dahlmann, R. and Devi, A. and Gergs, T. and Jenderny, J. and von Keudell, A. and Kühne, T. D. and Kusmierz, S. and Müller, H. and Mussenbrock, T. and Trieschmann, J. and Zanders, D. and Zysk, F. and Grundmeier, G.
PLASMA PROCESSES AND POLYMERS. Volume: (2023) - 2023 • 7576
Investigating the microplastic behavior of hierarchical polycrystalline γ-TiAl microstructures
Motahari, S. and Chauniyal, A. and Janisch, R.
COMPUTATIONAL MATERIALS SCIENCE. Volume: 226 (2023) - 2023 • 7575
PECVD and PEALD on polymer substrates (part II): Understanding and tuning of barrier and membrane properties of thin films
de los Arcos, T. and Awakowicz, P. and Böke, M. and Boysen, N. and Brinkmann, R. P. and Dahlmann, R. and Devi, A. and Eremin, D. and Franke, J. and Gergs, T. and Jenderny, J. and Kemaneci, E. and Kühne, T. D. and Kusmierz, S. and Mussenbrock, T. and Rubner, J. and Trieschmann, J. and Wessling, M. and Xie, X. and Zanders, D. and Zysk, F. and Grundmeier, G.
PLASMA PROCESSES AND POLYMERS. Volume: (2023) - 2023 • 7574
Efficient identification of material parameters for an orthotropic hyperelastic nonlinear material model for textile membranes
Motevalli, M. and Balzani, D.
CONSTRUCTION AND BUILDING MATERIALS. Volume: 378 (2023) - 2023 • 7573
Size and Shape Exclusion in 2D Silicon Dioxide Membranes
Dementyev, P. and Khayya, N. and Zanders, D. and Ennen, I. and Devi, A. and Altman, E.I.
SMALL. Volume: 19 (2023) - 2023 • 7572
A high-purity gas–solid photoreactor for reliable and reproducible photocatalytic CO2 reduction measurements
Moustakas, N.G. and Klahn, M. and Mei, B.T. and Pougin, A. and Dilla, M. and Peppel, T. and Ristig, S. and Strunk, J.
HARDWAREX. Volume: 15 (2023) - 2023 • 7571
Prediction in regression models with continuous observations
Dette, H. and Pepelyshev, A. and Zhigljavsky, A.
STATISTICAL PAPERS. Volume: (2023) - 2023 • 7570
The effect of intraday periodicity on realized volatility measures
Dette, H. and Golosnoy, V. and Kellermann, J.
METRIKA. Volume: 86 (2023) - 2023 • 7569
Detecting relevant changes in the spatiotemporal mean function
Dette, H. and Quanz, P.
JOURNAL OF TIME SERIES ANALYSIS. Volume: 44 (2023) - 2023 • 7568
Damage Index Implementation for Structural Health Monitoring
Diab, A. and Nestorović, T.
MECHANISMS AND MACHINE SCIENCE. Volume: 125 MMS (2023) - 2023 • 7567
SERS Reveals the Presence of Au-O-O-H and Enhanced Catalytic Activity of Electrochemically Dealloyed AgAu Nanoparticles
Murke, S. and Wonner, K. and Alfarano, S.R. and Rurainsky, C. and Cignoni, P. and Tschulik, K. and Havenith, M.
JOURNAL OF PHYSICAL CHEMISTRY C. Volume: 127 (2023) - 2023 • 7566
Numerical Investigation of the Time-of-Flight and Wave Energy Dependent Hybrid Method for Structural Damage Detection
Diab, A. and Nestorović, T.
JOURNAL OF VIBRATION ENGINEERING AND TECHNOLOGIES. Volume: 11 (2023) - 2023 • 7565
Catalytic effects of molybdate and chromate–molybdate films deposited on platinum for efficient hydrogen evolution
Diaz-Morales, O. and Lindberg, A. and Smulders, V. and Anil, A. and Simic, N. and Wildlock, M. and Alvarez, G.S. and Mul, G. and Mei, B. and Cornell, A.
JOURNAL OF CHEMICAL TECHNOLOGY AND BIOTECHNOLOGY. Volume: 98 (2023) - 2023 • 7564
Fluctuations of the diagonal entries of a large sample precision matrix
Dörnemann, N. and Dette, H.
STATISTICS AND PROBABILITY LETTERS. Volume: 198 (2023) - 2023 • 7563
Influence of Microstructural Homogenization on the Localized Deformation Behavior of Single-Crystal Ni-Based Superalloy, CMSX-4
Nath, P. and Scholz, F. and Pfetzing, J. and Frenzel, J. and Eggeler, G. and Roy, S. and Sen, I.
METALLURGICAL AND MATERIALS TRANSACTIONS A: PHYSICAL METALLURGY AND MATERIALS SCIENCE. Volume: 54 (2023) - 2023 • 7562
Virtual Design Laboratory for Sustainable Fiber Reinforced Concrete Structures: From Discrete Fibers to Structural Optimization Under Uncertainty
Neu, G.E. and Gudžulic, V. and Meschke, G.
RILEM BOOKSERIES. Volume: 38 (2023) - 2023 • 7561
Deformation-induced homogenization of the multi-phase senary high-entropy alloy MoNbTaTiVZr processed by high-pressure torsion
Duan, C. and Kostka, A. and Li, X. and Peng, Z. and Kutlesa, P. and Pippan, R. and Werner, E.
MATERIALS SCIENCE AND ENGINEERING A. Volume: 871 (2023) - 2023 • 7560
The effects of catalyst conductivity and loading of dielectric surface structures on plasma dynamics in patterned dielectric barrier discharges
Neuroth, C. and Mujahid, Z. and Berger, B. and Oberste-Beulmann, C. and Oppotsch, T. and Zhang, Q. and Muhler, M. and Mussenbrock, T. and Korolov, I. and Schulze, J.
PLASMA SOURCES SCIENCE AND TECHNOLOGY. Volume: 32 (2023) - 2023 • 7559
Mineral effects on chemical and physical transformations of fast pyrolysis products of cellulose-based model fuels in N2 and CO2
Eckhard, T. and Pflieger, C. and Russo, C. and Freisewinkel, E. and Eisenbach, T. and Böttger, J. and Senneca, O. and Apicella, B. and Schiemann, M. and Span, R. and Scherer, V. and Muhler, M. and Cerciello, F.
FUEL. Volume: 340 (2023) - 2023 • 7558
The effects of catalyst conductivity and loading of dielectric surface structures on plasma dynamics in patterned dielectric barrier discharges
Neuroth, C. and Mujahid, Z. and Berger, B. and Oberste-Beulmann, C. and Oppotsch, T. and Zhang, Q. and Muhler, M. and Mussenbrock, T. and Korolov, I. and Schulze, J.
PLASMA SOURCES SCIENCE AND TECHNOLOGY. Volume: 32 (2023) - 2023 • 7557
The Catalytic Effect of Iron and Alkali and Alkaline Earth Metal Sulfates Loading Series on the Conversion of Cellulose-Derived Hydrochars and Chars
Eckhard, T. and Pflieger, C. and Böttger, J. and Telaar, P. and Cerciello, F. and Muhler, M.
ACS OMEGA. Volume: 8 (2023) - 2023 • 7556
Long-range Ising spins models emerging from frustrated Josephson junctions arrays with topological constraints
Neyenhuys, O. and Fistul, M. V. and Eremin, I. M.
PHYSICAL REVIEW B. Volume: 108 (2023) - 2023 • 7555
Magnetic bond-order potential for iron-cobalt alloys
Egorov, A. and Subramanyam, A. P. A. and Yuan, Z. and Drautz, R. and Hammerschmidt, T.
PHYSICAL REVIEW MATERIALS. Volume: 7 (2023) - 2023 • 7554
Modeling of very high frequency large-electrode capacitively coupled plasmas with a fully electromagnetic particle-in-cell code
Eremin, D. and Kemaneci, E. and Matsukuma, M. and Mussenbrock, T. and Brinkmann, R.P.
PLASMA SOURCES SCIENCE AND TECHNOLOGY. Volume: 32 (2023) - 2023 • 7553
Nonlocal dynamics of secondary electrons in capacitively coupled radio frequency discharges
Noesges, K. and Klich, M. and Derzsi, A. and Horváth, B. and Schulze, J. and Brinkmann, R.P. and Mussenbrock, T. and Wilczek, S.
PLASMA SOURCES SCIENCE AND TECHNOLOGY. Volume: 32 (2023) - 2023 • 7552
Electron Tunneling at Electrocatalytic Interfaces
Nouri, M.R. and Kluge, R.M. and Haid, R.W. and Fortmann, J. and Ludwig, Al. and Bandarenka, A.S. and Alexandrov, V.
JOURNAL OF PHYSICAL CHEMISTRY C. Volume: (2023) - 2023 • 7551
The effect of toluene trace admixtures on the plasma-assisted oxygen trace removal from hydrogen-rich gas mixtures in a surface dielectric barrier discharge reactor
Oberste-Beulmann, C. and Oppotsch, T. and Muhler, M.
PLASMA PROCESSES AND POLYMERS. Volume: 20 (2023) - 2023 • 7550
Investigation of flow characteristics in a twin-surface dielectric barrier discharge reactor by Schlieren imaging
Ollegott, K. and Wirth, P. and Oberste-Beulmann, C. and Sakthi, G. S. M. and Magazova, A. and Hermanns, P. and Peters, N. and Schücke, L. and Bracht, V. and Agar, D. W. and Awakowicz, P. and Muhler, M.
JOURNAL OF PHYSICS D: APPLIED PHYSICS. Volume: 56 (2023) - 2023 • 7549
Peculiar bond length dependence in (Ag,Cu)GaSe2 alloys and its impact on the bandgap bowing
Falk, H. H. and Eckner, S. and Seifert, M. and Ritter, K. and Levcenko, S. and Pfeiffelmann, T. and Welter, E. and Botti, S. and Shafarman, W. N. and Schnohr, C. S.
APL MATERIALS. Volume: 11 (2023) - 2023 • 7548
Spectral broadening of 2-mJ femtosecond pulses in a compact air-filled convex-concave multi-pass cell
Omar, A. and Vogel, T. and Hoffmann, M. and Saraceno, C.J.
OPTICS LETTERS. Volume: 48 (2023) - 2023 • 7547
Investigation on the crack formation in carbon concrete for the use in deck caps on cantilever slabs of bridges
Farwig, K. and Schulte-Schrepping, C. and Curbach, M. and Breitenbücher, R.
STRUCTURAL CONCRETE. Volume: 24 (2023) - 2023 • 7546
Simulating short-range order in compositionally complex materials
Ferrari, A. and Körmann, F. and Asta, M. and Neugebauer, J.
NATURE COMPUTATIONAL SCIENCE. Volume: 3 (2023) - 2023 • 7545
On the Impact of an Integrated HIP Treatment on the Very High Cycle Fatigue Life of Ni-Based SX Superalloys
Ormastroni, L.M.B. and Lopez-Galilea, I. and Ruttert, B. and Weber, S. and Mauget, F. and Villechaise, P. and Cormier, J.
METALLURGICAL AND MATERIALS TRANSACTIONS A: PHYSICAL METALLURGY AND MATERIALS SCIENCE. Volume: 54 (2023) - 2023 • 7544
Random forest classifier and neural network for fraction identification of refuse-derived fuel images
Fischer, J. and Wirtz, S. and Scherer, V.
FUEL. Volume: 341 (2023) - 2023 • 7543
Assessment of Deviations in Concrete Properties Quantified Under Laboratory Conditions and from the Construction Site
Ov, D. and Lozano Valcarcel, J. M. and Kränkel, T. and Breitenbücher, R. and Gehlen, C.
RILEM BOOKSERIES. Volume: 43 (2023) - 2023 • 7542
Simultaneous direct measurement of the electrocaloric and dielectric dynamics of ferroelectrics with microsecond temporal resolution
Fischer, J. and Döntgen, J. and Molin, C. and Gebhardt, S.E. and Hambal, Y. and Shvartsman, V.V. and Lupascu, D.C. and Hägele, D. and Rudolph, J.
REVIEW OF SCIENTIFIC INSTRUMENTS. Volume: 94 (2023) - 2023 • 7541
Investigation on the stability of raft structure in single crystal superalloy
Pang, L. and Zhang, Z.X. and Kong, L.W. and Xing, Z.B. and Shu, Y. and Li, P. and Eggeler, G.
JOURNAL OF ALLOYS AND COMPOUNDS. Volume: 944 (2023) - 2023 • 7540
Influence of Yttria-Stabilized Zirconium Oxide Thermal Swing Coating on the Flame-Wall Interaction in Spark Ignition Engines
Fischer, M. and Nolte, A. and Wu, X. and Zhou, D. and Pischinger, S. and Heufer, K.A. and Simon, U. and Vaßen, R.
ENERGIES. Volume: 16 (2023) - 2023 • 7539
Toward the design of ultrahigh-entropy alloys via mining six million texts
Pei, Z. and Yin, J. and Liaw, P.K. and Raabe, D.
NATURE COMMUNICATIONS. Volume: 14 (2023) - 2023 • 7538
Hydration at Highly Crowded Interfaces
Penschke, C. and Thomas, J. and Bertram, C. and Michaelides, A. and Morgenstern, K. and Saalfrank, P. and Bovensiepen, U.
PHYSICAL REVIEW LETTERS. Volume: 130 (2023) - 2023 • 7537
Preferred corrosion pathways for oxygen in Al2Ca – twin boundaries and dislocations
Peter, N.J. and Zander, D. and Cao, X. and Tian, C. and Zhang, S. and Du, K. and Scheu, C. and Dehm, G.
JOURNAL OF ALLOYS AND COMPOUNDS. Volume: 936 (2023) - 2023 • 7536
Material reduced tunnel lining segments for moderately swelling soils
Petraroia, D.N. and Medeghini, F. and Plückelmann, S. and Mark, P. and Breitenbücher, R.
STRUCTURAL CONCRETE. Volume: (2023) - 2023 • 7535
Melt pool signatures of TiN nanoparticle dry-coated Co25Cr25Fe25Ni25 metal powder in laser-powder-bed-fusion
Gärtner, E. and Witte, A. and Peter, N.J. and Devulapalli, V. and Ellendt, N. and Dehm, G. and Jägle, E.A. and Uhlenwinkel, V. and Mädler, L.
MATERIALS AND DESIGN. Volume: 226 (2023) - 2023 • 7534
Increasing the friction stress decreases the size dependence of strength in a family of face-centered cubic high- and medium-entropy alloy micropillars
Pfetzing-Micklich, J. and Fox, F. and Thome, P. and George, E.P. and Eggeler, G.
MATERIALS SCIENCE AND ENGINEERING: A. Volume: 885 (2023) - 2023 • 7533
Tracer diffusion under a concentration gradient: A pathway for a consistent development of mobility databases in multicomponent alloys
Gaertner, D. and Kundin, J. and Esakkiraja, N. and Berndt, J. and Durand, A. and Kottke, J. and Klemme, S. and Laplanche, G. and Eggeler, G. and Wilde, G. and Paul, A. and Steinbach, I. and Divinski, S.V.
JOURNAL OF ALLOYS AND COMPOUNDS. Volume: 930 (2023) - 2023 • 7532
Evolution of local misorientations in the γ/γ’-microstructure of single crystal superalloys during creep studied with the rotation vector baseline (RVB) EBSD method
Gamanov, S. and Dlouhy, A. and Bürger, D. and Eggeler, G. and Thome, P.
MICROSCOPY RESEARCH AND TECHNIQUE. Volume: (2023) - 2023 • 7531
Influence of Surface Roughness on Material Classification for Reflective THz-TDS Measurements
Gassel, S. T. and Hofmann, M. R. and Brenner, C.
INTERNATIONAL CONFERENCE ON INFRARED, MILLIMETER, AND TERAHERTZ WAVES, IRMMW-THZ. Volume: (2023) - 2023 • 7530
Probing the Gold/Water Interface with Surface-Specific Spectroscopy
Piontek, S.M. and Naujoks, D. and Tabassum, T. and DelloStritto, M.J. and Jaugstetter, M. and Hosseini, P. and Corva, M. and Ludwig, Al. and Tschulik, K. and Klein, M.L. and Petersen, P.B.
ACS PHYSICAL CHEMISTRY AU. Volume: 3 (2023) - 2023 • 7529
Unusual Phase Formation in Reactively Sputter-Deposited La—Co—O Thin-Film Libraries
Piotrowiak, T. H. and Zehl, R. and Suhr, E. and Banko, L. and Kohnen, B. and Rogalla, D. and Ludwig, A.
ADVANCED ENGINEERING MATERIALS. Volume: 25 (2023) - 2023 • 7528
LaCo1-xFexO3 Nanoparticles in Cyclohexene Oxidation
Geiss, J. and Büker, J. and Schulte, J. and Peng, B. and Muhler, M. and Winterer, M.
JOURNAL OF PHYSICAL CHEMISTRY C. Volume: 127 (2023) - 2023 • 7527
Charge-optimized many-body interaction potential for AlN revisited to explore plasma–surface interactions
Gergs, T. and Mussenbrock, T. and Trieschmann, J.
SCIENTIFIC REPORTS. Volume: 13 (2023) - 2023 • 7526
Physics-separating artificial neural networks for predicting sputtering and thin film deposition of AlN in Ar/N2 discharges on experimental timescales
Gergs, T. and Mussenbrock, T. and Trieschmann, J.
JOURNAL OF PHYSICS D: APPLIED PHYSICS. Volume: 56 (2023) - 2023 • 7525
Physics-separating artificial neural networks for predicting initial stages of Al sputtering and thin film deposition in Ar plasma discharges
Gergs, T. and Mussenbrock, T. and Trieschmann, J.
JOURNAL OF PHYSICS D: APPLIED PHYSICS. Volume: 56 (2023) - 2023 • 7524
Mechanical properties and thermal stability of ZrCuAlx thin film metallic glasses: Experiments and first-principle calculations
Poltronieri, C. and Brognara, A. and Bignoli, F. and Evertz, S. and Djemia, P. and Faurie, D. and Challali, F. and Li, C.H. and Belliard, L. and Dehm, G. and Best, J.P. and Ghidelli, M.
ACTA MATERIALIA. Volume: 258 (2023) - 2023 • 7523
Systematic atomic structure datasets for machine learning potentials: Application to defects in magnesium
Poul, M. and Huber, L. and Bitzek, E. and Neugebauer, J.
PHYSICAL REVIEW B. Volume: 107 (2023) - 2023 • 7522
Interplay of Precursor and Plasma for The Deposition of HfO2 via PEALD: Film Growth and Dielectric Properties
Preischel, F. and Zanders, D. and Berning, T. and Kostka, A. and Rogalla, D. and Bock, C. and Devi, A.
ADVANCED MATERIALS INTERFACES. Volume: 10 (2023) - 2023 • 7521
Thermally Sprayed Functional Coatings and Multilayers: A Selection of Historical Applications and Potential Pathways for Future Innovation
Gildersleeve, E.J., V and Vaßen, R.
JOURNAL OF THERMAL SPRAY TECHNOLOGY. Volume: (2023) - 2023 • 7520
Austenite grain boundary segregation and precipitation of boron in low-C steels and their role on the heterogeneous nucleation of ferrite
Prithiv, T.S. and Gault, B. and Li, Y. and Andersen, D. and Valle, N. and Eswara, S. and Ponge, D. and Raabe, D.
ACTA MATERIALIA. Volume: 252 (2023) - 2023 • 7519
Continuous ex vivo glucose sensing in human physiological fluids using an enzymatic sensor in a vein replica
Psotta, C. and Cirovic, S. and Gudmundsson, P. and Falk, M. and Mandal, T. and Reichhart, T. and Leech, D. and Ludwig, R. and Kittel, R. and Schuhmann, W. and Shleev, S.
BIOELECTROCHEMISTRY. Volume: 152 (2023) - 2023 • 7518
Revisiting the metal-to-metal transition in 2H-AgNiO2
Gondolf, J. and Eremin, I. M. and Lechermann, F.
EPL. Volume: 144 (2023) - 2023 • 7517
Robust Conveniently Sealable Container for High-Temperature Single-Crystal Growth Out of Reactive Melts with High Vapor Pressure
Püttmann, J. and Sangeetha, N.S. and Thomas, T. R. and Meermann, J. and Kreyssig, A. and Böhmer, A. E.
CRYSTALS. Volume: 13 (2023) - 2023 • 7516
Atomic Cluster Expansion for Quantum-Accurate Large-Scale Simulations of Carbon
Qamar, M. and Mrovec, M. and Lysogorskiy, Y. and Bochkarev, A. and Drautz, R.
JOURNAL OF CHEMICAL THEORY AND COMPUTATION. Volume: 19 (2023) - 2023 • 7515
Functionally graded tungsten/EUROFER coating for DEMO first wall: From laboratory to industrial production
Grammes, T. and Emmerich, T. and Qu, D. and Heinze, O. and Vaßen, R. and Aktaa, J.
FUSION ENGINEERING AND DESIGN. Volume: 188 (2023) - 2023 • 7514
Atomistic simulations of diffusion in γ′ -strengthened Co-based superalloys and its connection to selective alumina formation in early-stage oxidation
Qin, L. and Kubacka, D. and Spiecker, E. and Drautz, R. and Rogal, J.
PHYSICAL REVIEW MATERIALS. Volume: 7 (2023) - 2023 • 7513
Accelerating the design of compositionally complex materials via physics-informed artificial intelligence
Raabe, D. and Mianroodi, J.R. and Neugebauer, J.
NATURE COMPUTATIONAL SCIENCE. Volume: 3 (2023) - 2023 • 7512
The Materials Science behind Sustainable Metals and Alloys
Raabe, D.
CHEMICAL REVIEWS. Volume: 123 (2023) - 2023 • 7511
Hydrogen Plasma Reduction of Iron Oxides
Raabe, D. and Klug, M.J. and Ma, Y. and Büyükuslu, Ö. and Springer, H. and Souza Filho, I.
MINERALS, METALS AND MATERIALS SERIES. Volume: (2023) - 2023 • 7510
A novel powder-metallurgical eco-friendly recycling process for tool steel grinding sludge
Großwendt, F. and Bürk, V. and Kopanka, B. and Jäger, S. and Pollak, S. and Leich, L. and Röttger, A. and Petermann, M. and Weber, S.
JOURNAL OF CLEANER PRODUCTION. Volume: 392 (2023) - 2023 • 7509
Hydrogen-Based Direct Reduction of Iron Oxides
Raabe, D. and Springer, H. and Filho, I.S. and Ma, Y.
MINERALS, METALS AND MATERIALS SERIES. Volume: (2023) - 2023 • 7508
Tailoring Pore Size and Catalytic Activity in Cobalt Iron Layered Double Hydroxides and Spinels by Microemulsion-Assisted pH-Controlled Co-Precipitation
Rabe, A. and Jaugstetter, M. and Hiege, F. and Cosanne, N. and Ortega, K.F. and Linnemann, J. and Tschulik, K. and Behrens, M.
CHEMSUSCHEM. Volume: (2023) - 2022 • 7507
Ferromagnetic Europium Sulfide Thin Films: Influence of Precursors on Magneto-Optical Properties
Beer, S.M.J. and Muriqi, A. and Lindner, P. and Winter, M. and Rogalla, D. and Nolan, M. and Ney, A. and Debus, J. and Devi, A.
CHEMISTRY OF MATERIALS. Volume: 34 (2022) - 2022 • 7506
Selective hydrogenation of highly concentrated acetylene streams over mechanochemically synthesized PdAg supported catalysts
Kley, K.S. and De Bellis, J. and Schüth, F.
CATALYSIS SCIENCE AND TECHNOLOGY. Volume: 13 (2022) - 2022 • 7505
Hydrogen trapping and embrittlement in high-strength Al alloys
Zhao, H. and Chakraborty, P. and Ponge, D. and Hickel, T. and Sun, B. and Wu, C.-H. and Gault, B. and Raabe, D.
NATURE. Volume: 602 (2022) - 2022 • 7504
Validation of the smooth step model by particle-in-cell/Monte Carlo collisions simulations
Klich, M. and Löwer, J. and Wilczek, S. and Mussenbrock, T. and Brinkmann, R.P.
PLASMA SOURCES SCIENCE AND TECHNOLOGY. Volume: 31 (2022) - 2022 • 7503
Low-temperature ALD process development of 200 mm wafer-scale MoS2 for gas sensing application
Neubieser, R.-M. and Wree, J.-L. and Jagosz, J. and Becher, M. and Ostendorf, A. and Devi, A. and Bock, C. and Michel, M. and Grabmaier, A.
MICRO AND NANO ENGINEERING. Volume: 15 (2022) - 2022 • 7502
Thermal stability of nanoscale ferroelectric domains by molecular dynamics modeling
Klomp, A.J. and Khachaturyan, R. and Wallis, T. and Albe, K. and Grünebohm, A.
PHYSICAL REVIEW MATERIALS. Volume: 6 (2022) - 2022 • 7501
Optimal Data-Generation Strategy for Machine Learning Yield Functions in Anisotropic Plasticity
Shoghi, R. and Hartmaier, A.
FRONTIERS IN MATERIALS. Volume: 9 (2022) - 2022 • 7500
Thermal softening-suppressed inter-granular embrittlement of polycrystalline 3C-SiC under diamond cutting
Zhao, L. and Zhang, J. and Fu, Y. and Zhang, J. and Hartmaier, A. and Sun, T.
MATERIALS AND DESIGN. Volume: 223 (2022) - 2022 • 7499
Layer thickness controlling in Direct Energy Deposition process by adjusting the powder flow rate
Behlau, F. and Thiele, M. and Maack, P. and Esen, C. and Ostendorf, A.
PROCEDIA CIRP. Volume: 111 (2022) - 2022 • 7498
THIN CONCRETE OVERLAYS WIT CARBON REINFORCEMENT ON DETERIORATED CONCRETE PAVEMENTS
Neumann, J. and Breitenbücher, R.
ACTA POLYTECHNICA CTU PROCEEDINGS. Volume: 33 (2022) - 2022 • 7497
Formation of high density stacking faults in polycrystalline 3C-SiC by vibration-assisted diamond cutting
Zhao, L. and Zhang, J. and Zhang, J. and Hartmaier, A. and Sun, T.
JOURNAL OF THE EUROPEAN CERAMIC SOCIETY. Volume: 42 (2022) - 2022 • 7496
Investigation of the Influence of Surface Roughness on Reflective THz Measurements
Gassel, S.T. and Azih, D.A. and Hofmann, M.R. and Brenner, C.
INTERNATIONAL CONFERENCE ON INFRARED, MILLIMETER, AND TERAHERTZ WAVES, IRMMW-THZ. Volume: 2022-August (2022) - 2022 • 7495
On the Mediated Electron Transfer of Immobilized Galactose Oxidase for Biotechnological Applications
Zhao, F. and Brix, A.C. and Lielpetere, A. and Schuhmann, W. and Conzuelo, F.
CHEMISTRY - A EUROPEAN JOURNAL. Volume: 28 (2022) - 2022 • 7494
Investigation of Laser-Induced Periodic Surface Structures Using Synthetic Optical Holography
Neutsch, K. and Gurevich, E.L. and Hofmann, M.R. and Gerhardt, N.C.
NANOMATERIALS. Volume: 12 (2022) - 2022 • 7493
Oxidation behavior of double-ceramic-layer thermal barrier coatings deposited by atmospheric plasma spraying and suspension plasma spraying
Zhao, Y. and Ge, Y. and Jin, X. and Koch, D. and Vaßen, R. and Chen, Y. and Fan, X.
CERAMICS INTERNATIONAL. Volume: 48 (2022) - 2022 • 7492
Electrochemical CO2 reduction - The macroscopic world of electrode design, reactor concepts & economic aspects
Gawel, A. and Jaster, T. and Siegmund, D. and Holzmann, J. and Lohmann, H. and Klemm, E. and Apfel, U.-P.
ISCIENCE. Volume: 25 (2022) - 2022 • 7491
Surface Diffusion Aided by a Chirality Change of Self-Assembled Oligomers under 2D Confinement
Bera, A. and Henkel, S. and Mieres-Perez, J. and Andargie Tsegaw, Y. and Sanchez-Garcia, E. and Sander, W. and Morgenstern, K.
ANGEWANDTE CHEMIE - INTERNATIONAL EDITION. Volume: 61 (2022) - 2022 • 7490
μs and ns twin surface dielectric barrier discharges operated in air: From electrode erosion to plasma characteristics
Nguyen-Smith, R.T. and Böddecker, A. and Schücke, L. and Bibinov, N. and Korolov, I. and Zhang, Q.-Z. and Mussenbrock, T. and Awakowicz, P. and Schulze, J.
PLASMA SOURCES SCIENCE AND TECHNOLOGY. Volume: 31 (2022) - 2022 • 7489
Noble-metal-free electrocatalysts for water splitting metal chalcogenides
Siegmund, D. and Apfel, U.-P.
CATALYTIC SCIENCE SERIES. Volume: 22 (2022) - 2022 • 7488
Revealing in-plane grain boundary composition features through machine learning from atom probe tomography data
Zhou, X. and Wei, Y. and Kühbach, M. and Zhao, H. and Vogel, F. and Darvishi Kamachali, R. and Thompson, G.B. and Raabe, D. and Gault, B.
ACTA MATERIALIA. Volume: 226 (2022) - 2022 • 7487
In Situ Growth and Bias-Dependent Modification of NaBr Ionic Layers on Ag(111)
Bera, A. and Morgenstern, K.
JOURNAL OF PHYSICAL CHEMISTRY C. Volume: 126 (2022) - 2022 • 7486
The role of momentum conservation on the tunneling between a two-dimensional electron gas and self-assembled quantum dots
Zhou, D. and Kerski, J. and Beckel, A. and Geller, M. and Lorke, A. and Ludwig, A. and Wieck, A.D. and Chen, X. and Lu, W.
JOURNAL OF APPLIED PHYSICS. Volume: 132 (2022) - 2022 • 7485
Ultralong one-dimensional plastic zone created in aluminum underneath a nanoscale indent
Nie, Z.-Y. and Sato, Y. and Ogata, S. and Duarte, M.J. and Dehm, G. and Li, J. and Ma, E. and Xie, D.-G. and Shan, Z.-W.
ACTA MATERIALIA. Volume: 232 (2022) - 2022 • 7484
Stacking faults in a mechanically strong Al(Mg)–Al3Mg2 composite
Zhou, D. and Zhang, X. and Tehranchi, A. and Hou, J. and Lu, W. and Hickel, T. and Ponge, D. and Raabe, D. and Zhang, D.
COMPOSITES PART B: ENGINEERING. Volume: 245 (2022) - 2022 • 7483
Impact of Thermophysical Properties of High-Alloy Tool Steels on Their Performance in Re-Purposing Applications
Berger, A. and Benito, S. and Kronenberg, P. and Weber, S.
MATERIALS. Volume: 15 (2022) - 2022 • 7482
Neural Network Potentials: A Concise Overview of Methods
Kocer, E. and Ko, T.W. and Behler, J.
ANNUAL REVIEW OF PHYSICAL CHEMISTRY. Volume: 73 (2022) - 2022 • 7481
Study on the Effect of Electrolyte pH during Kolbe Electrolysis of Acetic Acid on Pt Anodes
Nordkamp, M.O. and Mei, B. and Venderbosch, R. and Mul, G.
CHEMCATCHEM. Volume: 14 (2022) - 2022 • 7480
Highly sensitive and stable MEMS acetone sensors based on well-designed α-Fe2O3/C mesoporous nanorods
Zhu, L.-Y. and Yuan, K. and Li, Z.-C. and Miao, X.-Y. and Wang, J.-C. and Sun, S. and Devi, A. and Lu, H.-L.
JOURNAL OF COLLOID AND INTERFACE SCIENCE. Volume: 622 (2022) - 2022 • 7479
Integration of Hot Isostatic Pressing and Heat Treatment for Advanced Modified γ-TiAl TNM Alloys
Bernal, D. and Chamorro, X. and Hurtado, I. and Lopez-Galilea, I. and Bürger, D. and Weber, S. and Madariaga, I.
MATERIALS. Volume: 15 (2022) - 2022 • 7478
Controlled quantum dot array segmentation via highly tunable interdot tunnel coupling
Nurizzo, M. and Jadot, B. and Mortemousque, P.-A. and Thiney, V. and Chanrion, E. and Dartiailh, M. and Ludwig, Ar. and Wieck, A.D. and Bäuerle, C. and Urdampilleta, M. and Meunier, T.
APPLIED PHYSICS LETTERS. Volume: 121 (2022) - 2022 • 7477
Redox Replacement of Silver on MOF-Derived Cu/C Nanoparticles on Gas Diffusion Electrodes for Electrocatalytic CO2 Reduction
Sikdar, N. and Junqueira, J.R.C. and Öhl, D. and Dieckhöfer, S. and Quast, T. and Braun, M. and Aiyappa, H.B. and Seisel, S. and Andronescu, C. and Schuhmann, W.
CHEMISTRY - A EUROPEAN JOURNAL. Volume: 28 (2022) - 2022 • 7476
Heterostructured α-Fe2O3@ZnO@ZIF-8 Core–Shell Nanowires for a Highly Selective MEMS-Based ppb-Level H2S Gas Sensor System
Zhu, L.-Y. and Miao, X.-Y. and Ou, L.-X. and Mao, L.-W. and Yuan, K. and Sun, S. and Devi, A. and Lu, H.-L.
SMALL. Volume: (2022) - 2022 • 7475
Nucleation and growth studies of large-area deposited WS2 on flexible substrates
Berning, T. and Becher, M. and Wree, J.-L. and Jagosz, J. and Kostka, A. and Ostendorf, A. and Devi, A. and Bock, C.
MATERIALS RESEARCH EXPRESS. Volume: 9 (2022) - 2022 • 7474
Microstructure property classification of nickel-based superalloys using deep learning
Nwachukwu, U. and Obaied, A. and Horst, O.M. and Ali, M.A. and Steinbach, I. and Roslyakova, I.
MODELLING AND SIMULATION IN MATERIALS SCIENCE AND ENGINEERING. Volume: 30 (2022) - 2022 • 7473
Single Particle Electrochemical Oxidation of Polyvinylpyrrolidone-Capped Silver Nanospheres, Nanocubes, and Nanoplates in Potassium Nitrate and Potassium Hydroxide Solutions
Sikes, J.C. and Niyonshuti, I.I. and Kanokkanchana, K. and Chen, J. and Tschulik, K. and Fritsch, I.
JOURNAL OF THE ELECTROCHEMICAL SOCIETY. Volume: 169 (2022) - 2022 • 7472
Interplay of Halogen and Weak Hydrogen Bonds in the Formation of Magic Nanoclusters on Surfaces
Bertram, C. and Miller, D.P. and Schunke, C. and Kemeny, I. and Kimura, M. and Bovensiepen, U. and Zurek, E. and Morgenstern, K.
JOURNAL OF PHYSICAL CHEMISTRY C. Volume: 126 (2022) - 2022 • 7471
On-Demand Source of Dual-Rail Photon Pairs Based on Chiral Interaction in a Nanophotonic Waveguide
Østfeldt, F.T. and González-Ruiz, E.M. and Hauff, N. and Wang, Y. and Wieck, A.D. and Ludwig, Ar. and Schott, R. and Midolo, L. and Sørensen, A.S. and Uppu, R. and Lodahl, P.
PRX QUANTUM. Volume: 3 (2022) - 2022 • 7470
Characterization of Nanoparticles in Diverse Mixtures Using Localized Surface Plasmon Resonance and Nanoparticle Tracking by Dark-Field Microscopy with Redox Magnetohydrodynamics Microfluidics
Sikes, J.C. and Wonner, K. and Nicholson, A. and Cignoni, P. and Fritsch, I. and Tschulik, K.
ACS PHYSICAL CHEMISTRY AU. Volume: 2 (2022) - 2022 • 7469
Degradation and lifetime of self-healing thermal barrier coatings containing MoSi2 as self-healing particles in thermo-cycling testing
Koch, D. and Mack, D.E. and Vaßen, R.
SURFACE AND COATINGS TECHNOLOGY. Volume: 437 (2022) - 2022 • 7468
Atom Pair Frequencies as a Quantitative Structure-Activity Relationship for Catalytic 2-Propanol Oxidation over Nanocrystalline Cobalt-Iron-Spinel
Geiss, J. and Falk, T. and Ognjanovic, S. and Anke, S. and Peng, B. and Muhler, M. and Winterer, M.
JOURNAL OF PHYSICAL CHEMISTRY C. Volume: 126 (2022) - 2022 • 7467
Adaptive convexification of microsphere-based incremental damage for stress and strain softening at finite strains
Köhler, M. and Neumeier, T. and Melchior, J. and Peter, M.A. and Peterseim, D. and Balzani, D.
ACTA MECHANICA. Volume: 233 (2022) - 2022 • 7466
Continuum multiscale modeling of absorption processes in micro- and nanocatalysts
Köhler, M. and Junker, P. and Balzani, D.
ARCHIVE OF APPLIED MECHANICS. Volume: 92 (2022) - 2022 • 7465
Photochemical Sandmeyer-type Halogenation of Arenediazonium Salts
Sivendran, N. and Belitz, F. and Sowa Prendes, D. and Manu Martínez, Á. and Schmid, R. and Gooßen, L.J.
CHEMISTRY - A EUROPEAN JOURNAL. Volume: 28 (2022) - 2022 • 7464
Processing of a Martensitic Tool Steel by Wire-Arc Additive Manufacturing
Ziesing, U. and Lentz, J. and Röttger, A. and Theisen, W. and Weber, S.
MATERIALS. Volume: 15 (2022) - 2022 • 7463
Control of spoke movement in DCMS plasmas
George, M. and Breilmann, W. and Held, J. and von Keudell, A.
PLASMA SOURCES SCIENCE AND TECHNOLOGY. Volume: 31 (2022) - 2022 • 7462
MD studies of methanol confined in the metal-organic framework MOF MIL-88B-Cl
Siwaipram, S. and Bopp, P.A. and Ponchai, P. and Soetens, J.-C. and Hasegawa, J.-Y. and Schmid, R. and Bureekaew, S.
JOURNAL OF MOLECULAR LIQUIDS. Volume: 359 (2022) - 2022 • 7461
Extending the time of coherent optical response in ensemble of singly-charged InGaAs quantum dots
Kosarev, A.N. and Trifonov, A.V. and Yugova, I.A. and Yanibekov, I.I. and Poltavtsev, S.V. and Kamenskii, A.N. and Scholz, S.E. and Sgroi, C.A. and Ludwig, A. and Wieck, A.D. and Yakovlev, D.R. and Bayer, M. and Akimov, I.A.
COMMUNICATIONS PHYSICS. Volume: 5 (2022) - 2022 • 7460
A simple and efficient adaptive time stepping technique for low-order operator splitting schemes applied to cardiac electrophysiology
Ogiermann, D. and Perotti, L.E. and Balzani, D.
INTERNATIONAL JOURNAL FOR NUMERICAL METHODS IN BIOMEDICAL ENGINEERING. Volume: (2022) - 2022 • 7459
Molecular dynamics study on the role of Ar ions in the sputter deposition of Al thin films
Gergs, T. and Mussenbrock, T. and Trieschmann, J.
JOURNAL OF APPLIED PHYSICS. Volume: 132 (2022) - 2022 • 7458
Modeling and Control Design of a Contact-Based, Electrostatically Actuated Rotating Sphere
Olbrich, M. and Farny, M. and Hoffmann, M. and Ament, C.
ACTUATORS. Volume: 11 (2022) - 2022 • 7457
Similarity of competing risks models with constant intensities in an application to clinical healthcare pathways involving prostate cancer surgery
Binder, N. and Möllenhoff, K. and Sigle, A. and Dette, H.
STATISTICS IN MEDICINE. Volume: 41 (2022) - 2022 • 7456
Nanoporous SiOx plasma polymer films as carrier for liquid-infused surfaces
Gergs, T. and Monti, C. and Gaiser, S. and Amberg, M. and Schütz, U. and Mussenbrock, T. and Trieschmann, J. and Heuberger, M. and Hegemann, D.
PLASMA PROCESSES AND POLYMERS. Volume: 19 (2022) - 2022 • 7455
A thermodynamic framework for unified continuum models for the healing of damaged soft biological tissue
Zuo, D. and He, Y. and Avril, S. and Yang, H. and Hackl, K.
JOURNAL OF THE MECHANICS AND PHYSICS OF SOLIDS. Volume: 158 (2022) - 2022 • 7454
Data Mining in Urology: Understanding Real-world Treatment Pathways for Lower Urinary Tract Systems via Exploration of Big Data
Binder, N. and Dette, H. and Franz, J. and Zöller, D. and Suarez-Ibarrola, R. and Gratzke, C. and Binder, H. and Miernik, A.
EUROPEAN UROLOGY FOCUS. Volume: 8 (2022) - 2022 • 7453
Theoretical simulation and experimental verification of dynamic caustic manipulation using a deformable mirror for laser material processing
Smarra, M. and Gurevich, E.L. and Ostendorf, A.
OPTICS AND LASER TECHNOLOGY. Volume: 149 (2022) - 2022 • 7452
Linear growth of reaction layer during in-situ TEM annealing of thin film Al/Ni diffusion couples
Kostka, A. and Naujoks, D. and Oellers, T. and Salomon, S. and Somsen, C. and Öztürk, E. and Savan, A. and Ludwig, A. and Eggeler, G.
JOURNAL OF ALLOYS AND COMPOUNDS. Volume: 922 (2022) - 2022 • 7451
Inner relaxations in equiatomic single-phase high-entropy cantor alloy
Smekhova, A. and Kuzmin, A. and Siemensmeyer, K. and Abrudan, R. and Reinholz, U. and Buzanich, A.G. and Schneider, M. and Laplanche, G. and Yusenko, K.V.
JOURNAL OF ALLOYS AND COMPOUNDS. Volume: 920 (2022) - 2022 • 7450
Dislocation-enhanced electrical conductivity in rutile TiO2 accessed by room-temperature nanoindentation
Bishara, H. and Tsybenko, H. and Nandy, S. and Muhammad, Q.K. and Frömling, T. and Fang, X. and Best, J.P. and Dehm, G.
SCRIPTA MATERIALIA. Volume: 212 (2022) - 2022 • 7449
Trimetallic Pentlandites (Fe,Co,Ni)9S8for the Electrocatalytical HER in Acidic Media
Smialkowski, M. and Siegmund, D. and Stier, K. and Hensgen, L. and Checinski, M.P. and Apfel, U.-P.
ACS MATERIALS AU. Volume: 2 (2022) - 2022 • 7448
Effect of hybridization in PdAlY-(Ni/Au/Ir) metallic glasses thin films on electrical resistivity
Bishara, H. and Kontis, P. and Dehm, G. and Schneider, J.M. and Evertz, S.
SCRIPTA MATERIALIA. Volume: 214 (2022) - 2022 • 7447
Synthesis and Characterization of Phosphorus-Containing Isocyclam Macrocycles and Their Nickel Complexes
Gerschel, P. and Guseva, T. and Siegmund, D. and Apfel, U.-P.
JOURNAL OF ORGANIC CHEMISTRY. Volume: 87 (2022) - 2022 • 7446
Ab initio investigations of point and complex defect structures in B2-FeAl
Sözen, H.I. and Mendive-Tapia, E. and Hickel, T. and Neugebauer, J.
PHYSICAL REVIEW MATERIALS. Volume: 6 (2022) - 2022 • 7445
Hydrogen Diffusion in Clinopyroxene at Low Temperatures (195°C–400°C) and Consequences for Subsurface Processes
Bissbort, T. and Lynn, K.J. and Becker, H.-W. and Chakraborty, S.
GEOCHEMISTRY, GEOPHYSICS, GEOSYSTEMS. Volume: 23 (2022) - 2022 • 7444
Locally varying formation of nanoclusters across a low-intensity ultra-short laser spot
Osterloh, N. and Pan, T. and Morgenstern, K.
NANOSCALE HORIZONS. Volume: 8 (2022) - 2022 • 7443
A hybrid approach for the efficient computation of polycrystalline yield loci with the accuracy of the crystal plasticity finite element method
Biswas, A. and Kalidindi, S.R. and Hartmaier, A.
MODELLING AND SIMULATION IN MATERIALS SCIENCE AND ENGINEERING. Volume: 30 (2022) - 2022 • 7442
Qubit Bias using a CMOS DAC at mK Temperatures
Otten, R. and Schreckenberg, L. and Vliex, P. and Ritzmann, J. and Ludwig, Ar. and Wieck, A.D. and Bluhm, H.
ICECS 2022 - 29TH IEEE INTERNATIONAL CONFERENCE ON ELECTRONICS, CIRCUITS AND SYSTEMS, PROCEEDINGS. Volume: (2022) - 2022 • 7441
Short-range order and phase stability of CrCoNi explored with machine learning potentials
Ghosh, S. and Sotskov, V. and Shapeev, A.V. and Neugebauer, J. and Körmann, F.
PHYSICAL REVIEW MATERIALS. Volume: 6 (2022) - 2022 • 7440
Martensite Transformation in Tool Steels under Isostatic Pressure–Implementation of In-Situ Electrical Resistivity Measurements into a Hot Isostatic Press with Rapid Quenching Technology
Kramer, B. and Deng, Y. and Lentz, J. and Broeckmann, C. and Theisen, W. and Weber, S.
METALS. Volume: 12 (2022) - 2022 • 7439
Nonparametric and high-dimensional functional graphical models
Solea, E. and Dette, H.
ELECTRONIC JOURNAL OF STATISTICS. Volume: 16 (2022) - 2022 • 7438
INFLUENCE OF STEEL FIBERS ON THE FATIGUE BEHAVIOR OF HIGH-PERFORMANCE CONCRETES UNDER CYCLIC LOADING
Ov, D. and Breitenbücher, R.
ACTA POLYTECHNICA CTU PROCEEDINGS. Volume: 33 (2022) - 2022 • 7437
Green steel at its crossroads: Hybrid hydrogen-based reduction of iron ores
Souza Filho, I.R. and Springer, H. and Ma, Y. and Mahajan, A. and da Silva, C.C. and Kulse, M. and Raabe, D.
JOURNAL OF CLEANER PRODUCTION. Volume: 340 (2022) - 2022 • 7436
Efficient parametrization of the atomic cluster expansion
Bochkarev, A. and Lysogorskiy, Y. and Menon, S. and Qamar, M. and Mrovec, M. and Drautz, R.
PHYSICAL REVIEW MATERIALS. Volume: 6 (2022) - 2022 • 7435
Amphiphilic Alginate-Based Layer-by-Layer Coatings Exhibiting Resistance against Nonspecific Protein Adsorption and Marine Biofouling
Gnanasampanthan, T. and Karthäuser, J.F. and Spöllmann, S. and Wanka, R. and Becker, H.-W. and Rosenhahn, A.
ACS APPLIED MATERIALS AND INTERFACES. Volume: 14 (2022) - 2022 • 7434
Multilayer atomic cluster expansion for semilocal interactions
Bochkarev, A. and Lysogorskiy, Y. and Ortner, C. and Csányi, G. and Drautz, R.
PHYSICAL REVIEW RESEARCH. Volume: 4 (2022) - 2022 • 7433
Competing instabilities of the extended Hubbard model on the triangular lattice: Truncated-unity functional renormalization group and application to moiré materials
Gneist, N. and Classen, L. and Scherer, M.M.
PHYSICAL REVIEW B. Volume: 106 (2022) - 2022 • 7432
Superconducting Instabilities in Strongly Correlated Infinite-Layer Nickelates
Kreisel, A. and Andersen, B.M. and Rømer, A.T. and Eremin, I.M. and Lechermann, F.
PHYSICAL REVIEW LETTERS. Volume: 129 (2022) - 2022 • 7431
Multilayer atomic cluster expansion for semilocal interactions
Bochkarev, A. and Lysogorskiy, Y. and Ortner, C. and Csányi, G. and Drautz, R.
PHYSICAL REVIEW RESEARCH. Volume: 4 (2022) - 2022 • 7430
Functional renormalization of spinless triangular-lattice fermions: N-patch vs. truncated-unity scheme
Gneist, N. and Kiese, D. and Henkel, R. and Thomale, R. and Classen, L. and Scherer, M.M.
EUROPEAN PHYSICAL JOURNAL B. Volume: 95 (2022) - 2022 • 7429
Swing Up and Balance of an Inverted Pendulum Using Reinforced Learning Approach Coupled with a Proportional-Integral-Derivative Controller
Pal, A.K. and Nestorović, T.
INTERNATIONAL CONFERENCE ON ELECTRICAL, COMPUTER, COMMUNICATIONS AND MECHATRONICS ENGINEERING, ICECCME 2022. Volume: (2022) - 2022 • 7428
Unveiling nonmonotonic chemical trends in the solubility of H in complex Fe-Cr-Mn carbides by means of ab initio based approaches
Sreekala, L. and Dey, P. and Hickel, T. and Neugebauer, J.
PHYSICAL REVIEW MATERIALS. Volume: 6 (2022) - 2022 • 7427
Bone tunnel placement influences shear stresses at the coracoid process after coracoclavicular ligament reconstruction: a finite element study and radiological analysis
Bockmann, B. and Dankl, L. and Kucinskaite, G. and Kumar, A. and Timothy, J.J. and Meschke, G. and Venjakob, A.J. and Schulte, T.L.
ARCHIVES OF ORTHOPAEDIC AND TRAUMA SURGERY. Volume: (2022) - 2022 • 7426
Comparison of the performance of a microwave plasma torch and a gliding arc plasma for hydrogen production via methane pyrolysis
Kreuznacht, S. and Purcel, M. and Böddeker, S. and Awakowicz, P. and Xia, W. and Muhler, M. and Böke, M. and Keudell, A.V.
PLASMA PROCESSES AND POLYMERS. Volume: (2022) - 2022 • 7425
A scalable twin surface dielectric barrier discharge system for pollution remediation at high gas flow rates
Böddecker, A. and Bodnar, A. and Schücke, L. and Giesekus, J. and Wenselau, K. and Nguyen-Smith, R.T. and Oppotsch, T. and Oberste-Beulmann, C. and Muhler, M. and Gibson, A.R. and Awakowicz, P.
REACTION CHEMISTRY AND ENGINEERING. Volume: (2022) - 2022 • 7424
Photocatalytic nanocomposite membranes for environmental remediation
Golmohammadi, M. and Sabbagh Alvani, A.A. and Sameie, H. and Mei, B. and Salimi, R. and Poelman, D. and Rosei, F.
NANOTECHNOLOGY. Volume: 33 (2022) - 2022 • 7423
Tuning the Electronic Properties of Homoleptic Silver(I) bis-BIAN Complexes towards Efficient Electrocatalytic CO2 Reduction
Krisch, D. and Sun, H. and Pellumbi, K. and Faust, K. and Apfel, U.-P. and Schöfberger, W.
CATALYSTS. Volume: 12 (2022) - 2022 • 7422
Angular-dependent interatomic potential for large-scale atomistic simulation of the Fe-Cr-H ternary system
Starikov, S. and Smirnova, D. and Pradhan, T. and Gordeev, I. and Drautz, R. and Mrovec, M.
PHYSICAL REVIEW MATERIALS. Volume: 6 (2022) - 2022 • 7421
Nematicity and nematic fluctuations in iron-based superconductors
Böhmer, A.E. and Chu, J.-H. and Lederer, S. and Yi, M.
NATURE PHYSICS. Volume: 18 (2022) - 2022 • 7420
On the interplay between microstructure, residual stress and fracture toughness of (Hf-Nb-Ta-Zr)C multi-metal carbide hard coatings
Gopalan, H. and Marshal, A. and Hans, M. and Primetzhofer, D. and Cautaerts, N. and Breitbach, B. and Völker, B. and Kirchlechner, C. and Schneider, J.M. and Dehm, G.
MATERIALS AND DESIGN. Volume: 224 (2022) - 2022 • 7419
Efficient Prediction of Grain Boundary Energies from Atomistic Simulations via Sequential Design
Kroll, M. and Schmalofski, T. and Dette, H. and Janisch, R.
ADVANCED THEORY AND SIMULATIONS. Volume: 5 (2022) - 2022 • 7418
THE INTEGRATED COPULA SPECTRUM
Goto, Y. and Kley, T. and van Hecke, R. and Volgushev, S. and Dette, H. and Hallin, M.
ANNALS OF STATISTICS. Volume: 50 (2022) - 2022 • 7417
Microstructure and Properties of a Novel Carbon-Martensitic Hot Work Tool Steel Processed by Laser Additive Manufacturing without Preheating
Boes, J. and Röttger, A. and Mutke, C. and Escher, C. and Weber, S.
STEEL RESEARCH INTERNATIONAL. Volume: (2022) - 2022 • 7416
Quantification of methods used in field metallography using the example of quality assurance measures for a circular economy for high-alloy steels [Quantifizierung von Methoden der Ambulanten Metallographie an qualitätssichernden Maßnahmen einer zirkulären Wertschöpfung hochlegierter Stähle]
Kronenberg, P. and Wieczorek, L. and Weber, S.L. and Röttger, A.
PRAKTISCHE METALLOGRAPHIE/PRACTICAL METALLOGRAPHY. Volume: 59 (2022) - 2022 • 7415
Feedback of Non-Local dxy Nematicity on the Magnetic Anisotropy in FeSe
Bötzel, S. and Eremin, I.M.
FRONTIERS IN PHYSICS. Volume: 10 (2022) - 2022 • 7414
Influence of crystal orientation on twinning in austenitic stainless-steel during single micro-asperity tribology and nanoindentation
Patil, P. and Lee, S. and Dehm, G. and Brinckmann, S.
WEAR. Volume: 504-505 (2022) - 2022 • 7413
Improving the Defect Tolerance of PBF-LB/M Processed 316L Steel by Increasing the Nitrogen Content
Stern, F. and Becker, L. and Cui, C. and Tenkamp, J. and Uhlenwinkel, V. and Steinbacher, M. and Boes, J. and Lentz, J. and Fechte-Heinen, R. and Weber, S. and Walther, F.
ADVANCED ENGINEERING MATERIALS. Volume: (2022) - 2022 • 7412
Quantum Dot Molecule Devices with Optical Control of Charge Status and Electronic Control of Coupling
Bopp, F. and Rojas, J. and Revenga, N. and Riedl, H. and Sbresny, F. and Boos, K. and Simmet, T. and Ahmadi, A. and Gershoni, D. and Kasprzak, J. and Ludwig, Ar. and Reitzenstein, S. and Wieck, A. and Reuter, D. and Müller, K. and Finley, J.J.
ADVANCED QUANTUM TECHNOLOGIES. Volume: 5 (2022) - 2022 • 7411
Spatial Distribution of Intracellular Ion Concentrations in Aggregate-Forming HeLa Cells Analyzed by μ-XRF Imaging
Gräfenstein, A. and Rumancev, C. and Pollak, R. and Hämisch, B. and Galbierz, V. and Schroeder, W.H. and Garrevoet, J. and Falkenberg, G. and Vöpel, T. and Huber, K. and Ebbinghaus, S. and Rosenhahn, A.
CHEMISTRYOPEN. Volume: 11 (2022) - 2022 • 7410
Machine-learning-based surrogate modeling of microstructure evolution using phase-field
Peivaste, I. and Siboni, N.H. and Alahyarizadeh, G. and Ghaderi, R. and Svendsen, B. and Raabe, D. and Mianroodi, J.R.
COMPUTATIONAL MATERIALS SCIENCE. Volume: 214 (2022) - 2022 • 7409
Dissecting Mechanochemistry III
Borchardt, L. and Hernández, J.G.
BEILSTEIN JOURNAL OF ORGANIC CHEMISTRY. Volume: 18 (2022) - 2022 • 7408
Opening the pathway towards a scalable electrochemical semi-hydrogenation of alkynols via earth-abundant metal chalcogenides
Pellumbi, K. and Wickert, L. and Kleinhaus, J.T. and Wolf, J. and Leonard, A. and Tetzlaff, D. and Goy, R. and Medlock, J.A. and junge Puring, K. and Cao, R. and Siegmund, D. and Apfel, U.-P.
CHEMICAL SCIENCE. Volume: (2022) - 2022 • 7407
Quantitative analysis of grain boundary diffusion, segregation and precipitation at a sub-nanometer scale
Peng, Z. and Meiners, T. and Lu, Y. and Liebscher, C.H. and Kostka, A. and Raabe, D. and Gault, B.
ACTA MATERIALIA. Volume: 225 (2022) - 2022 • 7406
Inverse modeling of cancellous bone using artificial neural networks
Stieve, V. and Blaszczyk, M. and Hackl, K.
ZAMM ZEITSCHRIFT FUR ANGEWANDTE MATHEMATIK UND MECHANIK. Volume: (2022) - 2022 • 7405
The mechanochemical synthesis of polymers
Krusenbaum, A. and Grätz, S. and Tigineh, G.T. and Borchardt, L. and Kim, J.G.
CHEMICAL SOCIETY REVIEWS. Volume: 51 (2022) - 2022 • 7404
General Surface-Casting Synthesis of Mesoporous Metal Oxides with Hollow Structures and Ultrahigh Surface Areas
Peng, Y. and Song, S. and Liu, F. and Yin, Z. and Zhong, Y. and Yi, X. and Zheng, A. and Schüth, F. and Gu, D.
CHEMISTRY OF MATERIALS. Volume: 34 (2022) - 2022 • 7403
The mechanochemical Friedel-Crafts polymerization as a solvent-free cross-linking approach toward microporous polymers
Krusenbaum, A. and Geisler, J. and Kraus, F.J.L. and Grätz, S. and Höfler, M.V. and Gutmann, T. and Borchardt, L.
JOURNAL OF POLYMER SCIENCE. Volume: 60 (2022) - 2022 • 7402
Semi-flowable Zn semi-solid electrodes as renewable energy carrier for refillable Zn–Air batteries
Perez-Antolin, D. and Schuhmann, W. and Palma, J. and Ventosa, E.
JOURNAL OF POWER SOURCES. Volume: 536 (2022) - 2022 • 7401
Carbon-nitrogen bond formation on Cu electrodes during CO2 reduction in NO3- solution
Krzywda, P.M. and Paradelo Rodríguez, A. and Benes, N.E. and Mei, B.T. and Mul, G.
APPLIED CATALYSIS B: ENVIRONMENTAL. Volume: 316 (2022) - 2022 • 7400
Effect of Electrolyte and Electrode Configuration on Cu-Catalyzed Nitric Oxide Reduction to Ammonia
Krzywda, P.M. and Paradelo Rodríguez, A. and Benes, N.E. and Mei, B.T. and Mul, G.
CHEMELECTROCHEM. Volume: 9 (2022) - 2022 • 7399
Operando X-ray Powder Diffraction Study of Mechanochemical Activation Tested for the CO Oxidation over Au@Fe2O3 as Model Reaction
Petersen, H. and De Bellis, J. and Leiting, S. and Das, S.M. and Schmidt, W. and Schüth, F. and Weidenthaler, C.
CHEMCATCHEM. Volume: 14 (2022) - 2022 • 7398
Electroreduction of NO3− on tubular porous Ti electrodes
Krzywda, P.M. and Paradelo Rodríguez, A. and Cino, L. and Benes, N.E. and Mei, B.T. and Mul, G.
CATALYSIS SCIENCE AND TECHNOLOGY. Volume: (2022) - 2022 • 7397
Advancing Critical Chemical Processes for a Sustainable Future: Challenges for Industry and the Max Planck–Cardiff Centre on the Fundamentals of Heterogeneous Catalysis (FUNCAT)
Bowker, M. and DeBeer, S. and Dummer, N.F. and Hutchings, G.J. and Scheffler, M. and Schüth, F. and Taylor, S.H. and Tüysüz, H.
ANGEWANDTE CHEMIE - INTERNATIONAL EDITION. Volume: (2022) - 2022 • 7396
Role of Anionic Backbone in NHC-Stabilized Coinage Metal Complexes: New Precursors for Atomic Layer Deposition**
Boysen, N. and Philip, A. and Rogalla, D. and Karppinen, M. and Devi, A.
CHEMISTRY - A EUROPEAN JOURNAL. Volume: (2022) - 2022 • 7395
Interplay of domain structure and phase transitions: Theory, experiment and functionality
Grünebohm, A. and Marathe, M. and Khachaturyan, R. and Schiedung, R. and Lupascu, D.C. and Shvartsman, V.V.
JOURNAL OF PHYSICS CONDENSED MATTER. Volume: 34 (2022) - 2022 • 7394
Dislocation structure analysis in the strain gradient of torsion loading: A comparison between modelling and experiment
Stricker, M. and Ziemann, M. and Walter, M. and Weygand, S.M. and Gruber, P. and Weygand, D.
MODELLING AND SIMULATION IN MATERIALS SCIENCE AND ENGINEERING. Volume: 30 (2022) - 2022 • 7393
High-Performance Iridium Thin Films for Water Splitting by CVD Using New Ir(I) Precursors
Boysen, N. and Wree, J.-L. and Zanders, D. and Rogalla, D. and Öhl, D. and Schuhmann, W. and Devi, A.
ACS APPLIED MATERIALS AND INTERFACES. Volume: 14 (2022) - 2022 • 7392
Thermicity of the Decomposition of Oxygen Functional Groups on Cellulose-Derived Chars
Pflieger, C. and Eckhard, T. and Schmitz, G. and Angenent, V. and Göckeler, M. and Senneca, O. and Schmid, R. and Cerciello, F. and Muhler, M.
ACS OMEGA. Volume: 7 (2022) - 2022 • 7391
Influence of a Partial Substitution of Co by Fe on the Phase Stability and Fatigue Behavior of a CoCrWC Hard Alloy at Room Temperature
Brackmann, L. and Schuppener, J. and Röttger, A. and Weber, S.
METALLURGICAL AND MATERIALS TRANSACTIONS A: PHYSICAL METALLURGY AND MATERIALS SCIENCE. Volume: 53 (2022) - 2022 • 7390
Foundations of physical vapor deposition with plasma assistance
Gudmundsson, J.T. and Anders, A. and von Keudell, A.
PLASMA SOURCES SCIENCE AND TECHNOLOGY. Volume: 31 (2022) - 2022 • 7389
First evidence of crucible steel production in Medieval Anatolia, Kubadabad: A trace for possible technology exchange between Anatolia and Southern Asia
Güder, Ü. and Çeken, M. and Yavaş, A. and Yalçın, Ü. and Raabe, D.
JOURNAL OF ARCHAEOLOGICAL SCIENCE. Volume: 137 (2022) - 2022 • 7388
Low-temperature ALD/MLD growth of alucone and zincone thin films from non-pyrophoric precursors
Philip, A. and Mai, L. and Ghiyasi, R. and Devi, A. and Karppinen, M.
DALTON TRANSACTIONS. Volume: 51 (2022) - 2022 • 7387
Electrooxidation of Alcohols on Mixed Copper–Cobalt Hydroxycarbonates in Alkaline Solution
Braun, M. and Behrendt, G. and Krebs, M.L. and Dimitri, P. and Kumar, P. and Sanjuán, I. and Cychy, S. and Brix, A.C. and Morales, D.M. and Hörlöck, J. and Hartke, B. and Muhler, M. and Schuhmann, W. and Behrens, M. and Andronescu, C.
CHEMELECTROCHEM. Volume: 9 (2022) - 2022 • 7386
The Direct Mechanocatalytic Suzuki–Miyaura Reaction of Small Organic Molecules
Pickhardt, W. and Beaković, C. and Mayer, M. and Wohlgemuth, M. and Kraus, F.J.L. and Etter, M. and Grätz, S. and Borchardt, L.
ANGEWANDTE CHEMIE - INTERNATIONAL EDITION. Volume: (2022) - 2022 • 7385
Discovery of High-Entropy Oxide Electrocatalysts: From Thin-Film Material Libraries to Particles
Strotkötter, V. and Krysiak, O.A. and Zhang, J. and Wang, X. and Suhr, E. and Schuhmann, W. and Ludwig, Al.
CHEMISTRY OF MATERIALS. Volume: 34 (2022) - 2022 • 7384
SUSTAINABILITY OF CONCRETE PAVEMENTS CONSIDERING TRAFFIC AND DE-ICING AGENTS
Breitenbücher, R. and Przondziono, R.
ACTA POLYTECHNICA CTU PROCEEDINGS. Volume: 33 (2022) - 2022 • 7383
Revisiting the Growth of Large (Mg,Zr):SrGa12O19Single Crystals: Core Formation and Its Impact on Structural Homogeneity Revealed by Correlative X-ray Imaging
Guguschev, C. and Richter, C. and Brützam, M. and Dadzis, K. and Hirschle, C. and Gesing, T.M. and Schulze, M. and Kwasniewski, A. and Schreuer, J. and Schlom, D.G.
CRYSTAL GROWTH AND DESIGN. Volume: 22 (2022) - 2022 • 7382
Indirect Electrooxidation of Methane to Methyl Bisulfate on a Boron-Doped Diamond Electrode
Britschgi, J. and Bilke, M. and Schuhmann, W. and Schüth, F.
CHEMELECTROCHEM. Volume: 9 (2022) - 2022 • 7381
Role-Specialized Division of Labor in CO2 Reduction with Doubly-Functionalized Iron Porphyrin Atropisomers
Guo, K. and Li, X. and Lei, H. and Guo, H. and Jin, X. and Zhang, X.-P. and Zhang, W. and Apfel, U.-P. and Cao, R.
ANGEWANDTE CHEMIE - INTERNATIONAL EDITION. Volume: 61 (2022) - 2022 • 7380
Adaptation of the Chemical Percolation Devolatilization Model for Low Temperature Pyrolysis in a Fluidized Bed Reactor
Pielsticker, S. and Ontyd, C. and Kreitzberg, T. and Hatzfeld, O. and Schiemann, M. and Scherer, V. and Kneer, R.
COMBUSTION SCIENCE AND TECHNOLOGY. Volume: 194 (2022) - 2022 • 7379
Electrochemically Initiated Synthesis of Methanesulfonic Acid
Britschgi, J. and Kersten, W. and Waldvogel, S.R. and Schüth, F.
ANGEWANDTE CHEMIE - INTERNATIONAL EDITION. Volume: (2022) - 2022 • 7378
Roadmap on Machine learning in electronic structure
Kulik, H.J. and Hammerschmidt, T. and Schmidt, J. and Botti, S. and Marques, M.A.L. and Boley, M. and Scheffler, M. and Todorović, M. and Rinke, P. and Oses, C. and Smolyanyuk, A. and Curtarolo, S. and Tkatchenko, A. and Bartók, A.P. and Manzhos, S. and Ihara, M. and Carrington, T. and Behler, J. and Isayev, O. and Veit, M. and Grisafi, A. and Nigam, J. and Ceriotti, M. and Schütt, K.T. and Westermayr, J. and Gastegger, M. and Maurer, R.J. and Kalita, B. and Burke, K. and Nagai, R. and Akashi, R. and Sugino, O. and Hermann, J. and Noé, F. and Pilati, S. and Draxl, C. and Kuban, M. and Rigamonti, S. and Scheidgen, M. and Esters, M. and Hicks, D. and Toher, C. and Balachandran, P.V. and Tamblyn, I. and Whitelam, S. and Bellinger, C. and Ghiringhelli, L.M.
ELECTRONIC STRUCTURE. Volume: 4 (2022) - 2022 • 7377
The influence of temperature on the strain-hardening behavior of Fe-22/25/28Mn-3Al-3Si TRIP/TWIP steels
Pierce, D.T. and Benzing, J.T. and Jiménez, J.A. and Hickel, T. and Bleskov, I. and Keum, J. and Raabe, D. and Wittig, J.E.
MATERIALIA. Volume: 22 (2022) - 2022 • 7376
The AlMo0.5NbTa0.5TiZr refractory high entropy superalloy: Experimental findings and comparison with calculations using the CALPHAD method
Suárez Ocaño, P. and Fries, S.G. and Lopez-Galilea, I. and Darvishi Kamachali, R. and Roik, J. and Agudo Jácome, L.
MATERIALS AND DESIGN. Volume: 217 (2022) - 2022 • 7375
Structure-Performance Relationship of LaFe1-xCoxO3 Electrocatalysts for Oxygen Evolution, Isopropanol Oxidation, and Glycerol Oxidation
Brix, A.C. and Dreyer, M. and Koul, A. and Krebs, M. and Rabe, A. and Hagemann, U. and Varhade, S. and Andronescu, C. and Behrens, M. and Schuhmann, W. and Morales, D.M.
CHEMELECTROCHEM. Volume: 9 (2022) - 2022 • 7374
Defective Metal-Organic Frameworks with Tunable Porosity and Metal Active Sites for Significantly Improved Performance in Styrene Oxidation
Guo, P. and Cheng, H. and Zeng, X. and Tao, L. and Peng, B. and Zhang, S. and Fischer, R.A. and Muhler, M.
CHEMPLUSCHEM. Volume: 87 (2022) - 2022 • 7373
Influence of the ZnCrAl Oxide Composition on the Formation of Hydrocarbons from Syngas
Kull, T. and Wiesmann, T. and Wilmsen, A. and Purcel, M. and Muhler, M. and Lohmann, H. and Zeidler-Fandrich, B. and Apfel, U.-P.
ACS OMEGA. Volume: 7 (2022) - 2022 • 7372
Recrystallization in non-conventional microstructures of 316L stainless steel produced via laser powder-bed fusion: effect of particle coarsening kinetics
Pinto, F.C. and Aota, L.S. and Souza Filho, I.R. and Raabe, D. and Sandim, H.R.Z.
JOURNAL OF MATERIALS SCIENCE. Volume: (2022) - 2022 • 7371
Application of Design of Experiments for Catalytic Oxygen Removal over Pt/γ-Al2O3 Catalyst
Suh, S.Y. and Geitner, C. and Hänel, M. and Wiesmann, T. and Watermann, C.M. and Lohmann, H. and Apfel, U.-P. and Zeidler-Fandrich, B.
CHEMIE-INGENIEUR-TECHNIK. Volume: 94 (2022) - 2022 • 7370
Effect of composition and nanostructure on the mechanical properties and thermal stability of Zr100-xCux thin film metallic glasses
Brognara, A. and Best, J.P. and Djemia, P. and Faurie, D. and Dehm, G. and Ghidelli, M.
MATERIALS AND DESIGN. Volume: 219 (2022) - 2022 • 7369
Unusual Phase Formation in Reactively Sputter-Deposited La—Co—O Thin-Film Libraries
Piotrowiak, T.H. and Zehl, R. and Suhr, E. and Banko, L. and Kohnen, B. and Rogalla, D. and Ludwig, Al.
ADVANCED ENGINEERING MATERIALS. Volume: (2022) - 2022 • 7368
Rejuvenation in Deep Thermal Cycling of a Generic Model Glass: A Study of Per-Particle Energy Distribution
Bruns, M. and Varnik, F.
MATERIALS. Volume: 15 (2022) - 2022 • 7367
Antimicrobial and Antibiofouling Electrically Conducting Laser-Induced Graphene Spacers in Reverse Osmosis Membrane Modules
Pisharody, L. and Thamaraiselvan, C. and Manderfeld, E. and Singh, S.P. and Rosenhahn, A. and Arnusch, C.J.
ADVANCED MATERIALS INTERFACES. Volume: (2022) - 2022 • 7366
Thermodynamics-guided alloy and process design for additive manufacturing
Sun, Z. and Ma, Y. and Ponge, D. and Zaefferer, S. and Jägle, E.A. and Gault, B. and Rollett, A.D. and Raabe, D.
NATURE COMMUNICATIONS. Volume: 13 (2022) - 2022 • 7365
Enhanced dynamics in deep thermal cycling of a model glass
Bruns, M. and Varnik, F.
JOURNAL OF CHEMICAL PHYSICS. Volume: 156 (2022) - 2022 • 7364
The role of electrons during the martensitic phase transformation in NiTi-based shape memory alloys
Kunzmann, A. and Frenzel, J. and Wolff, U. and Han, J.W. and Giebeler, L. and Piorunek, D. and Mittendorff, M. and Scheiter, J. and Reith, H. and Perez, N. and Nielsch, K. and Eggeler, G. and Schierning, G.
MATERIALS TODAY PHYSICS. Volume: 24 (2022) - 2022 • 7363
Physical metallurgy of medium-Mn advanced high-strength steels
Sun, B. and Kwiatkowski da Silva, A. and Wu, Y. and Ma, Y. and Chen, H. and Scott, C. and Ponge, D. and Raabe, D.
INTERNATIONAL MATERIALS REVIEWS. Volume: (2022) - 2022 • 7362
Real-Time Observation of Charge-Spin Cooperative Dynamics Driven by a Nonequilibrium Phonon Environment
Kuroyama, K. and Matsuo, S. and Muramoto, J. and Yabunaka, S. and Valentin, S.R. and Ludwig, Ar. and Wieck, A.D. and Tokura, Y. and Tarucha, S.
PHYSICAL REVIEW LETTERS. Volume: 129 (2022) - 2022 • 7361
Structure-activity correlation in aerobic cyclohexene oxidation and peroxide decomposition over CoxFe3−xO4 spinel oxides
Büker, J. and Angel, S. and Salamon, S. and Landers, J. and Falk, T. and Wende, H. and Wiggers, H. and Schulz, C. and Muhler, M. and Peng, B.
CATALYSIS SCIENCE AND TECHNOLOGY. Volume: 12 (2022) - 2022 • 7360
Statistical inference for the slope parameter in functional linear regression
Kutta, T. and Dierickx, G. and Dette, H.
ELECTRONIC JOURNAL OF STATISTICS. Volume: 16 (2022) - 2022 • 7359
A single-Pt-atom-on-Ru-nanoparticle electrocatalyst for CO-resilient methanol oxidation
Poerwoprajitno, A.R. and Gloag, L. and Watt, J. and Cheong, S. and Tan, X. and Lei, H. and Tahini, H.A. and Henson, A. and Subhash, B. and Bedford, N.M. and Miller, B.K. and O’Mara, P.B. and Benedetti, T.M. and Huber, D.L. and Zhang, W. and Smith, S.C. and Gooding, J.J. and Schuhmann, W. and Tilley, R.D.
NATURE CATALYSIS. Volume: 5 (2022) - 2022 • 7358
Slotted Y-branch laser for cw-THz thickness measurements at 1 THz
Surkamp, N. and Gerling, A. and OGorman, J. and Honsberg, M. and Schmidtmann, S. and Nandi, U. and Preu, S. and Sacher, J. and Brenner, C. and Hofmann, M.R.
PROCEEDINGS OF SPIE - THE INTERNATIONAL SOCIETY FOR OPTICAL ENGINEERING. Volume: 12021 (2022) - 2022 • 7357
A CutFEM based framework for numerical simulations of machine driven tunnels with arbitrary alignments
Bui, H.-G. and Schillinger, D. and Zendaki, Y. and Meschke, G.
COMPUTERS AND GEOTECHNICS. Volume: 144 (2022) - 2022 • 7356
More than recycling – The potential of the circular economy shown by a case study of the metal working industry
Hagedorn, W. and Jäger, S. and Wieczorek, L. and Kronenberg, P. and Greiff, K. and Weber, S. and Roettger, A.
JOURNAL OF CLEANER PRODUCTION. Volume: 377 (2022) - 2022 • 7355
Novel Development of an NbC-Containing Powder-Metallurgical Martensitic Steel with Outstanding Tribocorrosion Resistance
Hahn, I. and Siebert, S. and Fluch, R. and Theisen, W. and Weber, S.
STEEL RESEARCH INTERNATIONAL. Volume: (2022) - 2022 • 7354
Practical guide to replica exchange transition interface sampling and forward flux sampling
Hall, S.W. and Díaz Leines, G. and Sarupria, S. and Rogal, J.
JOURNAL OF CHEMICAL PHYSICS. Volume: 156 (2022) - 2022 • 7353
A sustainable ultra-high strength Fe18Mn3Ti maraging steel through controlled solute segregation and α-Mn nanoprecipitation
Kwiatkowski da Silva, A. and Souza Filho, I.R. and Lu, W. and Zilnyk, K.D. and Hupalo, M.F. and Alves, L.M. and Ponge, D. and Gault, B. and Raabe, D.
NATURE COMMUNICATIONS. Volume: 13 (2022) - 2022 • 7352
Temperature Rise Inside Shear Bands in a Simple Model Glass
Lagogianni, A.E. and Varnik, F.
INTERNATIONAL JOURNAL OF MOLECULAR SCIENCES. Volume: 23 (2022) - 2022 • 7351
Ion-induced secondary electron emission of oxidized nickel and copper studied in beam experiments
Buschhaus, R. and Prenzel, M. and Von Keudell, A.
PLASMA SOURCES SCIENCE AND TECHNOLOGY. Volume: 31 (2022) - 2022 • 7350
Identification of texture characteristics for improved creep behavior of a L-PBF fabricated IN738 alloy through micromechanical simulations
Prasad, M.R.G. and Biswas, A. and Vajragupta, N. and Hartmaier, A.
MODELLING AND SIMULATION IN MATERIALS SCIENCE AND ENGINEERING. Volume: 30 (2022) - 2022 • 7349
Combustion of Straw Pellets in an Agitated Fuel Bed: Experiments and DEM/CFD Simulations
Buss, F. and Wirtz, S. and Scherer, V.
COMBUSTION SCIENCE AND TECHNOLOGY. Volume: 194 (2022) - 2022 • 7348
A mechanically strong and ductile soft magnet with extremely low coercivity
Han, L. and Maccari, F. and Souza Filho, I.R. and Peter, N.J. and Wei, Y. and Gault, B. and Gutfleisch, O. and Li, Z. and Raabe, D.
NATURE. Volume: 608 (2022) - 2022 • 7347
NdFeB Magnets with Well-Pronounced Anisotropic Magnetic Properties Made by Electric Current-Assisted Sintering
Prasad Mishra, T. and Leich, L. and Krengel, M. and Weber, S. and Röttger, A. and Bram, M.
ADVANCED ENGINEERING MATERIALS. Volume: (2022) - 2022 • 7346
Strain rate dependent deformation behavior of BCC-structured Ti29Zr24Nb23Hf24 high entropy alloy at elevated temperatures
Cao, T. and Guo, W. and Lu, W. and Xue, Y. and Lu, W. and Su, J. and Liebscher, C.H. and Li, C. and Dehm, G.
JOURNAL OF ALLOYS AND COMPOUNDS. Volume: 891 (2022) - 2022 • 7345
High-pressure CO, H2, CO2 and Ethylene Pulses Applied in the Hydrogenation of CO to Higher Alcohols over a Bulk Co-Cu Catalyst
Telaar, P. and Schwiderowski, P. and Schmidt, S. and Stürmer, S. and Muhler, M.
CHEMCATCHEM. Volume: 14 (2022) - 2022 • 7344
Real-Time Risk Assessment of Tunneling-Induced Building Damage Considering Polymorphic Uncertainty
Cao, B.T. and Obel, M. and Freitag, S. and Heußner, L. and Meschke, G. and Mark, P.
ASCE-ASME JOURNAL OF RISK AND UNCERTAINTY IN ENGINEERING SYSTEMS, PART A: CIVIL ENGINEERING. Volume: 8 (2022) - 2022 • 7343
Bioinspired iron porphyrins with appended poly-pyridine/amine units for boosted electrocatalytic CO2 reduction reaction
Han, J. and Wang, N. and Li, X. and Lei, H. and Wang, Y. and Guo, H. and Jin, X. and Zhang, Q. and Peng, X. and Zhang, X.-P. and Zhang, W. and Apfel, U.-P. and Cao, R.
ESCIENCE. Volume: 2 (2022) - 2022 • 7342
Investigation of phase transformation related electrical conductivity of long-term heat treated aluminium electrolysis cathodes
Hankel, J. and Kernebeck, S. and Deuerler, F. and Weber, S.
SN APPLIED SCIENCES. Volume: 4 (2022) - 2022 • 7341
Free, flexible and fast: Orientation mapping using the multi-core and GPU-accelerated template matching capabilities in the Python-based open source 4D-STEM analysis toolbox Pyxem
Cautaerts, N. and Crout, P. and Ånes, H.W. and Prestat, E. and Jeong, J. and Dehm, G. and Liebscher, C.H.
ULTRAMICROSCOPY. Volume: 237 (2022) - 2022 • 7340
Processability of a Hot Work Tool Steel Powder Mixture in Laser-Based Powder Bed Fusion
Hantke, N. and Großwendt, F. and Strauch, A. and Fechte-Heinen, R. and Röttger, A. and Theisen, W. and Weber, S. and Sehrt, J.T.
MATERIALS. Volume: 15 (2022) - 2022 • 7339
Effects of aging on the stress-induced martensitic transformation and cyclic superelastic properties in Co-Ni-Ga shape memory alloy single crystals under compression
Lauhoff, C. and Reul, A. and Langenkämper, D. and Krooß, P. and Somsen, C. and Gutmann, M.J. and Pedersen, B. and Kireeva, I.V. and Chumlyakov, Y.I. and Eggeler, G. and Schmahl, W.W. and Niendorf, T.
ACTA MATERIALIA. Volume: 226 (2022) - 2022 • 7338
Linear response for pseudo-Hermitian Hamiltonian systems: Application to PT -symmetric qubits
Tetling, L. and Fistul, M.V. and Eremin, I.M.
PHYSICAL REVIEW B. Volume: 106 (2022) - 2022 • 7337
A Novel Thermo-Mechanical Processing Route Exploiting Abnormal Grain Growth in Heusler-Type Co–Ni–Ga Shape Memory Alloys
Lauhoff, C. and Pham, T. and Paulsen, A. and Krooß, P. and Frenzel, J. and Eggeler, G. and Niendorf, T.
METALLURGICAL AND MATERIALS TRANSACTIONS A: PHYSICAL METALLURGY AND MATERIALS SCIENCE. Volume: 53 (2022) - 2022 • 7336
[NiFe]-(Oxy)Sulfides Derived from NiFe2O4 for the Alkaline Hydrogen Evolution Reaction
Tetzlaff, D. and Alagarasan, V. and Simon, C. and Siegmund, D. and Puring, K.J. and Marschall, R. and Apfel, U.-P.
ENERGIES. Volume: 15 (2022) - 2022 • 7335
Aerosol-Based Synthesis of Multi-metal Electrocatalysts for Oxygen Evolution and Glycerol Oxidation
Cechanaviciute, I.A. and Bobrowski, T. and Jambrec, D. and Krysiak, O.A. and Brix, A.C. and Braun, M. and Quast, T. and Wilde, P. and Morales, D.M. and Andronescu, C. and Schuhmann, W.
CHEMELECTROCHEM. Volume: 9 (2022) - 2022 • 7334
Silver Thin-Film Electrodes Grown by Low-Temperature Plasma-Enhanced Spatial Atomic Layer Deposition at Atmospheric Pressure
Hasselmann, T. and Misimi, B. and Boysen, N. and Zanders, D. and Wree, J.-L. and Rogalla, D. and Haeger, T. and Zimmermann, F. and Brinkmann, K.O. and Schädler, S. and Theirich, D. and Heiderhoff, R. and Devi, A. and Riedl, T.
ADVANCED MATERIALS TECHNOLOGIES. Volume: (2022) - 2022 • 7333
Formation of tungsten carbide by focused ion beam process: A route to high magnetic field resilient patterned superconducting nanostructures
Chakraborti, H. and Joshi, B.P. and Barman, C.K. and Jain, A.K. and Pal, B. and Barik, B.C. and Maiti, T. and Schott, R. and Wieck, A.D. and Prasad, M.J.N.V. and Dhar, S. and Pal, H.K. and Alam, A. and Das Gupta, K.
APPLIED PHYSICS LETTERS. Volume: 120 (2022) - 2022 • 7332
Microstructure and residual stress evolution in nanocrystalline Cu-Zr thin films
Chakraborty, J. and Oellers, T. and Raghavan, R. and Ludwig, A. and Dehm, G.
JOURNAL OF ALLOYS AND COMPOUNDS. Volume: 896 (2022) - 2022 • 7331
Splicing the active phases of copper/cobalt-based catalysts achieves high-rate tandem electroreduction of nitrate to ammonia
He, W. and Zhang, J. and Dieckhöfer, S. and Varhade, S. and Brix, A.C. and Lielpetere, A. and Seisel, S. and Junqueira, J.R.C. and Schuhmann, W.
NATURE COMMUNICATIONS. Volume: 13 (2022) - 2022 • 7330
Diffusion chronometry of volcanic rocks: looking backward and forward
Chakraborty, S. and Dohmen, R.
BULLETIN OF VOLCANOLOGY. Volume: 84 (2022) - 2022 • 7329
In-flight detection of few electrons using a singlet-triplet spin qubit
Thiney, V. and Mortemousque, P.-A. and Rogdakis, K. and Thalineau, R. and Ludwig, Ar. and Wieck, A.D. and Urdampilleta, M. and Bäuerle, C. and Meunier, T.
PHYSICAL REVIEW RESEARCH. Volume: 4 (2022) - 2022 • 7328
Potential of novel porous materials for capture of toluene traces in air under humid conditions
Pujol, Q. and Weber, G. and Bellat, J.-P. and Grätz, S. and Krusenbaum, A. and Borchardt, L. and Bezverkhyy, I.
MICROPOROUS AND MESOPOROUS MATERIALS. Volume: 344 (2022) - 2022 • 7327
The effect of deviations from precise [001] tensile direction on creep of Ni-base single crystal superalloys
Heep, L. and Bürger, D. and Bonnekoh, C. and Wollgramm, P. and Dlouhy, A. and Eggeler, G.
SCRIPTA MATERIALIA. Volume: 207 (2022) - 2022 • 7326
Metal-Corrole-Based Porous Organic Polymers for Electrocatalytic Oxygen Reduction and Evolution Reactions
Lei, H. and Zhang, Q. and Liang, Z. and Guo, H. and Wang, Y. and Lv, H. and Li, X. and Zhang, W. and Apfel, U.-P. and Cao, R.
ANGEWANDTE CHEMIE - INTERNATIONAL EDITION. Volume: 61 (2022) - 2022 • 7325
Crystallographic Analysis of Plate and Lath Martensite in Fe-Ni Alloys
Thome, P. and Schneider, M. and Yardley, V.A. and Payton, E.J. and Eggeler, G.
CRYSTALS. Volume: 12 (2022) - 2022 • 7324
Spin-Lasing in Bimodal Quantum Dot Micropillar Cavities
Heermeier, N. and Heuser, T. and Große, J. and Jung, N. and Kaganskiy, A. and Lindemann, M. and Gerhardt, N.C. and Hofmann, M.R. and Reitzenstein, S.
LASER AND PHOTONICS REVIEWS. Volume: 16 (2022) - 2022 • 7323
Publisher Correction: Dynamical photon–photon interaction mediated by a quantum emitter (Nature Physics, (2022), 18, 10, (1191-1195), 10.1038/s41567-022-01720-x)
Le Jeannic, H. and Tiranov, A. and Carolan, J. and Ramos, T. and Wang, Y. and Appel, M.H. and Scholz, S. and Wieck, A.D. and Ludwig, Ar. and Rotenberg, N. and Midolo, L. and García-Ripoll, J.J. and Sørensen, A.S. and Lodahl, P.
NATURE PHYSICS. Volume: 18 (2022) - 2022 • 7322
Improving the intermediate- and high-temperature strength of L12-Co3(Al,W) by Ni and Ta additions
Chen, Z. and Kishida, K. and Inui, H. and Heilmaier, M. and Glatzel, U. and Eggeler, G.
ACTA MATERIALIA. Volume: 238 (2022) - 2022 • 7321
Boosting the overall electrochemical water splitting performance of pentlandites through non-metallic heteroatom incorporation
Hegazy, M.B.Z. and Harrath, K. and Tetzlaff, D. and Smialkowski, M. and Siegmund, D. and Li, J. and Cao, R. and Apfel, U.-P.
ISCIENCE. Volume: 25 (2022) - 2022 • 7320
Lower degree of dissociation of pyruvic acid at water surfaces than in bulk
Lesnicki, D. and Wank, V. and Cyran, J.D. and Backus, E.H.G. and Sulpizi, M.
PHYSICAL CHEMISTRY CHEMICAL PHYSICS. Volume: 24 (2022) - 2022 • 7319
Making sustainable aluminum by recycling scrap: The science of “dirty” alloys
Raabe, D. and Ponge, D. and Uggowitzer, P.J. and Roscher, M. and Paolantonio, M. and Liu, C. and Antrekowitsch, H. and Kozeschnik, E. and Seidmann, D. and Gault, B. and De Geuser, F. and Deschamps, A. and Hutchinson, C. and Liu, C. and Li, Z. and Prangnell, P. and Robson, J. and Shanthraj, P. and Vakili, S. and Sinclair, C. and Bourgeois, L. and Pogatscher, S.
PROGRESS IN MATERIALS SCIENCE. Volume: 128 (2022) - 2022 • 7318
Ab initio molecular dynamics simulation of vibrational energy relaxation at the solid/liquid interface: Charge defects at the fluorite/water interface allow very fast intermolecular vibrational energy transfer
Lesnicki, D. and Sulpizi, M.
HIGH PERFORMANCE COMPUTING IN SCIENCE AND ENGINEERING '20: TRANSACTIONS OF THE HIGH PERFORMANCE COMPUTING CENTER, STUTTGART (HLRS) 2020. Volume: (2022) - 2022 • 7317
Introducing Stacking Faults into Three-Dimensional Branched Nickel Nanoparticles for Improved Catalytic Activity
Ramadhan, Z.R. and Poerwoprajitno, A.R. and Cheong, S. and Webster, R.F. and Kumar, P.V. and Cychy, S. and Gloag, L. and Benedetti, T.M. and Marjo, C.E. and Muhler, M. and Wang, D.-W. and Gooding, J.J. and Schuhmann, W. and Tilley, R.D.
JOURNAL OF THE AMERICAN CHEMICAL SOCIETY. Volume: 144 (2022) - 2022 • 7316
Effect of microstructure heterogeneity on the mechanical properties of friction stir welded reduced activation ferritic/martensitic steel
Li, S. and Vajragupta, N. and Biswas, A. and Tang, W. and Wang, H. and Kostka, A. and Yang, X. and Hartmaier, A.
SCRIPTA MATERIALIA. Volume: 207 (2022) - 2022 • 7315
Effects of thermal, elastic, and surface properties on the stability of SiC polytypes
Ramakers, S. and Marusczyk, A. and Amsler, M. and Eckl, T. and Mrovec, M. and Hammerschmidt, T. and Drautz, R.
PHYSICAL REVIEW B. Volume: 106 (2022) - 2022 • 7314
On the Tunability of Mode Locked Laser Diodes for Use as Local Oscillators in Photonic Terahertz Systems
Cherniak, V. and Zander, M. and Moehrle, M. and Rehbein, W. and Brenner, C. and Hofmann, M. and Balzer, J.C.
INTERNATIONAL CONFERENCE ON INFRARED, MILLIMETER, AND TERAHERTZ WAVES, IRMMW-THZ. Volume: 2022-August (2022) - 2022 • 7313
A Study of the Usability of Monolithically Integrated Photonic Oscillators for Wireless Millimeter Wave and Terahertz Communication
Cherniak, V. and Endemann, W. and Frischkorn, B. and Kleemann, N. and Brenner, C. and Hofmann, M. and Balzer, J.C.
INTERNATIONAL CONFERENCE ON INFRARED, MILLIMETER, AND TERAHERTZ WAVES, IRMMW-THZ. Volume: 2022-August (2022) - 2022 • 7312
Introducing Water-Network-Assisted Proton Transfer for Boosted Electrocatalytic Hydrogen Evolution with Cobalt Corrole
Li, X. and Lv, B. and Zhang, X.-P. and Jin, X. and Guo, K. and Zhou, D. and Bian, H. and Zhang, W. and Apfel, U.-P. and Cao, R.
ANGEWANDTE CHEMIE - INTERNATIONAL EDITION. Volume: 61 (2022) - 2022 • 7311
A Data-driven Multi-fidelity Physics-informed Learning Framework for Smart Manufacturing: A Composites Processing Case Study
Ramezankhani, M. and Nazemi, A. and Narayan, A. and Voggenreiter, H. and Harandi, M. and Seethaler, R. and Milani, A.S.
PROCEEDINGS - 2022 IEEE 5TH INTERNATIONAL CONFERENCE ON INDUSTRIAL CYBER-PHYSICAL SYSTEMS, ICPS 2022. Volume: (2022) - 2022 • 7310
Spoke-resolved electron density, temperature and potential in direct current magnetron sputtering and HiPIMS discharges
Held, J. and George, M. and von Keudell, A.
PLASMA SOURCES SCIENCE AND TECHNOLOGY. Volume: 31 (2022) - 2022 • 7309
Experimental investigation of laser surface texturing and related biocompatibility of pure titanium
Li, H. and Wang, X. and Zhang, J. and Wang, B. and Breisch, M. and Hartmaier, A. and Rostotskyi, I. and Voznyy, V. and Liu, Y.
INTERNATIONAL JOURNAL OF ADVANCED MANUFACTURING TECHNOLOGY. Volume: (2022) - 2022 • 7308
Trimethylamine Probes Isolated Silicon Dangling Bonds and Surface Hydroxyls of (H,OH)-Si(001)
Ramírez, L.P. and Fornefeld, N. and Bournel, F. and Kubsky, S. and Magnano, E. and Bondino, F. and Köhler, U. and Carniato, S. and Gallet, J.-J. and Rochet, F.
JOURNAL OF PHYSICAL CHEMISTRY C. Volume: 126 (2022) - 2022 • 7307
Elucidation of formation and transformation mechanisms of Ca-rich Laves phase in Mg-Al-Ca-Mn alloys
Li, J. and Zhou, X. and Breen, A. and Peng, Z. and Su, J. and Kürnsteiner, P. and Correa, M.J.D. and Chwałek, M.L. and Wang, H. and Holec, D. and Mayer, J. and Dehm, G.
JOURNAL OF ALLOYS AND COMPOUNDS. Volume: 928 (2022) - 2022 • 7306
Elucidating dynamic precipitation and yield strength of rolled Mg–Al–Ca–Mn alloy
Li, J. and Zhou, X. and Su, J. and Breitbach, B. and Chwałek, M.L. and Wang, H. and Dehm, G.
MATERIALS SCIENCE AND ENGINEERING A. Volume: 856 (2022) - 2022 • 7305
Machine learning–enabled high-entropy alloy discovery
Rao, Z. and Tung, P.-Y. and Xie, R. and Wei, Y. and Zhang, H. and Ferrari, A. and Klaver, T.P.C. and Körmann, F. and Sukumar, P.T. and da Silva, A.K. and Chen, Y. and Li, Z. and Ponge, D. and Neugebauer, J. and Gutfleisch, O. and Bauer, S. and Raabe, D.
SCIENCE. Volume: 378 (2022) - 2022 • 7304
Phase transformations and microstructure evolution during combustion of iron powder
Choisez, L. and van Rooij, N.E. and Hessels, C.J.M. and da Silva, A.K. and Filho, I.R.S. and Ma, Y. and de Goey, P. and Springer, H. and Raabe, D.
ACTA MATERIALIA. Volume: 239 (2022) - 2022 • 7303
Model-free data-driven simulation of inelastic materials using structured data sets, tangent space information and transition rules
Ciftci, K. and Hackl, K.
COMPUTATIONAL MECHANICS. Volume: 70 (2022) - 2022 • 7302
Advances and challenges in photosynthetic hydrogen production
Redding, K.E. and Appel, J. and Boehm, M. and Schuhmann, W. and Nowaczyk, M.M. and Yacoby, I. and Gutekunst, K.
TRENDS IN BIOTECHNOLOGY. Volume: 40 (2022) - 2022 • 7301
A Hessian-based assessment of atomic forces for training machine learning interatomic potentials
Herbold, M. and Behler, J.
JOURNAL OF CHEMICAL PHYSICS. Volume: 156 (2022) - 2022 • 7300
Atomic-scale characterization of (electro-)catalysts and battery materials by atom probe tomography
Li, T. and Devaraj, A. and Kruse, N.
CELL REPORTS PHYSICAL SCIENCE. Volume: 3 (2022) - 2022 • 7299
High-order nonlinear terahertz probing of the two-band superconductor MgB2: Third- and fifth-order harmonic generation
Reinhoffer, C. and Pilch, P. and Reinold, A. and Derendorf, P. and Kovalev, S. and Deinert, J.-C. and Ilyakov, I. and Ponomaryov, A. and Chen, M. and Xu, T.-Q. and Wang, Y. and Gan, Z.-Z. and Wu, D.-S. and Luo, J.-L. and Germanskiy, S. and Mashkovich, E.A. and Van Loosdrecht, P.H.M. and Eremin, I.M. and Wang, Z.
PHYSICAL REVIEW B. Volume: 106 (2022) - 2022 • 7298
SO(4) multicriticality of two-dimensional Dirac fermions
Herbut, I.F. and Scherer, M.M.
PHYSICAL REVIEW B. Volume: 106 (2022) - 2022 • 7297
Extrusion process simulation and layer shape prediction during 3D-concrete-printing using the Particle Finite Element Method
Reinold, J. and Nerella, V.N. and Mechtcherine, V. and Meschke, G.
AUTOMATION IN CONSTRUCTION. Volume: 136 (2022) - 2022 • 7296
Algorithm for aging materials with evolving stiffness based on a multiplicative split
Reinold, J. and Meschke, G.
COMPUTER METHODS IN APPLIED MECHANICS AND ENGINEERING. Volume: 397 (2022) - 2022 • 7295
50-W average power Ho:YAG SESAM-modelocked thin-disk oscillator at 2.1 µm
Tomilov, S. and Wang, Y. and Hoffmann, M. and Heidrich, J. and Golling, M. and Keller, U. and Saraceno, C.J.
OPTICS EXPRESS. Volume: 30 (2022) - 2022 • 7294
Non-gradient full waveform inversion approaches for exploration during mechanized tunneling applied to surrogate laboratory measurements
Trapp, M. and Nestorović, T.
TUNNELLING AND UNDERGROUND SPACE TECHNOLOGY. Volume: 120 (2022) - 2022 • 7293
Massive interstitial solid solution alloys achieve near-theoretical strength
Liu, C. and Lu, W. and Xia, W. and Du, C. and Rao, Z. and Best, J.P. and Brinckmann, S. and Lu, J. and Gault, B. and Dehm, G. and Wu, G. and Li, Z. and Raabe, D.
NATURE COMMUNICATIONS. Volume: 13 (2022) - 2022 • 7292
Differential Tafel Analysis: A Quick and Robust Tool to Inspect and Benchmark Charge Transfer in Electrocatalysis
Corva, M. and Blanc, N. and Bondue, C.J. and Tschulik, K.
ACS CATALYSIS. Volume: (2022) - 2022 • 7291
Terahertz Beam Steering Using a MEMS-Based Reflectarray Configured by a Genetic Algorithm
Liu, X. and Schmitt, L. and Sievert, B. and Lipka, J. and Geng, C. and Kolpatzeck, K. and Erni, D. and Rennings, A. and Balzer, J.C. and Hoffmann, M. and Czylwik, A.
IEEE ACCESS. Volume: 10 (2022) - 2022 • 7290
Mechanochemically-Assisted Synthesis of Polyimides
Rensch, T. and Fabig, S. and Grätz, S. and Borchardt, L.
CHEMSUSCHEM. Volume: 15 (2022) - 2022 • 7289
A biophotoelectrode based on boronic acid-modified Chlorella vulgaris cells integrated within a redox polymer
Herrero-Medina, Z. and Wang, P. and Lielpetere, A. and Bashammakh, A.S. and Alyoubi, A.O. and Katakis, I. and Conzuelo, F. and Schuhmann, W.
BIOELECTROCHEMISTRY. Volume: 146 (2022) - 2022 • 7288
CALPHAD-informed phase-field model for two-sublattice phases based on chemical potentials: η-phase precipitation in Al-Zn-Mg-Cu alloys
Liu, C. and Davis, A. and Fellowes, J. and Prangnell, P.B. and Raabe, D. and Shanthraj, P.
ACTA MATERIALIA. Volume: 226 (2022) - 2022 • 7287
Scale-Up of Solvent-Free, Mechanochemical Precursor Synthesis for Nanoporous Carbon Materials via Extrusion
Rensch, T. and Chantrain, V. and Sander, M. and Grätz, S. and Borchardt, L.
CHEMSUSCHEM. Volume: (2022) - 2022 • 7286
Deformation and phase transformation in polycrystalline cementite (Fe3C) during single- and multi-pass sliding wear
Tsybenko, H. and Tian, C. and Rau, J. and Breitbach, B. and Schreiber, P. and Greiner, C. and Dehm, G. and Brinckmann, S.
ACTA MATERIALIA. Volume: 227 (2022) - 2022 • 7285
Optimizing the Radiation Pattern of a MEMS-Based Reflectarray Using a Genetic Algorithm for Beam Steering Applications
Liu, X. and Schmitt, L. and Lipka, J. and Kolpatzeck, K. and Balzer, J.C. and Hoffmann, M. and Czylwik, A.
INTERNATIONAL CONFERENCE ON INFRARED, MILLIMETER, AND TERAHERTZ WAVES, IRMMW-THZ. Volume: 2022-August (2022) - 2022 • 7284
On the impact of nanometric γ’ precipitates on the tensile deformation of superelastic Co49Ni21Ga30
Reul, A. and Lauhoff, C. and Krooß, P. and Somsen, C. and Langenkämper, D. and Gutmann, M.J. and Pedersen, B. and Hofmann, M. and Gan, W.M. and Kireeva, I. and Chumlyakov, Y.I. and Eggeler, G. and Niendorf, T. and Schmahl, W.W.
ACTA MATERIALIA. Volume: 230 (2022) - 2022 • 7283
Laser Additive Manufacturing of Duplex Stainless Steel via Powder Mixture
Cui, C. and Becker, L. and Gärtner, E. and Boes, J. and Lentz, J. and Uhlenwinkel, V. and Steinbacher, M. and Weber, S. and Fechte-Heinen, R.
JOURNAL OF MANUFACTURING AND MATERIALS PROCESSING. Volume: 6 (2022) - 2022 • 7282
Facet-Dependent Intrinsic Activity of Single Co3O4 Nanoparticles for Oxygen Evolution Reaction
Liu, Z. and Amin, H.M.A. and Peng, Y. and Corva, M. and Pentcheva, R. and Tschulik, K.
ADVANCED FUNCTIONAL MATERIALS. Volume: (2022) - 2022 • 7281
Influence of machining on the surface integrity of high- and medium-entropy alloys
Richter, T. and Schroepfer, D. and Rhode, M. and Boerner, A. and Neumann, R.S. and Schneider, M. and Laplanche, G.
MATERIALS CHEMISTRY AND PHYSICS. Volume: 275 (2022) - 2022 • 7280
Selective Anodic Oxidation of Solketal as Acetal-Protected Glycerol over Nickel Boride in Alkaline Media to Glyceric Acid**
Cychy, S. and Lechler, S. and Muhler, M.
CHEMELECTROCHEM. Volume: 9 (2022) - 2022 • 7279
A hybrid exploration approach for the prediction of geological changes ahead of mechanized tunnel excavation
Riedel, C. and Mahmoudi, E. and Trapp, M. and Lamert, A. and Hölter, R. and Zhao, C. and Musayev, K. and Baitsch, M. and König, M. and Hackl, K. and Nestorović, T. and Friederich, W.
JOURNAL OF APPLIED GEOPHYSICS. Volume: 203 (2022) - 2022 • 7278
Charge and spin supercurrents in magnetic Josephson junctions with spin filters and domain walls
Dahir, S.M. and Volkov, A.F. and Eremin, I.M.
PHYSICAL REVIEW B. Volume: 105 (2022) - 2022 • 7277
Mixing and segregation of spheres of three different sizes on a batch stoker grate: Experiments and discrete element simulation
Hilse, N. and Kriegeskorte, M. and Illana, E. and Wirtz, S. and Scherer, V.
POWDER TECHNOLOGY. Volume: 400 (2022) - 2022 • 7276
A deterministic source of single photons
Lodahl, P. and Ludwig, Ar. and Warburton, R.J.
PHYSICS TODAY. Volume: 75 (2022) - 2022 • 7275
Simulation of Arterial Walls: Growth, Fiber Reorientation, and Active Response
Uhlmann, K. and Zahn, A. and Balzani, D.
STUDIES IN MECHANOBIOLOGY, TISSUE ENGINEERING AND BIOMATERIALS. Volume: 24 (2022) - 2022 • 7274
A Pure and Indistinguishable Single-Photon Source at Telecommunication Wavelength
Da Lio, B. and Faurby, C. and Zhou, X. and Chan, M.L. and Uppu, R. and Thyrrestrup, H. and Scholz, S. and Wieck, A.D. and Ludwig, Ar. and Lodahl, P. and Midolo, L.
ADVANCED QUANTUM TECHNOLOGIES. Volume: 5 (2022) - 2022 • 7273
Role of surface structures on long term stability of adhesive joints between Ti–15V–3Cr–3Sn–3Al and polyether-ether-ketone
Löbbecke, M. and Bayerbasi, T.J. and Bartsch, M. and Haubrich, J.
INTERNATIONAL JOURNAL OF ADHESION AND ADHESIVES. Volume: (2022) - 2022 • 7272
Robust and Efficient Finite Element Discretizations for Higher-Order Gradient Formulations
Riesselmann, J. and Ketteler, J.W. and Schedensack, M. and Balzani, D.
LECTURE NOTES IN APPLIED AND COMPUTATIONAL MECHANICS. Volume: 98 (2022) - 2022 • 7271
Turning of high strength, austenitic stainless steels
Rinschede, T. and Felinks, N. and Biermann, D. and Kimm, J. and Weber, S. and Niederhofer, P. and Herrera, C. and Kalveram, M.
TRIBOLOGIE UND SCHMIERUNGSTECHNIK. Volume: 69 (2022) - 2022 • 7270
The origin of jerky dislocation motion in high-entropy alloys
Utt, D. and Lee, S. and Xing, Y. and Jeong, H. and Stukowski, A. and Oh, S.H. and Dehm, G. and Albe, K.
NATURE COMMUNICATIONS. Volume: 13 (2022) - 2022 • 7269
Mechanical and hydrodynamic characteristics of emerged porous Gyroid breakwaters based on triply periodic minimal surfaces
Dang, B.-L. and Nguyen-Van, V. and Tran, P. and Wahab, M.A. and Lee, J. and Hackl, K. and Nguyen-Xuan, H.
OCEAN ENGINEERING. Volume: 254 (2022) - 2022 • 7268
Engineering mesoporous silica nanoparticles for drug delivery: where are we after two decades?
Vallet-Regí, M. and Schüth, F. and Lozano, D. and Colilla, M. and Manzano, M.
CHEMICAL SOCIETY REVIEWS. Volume: 51 (2022) - 2022 • 7267
Pivotal tests for relevant differences in the second order dynamics of functional time series
VAN DELFT, A. and Dette, H.
BERNOULLI. Volume: 28 (2022) - 2022 • 7266
Hybrid additive manufacturing of metal laminated forming tools
Dardaei Joghan, H. and Hahn, M. and Sehrt, J.T. and Tekkaya, A.E., (1)
CIRP ANNALS. Volume: 71 (2022) - 2022 • 7265
THz metrology with monolithic tunable two-color diode lasers
Hofmann, M.R. and Surkamp, N. and Gerling, A. and O’Gorman, J. and Honsberg, M. and Schmidtmann, S. and Nandi, U. and Preu, S. and Sacher, J. and Brenner, C.
PROCEEDINGS OF SPIE - THE INTERNATIONAL SOCIETY FOR OPTICAL ENGINEERING. Volume: 12134 (2022) - 2022 • 7264
A Perspective on Thermally Sprayed Thermal Barrier Coatings: Current Status and Trends
Vaßen, R. and Bakan, E. and Mack, D.E. and Guillon, O.
JOURNAL OF THERMAL SPRAY TECHNOLOGY. Volume: 31 (2022) - 2022 • 7263
Combinatorial sputter deposition of CrMnFeCoNi high entropy alloy thin films on agitated particles
Lourens, F. and Ludwig, Al.
SURFACE AND COATINGS TECHNOLOGY. Volume: 449 (2022) - 2022 • 7262
Olfactory Stem Cells for the Treatment of Spinal Cord Injury—A New Pathway to the Cure?
Rövekamp, M. and von Glinski, A. and Volkenstein, S. and Dazert, S. and Sengstock, C. and Schildhauer, T.A. and Breisch, M.
WORLD NEUROSURGERY. Volume: (2022) - 2022 • 7261
Coupled Cluster Molecular Dynamics of Condensed Phase Systems Enabled by Machine Learning Potentials: Liquid Water Benchmark
Daru, J. and Forbert, H. and Behler, J. and Marx, D.
PHYSICAL REVIEW LETTERS. Volume: 129 (2022) - 2022 • 7260
Elastic energy of multi-component solid solutions and strain origins of phase stability in high-entropy alloys
Darvishi Kamachali, R. and Wang, L.
SCRIPTA MATERIALIA. Volume: 206 (2022) - 2022 • 7259
Chapter 11: Pathways in Classification Space: Machine Learning as a Route to Predicting Kinetics of Structural Transitions in Atomic Crystals
Rogal, J. and Tuckerman, M.E.
RSC THEORETICAL AND COMPUTATIONAL CHEMISTRY SERIES. Volume: 2022-January (2022) - 2022 • 7258
Recent developments in the functional renormalization group approach to correlated electron systems
Honerkamp, C. and Kennes, D.M. and Meden, V. and Scherer, M.M. and Thomale, R.
EUROPEAN PHYSICAL JOURNAL B. Volume: 95 (2022) - 2022 • 7257
Elevated-temperature cyclic deformation mechanisms of CoCrNi in comparison to CoCrFeMnNi
Lu, K. and Knöpfle, F. and Chauhan, A. and Litvinov, D. and Schneider, M. and Laplanche, G. and Aktaa, J.
SCRIPTA MATERIALIA. Volume: 220 (2022) - 2022 • 7256
Experimental investigations of plasma dynamics in the hysteresis regime of reactive RF sputter processes
Roggendorf, J. and Berger, B. and Eremin, D. and Oberberg, M. and Engel, D. and Wölfel, C. and Zhang, Q.-Z. and Awakowicz, P. and Lunze, J. and Schulze, J.
PLASMA SOURCES SCIENCE AND TECHNOLOGY. Volume: 31 (2022) - 2022 • 7255
Charging of Dielectric Surfaces in Contact with Aqueous Electrolytesthe Influence of CO2
Vogel, P. and Möller, N. and Qaisrani, M.N. and Bista, P. and Weber, S.A.L. and Butt, H.-J. and Liebchen, B. and Sulpizi, M. and Palberg, T.
JOURNAL OF THE AMERICAN CHEMICAL SOCIETY. Volume: 144 (2022) - 2022 • 7254
Recent Advances in Understanding Diffusion in Multiprincipal Element Systems
Dash, A. and Paul, A. and Sen, S. and Divinski, S. and Kundin, J. and Steinbach, I. and Grabowski, B. and Zhang, X.
ANNUAL REVIEW OF MATERIALS RESEARCH. Volume: 52 (2022) - 2022 • 7253
Hidden parameters for electrochemical carbon dioxide reduction in zero-gap electrolyzers
Hoof, L. and Thissen, N. and Pellumbi, K. and junge Puring, K. and Siegmund, D. and Mechler, A.K. and Apfel, U.-P.
CELL REPORTS PHYSICAL SCIENCE. Volume: 3 (2022) - 2022 • 7252
Influence of surface activation on the microporosity of PE-CVD and PE-ALD SiOx thin films on PDMS
Hoppe, C. and Mitschker, F. and Mai, L. and Liedke, M.O. and de los Arcos, T. and Awakowicz, P. and Devi, A. and Attallah, A.G. and Butterling, M. and Wagner, A. and Grundmeier, G.
PLASMA PROCESSES AND POLYMERS. Volume: (2022) - 2022 • 7251
Surface and Bulk Chemistry of Mechanochemically Synthesized Tohdite Nanoparticles
De Bellis, J. and Ochoa-Hernández, C. and Farès, C. and Petersen, H. and Ternieden, J. and Weidenthaler, C. and Amrute, A.P. and Schüth, F.
JOURNAL OF THE AMERICAN CHEMICAL SOCIETY. Volume: 144 (2022) - 2022 • 7250
Direct Dry Synthesis of Supported Bimetallic Catalysts: A Study on Comminution and Alloying of Metal Nanoparticles
De Bellis, J. and Petersen, H. and Ternieden, J. and Pfänder, N. and Weidenthaler, C. and Schüth, F.
ANGEWANDTE CHEMIE - INTERNATIONAL EDITION. Volume: (2022) - 2022 • 7249
Design and validation of a low-cost open-source impedance based quartz crystal microbalance for electrochemical research
Horst, R.J. and Katzourakis, A. and Mei, B.T. and de Beer, S.
HARDWAREX. Volume: 12 (2022) - 2022 • 7248
Obtaining different orientation relationships for Cu films grown on (0001) α-Al2O3substrates by magnetron sputtering
Dehm, G. and Edongué, H. and Wagner, T. and Oh, S.H. and Arzt, E.
INTERNATIONAL JOURNAL OF MATERIALS RESEARCH. Volume: 96 (2022) - 2022 • 7247
Size scaling in bi-crystalline Cu micropillars containing a coherent twin boundary
Hosseinabadi, R. and Riesch-Oppermann, H. and Best, J.P. and Dehm, G. and Kirchlechner, C.
ACTA MATERIALIA. Volume: 230 (2022) - 2022 • 7246
Implication of grain-boundary structure and chemistry on plasticity and failure
Dehm, G. and Cairney, J.
MRS BULLETIN. Volume: 47 (2022) - 2022 • 7245
Hierarchical nature of hydrogen-based direct reduction of iron oxides
Ma, Y. and Souza Filho, I.R. and Bai, Y. and Schenk, J. and Patisson, F. and Beck, A. and van Bokhoven, J.A. and Willinger, M.G. and Li, K. and Xie, D. and Ponge, D. and Zaefferer, S. and Gault, B. and Mianroodi, J.R. and Raabe, D.
SCRIPTA MATERIALIA. Volume: (2022) - 2022 • 7244
Additive Manufacturing of Columnar Thermal Barrier Coatings by Laser Cladding of Ceramic Feedstock
Vorkötter, C. and Mack, D.E. and Vaßen, R. and Guillon, O.
ADVANCED MATERIALS TECHNOLOGIES. Volume: 7 (2022) - 2022 • 7243
Non-Classical Conversion of Methanol to Formaldehyde
Deitermann, M. and Huang, Z. and Lechler, S. and Merko, M. and Muhler, M.
CHEMIE-INGENIEUR-TECHNIK. Volume: 94 (2022) - 2022 • 7242
Laser performance of LD side-pumped Er:YSGG crystal rods with different diameters
Hu, L. and Sun, D. and Hou, Y. and Gurevich, E.L. and Ostendorf, A. and Guo, Q.
PROCEEDINGS OF SPIE - THE INTERNATIONAL SOCIETY FOR OPTICAL ENGINEERING. Volume: 12310 (2022) - 2022 • 7241
Effect of Low-CTE Oxide-Dispersion-Strengthened Bond Coats on Columnar-Structured YSZ Coatings
Vorkötter, C. and Mack, D.E. and Zhou, D. and Guillon, O. and Vaßen, R.
COATINGS. Volume: 12 (2022) - 2022 • 7240
Highly dispersed Pd clusters/nanoparticles encapsulated in MOFs via in situ auto-reduction method for aqueous phenol hydrogenation
Huang, X. and Li, X. and Xia, W. and Hu, B. and Muhler, M. and Peng, B.
JOURNAL OF MATERIALS SCIENCE AND TECHNOLOGY. Volume: 109 (2022) - 2022 • 7239
Hydrogen-based direct reduction of iron oxide at 700°C: Heterogeneity at pellet and microstructure scales
Ma, Y. and Souza Filho, I.R. and Zhang, X. and Nandy, S. and Barriobero-Vila, P. and Requena, G. and Vogel, D. and Rohwerder, M. and Ponge, D. and Springer, H. and Raabe, D.
INTERNATIONAL JOURNAL OF MINERALS, METALLURGY AND MATERIALS. Volume: 29 (2022) - 2022 • 7238
Surface modification of silicon by femtosecond laser ablation in liquid
Maack, P. and Kanitz, A. and Hoppius, J. and Köhler, J. and Esen, C. and Ostendorf, A.
PROCEEDINGS OF SPIE - THE INTERNATIONAL SOCIETY FOR OPTICAL ENGINEERING. Volume: 11989 (2022) - 2022 • 7237
The effects of the driving frequencies on micro atmospheric pressure He/N2plasma jets driven by tailored voltage waveforms
Hübner, G. and Bischoff, L. and Korolov, I. and Donkó, Z. and Leimkühler, M. and Liu, Y. and Böke, M. and Schulz-Von Der Gathen, V. and Mussenbrock, T. and Schulze, J.
JOURNAL OF PHYSICS D: APPLIED PHYSICS. Volume: 55 (2022) - 2022 • 7236
Effects of Cr/Ni ratio on physical properties of Cr-Mn-Fe-Co-Ni high-entropy alloys
Wagner, C. and Ferrari, A. and Schreuer, J. and Couzinié, J.-P. and Ikeda, Y. and Körmann, F. and Eggeler, G. and George, E.P. and Laplanche, G.
ACTA MATERIALIA. Volume: 227 (2022) - 2022 • 7235
One-Dimensional Water Structures upon Cs Hydration on the Moiré Pattern of Graphitic ZnO
Hung, T.-C. and Morgenstern, K.
JOURNAL OF PHYSICAL CHEMISTRY C. Volume: 126 (2022) - 2022 • 7234
Combining 2D and 3D Characterization Techniques for Determining Effects of HIP Rejuvenation After Fatigue Testing of SX Microstructures
Dennstedt, A. and Lopez-Galilea, I. and Ruttert, B. and Theisen, W. and Bartsch, M.
METALLURGICAL AND MATERIALS TRANSACTIONS A: PHYSICAL METALLURGY AND MATERIALS SCIENCE. Volume: (2022) - 2022 • 7233
Influence of the Moiré Pattern of Ag(111)-Supported Graphitic ZnO on Water Distribution
Hung, T.-C. and Le, D. and Rahman, T. and Morgenstern, K.
JOURNAL OF PHYSICAL CHEMISTRY C. Volume: 126 (2022) - 2022 • 7232
Influence of different ester side groups in polymers on the vapor phase infiltration with trimethyl aluminum
Mai, L. and Maniar, D. and Zysk, F. and Schöbel, J. and Kühne, T.D. and Loos, K. and Devi, A.
DALTON TRANSACTIONS. Volume: 51 (2022) - 2022 • 7231
Comparative Study of Automotive MIMO Radar Measurements in W-Band and D-Band
Wagner, J. and Dahl, C. and Rolfes, I. and Barowski, J.
2022 19TH EUROPEAN RADAR CONFERENCE, EURAD 2022. Volume: (2022) - 2022 • 7230
Signatures of collective modes in fifth harmonic generation of BCS superconductor
Derendorf, P. and Müller, M.A. and Eremin, I.M.
FARADAY DISCUSSIONS. Volume: 237 (2022) - 2022 • 7229
Influence of the PTFE Membrane Thickness on the CO2 Electroreduction Performance of Sputtered Cu-PTFE Gas Diffusion Electrodes
Huq, F. and Sanjuán, I. and Baha, S. and Braun, M. and Kostka, A. and Chanda, V. and Junqueira, J.R.C. and Sikdar, N. and Ludwig, A. and Andronescu, C.
CHEMELECTROCHEM. Volume: 9 (2022) - 2022 • 7228
BioSAXS–an emerging method to accelerate, enrich and de-risk antimicrobial drug development
Rumancev, C. and Rosenhahn, A. and Hilpert, K.
FRONTIERS IN PHARMACOLOGY. Volume: 13 (2022) - 2022 • 7227
Resolving particle shape in DEM simulations from tabulated geometry information
Deshpande, R. and Mahiques, E. and Wirtz, S. and Scherer, V.
POWDER TECHNOLOGY. Volume: 407 (2022) - 2022 • 7226
Electrochemical dealloying in a magnetic field – Tapping the potential for catalyst and material design
Rurainsky, C. and Nettler, D.-R. and Pahl, T. and Just, A. and Cignoni, P. and Kanokkanchana, K. and Tschulik, K.
ELECTROCHIMICA ACTA. Volume: 426 (2022) - 2022 • 7225
SnO deposition via water based ALD employing tin(ii) formamidinate: precursor characterization and process development
Huster, N. and Ghiyasi, R. and Zanders, D. and Rogalla, D. and Karppinen, M. and Devi, A.
DALTON TRANSACTIONS. Volume: 51 (2022) - 2022 • 7224
Thermoregeneration of Fouling-Inhibiting Plastrons on Conductive Laser-Induced Graphene Coatings by Joule Heating
Manderfeld, E. and Nunes Kleinberg, M. and Thamaraiselvan, C. and Arnusch, C.J. and Rosenhahn, A.
ADVANCED MATERIALS INTERFACES. Volume: (2022) - 2022 • 7223
Interactions of water and short-chain alcohols with CoFe2O4(001) surfaces at low coverages
Rushiti, A. and Falk, T. and Muhler, M. and Hättig, C.
PHYSICAL CHEMISTRY CHEMICAL PHYSICS. Volume: 24 (2022) - 2022 • 7222
Asymptotic equivalence for nonparametric regression with dependent errors: Gauss–Markov processes
Dette, H. and Kroll, M.
ANNALS OF THE INSTITUTE OF STATISTICAL MATHEMATICS. Volume: 74 (2022) - 2022 • 7221
Bacterial surface attachment and fouling assay on polymer and carbon surfaces using Rheinheimera sp. identified using bacteria community analysis of brackish water
Manderfeld, E. and Thamaraiselvan, C. and Nunes Kleinberg, M. and Jusufagic, L. and Arnusch, C.J. and Rosenhahn, A.
BIOFOULING. Volume: 38 (2022) - 2022 • 7220
Fluid-structure interaction simulation of tissue degradation and its effects on intra-aneurysm hemodynamics
Wang, H. and Uhlmann, K. and Vedula, V. and Balzani, D. and Varnik, F.
BIOMECHANICS AND MODELING IN MECHANOBIOLOGY. Volume: (2022) - 2022 • 7219
Quantifying deviations from separability in space-time functional processes
Dette, H. and Dierickx, G. and Kutta, T.
BERNOULLI. Volume: 28 (2022) - 2022 • 7218
Exploring stability of a nanoscale complex solid solution thin film by in situ heating transmission electron microscopy
Manjón, A.G. and Zhang, S. and Völker, B. and Meischein, M. and Ludwig, Al. and Scheu, C.
MRS BULLETIN. Volume: (2022) - 2022 • 7217
A restricted additive Vanka smoother for geometric multigrid
Saberi, S. and Meschke, G. and Vogel, A.
JOURNAL OF COMPUTATIONAL PHYSICS. Volume: 459 (2022) - 2022 • 7216
Effect of laser shock peening without protective coating on the surface mechanical properties of NiTi alloy
Wang, H. and Keller, S. and Chang, Y. and Kashaev, N. and Yan, K. and Gurevich, E.L. and Ostendorf, A.
JOURNAL OF ALLOYS AND COMPOUNDS. Volume: 896 (2022) - 2022 • 7215
DESIGN ADMISSIBILITY AND DE LA GARZA PHENOMENON IN MULTIFACTOR EXPERIMENTS
Dette, H. and Liu, X. and Yue, R.-X.
ANNALS OF STATISTICS. Volume: 50 (2022) - 2022 • 7214
The dual role of martensitic transformation in fatigue crack growth
Wang, X. and Liu, C. and Sun, B. and Ponge, D. and Jiang, C. and Raabe, D.
PROCEEDINGS OF THE NATIONAL ACADEMY OF SCIENCES OF THE UNITED STATES OF AMERICA. Volume: 119 (2022) - 2022 • 7213
The effect of intraday periodicity on realized volatility measures
Dette, H. and Golosnoy, V. and Kellermann, J.
METRIKA. Volume: (2022) - 2022 • 7212
Tuning Electronic Structures of Covalent Co Porphyrin Polymers for Electrocatalytic CO2Reduction in Aqueous Solutions
Wang, Y. and Zhang, X.-P. and Lei, H. and Guo, K. and Xu, G. and Xie, L. and Li, X. and Zhang, W. and Apfel, U.-P. and Cao, R.
CCS CHEMISTRY. Volume: 41 (2022) - 2022 • 7211
Detecting relevant changes in the spatiotemporal mean function
Dette, H. and Quanz, P.
JOURNAL OF TIME SERIES ANALYSIS. Volume: (2022) - 2022 • 7210
Machine-learning based analysis of time sequences for multiplexed microresonator sensor
Saetchnikov, A. and Tcherniavskaia, E. and Saetchnikov, V. and Ostendorf, A.
PROCEEDINGS OF SPIE - THE INTERNATIONAL SOCIETY FOR OPTICAL ENGINEERING. Volume: 12139 (2022) - 2022 • 7209
Micro- and nano-devices for electrochemical sensing
Mariani, F. and Gualandi, I. and Schuhmann, W. and Scavetta, E.
MICROCHIMICA ACTA. Volume: 189 (2022) - 2022 • 7208
Microstructure, grain boundary evolution and anisotropic Fe segregation in (0001) textured Ti thin films
Devulapalli, V. and Hans, M. and Sukumar, P.T. and Schneider, J.M. and Dehm, G. and Liebscher, C.H.
ACTA MATERIALIA. Volume: 238 (2022) - 2022 • 7207
A guide to direct mechanocatalysis
Hwang, S. and Grätz, S. and Borchardt, L.
CHEMICAL COMMUNICATIONS. Volume: 58 (2022) - 2022 • 7206
Advanced oxidation processes for removal of organics from cooling tower blowdown: Efficiencies and evaluation of chlorinated species
Saha, P. and Wang, Y. and Moradi, M. and Brüninghoff, R. and Moussavi, G. and Mei, B. and Mul, G. and H. M. Rijnaarts, H. and Bruning, H.
SEPARATION AND PURIFICATION TECHNOLOGY. Volume: 278 (2022) - 2022 • 7205
Multiscale characterization of damage tolerance in barium titanate thin films
Mathews, N.G. and Saxena, A.K. and Venkataramani, N. and Dehm, G. and Jaya, B.N.
JOURNAL OF APPLIED PHYSICS. Volume: 132 (2022) - 2022 • 7204
Generation of a Single-Cycle Acoustic Pulse: A Scalable Solution for Transport in Single-Electron Circuits
Wang, J. and Ota, S. and Edlbauer, H. and Jadot, B. and Mortemousque, P.-A. and Richard, A. and Okazaki, Y. and Nakamura, S. and Ludwig, Ar. and Wieck, A.D. and Urdampilleta, M. and Meunier, T. and Kodera, T. and Kaneko, N.-H. and Takada, S. and Bäuerle, C.
PHYSICAL REVIEW X. Volume: 12 (2022) - 2022 • 7203
Template-Induced Precursor Formation in Heterogeneous Nucleation: Controlling Polymorph Selection and Nucleation Efficiency
Díaz Leines, G. and Rogal, J.
PHYSICAL REVIEW LETTERS. Volume: 128 (2022) - 2022 • 7202
Effect of laser shock peening with square laser spot on hardness and residual stress of Ti6Al4V alloy
Wang, H. and Kaufman, J. and Brajer, J. and Gurevich, E.L. and Ostendorf, A.
PROCEEDINGS OF SPIE - THE INTERNATIONAL SOCIETY FOR OPTICAL ENGINEERING. Volume: 11994 (2022) - 2022 • 7201
Elucidating dislocation core structures in titanium nitride through high-resolution imaging and atomistic simulations
Salamania, J. and Sangiovanni, D.G. and Kraych, A. and Calamba Kwick, K.M. and Schramm, I.C. and Johnson, L.J.S. and Boyd, R. and Bakhit, B. and Hsu, T.W. and Mrovec, M. and Rogström, L. and Tasnádi, F. and Abrikosov, I.A. and Odén, M.
MATERIALS AND DESIGN. Volume: 224 (2022) - 2022 • 7200
High stress twinning in a compositionally complex steel of very high stacking fault energy
Wang, Z. and Lu, W. and An, F. and Song, M. and Ponge, D. and Raabe, D. and Li, Z.
NATURE COMMUNICATIONS. Volume: 13 (2022) - 2022 • 7199
Operando Scanning Electrochemical Probe Microscopy during Electrocatalysis
Santana Santos, C. and Jaato, B.N. and Sanjuán, I. and Schuhmann, W. and Andronescu, C.
CHEMICAL REVIEWS. Volume: (2022) - 2022 • 7198
Design and Application of a High-Surface-Area Mesoporous δ-MnO2Electrocatalyst for Biomass Oxidative Valorization
Wang, C. and Bongard, H.-J. and Weidenthaler, C. and Wu, Y. and Schüth, F.
CHEMISTRY OF MATERIALS. Volume: 34 (2022) - 2022 • 7197
Pinning of domain walls by strontium layer in the BaTiO3 perovskite: An atomic-scale study
Dimou, A. and Hirel, P. and Grünebohm, A.
PHYSICAL REVIEW B. Volume: 106 (2022) - 2022 • 7196
Condition Monitoring of a Three-Cathode Cascaded Plasma Spray Torch Regarding Process Reliability
Mauer, G. and Kurze, F. and Rauwald, K.-H. and Vaßen, R.
MATERIALS. Volume: 15 (2022) - 2022 • 7195
Correlative Electrochemical Microscopy for the Elucidation of the Local Ionic and Electronic Properties of the Solid Electrolyte Interphase in Li-Ion Batteries
Santos, C.S. and Botz, A. and Bandarenka, A.S. and Ventosa, E. and Schuhmann, W.
ANGEWANDTE CHEMIE - INTERNATIONAL EDITION. Volume: 61 (2022) - 2022 • 7194
Plasma spraying porous thermal barrier coatings with high deposition efficiency: A solvable dilemma?
Mauer, G. and Vaßen, R.
SURFACE AND COATINGS TECHNOLOGY. Volume: 451 (2022) - 2022 • 7193
Machine learning for molecular simulations of crystal nucleation and growth
Sarupria, S. and Hall, S.W. and Rogal, J.
MRS BULLETIN. Volume: (2022) - 2022 • 7192
Gaining the Freedom of Scalable Gas Diffusion Electrodes for the CO2 Reduction Reaction
Wang, X. and Tomon, C. and Bobrowski, T. and Wilde, P. and Junqueira, J.R.C. and Quast, T. and He, W. and Sikdar, N. and Weidner, J. and Schuhmann, W.
CHEMELECTROCHEM. Volume: 9 (2022) - 2022 • 7191
Influence of Heat Treatment Parameters on the Carbide Morphology of PM High-Speed Steel HS 6-5-3-8
Disch, N. and Benito, S. and Röttger, A. and Weber, S.
STEEL RESEARCH INTERNATIONAL. Volume: (2022) - 2022 • 7190
Deep learning framework for uncovering compositional and environmental contributions to pitting resistance in passivating alloys
Sasidhar, K.N. and Siboni, N.H. and Mianroodi, J.R. and Rohwerder, M. and Neugebauer, J. and Raabe, D.
NPJ MATERIALS DEGRADATION. Volume: 6 (2022) - 2022 • 7189
Damage localization and characterization using one-dimensional convolutional neural network and a sparse network of transducers
Sattarifar, A. and Nestorović, T.
ENGINEERING APPLICATIONS OF ARTIFICIAL INTELLIGENCE. Volume: 115 (2022) - 2022 • 7188
Emergence of Machine Learning Techniques in Ultrasonic Guided Wave-based Structural Health Monitoring: A Narrative Review
Sattarifar, A. and Nestorović, T.
INTERNATIONAL JOURNAL OF PROGNOSTICS AND HEALTH MANAGEMENT. Volume: 13 (2022) - 2022 • 7187
Erratum: Anisotropic thermodynamic and transport properties of single-crystalline CaKFe4 As4 (Physical Review B (2016) 94 (064501) DOI: 10.1103/PhysRevB.94.064501)
Meier, W.R. and Kong, T. and Kaluarachchi, U.S. and Taufour, V. and Jo, N.H. and Drachuck, G. and Böhmer, A.E. and Saunders, S.M. and Sapkota, A. and Kreyssig, A. and Tanatar, M.A. and Prozorov, R. and Goldman, A.I. and Balakirev, F.F. and Gurevich, A. and Bud'Ko, S.L. and Canfield, P.C.
PHYSICAL REVIEW B. Volume: 105 (2022) - 2022 • 7186
Unravelling Anion Solvation in Water-Alcohol Mixtures by Single Entity Electrochemistry
Saw, E.N. and Kanokkanchana, K. and Amin, H.M.A. and Tschulik, K.
CHEMELECTROCHEM. Volume: (2022) - 2022 • 7185
Elemental (im-)miscibility determines phase formation of multinary nanoparticles co-sputtered in ionic liquids
Meischein, M. and Garzón-Manjón, A. and Hammerschmidt, T. and Xiao, B. and Zhang, S. and Abdellaoui, L. and Scheu, C. and Ludwig, A.
NANOSCALE ADVANCES. Volume: 4 (2022) - 2022 • 7184
Nanoscale copper and silver thin film systems display differences in antiviral and antibacterial properties
Meister, T.L. and Fortmann, J. and Breisch, M. and Sengstock, C. and Steinmann, E. and Köller, M. and Pfaender, S. and Ludwig, Al.
SCIENTIFIC REPORTS. Volume: 12 (2022) - 2022 • 7183
Revealing the influence of Mo addition on interphase precipitation in Ti-bearing low carbon steels
Dong, H. and Chen, H. and Riyahi khorasgani, A. and Zhang, B. and Zhang, Y. and Wang, Z. and Zhou, X. and Wang, W. and Wang, H. and Li, T. and Yang, Z. and van der Zwaag, S.
ACTA MATERIALIA. Volume: 223 (2022) - 2022 • 7182
Hydrogen-associated decohesion and localized plasticity in a high-Mn and high-Al two-phase lightweight steel
Dong, X. and Wang, D. and Thoudden-Sukumar, P. and Tehranchi, A. and Ponge, D. and Sun, B. and Raabe, D.
ACTA MATERIALIA. Volume: 239 (2022) - 2022 • 7181
Electrochemical CO2 reduction toward multicarbon alcohols - The microscopic world of catalysts & process conditions
Jaster, T. and Gawel, A. and Siegmund, D. and Holzmann, J. and Lohmann, H. and Klemm, E. and Apfel, U.-P.
ISCIENCE. Volume: 25 (2022) - 2022 • 7180
Ab initio calculation of the magnetic Gibbs free energy of materials using magnetically constrained supercells
Mendive-Tapia, E. and Neugebauer, J. and Hickel, T.
PHYSICAL REVIEW B. Volume: 105 (2022) - 2022 • 7179
Electrochemistry under confinement
Jaugstetter, M. and Blanc, N. and Kratz, M. and Tschulik, K.
CHEMICAL SOCIETY REVIEWS. Volume: 51 (2022) - 2022 • 7178
Si-addition contributes to overcoming the strength-ductility trade-off in high-entropy alloys
Wei, D. and Gong, W. and Tsuru, T. and Lobzenko, I. and Li, X. and Harjo, S. and Kawasaki, T. and Do, H.-S. and Bae, J.W. and Wagner, C. and Laplanche, G. and Koizumi, Y. and Adachi, H. and Aoyagi, K. and Chiba, A. and Lee, B.-J. and Kim, H.S. and Kato, H.
INTERNATIONAL JOURNAL OF PLASTICITY. Volume: 159 (2022) - 2022 • 7177
A small-scale creep test for calibrating an efficient lifetime model for high pressure turbine blades [Ein miniaturisierter Kriechversuch zur Kalibrierung eines effizienten Lebensdauermodells für Hochdruckturbinenschaufeln]
Dresbach, C. and Wischek, J. and Bartsch, M. and Prien, T.
MATERIALWISSENSCHAFT UND WERKSTOFFTECHNIK. Volume: 53 (2022) - 2022 • 7176
Graphene-Based Metal-Organic Framework Hybrids for Applications in Catalysis, Environmental, and Energy Technologies
Jayaramulu, K. and Mukherjee, S. and Morales, D.M. and Dubal, D.P. and Nanjundan, A.K. and Schneemann, A. and Masa, J. and Kment, S. and Schuhmann, W. and Otyepka, M. and Zbořil, R. and Fischer, R.A.
CHEMICAL REVIEWS. Volume: (2022) - 2022 • 7175
Silicon based Metamaterials for Dielectric Waveguides in the THz Range
Schenkel, F. and Barengolts, I. and Schmitt, L. and Rolfes, I. and Hoffmann, M. and Barowski, J.
MEDITERRANEAN MICROWAVE SYMPOSIUM. Volume: 2022-May (2022) - 2022 • 7174
MEAM interatomic potentials of Ni, Re, and Ni-Re alloys for atomistic fracture simulations
Alam, M. and Lymperakis, L. and Groh, S. and Neugebauer, J.
MODELLING AND SIMULATION IN MATERIALS SCIENCE AND ENGINEERING. Volume: 30 (2022) - 2022 • 7173
Dynamical photon–photon interaction mediated by a quantum emitter
Jeannic, H.L. and Tiranov, A. and Carolan, J. and Ramos, T. and Wang, Y. and Appel, M.H. and Scholz, S. and Wieck, A.D. and Ludwig, Ar. and Rotenberg, N. and Midolo, L. and García-Ripoll, J.J. and Sørensen, A.S. and Lodahl, P.
NATURE PHYSICS. Volume: 18 (2022) - 2022 • 7172
High-throughput exploration of activity and stability for identifying photoelectrochemical water splitting materials
Jenewein, K.J. and Thienhaus, S. and Kormányos, A. and Ludwig, Al. and Cherevko, S.
CHEMICAL SCIENCE. Volume: 13 (2022) - 2022 • 7171
Chiral superconductivity with enhanced quantized Hall responses in moiré transition metal dichalcogenides
Scherer, M.M. and Kennes, D.M. and Classen, L.
NPJ QUANTUM MATERIALS. Volume: 7 (2022) - 2022 • 7170
GOAT: a multipurpose optical simulation tool
Weigel, T. and Schweiger, G. and Ostendorf, A.
JOURNAL OF THE OPTICAL SOCIETY OF AMERICA B: OPTICAL PHYSICS. Volume: 39 (2022) - 2022 • 7169
Approximating the impact of nuclear quantum effects on thermodynamic properties of crystalline solids by temperature remapping
Dsouza, R. and Huber, L. and Grabowski, B. and Neugebauer, J.
PHYSICAL REVIEW B. Volume: 105 (2022) - 2022 • 7168
Non-oxidative Dehydrogenation of Methanol to Formaldehyde over Bulk β-Ga2O3
Merko, M. and Busser, G.W. and Muhler, M.
CHEMCATCHEM. Volume: 14 (2022) - 2022 • 7167
State of the art energy conversion at the nanointerface: general discussion
Albrecht, T. and Bohn, P. and Buckingham, M.A. and Cao, X.E. and Chen, D. and Chen, Q. and Corva, M. and Edwards, M.A. and Kamali, A.R. and Kanoufi, F. and Krause, S. and Linfield, S. and Liu, X. and Ma, H. and Mao, B.-W. and Pandey, P. and Tschulik, K. and Vakamulla Raghu, S.N. and Walcarius, A. and Xiao, L. and Ying, Y.-L.
FARADAY DISCUSSIONS. Volume: 233 (2022) - 2022 • 7166
Analysing the entropy of lithium-ion cells to trace anodic half-cell ageing
Mertin, G.K. and Wycisk, D. and Stadler, J. and von Kessel, O. and Richter, E. and Oldenburger, M. and Wieck, A.D. and Birke, K.P.
JOURNAL OF ENERGY STORAGE. Volume: 50 (2022) - 2022 • 7165
Electrochemical data mining: from information to knowledge: general discussion
Albrecht, T. and Cao, X.E. and Chen, D. and Corva, M. and Edwards, M.A. and Ewing, A. and Fornasaro, S. and Gooding, J.J. and Gundry, L. and Hirano-Iwata, A. and Jeffcoat, G. and Kamali, A.R. and Kanoufi, F. and Lemay, S.G. and Limani, N. and Linfield, S. and Liu, X. and Lu, S.-M. and Meloni, G.N. and Tian, Z. and Tschulik, K. and Vakamulla Raghu, S.N. and Wei, H. and Ying, Y.-L.
FARADAY DISCUSSIONS. Volume: 233 (2022) - 2022 • 7164
Dynamic measurement of the entropy coefficient for battery cells
Mertin, G.K. and Wycisk, D. and Oldenburger, M. and Stoye, G. and Fill, A. and Birke, K.P. and Wieck, A.D.
JOURNAL OF ENERGY STORAGE. Volume: 51 (2022) - 2022 • 7163
Multivariate Mean Comparison Under Differential Privacy
Dunsche, M. and Kutta, T. and Dette, H.
LECTURE NOTES IN COMPUTER SCIENCE (INCLUDING SUBSERIES LECTURE NOTES IN ARTIFICIAL INTELLIGENCE AND LECTURE NOTES IN BIOINFORMATICS). Volume: 13463 LNCS (2022) - 2022 • 7162
Modeling and simulation of microstructure in metallic systems based on multi-physics approaches
Mianroodi, J.R. and Shanthraj, P. and Liu, C. and Vakili, S. and Roongta, S. and Siboni, N.H. and Perchikov, N. and Bai, Y. and Svendsen, B. and Roters, F. and Raabe, D. and Diehl, M.
NPJ COMPUTATIONAL MATERIALS. Volume: 8 (2022) - 2022 • 7161
Data-oriented description of texture-dependent anisotropic material behavior
Schmidt, J. and Biswas, A. and Vajragupta, N. and Hartmaier, A.
MODELLING AND SIMULATION IN MATERIALS SCIENCE AND ENGINEERING. Volume: 30 (2022) - 2022 • 7160
Lossless multi-scale constitutive elastic relations with artificial intelligence
Mianroodi, J.R. and Rezaei, S. and Siboni, N.H. and Xu, B.-X. and Raabe, D.
NPJ COMPUTATIONAL MATERIALS. Volume: 8 (2022) - 2022 • 7159
Cost Analysis of Automated Additive Printer Farms
Schmidt, C. and Finsterwalder, F. and Griesbaum, R. and Sehrt, J.T.
IOP CONFERENCE SERIES: EARTH AND ENVIRONMENTAL SCIENCE. Volume: 1048 (2022) - 2022 • 7158
Modified Z-phase formation in a 12% Cr tempered martensite ferritic steel during long-term creep
Westraadt, J.E. and Goosen, W.E. and Kostka, A. and Wang, H. and Eggeler, G.
MATERIALS SCIENCE AND ENGINEERING A. Volume: 855 (2022) - 2022 • 7157
Indentation behavior of creep-feed grinding induced gradient microstructures in single crystal nickel-based superalloy
Miao, Q. and Ding, W. and Kuang, W. and Fu, Y. and Yin, Z. and Dai, C. and Cao, L. and Wang, H.
MATERIALS LETTERS. Volume: 306 (2022) - 2022 • 7156
Atomic cluster expansion: Completeness, efficiency and stability
Dusson, G. and Bachmayr, M. and Csányi, G. and Drautz, R. and Etter, S. and van der Oord, C. and Ortner, C.
JOURNAL OF COMPUTATIONAL PHYSICS. Volume: 454 (2022) - 2022 • 7155
A passive acceleration sensor with mechanical 6 bit memory and mechanical analog-to-digital converter
Schmitt, P. and Hoffmann, M.
MICRO AND NANO ENGINEERING. Volume: 15 (2022) - 2022 • 7154
Exploring the Impact of the Linker Length on Heat Transport in Metal-Organic Frameworks
Wieser, S. and Kamencek, T. and Schmid, R. and Bedoya-Martínez, N. and Zojer, E.
NANOMATERIALS. Volume: 12 (2022) - 2022 • 7153
A bioinspired redox-modulating copper(ii)-macrocyclic complex bearing non-steroidal anti-inflammatory drugs with anti-cancer stem cell activity
Johnson, A. and Iffland-Mühlhaus, L. and Northcote-Smith, J. and Singh, K. and Ortu, F. and Apfel, U.-P. and Suntharalingam, K.
DALTON TRANSACTIONS. Volume: 51 (2022) - 2022 • 7152
THz Phase Shifter based on MEMS-Actuated Slot Waveguides
Schmitt, L. and Barowski, J. and Hoffmann, M.
2022 5TH INTERNATIONAL WORKSHOP ON MOBILE TERAHERTZ SYSTEMS, IWMTS 2022. Volume: (2022) - 2022 • 7151
Weak itinerant magnetic phases of La2Ni7
Wilde, J.M. and Sapkota, A. and Tian, W. and Bud'Ko, S.L. and Ribeiro, R.A. and Kreyssig, A. and Canfield, P.C.
PHYSICAL REVIEW B. Volume: 106 (2022) - 2022 • 7150
Metallographic preparation methods for the Mg based system Mg-Al-Ca and its Laves phases
Andre, D. and Freund, M. and Rehman, U. and Delis, W. and Felten, M. and Nowak, J. and Tian, C. and Zubair, M. and Tanure, L. and Abdellaoui, L. and Springer, H. and Best, J.P. and Zander, D. and Dehm, G. and Sandlöbes-Haut, S. and Korte-Kerzel, S.
MATERIALS CHARACTERIZATION. Volume: 192 (2022) - 2022 • 7149
Catalytic effects for cellulose-based model fuels under low and high heating rate in air and oxy-fuel atmosphere
Eckhard, T. and Pflieger, C. and Schmidt, S. and Böttger, J. and Senneca, O. and Schiemann, M. and Scherer, V. and Muhler, M. and Cerciello, F.
FUEL. Volume: 324 (2022) - 2022 • 7148
Tracing the Force-Displacement Characteristics of Non-Linear Microsystems by In-Situ Characterization
Schmitt, P. and Hoffmann, M.
IEEE SYMPOSIUM ON MASS STORAGE SYSTEMS AND TECHNOLOGIES. Volume: 2022-January (2022) - 2022 • 7147
Canted antiferromagnetic phases in the candidate layered Weyl material EuMnSb2
Wilde, J.M. and Riberolles, S.X.M. and Das, A. and Liu, Y. and Heitmann, T.W. and Wang, X. and Straszheim, W.E. and Bud'Ko, S.L. and Canfield, P.C. and Kreyssig, A. and McQueeney, R.J. and Ryan, D.H. and Ueland, B.G.
PHYSICAL REVIEW B. Volume: 106 (2022) - 2022 • 7146
A Passive Micromechanical Counting Mechanism
Schmitt, P. and Hoffmann, M.
PROCEEDINGS OF IEEE SENSORS. Volume: 2022-October (2022) - 2022 • 7145
A chiral one-dimensional atom using a quantum dot in an open microcavity
Antoniadis, N.O. and Tomm, N. and Jakubczyk, T. and Schott, R. and Valentin, S.R. and Wieck, A.D. and Ludwig, A. and Warburton, R.J. and Javadi, A.
NPJ QUANTUM INFORMATION. Volume: 8 (2022) - 2022 • 7144
A cracking oxygen story: A new view of stress corrosion cracking in titanium alloys
Joseph, S. and Kontis, P. and Chang, Y. and Shi, Y. and Raabe, D. and Gault, B. and Dye, D.
ACTA MATERIALIA. Volume: 227 (2022) - 2022 • 7143
Strategies for damage tolerance enhancement in metal/ceramic thin films: Lessons learned from Ti/TiN
Mishra, A.K. and Gopalan, H. and Hans, M. and Kirchlechner, C. and Schneider, J.M. and Dehm, G. and Jaya, B.N.
ACTA MATERIALIA. Volume: 228 (2022) - 2022 • 7142
Simulation of crack propagation based on eigenerosion in brittle and ductile materials subject to finite strains
Wingender, D. and Balzani, D.
ARCHIVE OF APPLIED MECHANICS. Volume: (2022) - 2022 • 7141
Transient uptake measurements with a physisorption instrument: Trends in gas-phase diffusivities within mesoporous materials
Joshi, H. and Hopf, A. and Losch, P. and Schmidt, W. and Schüth, F.
MICROPOROUS AND MESOPOROUS MATERIALS. Volume: 330 (2022) - 2022 • 7140
METHOD FOR THE ANALYSIS OF EPISTEMIC AND ALEATORY UNCERTAINTIES FOR A RELIABLE EVALUATION OF FAILURE OF ENGINEERING STRUCTURES
Miska, N. and Balzani, D.
INTERNATIONAL JOURNAL FOR UNCERTAINTY QUANTIFICATION. Volume: 12 (2022) - 2022 • 7139
Simulation of crack propagation through voxel-based, heterogeneous structures based on eigenerosion and finite cells
Wingender, D. and Balzani, D.
COMPUTATIONAL MECHANICS. Volume: 70 (2022) - 2022 • 7138
Entangling a Hole Spin with a Time-Bin Photon: A Waveguide Approach for Quantum Dot Sources of Multiphoton Entanglement
Appel, M.H. and Tiranov, A. and Pabst, S. and Chan, M.L. and Starup, C. and Wang, Y. and Midolo, L. and Tiurev, K. and Scholz, S. and Wieck, A.D. and Ludwig, Ar. and Sørensen, A.S. and Lodahl, P.
PHYSICAL REVIEW LETTERS. Volume: 128 (2022) - 2022 • 7137
Hydrogen-assisted decohesion associated with nanosized grain boundary κ-carbides in a high-Mn lightweight steel
Elkot, M.N. and Sun, B. and Zhou, X. and Ponge, D. and Raabe, D.
ACTA MATERIALIA. Volume: 241 (2022) - 2022 • 7136
Propagation of nanosecond plasmas in liquids - Streamer velocities and streamer lengths
Jungling, E. and Grosse, K. and Von Keudell, A.
JOURNAL OF VACUUM SCIENCE AND TECHNOLOGY A: VACUUM, SURFACES AND FILMS. Volume: 40 (2022) - 2022 • 7135
Efficient and robust numerical treatment of a gradient-enhanced damage model at large deformations
Junker, P. and Riesselmann, J. and Balzani, D.
INTERNATIONAL JOURNAL FOR NUMERICAL METHODS IN ENGINEERING. Volume: 123 (2022) - 2022 • 7134
From Inert to Catalytically Active Milling Media: Galvanostatic Coating for Direct Mechanocatalysis
Wohlgemuth, M. and Mayer, M. and Rappen, M. and Schmidt, F. and Saure, R. and Grätz, S. and Borchardt, L.
ANGEWANDTE CHEMIE - INTERNATIONAL EDITION. Volume: (2022) - 2022 • 7133
High-Pressure Pulsing of Ammonia Results in Carbamate as Strongly Inhibiting Adsorbate of Methanol Synthesis over Cu/ZnO/Al2O3
Mockenhaupt, B. and Schwiderowski, P. and Jelic, J. and Studt, F. and Muhler, M. and Behrens, M.
JOURNAL OF PHYSICAL CHEMISTRY C. Volume: (2022) - 2022 • 7132
A Statistical FDFD Simulator for the Generation of Labeled Training Data Sets in the Context of Humanitarian Demining using GPR
Schorlemer, J. and Jebramcik, J. and Baer, C. and Rolfes, I. and Schulz, C.
2022 IEEE MTT-S INTERNATIONAL CONFERENCE ON NUMERICAL ELECTROMAGNETIC AND MULTIPHYSICS MODELING AND OPTIMIZATION, NEMO 2022. Volume: (2022) - 2022 • 7131
Efficient model-based bioequivalence testing
Möllenhoff, K. and Loingeville, F. and Bertrand, J. and Nguyen, T.T. and Sharan, S. and Zhao, L. and Fang, L. and Sun, G. and Grosser, S. and Mentré, F. and Dette, H.
BIOSTATISTICS (OXFORD, ENGLAND). Volume: 23 (2022) - 2022 • 7130
Testing for similarity of binary efficacy-toxicity responses
Möllenhoff, K. and Dette, H. and Bretz, F.
BIOSTATISTICS (OXFORD, ENGLAND). Volume: 23 (2022) - 2022 • 7129
Operando electrochemical SERS monitors nanoparticle reactions by capping agent fingerprints
Wonner, K. and Murke, S. and Alfarano, S.R. and Hosseini, P. and Havenith, M. and Tschulik, K.
NANO RESEARCH. Volume: (2022) - 2022 • 7128
Influence of Mo/Cr ratio on the lamellar microstructure and mechanical properties of as-cast Al0.75CoCrFeNi compositionally complex alloys
Asabre, A. and Gemagami, P. and Parsa, A.B. and Wagner, C. and Kostka, A. and Laplanche, G.
JOURNAL OF ALLOYS AND COMPOUNDS. Volume: 899 (2022) - 2022 • 7127
Tuning of optical coherence tomography texture features as a basis for tissue differentiation in glioblastoma samples
Möller, J. and Popanda, E. and Tischoff, I. and Aydın, N.H. and Welp, H. and Brenner, C. and Gerhardt, N.C. and Schmieder, K. and Miller, D. and Hofmann, M.R.
PROGRESS IN BIOMEDICAL OPTICS AND IMAGING - PROCEEDINGS OF SPIE. Volume: 11948 (2022) - 2022 • 7126
The Influence of Nanoconfinement on Electrocatalysis
Wordsworth, J. and Benedetti, T.M. and Somerville, S.V. and Schuhmann, W. and Tilley, R.D. and Gooding, J.J.
ANGEWANDTE CHEMIE - INTERNATIONAL EDITION. Volume: 61 (2022) - 2022 • 7125
Controlled Synthesis of Chromium-Oxide-Based Protective Layers on Pt: Influence of Layer Thickness on Selectivity
Worsley, M. and Smulders, V. and Mei, B.
CATALYSTS. Volume: 12 (2022) - 2022 • 7124
Ferromagnetic Cobalt Disulfide: A CVD Pathway Toward High-Quality and Phase-Pure Thin Films
Wree, J.-L. and Glauber, J.-P. and Zanders, D. and Rogalla, D. and Becher, M. and Griffiths, M.B.E. and Ostendorf, A. and Barry, S.T. and Ney, A. and Devi, A.
ACS APPLIED ELECTRONIC MATERIALS. Volume: 4 (2022) - 2022 • 7123
Bistable H2Pc Molecular Conductance Switch on Ag(100)
Kamiński, W. and Antczak, G. and Morgenstern, K.
JOURNAL OF PHYSICAL CHEMISTRY C. Volume: 126 (2022) - 2022 • 7122
Plasma-Enhanced Atomic Layer Deposition of Molybdenum Oxide Thin Films at Low Temperatures for Hydrogen Gas Sensing
Wree, J.-L. and Rogalla, D. and Ostendorf, A. and Schierbaum, K.D. and Devi, A.
ACS APPLIED MATERIALS AND INTERFACES. Volume: (2022) - 2022 • 7121
Materials for extreme environments
Eswarappa Prameela, S. and Pollock, T.M. and Raabe, D. and Meyers, M.A. and Aitkaliyeva, A. and Chintersingh, K.-L. and Cordero, Z.C. and Graham-Brady, L.
NATURE REVIEWS MATERIALS. Volume: (2022) - 2022 • 7120
Statistical Quantification of Differential Privacy: A Local Approach
Askin, O. and Kutta, T. and Dette, H.
PROCEEDINGS - IEEE SYMPOSIUM ON SECURITY AND PRIVACY. Volume: 2022-May (2022) - 2022 • 7119
Tuning the magnetic phase diagram of Ni-Mn-Ga by Cr and Co substitution
Schröter, M. and Herper, H.C. and Grünebohm, A.
JOURNAL OF PHYSICS D: APPLIED PHYSICS. Volume: 55 (2022) - 2022 • 7118
Electronic Circuit Simulations as a Tool to Understand Distorted Signals in Single-Entity Electrochemistry
Kanokkanchana, K. and Tschulik, K.
JOURNAL OF PHYSICAL CHEMISTRY LETTERS. Volume: 13 (2022) - 2022 • 7117
Optical absorption spectroscopy of reactive oxygen and nitrogen species in a surface dielectric barrier discharge
Schücke, L. and Bodnar, A. and Friedrichs, N. and Böddecker, A. and Peters, N. and Ollegott, K. and Oberste-Beulmann, C. and Wirth, P. and Nguyen-Smith, R.T. and Korolov, I. and Gibson, A.R. and Muhler, M. and Awakowicz, P.
JOURNAL OF PHYSICS D: APPLIED PHYSICS. Volume: 55 (2022) - 2022 • 7116
Electrocatalytic Conversion of Glycerol to Oxalate on Ni Oxide Nanoparticles-Modified Oxidized Multiwalled Carbon Nanotubes
Morales, D.M. and Jambrec, D. and Kazakova, M.A. and Braun, M. and Sikdar, N. and Koul, A. and Brix, A.C. and Seisel, S. and Andronescu, C. and Schuhmann, W.
ACS CATALYSIS. Volume: 12 (2022) - 2022 • 7115
Model for non-equilibrium vacancy diffusion applied to study the Kirkendall effect in high-entropy alloys
Xia, C.-H. and Kundin, J. and Steinbach, I. and Divinski, S.
ACTA MATERIALIA. Volume: 232 (2022) - 2022 • 7114
Impact of interstitial elements on the stacking fault energy of an equiatomic CoCrNi medium entropy alloy: theory and experiments
Moravcik, I. and Zelený, M. and Dlouhy, A. and Hadraba, H. and Moravcikova-Gouvea, L. and Papež, P. and Fikar, O. and Dlouhy, I. and Raabe, D. and Li, Z.
SCIENCE AND TECHNOLOGY OF ADVANCED MATERIALS. Volume: 23 (2022) - 2022 • 7113
3D atomic-scale imaging of mixed Co-Fe spinel oxide nanoparticles during oxygen evolution reaction
Xiang, W. and Yang, N. and Li, X. and Linnemann, J. and Hagemann, U. and Ruediger, O. and Heidelmann, M. and Falk, T. and Aramini, M. and DeBeer, S. and Muhler, M. and Tschulik, K. and Li, T.
NATURE COMMUNICATIONS. Volume: 13 (2022) - 2022 • 7112
Doping of Nanostructured Co3O4with Cr, Mn, Fe, Ni, and Cu for the Selective Oxidation of 2-Propanol
Falk, T. and Budiyanto, E. and Dreyer, M. and Büker, J. and Weidenthaler, C. and Behrens, M. and Tüysüz, H. and Muhler, M. and Peng, B.
ACS APPLIED NANO MATERIALS. Volume: 5 (2022) - 2022 • 7111
Optimizing Fouling Resistance of Poly(Sulfabetaine)s through Backbone and Charge Separation
Karthäuser, J.F. and Koc, J. and Schönemann, E. and Wanka, R. and Aldred, N. and Clare, A.S. and Rosenhahn, A. and Laschewsky, A.
ADVANCED MATERIALS INTERFACES. Volume: (2022) - 2022 • 7110
Revealing the Heterogeneity of Large-Area MoS2 Layers in the Electrocatalytic Hydrogen Evolution Reaction
Schumacher, S. and Madauß, L. and Liebsch, Y. and Tetteh, E.B. and Varhade, S. and Schuhmann, W. and Schleberger, M. and Andronescu, C.
CHEMELECTROCHEM. Volume: 9 (2022) - 2022 • 7109
Rapid Water Diffusion at Cryogenic Temperatures through an Inchworm-like Mechanism
Fang, W. and Meyer auf der Heide, K.M. and Zaum, C. and Michaelides, A. and Morgenstern, K.
NANO LETTERS. Volume: 22 (2022) - 2022 • 7108
Corrosion of NiTi stents induced by galvanic interaction with radiopaque materials
Kassab, E. and Frotscher, M. and Eggeler, G. and Ponciano Gomes, J.A.C.
MATERIALS TODAY COMMUNICATIONS. Volume: 33 (2022) - 2022 • 7107
High-throughput discovery of hydrogen evolution electrocatalysts in the complex solid solution system Co-Cr-Fe-Mo-Ni
Schumacher, S. and Baha, S. and Savan, A. and Andronescu, C. and Ludwig, A.
JOURNAL OF MATERIALS CHEMISTRY A. Volume: (2022) - 2022 • 7106
Atomic/molecular layer deposition of cerium(iii) hybrid thin films using rigid organic precursors
Kaur, P. and Muriqi, A. and Wree, J.-L. and Ghiyasi, R. and Safdar, M. and Nolan, M. and Karppinen, M. and Devi, A.
DALTON TRANSACTIONS. Volume: 51 (2022) - 2022 • 7105
Halogen and structure sensitivity of halobenzene adsorption on copper surfaces
Schunke, C. and Miller, D.P. and Zurek, E. and Morgenstern, K.
PHYSICAL CHEMISTRY CHEMICAL PHYSICS. Volume: 24 (2022) - 2022 • 7104
Mechanism of coupled phase/morphology transformation of 2D manganese oxides through Fe galvanic exchange reaction
Aymerich-Armengol, R. and Cignoni, P. and Ebbinghaus, P. and Linnemann, J. and Rabe, M. and Tschulik, K. and Scheu, C. and Lim, J.
JOURNAL OF MATERIALS CHEMISTRY A. Volume: 10 (2022) - 2022 • 7103
Influence of preheating temperatures on material properties of PBF-LB manufactured hot-work tool steel X37CrMoV5-1
Moritz, S. and Ziesing, U. and Boes, J. and Lentz, J. and Weber, S. and Reuber, M.
PROCEDIA CIRP. Volume: 111 (2022) - 2022 • 7102
Short-Term Heat Treatment of the High-Alloy Cold-Work Tool Steel X153CrMoV12: Calculation of Metastable Microstructural States
Schuppener, J. and Müller, S. and Benito, S. and Weber, S.
STEEL RESEARCH INTERNATIONAL. Volume: (2022) - 2022 • 7101
Investigation on the crack formation in carbon concrete for the use in deck caps on cantilever slabs of bridges
Farwig, K. and Schulte-Schrepping, C. and Curbach, M. and Breitenbücher, R.
STRUCTURAL CONCRETE. Volume: (2022) - 2022 • 7100
Impact of cobalt content and grain growth inhibitors in laser-based powder bed fusion of WC-Co
Schwanekamp, T. and Marginean, G. and Reuber, M. and Ostendorf, A.
INTERNATIONAL JOURNAL OF REFRACTORY METALS AND HARD MATERIALS. Volume: 105 (2022) - 2022 • 7099
Unexpectedly High Capacitance of the Metal Nanoparticle/Water Interface: Molecular-Level Insights into the Electrical Double Layer
Azimzadeh Sani, M. and Pavlopoulos, N.G. and Pezzotti, S. and Serva, A. and Cignoni, P. and Linnemann, J. and Salanne, M. and Gaigeot, M.-P. and Tschulik, K.
ANGEWANDTE CHEMIE - INTERNATIONAL EDITION. Volume: 61 (2022) - 2022 • 7098
OPTIMIZING THE ACID RESISTANCE OF CONCRETE WITH GRANULATED BLAST-FURNACE SLAG
Kempf, L.-A. and Breitenbücher, R. and Gerten, C. and Ehrenberg, A.
ACTA POLYTECHNICA CTU PROCEEDINGS. Volume: 33 (2022) - 2022 • 7097
Reduction of biofilm accumulation by constant and alternating potentials in static and dynamic field experiments
Schwarze, J. and Koc, J. and Koschitzki, F. and Gardner, H. and Hunsucker, K.Z. and Swain, G.W. and Rosenhahn, A.
BIOFOULING. Volume: (2022) - 2022 • 7096
Probing the methanol-assisted autocatalytic formation of methanol over Cu/ZnO/Al2O3 by high-pressure methanol and methyl formate pulses
Schwiderowski, P. and Stürmer, S. and Muhler, M.
REACTION CHEMISTRY AND ENGINEERING. Volume: 7 (2022) - 2022 • 7095
Evolution of an industrial-grade Zr-based bulk metallic glass during multiple laser beam melting
Yang, Z. and Wang, H. and Krauß, S. and Huber, F. and Merle, B. and Schmidt, M. and Markl, M. and Körner, C.
JOURNAL OF NON-CRYSTALLINE SOLIDS. Volume: 589 (2022) - 2022 • 7094
Current developments in CO2 hydrogenation towards methanol: A review related to industrial application
Schwiderowski, P. and Ruland, H. and Muhler, M.
CURRENT OPINION IN GREEN AND SUSTAINABLE CHEMISTRY. Volume: 38 (2022) - 2022 • 7093
8.7-W average power, in-band pumped femtosecond Ho:CALGO laser at 2.1 µm
Yao, W. and Wang, Y. and Tomilov, S. and Hoffmann, M. and Ahmed, S. and Liebald, C. and Rytz, D. and Peltz, M. and Wesemann, V. and Saraceno, C.J.
OPTICS EXPRESS. Volume: 30 (2022) - 2022 • 7092
Full wafer property control of local droplet etched GaAs quantum dots
Babin, H.-G. and Bart, N. and Schmidt, M. and Spitzer, N. and Wieck, A.D. and Ludwig, Ar.
JOURNAL OF CRYSTAL GROWTH. Volume: 591 (2022) - 2022 • 7091
Dynamic cryo-mechanical properties of additively manufactured nanocrystalline nickel 3D microarchitectures
Schwiedrzik, J. and Ramachandramoorthy, R. and Edwards, T.E.J. and Schürch, P. and Casari, D. and Duarte, M.J. and Mohanty, G. and Dehm, G. and Maeder, X. and Philippe, L. and Breguet, J.-M. and Michler, J.
MATERIALS AND DESIGN. Volume: 220 (2022) - 2022 • 7090
Stochastic behavior of an interface-based memristive device
Yarragolla, S. and Hemke, T. and Trieschmann, J. and Zahari, F. and Kohlstedt, H. and Mussenbrock, T.
JOURNAL OF APPLIED PHYSICS. Volume: 131 (2022) - 2022 • 7089
Efficient reconstruction of prior austenite grains in steel from etched light optical micrographs using deep learning and annotations from correlative microscopy
Bachmann, B.-I. and Müller, M. and Britz, D. and Durmaz, A.R. and Ackermann, M. and Shchyglo, O. and Staudt, T. and Mücklich, F.
FRONTIERS IN MATERIALS. Volume: 9 (2022) - 2022 • 7088
Physics inspired compact modelling of BiFeO 3 based memristors
Yarragolla, S. and Du, N. and Hemke, T. and Zhao, X. and Chen, Z. and Polian, I. and Mussenbrock, T.
SCIENTIFIC REPORTS. Volume: 12 (2022) - 2022 • 7087
In-Flight Measurements of Particle Temperature and Velocity with a High-Speed IR Camera During Cold Gas Spraying of In718 and TiAlCrNb
Fiebig, J. and Gagnon, J.-P. and Mauer, G. and Bakan, E. and Vaßen, R.
JOURNAL OF THERMAL SPRAY TECHNOLOGY. Volume: 31 (2022) - 2022 • 7086
Pseudoelastic cycling of ultra-fine-grained NiTi shape-memory wires
Yawny, A. and Sade, M. and Eggeler, G.
INTERNATIONAL JOURNAL OF MATERIALS RESEARCH. Volume: 96 (2022) - 2022 • 7085
Atomistic Simulation of the Lattice Properties of SnSe
Filanovich, A.N. and Lysogorskiy, Y.V. and Povzner, A.A.
SEMICONDUCTORS. Volume: 56 (2022) - 2022 • 7084
Domain Wall Acceleration by Ultrafast Field Application: An Ab Initio-Based Molecular Dynamics Study
Khachaturyan, R. and Dimou, A. and Grünebohm, A.
PHYSICA STATUS SOLIDI - RAPID RESEARCH LETTERS. Volume: 16 (2022) - 2022 • 7083
Determination and analysis of the constitutive parameters of temperature-dependent dislocation-density-based crystal plasticity models
Sedighiani, K. and Traka, K. and Roters, F. and Raabe, D. and Sietsma, J. and Diehl, M.
MECHANICS OF MATERIALS. Volume: 164 (2022) - 2022 • 7082
Exploring MgO/HA ceramic nano-composites for biodegradable implants: Exploring biological properties and micromechanics
Khalili, V. and Sengstock, C. and Kalchev, Y. and Pfetzing-Micklich, J. and Frenzel, J.
SURFACE AND COATINGS TECHNOLOGY. Volume: 445 (2022) - 2022 • 7081
Crystal plasticity simulation of in-grain microstructural evolution during large deformation of IF-steel
Sedighiani, K. and Traka, K. and Roters, F. and Sietsma, J. and Raabe, D. and Diehl, M.
ACTA MATERIALIA. Volume: 237 (2022) - 2022 • 7080
Origins of the hydrogen signal in atom probe tomography: Case studies of alkali and noble metals
Yoo, S.-H. and Kim, S.-H. and Woods, E. and Gault, B. and Todorova, M. and Neugebauer, J.
NEW JOURNAL OF PHYSICS. Volume: 24 (2022) - 2022 • 7079
Quantum dynamics of disordered arrays of interacting superconducting qubits: Signatures of quantum collective states
Fistul, M.V. and Neyenhuys, O. and Bocaz, A.B. and Lisitskiy, M. and Eremin, I.M.
PHYSICAL REVIEW B. Volume: 105 (2022) - 2022 • 7078
The impact of the physical state and the reaction phase in the direct mechanocatalytic Suzuki-Miyaura coupling reaction
Yoo, K. and Fabig, S. and Grätz, S. and Borchardt, L.
FARADAY DISCUSSIONS. Volume: (2022) - 2022 • 7077
Chemo-mechanical phase-field modeling of iron oxide reduction with hydrogen
Bai, Y. and Mianroodi, J.R. and Ma, Y. and da Silva, A.K. and Svendsen, B. and Raabe, D.
ACTA MATERIALIA. Volume: 231 (2022) - 2022 • 7076
Dynamic intralaminar fracture toughness characterisation of unidirectional carbon fibre-reinforced polymer composites using a high-speed servo-hydraulic test set-up
Yoo, S. and Dalli, D. and Catalanotti, G. and Toso, N. and Kessel, F. and Voggenreiter, H.
COMPOSITE STRUCTURES. Volume: 295 (2022) - 2022 • 7075
Laser-equipped gas reaction chamber for probing environmentally sensitive materials at near atomic scale
Khanchandani, H. and El-Zoka, A.A. and Kim, S.-H. and Tezins, U. and Vogel, D. and Sturm, A. and Raabe, D. and Gault, B. and Stephenson, L.T.
PLOS ONE. Volume: 17 (2022) - 2022 • 7074
Influence of non-convergent cation ordering on thermal expansion of rare-earth oxoborates RCa4O(BO3)3 (R = Er, Y, Dy, Gd, Sm, Nd, La)
Münchhalfen, M. and Schreuer, J. and Reuther, C. and Stöcker, H.
MATERIALIA. Volume: 26 (2022) - 2022 • 7073
Oxidation kinetics of atmospheric plasma sprayed environmental barrier coatings
Bakan, E. and Vaßen, R.
JOURNAL OF THE EUROPEAN CERAMIC SOCIETY. Volume: 42 (2022) - 2022 • 7072
Reassessment of mobility parameters for Cantor High Entropy Alloys through an automated procedure
Khorasgani, A.R. and Kundin, J. and Divinski, S.V. and Steinbach, I.
CALPHAD: COMPUTER COUPLING OF PHASE DIAGRAMS AND THERMOCHEMISTRY. Volume: 79 (2022) - 2022 • 7071
A review of the metastable omega phase in beta titanium alloys: the phase transformation mechanisms and its effect on mechanical properties
Ballor, J. and Li, T. and Prima, F. and Boehlert, C.J. and Devaraj, A.
INTERNATIONAL MATERIALS REVIEWS. Volume: (2022) - 2022 • 7070
Dislocation-mediated electronic conductivity in rutile
Muhammad, Q.K. and Bishara, H. and Porz, L. and Dietz, C. and Ghidelli, M. and Dehm, G. and Frömling, T.
MATERIALS TODAY NANO. Volume: 17 (2022) - 2022 • 7069
Trapping an Oxidized and Protonated Intermediate of the [FeFe]-Hydrogenase Cofactor under Mildly Reducing Conditions
Senger, M. and Duan, J. and Pavliuk, M.V. and Apfel, U.-P. and Haumann, M. and Stripp, S.T.
INORGANIC CHEMISTRY. Volume: 61 (2022) - 2022 • 7068
Elastoresistivity in the incommensurate charge density wave phase of BaNi2(As1−xPx)2
Frachet, M. and Wiecki, P. and Lacmann, T. and Souliou, S.M. and Willa, K. and Meingast, C. and Merz, M. and Haghighirad, A.-A. and Le Tacon, M. and Böhmer, A.E.
NPJ QUANTUM MATERIALS. Volume: 7 (2022) - 2022 • 7067
Catalytic Biosensors Operating under Quasi-Equilibrium Conditions for Mitigating the Changes in Substrate Diffusion
Muhs, A. and Bobrowski, T. and Lielpētere, A. and Schuhmann, W.
ANGEWANDTE CHEMIE - INTERNATIONAL EDITION. Volume: 61 (2022) - 2022 • 7066
The effect of buoyancy driven convection on the growth and dissolution of bubbles on electrodes
Sepahi, F. and Pande, N. and Chong, K.L. and Mul, G. and Verzicco, R. and Lohse, D. and Mei, B.T. and Krug, D.
ELECTROCHIMICA ACTA. Volume: 403 (2022) - 2022 • 7065
Unravelling Composition–Activity–Stability Trends in High Entropy Alloy Electrocatalysts by Using a Data-Guided Combinatorial Synthesis Strategy and Computational Modeling
Banko, L. and Krysiak, O.A. and Pedersen, J.K. and Xiao, B. and Savan, A. and Löffler, T. and Baha, S. and Rossmeisl, J. and Schuhmann, W. and Ludwig, Al.
ADVANCED ENERGY MATERIALS. Volume: 12 (2022) - 2022 • 7064
Light-driven in vitro catalysis with photosynthetic biohybrids [Lichtgetriebene in vitro-Katalyse mit photosynthetischen Biohybriden]
Frank, A. and Conzuelo, F. and Schuhmann, W. and Nowaczyk, M.M.
BIOSPEKTRUM. Volume: 28 (2022) - 2022 • 7063
Understanding Alkali Contamination in Colloidal Nanomaterials to Unlock Grain Boundary Impurity Engineering
Kim, S.-H. and Yoo, S.-H. and Chakraborty, P. and Jeong, J. and Lim, J. and El-Zoka, A.A. and Zhou, X. and Stephenson, L.T. and Hickel, T. and Neugebauer, J. and Scheu, C. and Todorova, M. and Gault, B.
JOURNAL OF THE AMERICAN CHEMICAL SOCIETY. Volume: 144 (2022) - 2022 • 7062
Propagation dynamics and interaction of multiple streamers at and above adjacent dielectric pellets in a packed bed plasma reactor
Mujahid, Z.-U.I. and Korolov, I. and Liu, Y. and Mussenbrock, T. and Schulze, J.
JOURNAL OF PHYSICS D: APPLIED PHYSICS. Volume: 55 (2022) - 2022 • 7061
Non-uniform He bubble formation in W/W2C composite: Experimental and ab-initio study
Šestan, A. and Sreekala, L. and Markelj, S. and Kelemen, M. and Zavašnik, J. and Liebscher, C.H. and Dehm, G. and Hickel, T. and Čeh, M. and Novak, S. and Jenuš, P.
ACTA MATERIALIA. Volume: 226 (2022) - 2022 • 7060
Reliability-based optimization of structural topologies using artificial neural networks
Freitag, S. and Peters, S. and Edler, P. and Meschke, G.
PROBABILISTIC ENGINEERING MECHANICS. Volume: (2022) - 2022 • 7059
Controlled Doping of Electrocatalysts through Engineering Impurities
Kim, S.-H. and Yoo, S.-H. and Shin, S. and El-Zoka, A.A. and Kasian, O. and Lim, J. and Jeong, J. and Scheu, C. and Neugebauer, J. and Lee, H. and Todorova, M. and Gault, B.
ADVANCED MATERIALS. Volume: 34 (2022) - 2022 • 7058
Understanding the Degradation of a Model Si Anode in a Li-Ion Battery at the Atomic Scale
Kim, S.-H. and Dong, K. and Zhao, H. and El-Zoka, A.A. and Zhou, X. and Woods, E.V. and Giuliani, F. and Manke, I. and Raabe, D. and Gault, B.
JOURNAL OF PHYSICAL CHEMISTRY LETTERS. Volume: 13 (2022) - 2022 • 7057
Coulomb blockade: Toward charge control of self-assembled GaN quantum dots at room temperature
Sgroi, C.A. and Brault, J. and Duboz, J.-Y. and Chenot, S. and Vennéguès, P. and Ludwig, Ar. and Wieck, A.D.
APPLIED PHYSICS LETTERS. Volume: 120 (2022) - 2022 • 7056
Integration of GaAs waveguides on a silicon substrate for quantum photonic circuits
Shadmani, A. and Thomas, R.A. and Liu, Z. and Papon, C. and Heck, M.J.R. and Volet, N. and Scholz, S. and Wieck, A.D. and Ludwig, Ar. and Lodahl, P. and Midolo, L.
OPTICS EXPRESS. Volume: 30 (2022) - 2022 • 7055
Unveiling Ruthenium(II) Diazadienyls for Gas Phase Deposition Processes: Low Resistivity Ru Thin Films and Their Performance in the Acidic Oxygen Evolution Reaction
Zanders, D. and Obenlüneschloß, J. and Wree, J.-L. and Jagosz, J. and Kaur, P. and Boysen, N. and Rogalla, D. and Kostka, A. and Bock, C. and Öhl, D. and Gock, M. and Schuhmann, W. and Devi, A.
ADVANCED MATERIALS INTERFACES. Volume: (2022) - 2022 • 7054
Wafer-scale epitaxial modulation of quantum dot density
Bart, N. and Dangel, C. and Zajac, P. and Spitzer, N. and Ritzmann, J. and Schmidt, M. and Babin, H.G. and Schott, R. and Valentin, S.R. and Scholz, S. and Wang, Y. and Uppu, R. and Najer, D. and Löbl, M.C. and Tomm, N. and Javadi, A. and Antoniadis, N.O. and Midolo, L. and Müller, K. and Warburton, R.J. and Lodahl, P. and Wieck, A.D. and Finley, J.J. and Ludwig, Ar.
NATURE COMMUNICATIONS. Volume: 13 (2022) - 2022 • 7053
Limitations of empirical supercell extrapolation for calculations of point defects in bulk, at surfaces, and in two-dimensional materials
Freysoldt, C. and Neugebauer, J. and Tan, A.M.Z. and Hennig, R.G.
PHYSICAL REVIEW B. Volume: 105 (2022) - 2022 • 7052
Molecular Permeation in Freestanding Bilayer Silica
Naberezhnyi, D. and Mai, L. and Doudin, N. and Ennen, I. and Hütten, A. and Altman, E.I. and Devi, A. and Dementyev, P.
NANO LETTERS. Volume: 22 (2022) - 2022 • 7051
An Unusual Tri-coordinate Co(II) Silylamide with Potential for Chemical Vapor Deposition Processes
Zanders, D. and Masuda, J.D. and Lowe, B. and Curtis, S. and Devi, A. and Barry, S.T.
ZEITSCHRIFT FUR ANORGANISCHE UND ALLGEMEINE CHEMIE. Volume: (2022) - 2022 • 7050
Dual phase patterning during a congruent grain boundary phase transition in elemental copper
Frommeyer, L. and Brink, T. and Freitas, R. and Frolov, T. and Dehm, G. and Liebscher, C.H.
NATURE COMMUNICATIONS. Volume: 13 (2022) - 2022 • 7049
Cementite decomposition in 100Cr6 bearing steel during high-pressure torsion: Influence of precipitate composition, size, morphology and matrix hardness
Kiranbabu, S. and Tung, P.-Y. and Sreekala, L. and Prithiv, T.S. and Hickel, T. and Pippan, R. and Morsdorf, L. and Herbig, M.
MATERIALS SCIENCE AND ENGINEERING A. Volume: 833 (2022) - 2022 • 7048
Characterization of a robot-assisted UV-C disinfection for the inactivation of surface-associated microorganisms and viruses
Fuchs, F.M. and Bibinov, N. and Blanco, E.V. and Pfaender, S. and Theiß, S. and Wolter, H. and Awakowicz, P.
JOURNAL OF PHOTOCHEMISTRY AND PHOTOBIOLOGY. Volume: 11 (2022) - 2022 • 7047
Electron g-factor determined for quantum dot circuit fabricated from (110)-oriented GaAs quantum well
Nakagawa, T. and Lamoureux, S. and Fujita, T. and Ritzmann, J. and Ludwig, Ar. and Wieck, A.D. and Oiwa, A. and Korkusinski, M. and Sachrajda, A. and Austing, D.G. and Gaudreau, L.
JOURNAL OF APPLIED PHYSICS. Volume: 131 (2022) - 2022 • 7046
Fusion of Optical and Millimeter Wave SAR Sensing for Object Recognition in Indoor Environment
Batra, A. and Hark, T. and Schorlemer, J. and Pohl, N. and Rolfes, I. and Wiemeler, M. and Gohringer, D. and Kaiser, T. and Barowski, J.
2022 5TH INTERNATIONAL WORKSHOP ON MOBILE TERAHERTZ SYSTEMS, IWMTS 2022. Volume: (2022) - 2022 • 7045
Characterizing Localized Microstructural Deformation of Multiphase Steel by Crystal Plasticity Simulation with Multi-Constitutive Law [複数の材料構成則を用いた結晶塑性解析による複相組織鋼の局所変形挙動評価]
Fujita, N. and Yasuda, K. and Ishikawa, N. and Diehl, M. and Roters, F. and Raabe, D.
JOURNAL OF THE JAPAN SOCIETY FOR TECHNOLOGY OF PLASTICITY. Volume: 63 (2022) - 2022 • 7044
High-dimensional neural network potentials for accurate vibrational frequencies: the formic acid dimer benchmark
Shanavas Rasheeda, D. and Martín Santa Daría, A. and Schröder, B. and Mátyus, E. and Behler, J.
PHYSICAL CHEMISTRY CHEMICAL PHYSICS. Volume: 24 (2022) - 2022 • 7043
Micromechanical Modeling of AlSi10Mg Processed by Laser-Based Additive Manufacturing: From as-Built to Heat-Treated Microstructures
Nammalvar Raja Rajan, A. and Krochmal, M. and Wegener, T. and Biswas, A. and Hartmaier, A. and Niendorf, T. and Moeini, G.
MATERIALS. Volume: 15 (2022) - 2022 • 7042
A Broadband Test Environment Concept for FMCW Radars based on Overmoded Waveguides
Funk, M. and Dahl, C. and Barowski, J. and Rolfes, I. and Schulz, C.
2022 19TH EUROPEAN RADAR CONFERENCE, EURAD 2022. Volume: (2022) - 2022 • 7041
Engineering of Cation Occupancy of CoFe2O4 Oxidation Catalysts by Nanosecond, Single-Pulse Laser Excitation in Water
Zerebecki, S. and Salamon, S. and Landers, J. and Yang, Y. and Tong, Y. and Budiyanto, E. and Waffel, D. and Dreyer, M. and Saddeler, S. and Kox, T. and Kenmoe, S. and Spohr, E. and Schulz, S. and Behrens, M. and Muhler, M. and Tüysüz, H. and Kramer Campen, R. and Wende, H. and Reichenberger, S. and Barcikowski, S.
CHEMCATCHEM. Volume: 14 (2022) - 2022 • 7040
Quantum interference of identical photons from remote GaAs quantum dots
Zhai, L. and Nguyen, G.N. and Spinnler, C. and Ritzmann, J. and Löbl, M.C. and Wieck, A.D. and Ludwig, Ar. and Javadi, A. and Warburton, R.J.
NATURE NANOTECHNOLOGY. Volume: 17 (2022) - 2022 • 7039
Bioelectrocatalytic CO2Reduction by Redox Polymer-Wired Carbon Monoxide Dehydrogenase Gas Diffusion Electrodes
Becker, J.M. and Lielpetere, A. and Szczesny, J. and Junqueira, J.R.C. and Rodríguez-Maciá, P. and Birrell, J.A. and Conzuelo, F. and Schuhmann, W.
ACS APPLIED MATERIALS AND INTERFACES. Volume: 14 (2022) - 2022 • 7038
In Situ Carbon Corrosion and Cu Leaching as a Strategy for Boosting Oxygen Evolution Reaction in Multimetal Electrocatalysts
Zhang, J. and Quast, T. and He, W. and Dieckhöfer, S. and Junqueira, J.R.C. and Öhl, D. and Wilde, P. and Jambrec, D. and Chen, Y.-T. and Schuhmann, W.
ADVANCED MATERIALS. Volume: (2022) - 2022 • 7037
Assembling a Low-volume Biofuel Cell on a Screen-printed Electrode for Glucose Sensing
Becker, J.M. and Lielpetere, A. and Szczesny, J. and Ruff, A. and Conzuelo, F. and Schuhmann, W.
ELECTROANALYSIS. Volume: 34 (2022) - 2022 • 7036
Unravelling the lamellar size-dependent fracture behavior of fully lamellar intermetallic γ-TiAl
Neogi, A. and Janisch, R.
ACTA MATERIALIA. Volume: 227 (2022) - 2022 • 7035
Validation of the Powder Metallurgical Processing of Duplex Stainless Steels through Hot Isostatic Pressing with Integrated Heat Treatment
Becker, L. and Lentz, J. and Kramer, B. and Rottstegge, A. and Broeckmann, C. and Theisen, W. and Weber, S.
MATERIALS. Volume: 15 (2022) - 2022 • 7034
Quantification of extremely small-structured ferritic-austenitic phase fractions in stainless steels manufactured by laser powder bed fusion
Becker, L. and Boes, J. and Lentz, J. and Cui, C. and Uhlenwinkel, V. and Steinbacher, M. and Fechte-Heinen, R. and Theisen, W. and Weber, S.
MATERIALIA. Volume: 22 (2022) - 2022 • 7033
Oxygen vacancies-enriched Ta-doped Bi2WO6 with Pt as cocatalyst for boosting the dehydrogenation of benzyl alcohol in water
Shen, Z. and Hu, Y. and Pan, Q. and Huang, C. and Zhu, B. and Xia, W. and Wang, H. and Yue, J. and Muhler, M. and Zhao, G. and Wang, X. and Huang, X.
APPLIED SURFACE SCIENCE. Volume: 571 (2022) - 2022 • 7032
Modeling of the Effect of Carrier Gas Injection on the Laminarity of the Plasma Jet Generated by a Cascaded Spray Gun
Zhang, H. and Mauer, G. and Liu, S. and Liu, M. and Jia, Y. and Li, C. and Li, C. and Vaßen, R.
COATINGS. Volume: 12 (2022) - 2022 • 7031
Numerical Multi-level Model for Fibre Reinforced Concrete: Validation and Comparison with Fib Model Code
Neu, G.E. and Gudžulić, V. and Meschke, G.
RILEM BOOKSERIES. Volume: 36 (2022) - 2022 • 7030
Reliability based optimization of steel-fibre segmental tunnel linings subjected to thrust jack loadings
Neu, G.E. and Edler, P. and Freitag, S. and Gudžulić, V. and Meschke, G.
ENGINEERING STRUCTURES. Volume: 254 (2022) - 2022 • 7029
Defect-characterized phase transition kinetics
Zhang, X. and Zhang, J. and Wang, H. and Rogal, J. and Li, H.-Y. and Wei, S.-H. and Hickel, T.
APPLIED PHYSICS REVIEWS. Volume: 9 (2022) - 2021 • 7028
Online Geological Anomaly Detection Using Machine Learning in Mechanized Tunneling
Cao, B.-T. and Saadallah, A. and Egorov, A. and Freitag, S. and Meschke, G. and Morik, K.
LECTURE NOTES IN CIVIL ENGINEERING. Volume: 125 (2021) - 2021 • 7027
Molecular Dynamics Simulations of the Breathing Phase Transition of MOF Nanocrystallites II: Explicitly Modeling the Pressure Medium
Schaper, L. and Keupp, J. and Schmid, R.
FRONTIERS IN CHEMISTRY. Volume: 9 (2021) - 2021 • 7026
Correlation of process conditions, porosity levels and crystallinity in atmospherically plasma sprayed Yb2Si2O7 environmental barrier coatings
Vaßen, R. and Bakan, E. and Sebold, D. and Sohn, Y.J.
JOURNAL OF COMPOSITES SCIENCE. Volume: 5 (2021) - 2021 • 7025
Low Fouling Polysulfobetaines with Variable Hydrophobic Content
Schardt, L. and Martínez Guajardo, A. and Koc, J. and Clarke, J.L. and Finlay, J.A. and Clare, A.S. and Gardner, H. and Swain, G.W. and Hunsucker, K. and Laschewsky, A. and Rosenhahn, A.
MACROMOLECULAR RAPID COMMUNICATIONS. Volume: (2021) - 2021 • 7024
Interplay between nematicity and Bardasis-Schrieffer modes in the short-time dynamics of unconventional superconductors
Müller, M.A. and Volkov, P.A. and Paul, I. and Eremin, I.M.
PHYSICAL REVIEW B. Volume: 103 (2021) - 2021 • 7023
Plasma State Supervision Utilizing 140 GHz Radar Measurements
Schenkel, F. and Schulz, C. and Baer, C. and Rolfes, I.
2021 18TH EUROPEAN RADAR CONFERENCE, EURAD 2021. Volume: (2021) - 2021 • 7022
Comparison between optical coherence tomography imaging and histological sections of peripheral nerves
Carolus, A.E. and Möller, J. and Hofmann, M.R. and van de Nes, J.A.P. and Welp, H. and Schmieder, K. and Brenke, C.
JOURNAL OF NEUROSURGERY. Volume: 134 (2021) - 2021 • 7021
Ignition and propagation of nanosecond pulsed plasmas in distilled water - Negative vs positive polarity applied to a pin electrode
Grosse, K. and Falke, M. and Von Keudell, A.
JOURNAL OF APPLIED PHYSICS. Volume: 129 (2021) - 2021 • 7020
Signatures of Bardasis-Schrieffer mode excitation in third-harmonic generated currents
Müller, M.A. and Eremin, I.M.
PHYSICAL REVIEW B. Volume: 104 (2021) - 2021 • 7019
Influence of surface wettability on methane hydrate formation in hydrophilic and hydrophobic mesoporous silicas
Casco, M.E. and Grätz, S. and Wallacher, D. and Grimm, N. and Többens, D.M. and Bilo, M. and Speil, N. and Fröba, M. and Borchardt, L.
CHEMICAL ENGINEERING JOURNAL. Volume: 405 (2021) - 2021 • 7018
Additive manufacturing of a carbon-martensitic hot-work tool steel using a powder mixture – Microstructure, post-processing, mechanical properties
Großwendt, F. and Röttger, A. and Strauch, A. and Chehreh, A. and Uhlenwinkel, V. and Fechte-Heinen, R. and Walther, F. and Weber, S. and Theisen, W.
MATERIALS SCIENCE AND ENGINEERING A. Volume: 827 (2021) - 2021 • 7017
Configurational Entropy Driven High-Pressure Behaviour of a Flexible Metal–Organic Framework (MOF)
Vervoorts, P. and Keupp, J. and Schneemann, A. and Hobday, C.L. and Daisenberger, D. and Fischer, R.A. and Schmid, R. and Kieslich, G.
ANGEWANDTE CHEMIE - INTERNATIONAL EDITION. Volume: 60 (2021) - 2021 • 7016
Cryo-EM photosystem I structure reveals adaptation mechanisms to extreme high light in Chlorella ohadii
Caspy, I. and Neumann, E. and Fadeeva, M. and Liveanu, V. and Savitsky, A. and Frank, A. and Kalisman, Y.L. and Shkolnisky, Y. and Murik, O. and Treves, H. and Hartmann, V. and Nowaczyk, M.M. and Schuhmann, W. and Rögner, M. and Willner, I. and Kaplan, A. and Schuster, G. and Nelson, N. and Lubitz, W. and Nechushtai, R.
NATURE PLANTS. Volume: 7 (2021) - 2021 • 7015
Impact of the allowed compositional range of additively manufactured 316l stainless steel on processability and material properties
Großwendt, F. and Becker, L. and Röttger, A. and Chehreh, A.B. and Strauch, A.L. and Uhlenwinkel, V. and Lentz, J. and Walther, F. and Fechte-Heinen, R. and Weber, S. and Theisen, W.
MATERIALS. Volume: 14 (2021) - 2021 • 7014
Investigation of the orientation relationship between nano-sized G-phase precipitates and austenite with scanning nano-beam electron diffraction using a pixelated detector
Cautaerts, N. and Rauch, E.F. and Jeong, J. and Dehm, G. and Liebscher, C.H.
SCRIPTA MATERIALIA. Volume: 201 (2021) - 2021 • 7013
B2 ordering in body-centered-cubic AlNbTiV refractory high-entropy alloys
Körmann, F. and Kostiuchenko, T. and Shapeev, A. and Neugebauer, J.
PHYSICAL REVIEW MATERIALS. Volume: 5 (2021) - 2021 • 7012
A new method for the in vivo identification of degenerated material property ranges of the human eye: feasibility analysis based on synthetic data
Muench, S. and Roellig, M. and Balzani, D.
BIOMECHANICS AND MODELING IN MECHANOBIOLOGY. Volume: (2021) - 2021 • 7011
Presentation of the Dana Medal of the Mineralogical Society of America for 2020 to Daniela Rubatto
Chakraborty, S.
AMERICAN MINERALOGIST. Volume: 106 (2021) - 2021 • 7010
Elastic, piezoelectric, and dielectric properties of rare-earth calcium oxoborates RCa4O(BO3)3(R = Er, Y, Dy, Gd, Sm, Nd, La)
Münchhalfen, M. and Schreuer, J. and Reuther, C. and Mehner, E. and Stöcker, H.
JOURNAL OF APPLIED PHYSICS. Volume: 130 (2021) - 2021 • 7009
On the fracture behavior of Cr2AlC coatings
Völker, B. and Stelzer, B. and Mráz, S. and Rueß, H. and Sahu, R. and Kirchlechner, C. and Dehm, G. and Schneider, J.M.
MATERIALS AND DESIGN. Volume: 206 (2021) - 2021 • 7008
Phase decomposition in nanocrystalline Cr0.8Cu0.2 thin films
Chakraborty, J. and Harzer, T.P. and Duarte, M.J. and Dehm, G.
JOURNAL OF ALLOYS AND COMPOUNDS. Volume: 888 (2021) - 2021 • 7007
Real-Time Measurement of Cellobiose and Glucose Formation during Enzymatic Biomass Hydrolysis
Chang, H. and Wohlschlager, L. and Csarman, F. and Ruff, A. and Schuhmann, W. and Scheiblbrandner, S. and Ludwig, R.
ANALYTICAL CHEMISTRY. Volume: 93 (2021) - 2021 • 7006
Probabilistic teleportation of a quantum dot spin qubit
Kojima, Y. and Nakajima, T. and Noiri, A. and Yoneda, J. and Otsuka, T. and Takeda, K. and Li, S. and Bartlett, S.D. and Ludwig, Ar. and Wieck, A.D. and Tarucha, S.
NPJ QUANTUM INFORMATION. Volume: 7 (2021) - 2021 • 7005
A MULTISCALE VISION—ILLUSTRATIVE APPLICATIONS FROM BIOLOGY TO ENGINEERING
Schlick, T. and Portillo-Ledesma, S. and Blaszczyk, M. and Dalessandro, L. and Ghosh, S. and Hackl, K. and Harnish, C. and Kotha, S. and Livescu, D. and Masud, A. and Matouš, K. and Moyeda, A. and Oskay, C. and Fish, J.
INTERNATIONAL JOURNAL FOR MULTISCALE COMPUTATIONAL ENGINEERING. Volume: 19 (2021) - 2021 • 7004
On the role of pre-existing defects in influencing hardness in nanoscale indentations — Insights from atomistic simulations
Chauniyal, A. and Dehm, G. and Janisch, R.
JOURNAL OF THE MECHANICS AND PHYSICS OF SOLIDS. Volume: 154 (2021) - 2021 • 7003
Finite-temperature interplay of structural stability, chemical complexity, and elastic properties of bcc multicomponent alloys from ab initio trained machine-learning potentials
Gubaev, K. and Ikeda, Y. and Tasnádi, F. and Neugebauer, J. and Shapeev, A.V. and Grabowski, B. and Körmann, F.
PHYSICAL REVIEW MATERIALS. Volume: 5 (2021) - 2021 • 7002
Understanding creep of a single-crystalline Co-Al-W-Ta superalloy by studying the deformation mechanism, segregation tendency and stacking fault energy
Volz, N. and Xue, F. and Zenk, C.H. and Bezold, A. and Gabel, S. and Subramanyam, A.P.A. and Drautz, R. and Hammerschmidt, T. and Makineni, S.K. and Gault, B. and Göken, M. and Neumeier, S.
ACTA MATERIALIA. Volume: 214 (2021) - 2021 • 7001
Oxygenated PAH Formation Chemistry Investigation in Anisole Jet Stirred Reactor Oxidation by a Thermodynamic Approach
Chen, B. and Kruse, S. and Schmid, R. and Cai, L. and Hansen, N. and Pitsch, H.
ENERGY AND FUELS. Volume: 35 (2021) - 2021 • 7000
Design of a Co–Al–W–Ta Alloy Series with Varying γ′ Volume Fraction and Their Thermophysical Properties
Volz, N. and Xue, F. and Bezold, A. and Zenk, C.H. and Fries, S.G. and Schreuer, J. and Neumeier, S. and Göken, M.
METALLURGICAL AND MATERIALS TRANSACTIONS A: PHYSICAL METALLURGY AND MATERIALS SCIENCE. Volume: 52 (2021) - 2021 • 6999
Efficient implementation of atom-density representations
Musil, F. and Veit, M. and Goscinski, A. and Fraux, G. and Willatt, M.J. and Stricker, M. and Junge, T. and Ceriotti, M.
JOURNAL OF CHEMICAL PHYSICS. Volume: 154 (2021) - 2021 • 6998
Comprehensive Data Set of Single Particle Combustion under Oxy-fuel Conditions, Part II: Data Set
Vorobiev, N. and Valentiner, S. and Schiemann, M. and Scherer, V.
COMBUSTION SCIENCE AND TECHNOLOGY. Volume: 193 (2021) - 2021 • 6997
Comprehensive Data Set of Single Particle Combustion under Oxy-fuel Conditions, Part I: Measurement Technique
Vorobiev, N. and Valentiner, S. and Schiemann, M. and Scherer, V.
COMBUSTION SCIENCE AND TECHNOLOGY. Volume: 193 (2021) - 2021 • 6996
Microstructural Changes During Short-Term Heat Treatment of Martensitic Stainless Steel—Simulation and Experimental Verification
Schmidtseifer, N. and Weber, S.
METALLURGICAL AND MATERIALS TRANSACTIONS A: PHYSICAL METALLURGY AND MATERIALS SCIENCE. Volume: 52 (2021) - 2021 • 6995
Highly Selective Guiding Springs for Large Displacements in Surface MEMS
Schmitt, P. and Schmitt, L. and Tsivin, N. and Hoffmann, M.
JOURNAL OF MICROELECTROMECHANICAL SYSTEMS. Volume: 30 (2021) - 2021 • 6994
Reduced order multiscale simulation of diffuse damage in concrete
Vu, G. and Diewald, F. and Timothy, J.J. and Gehlen, C. and Meschke, G.
MATERIALS. Volume: 14 (2021) - 2021 • 6993
Modelling of microstructure formation in metal additive manufacturing: Recent progress, research gaps and perspectives
Gunasegaram, D.R. and Steinbach, I.
METALS. Volume: 11 (2021) - 2021 • 6992
Large stepwise discrete microsystem displacements based on electrostatic bending plate actuation
Schmitt, L. and Hoffmann, M.
ACTUATORS. Volume: 10 (2021) - 2021 • 6991
A combined experimental and first-principles based assessment of finite-temperature thermodynamic properties of intermetallic al3sc
Gupta, A. and Tas, B. and Korbmacher, D. and Dutta, B. and Neitzel, Y. and Grabowski, B. and Hickel, T. and Esin, V. and Divinski, S.V. and Wilde, G. and Neugebauer, J.
MATERIALS. Volume: 14 (2021) - 2021 • 6990
Electrocatalytic oxidation of 2-propanol on PtxIr100-x bifunctional electrocatalysts – A thin-film materials library study
Kormányos, A. and Savan, A. and Ludwig, Al. and Speck, F.D. and Mayrhofer, K.J.J. and Cherevko, S.
JOURNAL OF CATALYSIS. Volume: 396 (2021) - 2021 • 6989
3-Bit digital-to-analog converter with mechanical amplifier for binary encoded large displacements
Schmitt, L. and Schmitt, P. and Hoffmann, M.
ACTUATORS. Volume: 10 (2021) - 2021 • 6988
The Influence of a Changing Local Environment during Photoinduced CO2 Dissociation
Vyshnepolsky, M. and Ding, Z.-B. and Srivastava, P. and Tesarik, P. and Mazhar, H. and Maestri, M. and Morgenstern, K.
ANGEWANDTE CHEMIE - INTERNATIONAL EDITION. Volume: (2021) - 2021 • 6987
Design and fabrication of MEMS reflectors for THz reflect-arrays
Schmitt, L. and Liu, X. and Czylwik, A. and Hoffmann, M.
2021 4TH INTERNATIONAL WORKSHOP ON MOBILE TERAHERTZ SYSTEMS, IWMTS 2021. Volume: (2021) - 2021 • 6986
Atomic oxygen generation in atmospheric pressure RF plasma jets driven by tailored voltage waveforms in mixtures of He and O2
Korolov, I. and Steuer, D. and Bischoff, L. and Hübner, G. and Liu, Y. and Schulz-Von der Gathen, V. and Böke, M. and Mussenbrock, T. and Schulze, J.
JOURNAL OF PHYSICS D: APPLIED PHYSICS. Volume: 54 (2021) - 2021 • 6985
A Perspective on Heterogeneous Catalysts for the Selective Oxidation of Alcohols
Najafishirtari, S. and Friedel Ortega, K. and Douthwaite, M. and Pattisson, S. and Hutchings, G.J. and Bondue, C.J. and Tschulik, K. and Waffel, D. and Peng, B. and Deitermann, M. and Busser, G.W. and Muhler, M. and Behrens, M.
CHEMISTRY - A EUROPEAN JOURNAL. Volume: (2021) - 2021 • 6984
Energy efficiency of voltage waveform tailoring for the generation of excited species in RF plasma jets operated in He/N2mixtures
Korolov, I. and Donkó, Z. and Hübner, G. and Liu, Y. and Mussenbrock, T. and Schulze, J.
PLASMA SOURCES SCIENCE AND TECHNOLOGY. Volume: 30 (2021) - 2021 • 6983
Suppression of Surface-Related Loss in a Gated Semiconductor Microcavity
Najer, D. and Tomm, N. and Javadi, A. and Korsch, A.R. and Petrak, B. and Riedel, D. and Dolique, V. and Valentin, S.R. and Schott, R. and Wieck, A.D. and Ludwig, Ar. and Warburton, R.J.
PHYSICAL REVIEW APPLIED. Volume: 15 (2021) - 2021 • 6982
Defect phases–thermodynamics and impact on material properties
Korte-Kerzel, S. and Hickel, T. and Huber, L. and Raabe, D. and Sandlöbes-Haut, S. and Todorova, M. and Neugebauer, J.
INTERNATIONAL MATERIALS REVIEWS. Volume: (2021) - 2021 • 6981
The role of Ca, Al and Zn on room temperature ductility and grain boundary cohesion of magnesium
Nandy, S. and Tsai, S.-P. and Stephenson, L. and Raabe, D. and Zaefferer, S.
JOURNAL OF MAGNESIUM AND ALLOYS. Volume: 9 (2021) - 2021 • 6980
Lattice-shifted nematic quantum critical point in FeSe1−xSx
Chibani, S. and Farina, D. and Massat, P. and Cazayous, M. and Sacuto, A. and Urata, T. and Tanabe, Y. and Tanigaki, K. and Böhmer, A.E. and Canfield, P.C. and Merz, M. and Karlsson, S. and Strobel, P. and Toulemonde, P. and Paul, I. and Gallais, Y.
NPJ QUANTUM MATERIALS. Volume: 6 (2021) - 2021 • 6979
Plasticity induced by nanoindentation in a CrCoNi medium-entropy alloy studied by accurate electron channeling contrast imaging revealing dislocation-low angle grain boundary interactions
Habiyaremye, F. and Guitton, A. and Schäfer, F. and Scholz, F. and Schneider, M. and Frenzel, J. and Laplanche, G. and Maloufi, N.
MATERIALS SCIENCE AND ENGINEERING A. Volume: 817 (2021) - 2021 • 6978
Amphiphilic Zwitterionic Acrylate/Methacrylate Copolymers for Marine Fouling-Release Coatings
Koschitzki, F. and Wanka, R. and Sobota, L. and Gardner, H. and Hunsucker, K.Z. and Swain, G.W. and Rosenhahn, A.
LANGMUIR. Volume: 37 (2021) - 2021 • 6977
An automatized workflow from molecular dynamic simulation to quantum chemical methods to identify elementary reactions and compute reaction constants
Schmitz, G. and Yönder, Ö. and Schnieder, B. and Schmid, R. and Hättig, C.
JOURNAL OF COMPUTATIONAL CHEMISTRY. Volume: 42 (2021) - 2021 • 6976
Ab initio based models for temperature-dependent magnetochemical interplay in bcc Fe-Mn alloys
Schneider, A. and Fu, C.-C. and Waseda, O. and Barreteau, C. and Hickel, T.
PHYSICAL REVIEW B. Volume: 103 (2021) - 2021 • 6975
Direct visualization of a static incommensurate antiferromagnetic order in Fe-doped Bi2Sr2CaCu2O8+δ
Wan, S. and Li, H. and Choubey, P. and Gu, Q. and Li, H. and Yang, H. and Eremin, I.M. and Gu, G. and Wen, H.-H.
PROCEEDINGS OF THE NATIONAL ACADEMY OF SCIENCES OF THE UNITED STATES OF AMERICA. Volume: 118 (2021) - 2021 • 6974
Data compilation regarding the effects of grain size and temperature on the strength of the single-phase FCC CrFeNi medium-entropy alloy
Schneider, M. and Laplanche, G.
DATA IN BRIEF. Volume: 34 (2021) - 2021 • 6973
The effect of laser nitriding on surface characteristics and wear resistance of niti alloy with low power fiber laser
Wang, H. and Nett, R. and Gurevich, E.L. and Ostendorf, A.
APPLIED SCIENCES (SWITZERLAND). Volume: 11 (2021) - 2021 • 6972
Parallel Dislocation Networks and Cottrell Atmospheres Reduce Thermal Conductivity of PbTe Thermoelectrics
Abdellaoui, L. and Chen, Z. and Yu, Y. and Luo, T. and Hanus, R. and Schwarz, T. and Bueno Villoro, R. and Cojocaru-Mirédin, O. and Snyder, G.J. and Raabe, D. and Pei, Y. and Scheu, C. and Zhang, S.
ADVANCED FUNCTIONAL MATERIALS. Volume: 31 (2021) - 2021 • 6971
Effects of temperature on mechanical properties and deformation mechanisms of the equiatomic CrFeNi medium-entropy alloy
Schneider, M. and Laplanche, G.
ACTA MATERIALIA. Volume: 204 (2021) - 2021 • 6970
Automated assessment of a kinetic database for fcc Co-Cr-Fe-Mn-Ni high entropy alloys
Abrahams, K. and Zomorodpoosh, S. and Khorasgani, A.R. and Roslyakova, I. and Steinbach, I. and Kundin, J.
MODELLING AND SIMULATION IN MATERIALS SCIENCE AND ENGINEERING. Volume: 29 (2021) - 2021 • 6969
CrMnFeCoNi high entropy alloys with carbon and nitrogen: mechanical properties, wear and corrosion resistance
Chmielak, L. and Mujica Roncery, L. and Niederhofer, P. and Weber, S. and Theisen, W.
SN APPLIED SCIENCES. Volume: 3 (2021) - 2021 • 6968
Influence of low Bi contents on phase transformation properties of VO2studied in a VO2:Bi thin film library
Wang, X. and Rogalla, D. and Kostka, A. and Ludwig, Al.
RSC ADVANCES. Volume: 11 (2021) - 2021 • 6967
Orientation-dependent plastic deformation mechanisms and competition with stress-induced phase transformation in microscale NiTi
Choi, W.S. and Pang, E.L. and Ko, W.-S. and Jun, H. and Bong, H.J. and Kirchlechner, C. and Raabe, D. and Choi, P.-P.
ACTA MATERIALIA. Volume: 208 (2021) - 2021 • 6966
Closing the Gap for Electronic Short-Circuiting: Photosystem I Mixed Monolayers Enable Improved Anisotropic Electron Flow in Biophotovoltaic Devices
Wang, P. and Frank, A. and Zhao, F. and Szczesny, J. and Junqueira, J.R.C. and Zacarias, S. and Ruff, A. and Nowaczyk, M.M. and Pereira, I.A.C. and Rögner, M. and Conzuelo, F. and Schuhmann, W.
ANGEWANDTE CHEMIE - INTERNATIONAL EDITION. Volume: 60 (2021) - 2021 • 6965
Electronic theory for scanning tunneling microscopy spectra in infinite-layer nickelate superconductors
Choubey, P. and Eremin, I.M.
PHYSICAL REVIEW B. Volume: 104 (2021) - 2021 • 6964
Improved tribocorrosion resistance of the martensitic steel X54CrMoVN17-1 due to expanded martensite [Verbesserte Tribokorrosions-beständigkeit des martensitischen Stahls X54CrMoVN17-1 durch die Erzeugung von expanded martensite]
Hahn, I. and Siebert, S. and Theisen, W. and Weber, S.
TRIBOLOGIE UND SCHMIERUNGSTECHNIK. Volume: 68 (2021) - 2021 • 6963
Twin-boundary assisted crack tip plasticity and toughening in lamellar γ-TiAl
Neogi, A. and Janisch, R.
ACTA MATERIALIA. Volume: 213 (2021) - 2021 • 6962
Investigation on flow dynamics and temperatures of solid fuel particles in a gas-assisted oxy-fuel combustion chamber
Schneider, H. and Valentiner, S. and Vorobiev, N. and Böhm, B. and Schiemann, M. and Scherer, V. and Kneer, R. and Dreizler, A.
FUEL. Volume: 286 (2021) - 2021 • 6961
Highly Ordered Mesoporous Co3O4 Electrocatalyst for Efficient, Selective, and Stable Oxidation of 5-Hydroxymethylfurfural to 2,5-Furandicarboxylic Acid
Wang, C. and Bongard, H.-J. and Yu, M. and Schüth, F.
CHEMSUSCHEM. Volume: (2021) - 2021 • 6960
Software-in-the-loop optimization of actuator and sensor placement for a smart piezoelectric funnel-shaped inlet of a magnetic resonance imaging tomograph
Nestorović, T. and Hassw, K. and Oveisi, A.
MECHANICAL SYSTEMS AND SIGNAL PROCESSING. Volume: 147 (2021) - 2021 • 6959
Density-based grain boundary phase diagrams: Application to Fe-Mn-Cr, Fe-Mn-Ni, Fe-Mn-Co, Fe-Cr-Ni and Fe-Cr-Co alloy systems
Wang, L. and Darvishi Kamachali, R.
ACTA MATERIALIA. Volume: 207 (2021) - 2021 • 6958
Materials breaking the rules: General discussion
Addicoat, M. and Bennett, T.D. and Brammer, L. and Craig, G. and Das, C. and Dichtel, W. and Doan, H. and Evans, A.M. and Evans, J. and Goodwin, A. and Horike, S. and Jiang, J. and Kaskel, S. and Kato, M. and Kitagawa, S. and Kobayashi, A. and Krause, S. and Lavenn, C. and Lee, J.-S.M. and Phillips, A.E. and Roseveare, T.M. and Schmid, R. and Shivanna, M. and Sirbu, D. and Tashiro, S. and Ting, V.P. and Van Der Veen, M.A. and Wilson, B. and Zhao, P.
FARADAY DISCUSSIONS. Volume: 225 (2021) - 2021 • 6957
Time will tell: Secular change in metamorphic timescales and the tectonic implications
Chowdhury, P. and Chakraborty, S. and Gerya, T.V.
GONDWANA RESEARCH. Volume: 93 (2021) - 2021 • 6956
Discovery and Implications of Hidden Atomic-Scale Structure in a Metallic Meteorite
Kovács, A. and Lewis, L.H. and Palanisamy, D. and Denneulin, T. and Schwedt, A. and Scott, E.R.D. and Gault, B. and Raabe, D. and Dunin-Borkowski, R.E. and Charilaou, M.
NANO LETTERS. Volume: 21 (2021) - 2021 • 6955
Effect of cooling rate on the microstructure and mechanical properties of a low-carbon low-alloyed steel
Wang, H. and Cao, L. and Li, Y. and Schneider, M. and Detemple, E. and Eggeler, G.
JOURNAL OF MATERIALS SCIENCE. Volume: 56 (2021) - 2021 • 6954
Confocal laser scanning holographic microscopy of buried structures
Schnitzler, L. and Neutsch, K. and Schellenberg, F. and Hofmann, M.R. and Gerhardt, N.C.
APPLIED OPTICS. Volume: 60 (2021) - 2021 • 6953
Electroabsorption in gated GaAs nanophotonic waveguides
Wang, Y. and Uppu, R. and Zhou, X. and Papon, C. and Scholz, S. and Wieck, A.D. and Ludwig, Ar. and Lodahl, P. and Midolo, L.
APPLIED PHYSICS LETTERS. Volume: 118 (2021) - 2021 • 6952
Lensless digital holographic microscopy as an efficient method to monitor enzymatic plastic degradation
Schnitzler, L. and Zarzycki, J. and Gerhard, M. and Konde, S. and Rexer, K.-H. and Erb, T.J. and Maier, U.G. and Koch, M. and Hofmann, M.R. and Moog, D.
MARINE POLLUTION BULLETIN. Volume: 163 (2021) - 2021 • 6951
Incorporating elasticity into CALPHAD-informed density-based grain boundary phase diagrams reveals segregation transition in Al-Cu and Al-Cu-Mg alloys
Wang, L. and Darvishi Kamachali, R.
COMPUTATIONAL MATERIALS SCIENCE. Volume: 199 (2021) - 2021 • 6950
Atomic-scale modeling of superalloys
Hammerschmidt, T. and Rogal, J. and Bitzek, E. and Drautz, R.
NICKEL BASE SINGLE CRYSTALS ACROSS LENGTH SCALES. Volume: (2021) - 2021 • 6949
Electron capture and emission dynamics of self-assembled quantum dots far from equilibrium with the environment
Schnorr, L. and Labes, J. and Kürten, L. and Heinzel, T. and Rothfuchs-Engels, C. and Scholz, S. and Ludwig, A. and Wieck, A.D.
PHYSICAL REVIEW B. Volume: 104 (2021) - 2021 • 6948
Application of nanoindentation technique to test surface hardness and residual stress of NiTi alloy after femtosecond laser shock peening
Wang, H. and Gurevich, E.L. and Ostendorf, A.
PROCEEDINGS OF SPIE - THE INTERNATIONAL SOCIETY FOR OPTICAL ENGINEERING. Volume: 11679 (2021) - 2021 • 6947
Ultrastrong and Ductile Soft Magnetic High-Entropy Alloys via Coherent Ordered Nanoprecipitates
Han, L. and Rao, Z. and Souza Filho, I.R. and Maccari, F. and Wei, Y. and Wu, G. and Ahmadian, A. and Zhou, X. and Gutfleisch, O. and Ponge, D. and Raabe, D. and Li, Z.
ADVANCED MATERIALS. Volume: 33 (2021) - 2021 • 6946
Hysteretic capacitance-voltage characteristics of self-assembled quantum dots far from equilibrium with their environment
Schnorr, L. and Khoukhi, O. and Berg, L. and Heinzel, T. and Rothfuchs-Engels, C. and Scholz, S. and Ludwig, Ar. and Wieck, A.D.
PHYSICAL REVIEW B. Volume: 104 (2021) - 2021 • 6945
TEM replica analysis of particle phases in a tempered martensite ferritic Cr steel after long term creep
Wang, H. and Kostka, A. and Goosen, W.E. and Eggeler, G. and Westraadt, J.E.
MATERIALS CHARACTERIZATION. Volume: 181 (2021) - 2021 • 6944
Aluminum depletion induced by co-segregation of carbon and boron in a bcc-iron grain boundary
Ahmadian, A. and Scheiber, D. and Zhou, X. and Gault, B. and Liebscher, C.H. and Romaner, L. and Dehm, G.
NATURE COMMUNICATIONS. Volume: 12 (2021) - 2021 • 6943
CrOx-Mediated Performance Enhancement of Ni/NiO-Mg:SrTiO3in Photocatalytic Water Splitting
Han, K. and Haiber, D.M. and Knöppel, J. and Lievens, C. and Cherevko, S. and Crozier, P. and Mul, G. and Mei, B.
ACS CATALYSIS. Volume: 11 (2021) - 2021 • 6942
From digital models to numerical analysis for mechanised tunnelling: A fully automated design-through-analysis workflow
Ninic, J. and Alsahly, A. and Vonthron, A. and Bui, H.-G. and Koch, C. and König, M. and Meschke, G.
TUNNELLING AND UNDERGROUND SPACE TECHNOLOGY. Volume: 107 (2021) - 2021 • 6941
Segmentation of Static and Dynamic Atomic-Resolution Microscopy Data Sets with Unsupervised Machine Learning Using Local Symmetry Descriptors
Wang, N. and Freysoldt, C. and Zhang, S. and Liebscher, C.H. and Neugebauer, J.
MICROSCOPY AND MICROANALYSIS. Volume: (2021) - 2021 • 6940
Finite temperature fluctuation-induced order and responses in magnetic topological insulators
Scholten, M. and Facio, J.I. and Ray, R. and Eremin, I.M. and van den Brink, J. and Nogueira, F.S.
PHYSICAL REVIEW RESEARCH. Volume: 3 (2021) - 2021 • 6939
Automated image analysis for quantification of materials microstructure evolution
Ahmed, M. and Horst, O.M. and Obaied, A. and Steinbach, I. and Roslyakova, I.
MODELLING AND SIMULATION IN MATERIALS SCIENCE AND ENGINEERING. Volume: 29 (2021) - 2021 • 6938
Effects of shear flow on the structure and dynamics of ionic liquids in a metallic nanoconfinement
Ntim, S. and Sulpizi, M.
PHYSICAL CHEMISTRY CHEMICAL PHYSICS. Volume: 23 (2021) - 2021 • 6937
A 3d analysis of dendritic solidification and mosaicity in ni-based single crystal superalloys
Scholz, F. and Cevik, M. and Hallensleben, P. and Thome, P. and Eggeler, G. and Frenzel, J.
MATERIALS. Volume: 14 (2021) - 2021 • 6936
Control of electron velocity distributions at the wafer by tailored voltage waveforms in capacitively coupled plasmas to compensate surface charging in high-aspect ratio etch features
Hartmann, P. and Wang, L. and Nösges, K. and Berger, B. and Wilczek, S. and Brinkmann, R.P. and Mussenbrock, T. and Juhasz, Z. and Donkó, Z. and Derzsi, A. and Lee, E. and Schulze, J.
JOURNAL OF PHYSICS D: APPLIED PHYSICS. Volume: 54 (2021) - 2021 • 6935
On the Multipole Resonance Probe: Current Status of Research and Development
Oberrath, J. and Friedrichs, M. and Gong, J. and Oberberg, M. and Pohle, D. and Schulz, C. and Wang, C. and Awakowicz, P. and Brinkmann, R.P. and Lapke, M. and Mussenbrock, T. and Musch, T. and Rolfes, I.
IEEE TRANSACTIONS ON PLASMA SCIENCE. Volume: (2021) - 2021 • 6934
Compensation of sensor movements in short-range fmcw synthetic aperture radar algorithms
Schorlemer, J. and Schulz, C. and Pohl, N. and Rolfes, I. and Barowski, J.
IEEE TRANSACTIONS ON MICROWAVE THEORY AND TECHNIQUES. Volume: 69 (2021) - 2021 • 6933
Rational Design of a Photosystem I Photoanode for the Fabrication of Biophotovoltaic Devices
Wang, P. and Zhao, F. and Frank, A. and Zerria, S. and Lielpetere, A. and Ruff, A. and Nowaczyk, M.M. and Schuhmann, W. and Conzuelo, F.
ADVANCED ENERGY MATERIALS. Volume: (2021) - 2021 • 6932
Efficient frequency domain sampling schemes for THz SAR systems
Schorlemer, J. and Kolpatzeck, K. and Balzer, J.C. and Czylwik, A. and Rolfes, I. and Barowski, J.
2021 4TH INTERNATIONAL WORKSHOP ON MOBILE TERAHERTZ SYSTEMS, IWMTS 2021. Volume: (2021) - 2021 • 6931
Finite element modeling of brittle and ductile modes in cutting of 3c-sic
Alam, M. and Zhao, L. and Vajragupta, N. and Zhang, J. and Hartmaier, A.
CRYSTALS. Volume: 11 (2021) - 2021 • 6930
Beam divergence reduction of vortex waves with a tailored lens and a tailored reflector
Hassan, M.H. and Sievert, B. and Svejda, J.T. and Ahmed, A.M. and Barowski, J. and Rennings, A. and Rolfes, I. and Sezgin, A. and Erni, D.
IEEE ACCESS. Volume: 9 (2021) - 2021 • 6929
Importance of catalyst–photoabsorber interface design configuration on the performance of Mo-doped BiVO4 water splitting photoanodes
Krysiak, O.A. and Junqueira, J.R.C. and Conzuelo, F. and Bobrowski, T. and Masa, J. and Wysmolek, A. and Schuhmann, W.
JOURNAL OF SOLID STATE ELECTROCHEMISTRY. Volume: 25 (2021) - 2021 • 6928
Comparison of Short-Range SAR Imaging Algorithms for the Detection of Landmines using Numerical Simulations
Schorlemer, J. and Jebramcik, J. and Rolfes, I. and Barowski, J.
2021 18TH EUROPEAN RADAR CONFERENCE, EURAD 2021. Volume: (2021) - 2021 • 6927
Geometry and flow properties affect the phase shift between pressure and shear stress waves in blood vessels
Wang, H. and Krüger, T. and Varnik, F.
FLUIDS. Volume: 6 (2021) - 2021 • 6926
Searching novel complex solid solution electrocatalysts in unconventional element combinations
Krysiak, O.A. and Schumacher, S. and Savan, A. and Schuhmann, W. and Ludwig, A. and Andronescu, C.
NANO RESEARCH. Volume: (2021) - 2021 • 6925
Optimal designs for series estimation in nonparametric regression with correlated data
Schorning, K. and Konstantinou, M. and Dette, H.
STATISTICA SINICA. Volume: 31 (2021) - 2021 • 6924
On the Potential Self-Amplification of Aneurysms Due to Tissue Degradation and Blood Flow Revealed From FSI Simulations
Wang, H. and Balzani, D. and Vedula, V. and Uhlmann, K. and Varnik, F.
FRONTIERS IN PHYSIOLOGY. Volume: 12 (2021) - 2021 • 6923
Optimal Designs for Comparing Regression Curves: Dependence Within and Between Groups
Schorning, K. and Dette, H.
JOURNAL OF STATISTICAL THEORY AND PRACTICE. Volume: 15 (2021) - 2021 • 6922
Adjoint-based sensitivity analysis of char combustion surface reaction kinetics
Hassan, A. and Sayadi, T. and Schiemann, M. and Scherer, V.
FUEL. Volume: 287 (2021) - 2021 • 6921
Grain boundary energy landscape from the shape analysis of synthetically stabilized embedded grains
Schratt, A.A. and Steinbach, I. and Mohles, V.
COMPUTATIONAL MATERIALS SCIENCE. Volume: 193 (2021) - 2021 • 6920
Synthesis and Characterization of Dendritic and Linear Glycol Methacrylates and Their Performance as Marine Antifouling Coatings
Wanka, R. and Koschitzki, F. and Puzovic, V. and Pahl, T. and Manderfeld, E. and Hunsucker, K.Z. and Swain, G.W. and Rosenhahn, A.
ACS APPLIED MATERIALS AND INTERFACES. Volume: (2021) - 2021 • 6919
Stripping away ion hydration shells in electrical double-layer formation: Water networks matter
Alfarano, S.R. and Pezzotti, S. and Stein, C.J. and Lin, Z. and Sebastiani, F. and Funke, S. and Hoberg, C. and Kolling, I. and Ma, C.Y. and Mauelshagen, K. and Ockelmann, T. and Schwaab, G. and Fu, L. and Brubach, J.-B. and Roy, P. and Head-Gordon, M. and Tschulik, K. and Gaigeot, M.-P. and Havenith, M.
PROCEEDINGS OF THE NATIONAL ACADEMY OF SCIENCES OF THE UNITED STATES OF AMERICA. Volume: 118 (2021) - 2021 • 6918
Study on the Austemperability of Thin-wall Ductile Cast Iron Produced by High-Pressure Die-casting
van gen Hassend, F. and Ninnemann, L. and Töberich, F. and Breuckmann, M. and Röttger, A. and Weber, S.
JOURNAL OF MATERIALS ENGINEERING AND PERFORMANCE. Volume: (2021) - 2021 • 6917
Optimization of processing parameters of ultrashort (100 fs-2 ps) pulsed laser shock peening of stainless steel
Kukreja, L.M. and Hoppius, J.S. and Elango, K. and Macias Barrientos, M. and Pöhl, F. and Walther, F. and Gurevich, E. and Ostendorf, A.
JOURNAL OF LASER APPLICATIONS. Volume: 33 (2021) - 2021 • 6916
Optimal Designs for Model Averaging in non-nested Models
Alhorn, K. and Dette, H. and Schorning, K.
SANKHYA A. Volume: (2021) - 2021 • 6915
Effect of interface dislocations on mass flow during high temperature and low stress creep of single crystal Ni-base superalloys
He, J. and Cao, L. and Makineni, S.K. and Gault, B. and Eggeler, G.
SCRIPTA MATERIALIA. Volume: 191 (2021) - 2021 • 6914
Mineral-bonded composites for enhanced structural impact safety: Material level investigations [Impaktsicherheit von Baukonstruktionen durch mineralisch gebundene Komposite: Materialebene]
Curosu, I. and Mechtcherine, V. and Vo, D.M.P. and Sennewald, C. and Cherif, C. and Wölfel, E. and Scheffler, C. and Gong, T. and Heravi, A.A. and Tamsen, E. and Balzani, D. and Shehni, A. and Häußler-Combe, U. and Fuchs, A. and Kaliske, M. and Scope, C. and Günther, E.
BETON- UND STAHLBETONBAU. Volume: 116 (2021) - 2021 • 6913
Columnar Thermal Barrier Coatings Produced by Different Thermal Spray Processes
Kumar, N. and Gupta, M. and Mack, D.E. and Mauer, G. and Vaßen, R.
JOURNAL OF THERMAL SPRAY TECHNOLOGY. Volume: (2021) - 2021 • 6912
The Effect of Modeling Assumptions on the ECG in Monodomain and Bidomain Simulations
Ogiermann, D. and Balzani, D. and Perotti, L.E.
LECTURE NOTES IN COMPUTER SCIENCE (INCLUDING SUBSERIES LECTURE NOTES IN ARTIFICIAL INTELLIGENCE AND LECTURE NOTES IN BIOINFORMATICS). Volume: 12738 LNCS (2021) - 2021 • 6911
Optimizing the nickel boride layer thickness in a spectroelectrochemical ATR-FTIR thin-film flow cell applied in glycerol oxidation
Cychy, S. and Lechler, S. and Huang, Z. and Braun, M. and Brix, A.C. and Blümler, P. and Andronescu, C. and Schmid, F. and Schuhmann, W. and Muhler, M.
CHINESE JOURNAL OF CATALYSIS. Volume: 42 (2021) - 2021 • 6910
Pair-exchange diffusion model for multicomponent alloys revisited
Kundin, J. and Steinbach, I. and Abrahams, K. and Divinski, S.V.
MATERIALIA. Volume: 16 (2021) - 2021 • 6909
Machine-learning-enhanced time-of-flight mass spectrometry analysis
Wei, Y. and Varanasi, R.S. and Schwarz, T. and Gomell, L. and Zhao, H. and Larson, D.J. and Sun, B. and Liu, G. and Chen, H. and Raabe, D. and Gault, B.
PATTERNS. Volume: 2 (2021) - 2021 • 6908
A MIMO Radar System based on Fractal Antenna Arrays for Level Measurement Applications
Dahl, C. and Vogt, M. and Rolfes, I.
ADVANCES IN RADIO SCIENCE. Volume: 19 (2021) - 2021 • 6907
The effect of short silica fibers (0.3 μm 3.2 μm) on macrophages
Olejnik, M. and Breisch, M. and Sokolova, V. and Loza, K. and Prymak, O. and Rosenkranz, N. and Westphal, G. and Bünger, J. and Köller, M. and Sengstock, C. and Epple, M.
SCIENCE OF THE TOTAL ENVIRONMENT. Volume: 769 (2021) - 2021 • 6906
Highly Anisotropic Fluorine-Based Plasma Etching of Ultralow Expansion Glass
Weigel, C. and Phi, H.B. and Denissel, F.A. and Hoffmann, M. and Sinzinger, S. and Strehle, S.
ADVANCED ENGINEERING MATERIALS. Volume: (2021) - 2021 • 6905
RF Analysis of a Sub-GHz InP-Based 1550 nm Monolithic Mode-Locked Laser Chip
Ali Alloush, M. and Van Delden, M. and Bassal, A. and Kleemann, N. and Brenner, C. and Lo, M. and Augustin, L. and Guzman, R. and Musch, T. and Carpintero, G. and Hofmann, M.R.
IEEE PHOTONICS TECHNOLOGY LETTERS. Volume: (2021) - 2021 • 6904
Elemental segregation to lattice defects in the CrMnFeCoNi high-entropy alloy during high temperature exposures
Heczko, M. and Mazánová, V. and Gröger, R. and Záležák, T. and Hooshmand, M.S. and George, E.P. and Mills, M.J. and Dlouhý, A.
ACTA MATERIALIA. Volume: 208 (2021) - 2021 • 6903
Femtosecond pulse generation from external cavity diode laser based on self-mode-locking
Alloush, M.A. and Brenner, C. and Calò, C. and Hofmann, M.R.
OPTICS LETTERS. Volume: 46 (2021) - 2021 • 6902
Nanoparticle additivation effects on laser powder bed fusion of metals and polymers—a theoretical concept for an inter-laboratory study design all along the process chain, including research data management
Kusoglu, I.M. and Huber, F. and Doñate-Buendía, C. and Ziefuss, A.R. and Gökce, B. and Sehrt, J.T. and Kwade, A. and Schmidt, M. and Barcikowski, S.
MATERIALS. Volume: 14 (2021) - 2021 • 6901
Control of Marine Bacteria and Diatom Biofouling by Constant and Alternating Potentials
Schwarze, J. and Schuhmann, W. and Rosenhahn, A.
LANGMUIR. Volume: 37 (2021) - 2021 • 6900
RF Analysis of a Sub-GHz InP-Based 1550 nm Monolithic Mode-Locked Laser Chip
Alloush, M.A. and Van Delden, M. and Bassal, A. and Kleemann, N. and Brenner, C. and Lo, M.-C. and Augustin, L. and Guzman, R. and Musch, T. and Carpintero, G. and Hofmann, M.R.
IEEE PHOTONICS TECHNOLOGY LETTERS. Volume: 33 (2021) - 2021 • 6899
Dislocation networks in gamma/gamma’-microstructures formed during selective laser melting of a Ni-base superalloy
Heep, L. and Schwalbe, C. and Heinze, C. and Dlouhy, A. and Rae, C.M.F. and Eggeler, G.
SCRIPTA MATERIALIA. Volume: 190 (2021) - 2021 • 6898
Large-deformation crystal plasticity simulation of microstructure and microtexture evolution through adaptive remeshing
Sedighiani, K. and Shah, V. and Traka, K. and Diehl, M. and Roters, F. and Sietsma, J. and Raabe, D.
INTERNATIONAL JOURNAL OF PLASTICITY. Volume: 146 (2021) - 2021 • 6897
Gd-Ru Nanoparticles Supported on Zr0.5Ce0.5O2Nanorods for Dry Methane Reforming
Das, S. and Sengupta, M. and Bag, A. and Saini, A. and Muhler, M. and Bordoloi, A.
ACS APPLIED NANO MATERIALS. Volume: 4 (2021) - 2021 • 6896
Properties of α-Brass Nanoparticles II: Structure and Composition
Weinreich, J. and Paleico, M.L. and Behler, J.
JOURNAL OF PHYSICAL CHEMISTRY C. Volume: 125 (2021) - 2021 • 6895
Soil-Building Interaction and Risk Assessment of Existing Structures During Mechanized Tunneling
Alsahly, A. and Marwan, A. and Obel, M. and Mark, P. and Meschke, G.
LECTURE NOTES IN CIVIL ENGINEERING. Volume: 126 (2021) - 2021 • 6894
Experimental determination of walnut shell pyrolysis kinetics in N2 and CO2 via thermogravimetric analysis, fluidized bed and drop tube reactors
Ontyd, C. and Pielsticker, S. and Yildiz, C. and Schiemann, M. and Hatzfeld, O. and Ströhle, J. and Epple, B. and Kneer, R. and Scherer, V.
FUEL. Volume: 287 (2021) - 2021 • 6893
Experimental analysis of the flight design effect on the temperature distribution in rotary kilns
Seidenbecher, J. and Herz, F. and Meitzner, C. and Specht, E. and Wirtz, S. and Scherer, V. and Liu, X.
CHEMICAL ENGINEERING SCIENCE. Volume: 240 (2021) - 2021 • 6892
Synergistic Electrocatalytic Hydrogen Evolution in Ni/NiS Nanoparticles Wrapped in Multi-Heteroatom-Doped Reduced Graphene Oxide Nanosheets
Hegazy, M.B.Z. and Berber, M.R. and Yamauchi, Y. and Pakdel, A. and Cao, R. and Apfel, U.-P.
ACS APPLIED MATERIALS AND INTERFACES. Volume: 13 (2021) - 2021 • 6891
Temperature analysis in flighted rotary drums and the influence of operating parameters
Seidenbecher, J. and Herz, F. and Meitzner, C. and Specht, E. and Wirtz, S. and Scherer, V. and Liu, X.
CHEMICAL ENGINEERING SCIENCE. Volume: 229 (2021) - 2021 • 6890
Mechanochemical Synthesis of Supported Bimetallic Catalysts
De Bellis, J. and Felderhoff, M. and Schüth, F.
CHEMISTRY OF MATERIALS. Volume: 33 (2021) - 2021 • 6889
Impact of magnetic transition on Mn diffusion in α -iron: Correlative state-of-the-art theoretical and experimental study
Hegde, O. and Kulitckii, V. and Schneider, A. and Soisson, F. and Hickel, T. and Neugebauer, J. and Wilde, G. and Divinski, S. and Fu, C.-C.
PHYSICAL REVIEW B. Volume: 104 (2021) - 2021 • 6888
Additive manufacturing of PA12 carbon nanotube composites with a novel laser polymer deposition process
Wencke, Y.L. and Kutlu, Y. and Seefeldt, M. and Esen, C. and Ostendorf, A. and Luinstra, G.A.
JOURNAL OF APPLIED POLYMER SCIENCE. Volume: 138 (2021) - 2021 • 6887
Tailoring the Electrocatalytic Activity of Pentlandite FexNi9-XS8 Nanoparticles via Variation of the Fe : Ni Ratio for Enhanced Water Oxidation
Amin, H.M.A. and Attia, M. and Tetzlaff, D. and Apfel, U.-P.
CHEMELECTROCHEM. Volume: 8 (2021) - 2021 • 6886
Calibration and validation of a comprehensive kinetic model of coal conversion in inert, air and oxy-fuel conditions using data from multiple test rigs
Debiagi, P. and Ontyd, C. and Pielsticker, S. and Schiemann, M. and Faravelli, T. and Kneer, R. and Hasse, C. and Scherer, V.
FUEL. Volume: 290 (2021) - 2021 • 6885
Selective Electrochemical Oxidation of H2O to H2O2Using Boron-Doped Diamond: An Experimental and Techno-Economic Evaluation
Wenderich, K. and Nieuweweme, B.A.M. and Mul, G. and Mei, B.T.
ACS SUSTAINABLE CHEMISTRY AND ENGINEERING. Volume: 9 (2021) - 2021 • 6884
Mechanochemical Synthesis of Catalytic Materials
Amrute, A.P. and De Bellis, J. and Felderhoff, M. and Schüth, F.
CHEMISTRY - A EUROPEAN JOURNAL. Volume: (2021) - 2021 • 6883
Revisiting ω phase embrittlement in metastable β titanium alloys: Role of elemental partitioning
Lai, M.J. and Li, T. and Yan, F.K. and Li, J.S. and Raabe, D.
SCRIPTA MATERIALIA. Volume: 193 (2021) - 2021 • 6882
Catalytic reactions in ball mills
Amrute, A.P. and Schüth, F.
CATALYSIS. Volume: 33 (2021) - 2021 • 6881
Dielectric Properties of Nanoconfined Water: A Canonical Thermopotentiostat Approach
Deißenbeck, F. and Freysoldt, C. and Todorova, M. and Neugebauer, J. and Wippermann, S.
PHYSICAL REVIEW LETTERS. Volume: 126 (2021) - 2021 • 6880
A similarity measure for second order properties of non-stationary functional time series with applications to clustering and testing
van Delft, A. and Dette, H.
BERNOULLI. Volume: 27 (2021) - 2021 • 6879
In situ correlation between metastable phase-transformation mechanism and kinetics in a metallic glass
Orava, J. and Balachandran, S. and Han, X. and Shuleshova, O. and Nurouzi, E. and Soldatov, I. and Oswald, S. and Gutowski, O. and Ivashko, O. and Dippel, A.-C. and Zimmermann, M. and Ivanov, Y.P. and Greer, A.L. and Raabe, D. and Herbig, M. and Kaban, I.
NATURE COMMUNICATIONS. Volume: 12 (2021) - 2021 • 6878
Electrocatalysis in confined space
Andronescu, C. and Masa, J. and Tilley, R.D. and Gooding, J.J. and Schuhmann, W.
CURRENT OPINION IN ELECTROCHEMISTRY. Volume: 25 (2021) - 2021 • 6877
A nonparametric test for stationarity in functional time series
van Delft, A. and Characiejus, V. and Dette, H.
STATISTICA SINICA. Volume: 31 (2021) - 2021 • 6876
Emerging symmetric strain response and weakening nematic fluctuations in strongly hole-doped iron-based superconductors
Wiecki, P. and Frachet, M. and Haghighirad, A.-A. and Wolf, T. and Meingast, C. and Heid, R. and Böhmer, A.E.
NATURE COMMUNICATIONS. Volume: 12 (2021) - 2021 • 6875
A distribution free test for changes in the trend function of locally stationary processes∗
Heinrichs, F. and Dette, H.
ELECTRONIC JOURNAL OF STATISTICS. Volume: 15 (2021) - 2021 • 6874
Constitutive modeling of cyclic plasticity at elevated temperatures for a nickel-based superalloy
Shahmardani, M. and Hartmaier, A.
INTERNATIONAL JOURNAL OF FATIGUE. Volume: 151 (2021) - 2021 • 6873
Edwards-Anderson parameter and local Ising nematicity in FeSe revealed via NMR spectral broadening
Wiecki, P. and Zhou, R. and Julien, M.-H. and Böhmer, A.E. and Schmalian, J.
PHYSICAL REVIEW B. Volume: 104 (2021) - 2021 • 6872
Nucleation and growth of α phase in a metastable β-Titanium Ti-5Al-5Mo-5V-3Cr alloy: Influence from the nano-scale, ordered-orthorhombic O″ phase and α compositional evolution
Antonov, S. and Shi, R. and Li, D. and Kloenne, Z. and Zheng, Y. and Fraser, H.L. and Raabe, D. and Gault, B.
SCRIPTA MATERIALIA. Volume: 194 (2021) - 2021 • 6871
Site-selective protonation of the one-electron reduced cofactor in [FeFe]-hydrogenase
Laun, K. and Baranova, I. and Duan, J. and Kertess, L. and Wittkamp, F. and Apfel, U.-P. and Happe, T. and Senger, M. and Stripp, S.T.
DALTON TRANSACTIONS. Volume: 50 (2021) - 2021 • 6870
Influence of crystal plasticity parameters on the strain hardening behavior of polycrystals
Shahmardani, M. and Vajragupta, N. and Hartmaier, A.
CRYSTALS. Volume: 11 (2021) - 2021 • 6869
A note on optimal designs for estimating the slope of a polynomial regression
Dette, H. and Melas, V.B. and Shpilev, P.
STATISTICS AND PROBABILITY LETTERS. Volume: 170 (2021) - 2021 • 6868
Comparative study of hydrogen embrittlement resistance between additively and conventionally manufactured 304L austenitic stainless steels
Lee, D.-H. and Sun, B. and Lee, S. and Ponge, D. and Jägle, E.A. and Raabe, D.
MATERIALS SCIENCE AND ENGINEERING A. Volume: 803 (2021) - 2021 • 6867
Recrystallization kinetics, mechanisms, and topology in alloys processed by laser powder-bed fusion: AISI 316L stainless steel as example
Aota, L.S. and Bajaj, P. and Zilnyk, K.D. and Jägle, E.A. and Ponge, D. and Sandim, H.R.Z. and Raabe, D.
MATERIALIA. Volume: 20 (2021) - 2021 • 6866
Identifying shifts between two regression curves
Dette, H. and Dhar, S.S. and Wu, W.
ANNALS OF THE INSTITUTE OF STATISTICAL MATHEMATICS. Volume: (2021) - 2021 • 6865
Structure and hardness of in situ synthesized nano-oxide strengthened CoCrFeNi high entropy alloy thin films
Lee, S. and Chatain, D. and Liebscher, C.H. and Dehm, G.
SCRIPTA MATERIALIA. Volume: 203 (2021) - 2021 • 6864
Coherent Spin-Photon Interface with Waveguide Induced Cycling Transitions
Appel, M.H. and Tiranov, A. and Javadi, A. and Löbl, M.C. and Wang, Y. and Scholz, S. and Wieck, A.D. and Ludwig, Ar. and Warburton, R.J. and Lodahl, P.
PHYSICAL REVIEW LETTERS. Volume: 126 (2021) - 2021 • 6863
Bio-equivalence tests in functional data by maximum deviation
Dette, H. and Kokot, K.
BIOMETRIKA. Volume: 108 (2021) - 2021 • 6862
Detecting relevant differences in the covariance operators of functional time series: a sup-norm approach
Dette, H. and Kokot, K.
ANNALS OF THE INSTITUTE OF STATISTICAL MATHEMATICS. Volume: (2021) - 2021 • 6861
Evidence for a large Rashba splitting in PtPb4 from angle-resolved photoemission spectroscopy
Lee, K. and Mou, D. and Jo, N.H. and Wu, Y. and Schrunk, B. and Wilde, J.M. and Kreyssig, A. and Estry, A. and Bud'Ko, S.L. and Nguyen, M.C. and Wang, L.-L. and Wang, C.-Z. and Ho, K.-M. and Canfield, P.C. and Kaminski, A.
PHYSICAL REVIEW B. Volume: 103 (2021) - 2021 • 6860
Detecting structural breaks in eigensystems of functional time series
Dette, H. and Kutta, T.
ELECTRONIC JOURNAL OF STATISTICS. Volume: 15 (2021) - 2021 • 6859
Investigation of the frequency dependent spatio-temporal dynamics and controllability of microdischarges in unipolar pulsed plasma electrolytic oxidation
Hermanns, P. and Boeddeker, S. and Bracht, V. and Bibinov, N. and Grundmeier, G. and Awakowicz, P.
JOURNAL OF PHYSICS D: APPLIED PHYSICS. Volume: 54 (2021) - 2021 • 6858
Experimental Reconstruction of the Few-Photon Nonlinear Scattering Matrix from a Single Quantum Dot in a Nanophotonic Waveguide
Le Jeannic, H. and Ramos, T. and Simonsen, S.F. and Pregnolato, T. and Liu, Z. and Schott, R. and Wieck, A.D. and Ludwig, Ar. and Rotenberg, N. and García-Ripoll, J.J. and Lodahl, P.
PHYSICAL REVIEW LETTERS. Volume: 126 (2021) - 2021 • 6857
Multi-atom quasiparticle scattering interference for superconductor energy-gap symmetry determination
Sharma, R. and Kreisel, A. and Sulangi, M.A. and Böker, J. and Kostin, A. and Allan, M.P. and Eisaki, H. and Böhmer, A.E. and Canfield, P.C. and Eremin, I. and Séamus Davis, J.C. and Hirschfeld, P.J. and Sprau, P.O.
NPJ QUANTUM MATERIALS. Volume: 6 (2021) - 2021 • 6856
Correcting Intraday Periodicity Bias in Realized Volatility Measures
Dette, H. and Golosnoy, V. and Kellermann, J.
ECONOMETRICS AND STATISTICS. Volume: (2021) - 2021 • 6855
Identifying the Bottleneck for Heat Transport in Metal–Organic Frameworks
Wieser, S. and Kamencek, T. and Dürholt, J.P. and Schmid, R. and Bedoya-Martínez, N. and Zojer, E.
ADVANCED THEORY AND SIMULATIONS. Volume: 4 (2021) - 2021 • 6854
A bioinspired oxoiron(iv) motif supported on a N2S2macrocyclic ligand
Deutscher, J. and Gerschel, P. and Warm, K. and Kuhlmann, U. and Mebs, S. and Haumann, M. and Dau, H. and Hildebrandt, P. and Apfel, U.-P. and Ray, K.
CHEMICAL COMMUNICATIONS. Volume: 57 (2021) - 2021 • 6853
Laser metal deposition of Al0.6CoCrFeNi with Ti & C additions using elemental powder blends
Asabre, A. and Wilms, M.B. and Kostka, A. and Gemagami, P. and Weisheit, A. and Laplanche, G.
SURFACE AND COATINGS TECHNOLOGY. Volume: 418 (2021) - 2021 • 6852
Influence of substrates and e-beam evaporation parameters on the microstructure of nanocrystalline and epitaxially grown Ti thin films
Devulapalli, V. and Bishara, H. and Ghidelli, M. and Dehm, G. and Liebscher, C.H.
APPLIED SURFACE SCIENCE. Volume: 562 (2021) - 2021 • 6851
Tissue differentiation using optical emission spectroscopy for gastric mucosal devitalisation
Hillebrand, B. and Jurjut, O. and Schuhmann, T. and Schürmann, M. and Neugebauer, A. and Kemen, M. and Awakowicz, P. and Enderle, M.
JOURNAL OF PHYSICS D: APPLIED PHYSICS. Volume: 54 (2021) - 2021 • 6850
Rational Designed Hybrid Peptides Show up to a 6-Fold Increase in Antimicrobial Activity and Demonstrate Different Ultrastructural Changes as the Parental Peptides Measured by BioSAXS
Hilpert, K. and Gani, J. and Rumancev, C. and Simpson, N. and Lopez-Perez, P.M. and Garamus, V.M. and von Gundlach, A.R. and Markov, P. and Scocchi, M. and Mikut, R. and Rosenhahn, A.
FRONTIERS IN PHARMACOLOGY. Volume: 12 (2021) - 2021 • 6849
Characterisation of an artesian groundwater system in the Valle de Iglesia in the Central Andes of Argentina
Hinzer, I. and Altherr, M. and Christiansen, R. and Schreuer, J. and Wohnlich, S.
INTERNATIONAL JOURNAL OF EARTH SCIENCES. Volume: (2021) - 2021 • 6848
Scattering and Roughness Analysis of Indoor Materials at Frequencies from 750 GHz to 1.1 THz
Sheikh, F. and Zantah, Y. and Mabrouk, I.B. and Alissa, M. and Barowski, J. and Rolfes, I. and Kaiser, T.
IEEE TRANSACTIONS ON ANTENNAS AND PROPAGATION. Volume: (2021) - 2021 • 6847
Is Cu instability during the CO2reduction reaction governed by the applied potential or the local CO concentration?
Wilde, P. and O'Mara, P.B. and Junqueira, J.R.C. and Tarnev, T. and Benedetti, T.M. and Andronescu, C. and Chen, Y.-T. and Tilley, R.D. and Schuhmann, W. and Gooding, J.J.
CHEMICAL SCIENCE. Volume: 12 (2021) - 2021 • 6846
On the relation of structural disorder and thermoelastic properties in ZnGa2O4 and Zn1−xMgxGa2O4 (x ≈ 0.33)
Hirschle, C. and Schreuer, J. and Galazka, Z. and Ritter, C.
JOURNAL OF ALLOYS AND COMPOUNDS. Volume: 886 (2021) - 2021 • 6845
Interplay of structural and dynamical heterogeneity in the nucleation mechanism in nickel
Díaz Leines, G. and Michaelides, A. and Rogal, J.
FARADAY DISCUSSIONS. Volume: 235 (2021) - 2021 • 6844
Online self tuning of parameters of a PID controller that uses a radial basis neural network
Pal, A.K. and Nestorovic, T.
INTERNATIONAL CONFERENCE ON ELECTRICAL, COMPUTER, COMMUNICATIONS AND MECHATRONICS ENGINEERING, ICECCME 2021. Volume: (2021) - 2021 • 6843
Artificial Intelligence Neural Network Approach to Self Tuning of a Discrete-Time PID Control System
Pal, A.K. and Nestorovic, T.
2021 9TH INTERNATIONAL CONFERENCE ON SYSTEMS AND CONTROL, ICSC 2021. Volume: (2021) - 2021 • 6842
A safety cap protects hydrogenase from oxygen attack
Winkler, M. and Duan, J. and Rutz, A. and Felbek, C. and Scholtysek, L. and Lampret, O. and Jaenecke, J. and Apfel, U.-P. and Gilardi, G. and Valetti, F. and Fourmond, V. and Hofmann, E. and Léger, C. and Happe, T.
NATURE COMMUNICATIONS. Volume: 12 (2021) - 2021 • 6841
Ultra-Shallow All-Epitaxial Aluminum Gate GaAs/AlxGa1−xAs Transistors with High Electron Mobility
Ashlea Alava, Y. and Wang, D.Q. and Chen, C. and Ritchie, D.A. and Ludwig, A. and Ritzmann, J. and Wieck, A.D. and Klochan, O. and Hamilton, A.R.
ADVANCED FUNCTIONAL MATERIALS. Volume: (2021) - 2021 • 6840
Pseudocapacitive Redox Polymers as Battery Materials: A Proof-of-Concept All-Polymer Aqueous Battery
Dieckhöfer, S. and Medina, D. and Ruff, A. and Conzuelo, F. and Schuhmann, W.
CHEMELECTROCHEM. Volume: 8 (2021) - 2021 • 6839
Influence of crystalline defects on magnetic nanodomains in a rare-earth-free magnetocrystalline anisotropic alloy
Palanisamy, D. and Kovács, A. and Hegde, O. and Dunin-Borkowski, R.E. and Raabe, D. and Hickel, T. and Gault, B.
PHYSICAL REVIEW MATERIALS. Volume: 5 (2021) - 2021 • 6838
Scattering and roughness analysis of indoor materials at frequencies from 750 GHz to 1.1 THz
Sheikh, F. and Zantah, Y. and Ben Mabrouk, I. and Alissa, M. and Barowski, J. and Rolfes, I. and Kaiser, T.
IEEE TRANSACTIONS ON ANTENNAS AND PROPAGATION. Volume: 69 (2021) - 2021 • 6837
Probing the Local Reaction Environment During High Turnover Carbon Dioxide Reduction with Ag-Based Gas Diffusion Electrodes
Dieckhöfer, S. and Öhl, D. and Junqueira, J.R.C. and Quast, T. and Turek, T. and Schuhmann, W.
CHEMISTRY - A EUROPEAN JOURNAL. Volume: 27 (2021) - 2021 • 6836
A bin and hash method for analyzing reference data and descriptors in machine learning potentials
Paleico, M.L. and Behler, J.
MACHINE LEARNING: SCIENCE AND TECHNOLOGY. Volume: 2 (2021) - 2021 • 6835
Accelerated Electrochemical Investigation of Li Plating Efficiency as Key Parameter for Li Metal Batteries Utilizing a Scanning Droplet Cell
Dieckhöfer, S. and Schuhmann, W. and Ventosa, E.
CHEMELECTROCHEM. Volume: 8 (2021) - 2021 • 6834
Investigation of an atomic-layer-deposited Al2O3 diffusion barrier between Pt and Si for the use in atomic scale atom probe tomography studies on a combinatorial processing platform
Li, Y. and Zanders, D. and Meischein, M. and Devi, A. and Ludwig, A.
SURFACE AND INTERFACE ANALYSIS. Volume: 53 (2021) - 2021 • 6833
Frustrated flexibility in metal-organic frameworks
Pallach, R. and Keupp, J. and Terlinden, K. and Frentzel-Beyme, L. and Kloß, M. and Machalica, A. and Kotschy, J. and Vasa, S.K. and Chater, P.A. and Sternemann, C. and Wharmby, M.T. and Linser, R. and Schmid, R. and Henke, S.
NATURE COMMUNICATIONS. Volume: 12 (2021) - 2021 • 6832
The influence of post-weld tempering temperatures on microstructure and strength in the stir zone of friction stir welded reduced activation ferritic/martensitic steel
Li, S. and Yang, X. and Vajragupta, N. and Tang, W. and Hartmaier, A. and Li, H.
MATERIALS SCIENCE AND ENGINEERING A. Volume: 814 (2021) - 2021 • 6831
Electroconvective Instability in Water Electrolysis: An Evaluation of Electroconvective Patterns and Their Onset Features
Pande, N. and Wood, J.A. and Mul, G. and Lohse, D. and Mei, B.T. and Krug, D.
PHYSICAL REVIEW APPLIED. Volume: 16 (2021) - 2021 • 6830
State-of-the-art progress in the selective photo-oxidation of alcohols
Shen, Z. and Hu, Y. and Li, B. and Zou, Y. and Li, S. and Wilma Busser, G. and Wang, X. and Zhao, G. and Muhler, M.
JOURNAL OF ENERGY CHEMISTRY. Volume: 62 (2021) - 2021 • 6829
Computational generation of virtual concrete mesostructures
Holla, V. and Vu, G. and Timothy, J.J. and Diewald, F. and Gehlen, C. and Meschke, G.
MATERIALS. Volume: 14 (2021) - 2021 • 6828
Control-oriented plasma modeling and controller design for reactive sputtering
Woelfel, C. and Oberberg, M. and Berger, B. and Engel, D. and Brinkmann, R.P. and Schulze, J. and Awakowicz, P. and Lunze, J.
IFAC JOURNAL OF SYSTEMS AND CONTROL. Volume: 16 (2021) - 2021 • 6827
Atomic scale understanding of phase stability and decomposition of a nanocrystalline CrMnFeCoNi Cantor alloy
Li, Y.J. and Savan, A. and Ludwig, A.
APPLIED PHYSICS LETTERS. Volume: 119 (2021) - 2021 • 6826
Improved process efficiency in laser-based powder bed fusion of nanoparticle coated maraging tool steel powder
Pannitz, O. and Großwendt, F. and Lüddecke, A. and Kwade, A. and Röttger, A. and Sehrt, J.T.
MATERIALS. Volume: 14 (2021) - 2021 • 6825
Nanoparticle impact electrochemistry
Azimzadeh Sani, M. and Tschulik, K.
FRONTIERS OF NANOSCIENCE. Volume: 18 (2021) - 2021 • 6824
Investigation of the in situ thermal conductivity and absorption behavior of nanocomposite powder materials in laser powder bed fusion processes
Pannitz, O. and Lüddecke, A. and Kwade, A. and Sehrt, J.T.
MATERIALS AND DESIGN. Volume: 201 (2021) - 2021 • 6823
Hierarchical crack buffering triples ductility in eutectic herringbone high-entropy alloys
Shi, P. and Li, R. and Li, Y. and Wen, Y. and Zhong, Y. and Ren, W. and Shen, Z. and Zheng, T. and Peng, J. and Liang, X. and Hu, P. and Min, N. and Zhang, Y. and Ren, Y. and Liaw, P.K. and Raabe, D. and Wang, Y.-D.
SCIENCE. Volume: 373 (2021) - 2021 • 6822
Thermoelastic properties and γ’-solvus temperatures of single-crystal Ni-base superalloys
Horst, O.M. and Schmitz, D. and Schreuer, J. and Git, P. and Wang, H. and Körner, C. and Eggeler, G.
JOURNAL OF MATERIALS SCIENCE. Volume: 56 (2021) - 2021 • 6821
Metal–Organic-Framework-Supported Molecular Electrocatalysis for the Oxygen Reduction Reaction
Liang, Z. and Guo, H. and Zhou, G. and Guo, K. and Wang, B. and Lei, H. and Zhang, W. and Zheng, H. and Apfel, U.-P. and Cao, R.
ANGEWANDTE CHEMIE - INTERNATIONAL EDITION. Volume: 60 (2021) - 2021 • 6820
Determining interface fracture toughness in multi layered environmental barrier coatings with laser textured silicon bond coat
Wolf, M. and Kakisawa, H. and Süß, F. and Mack, D.E. and Vaßen, R.
COATINGS. Volume: 11 (2021) - 2021 • 6819
Charge tunable gaas quantum dots in a photonic n-i-p diode
Babin, H.G. and Ritzmann, J. and Bart, N. and Schmidt, M. and Kruck, T. and Zhai, L. and Löbl, M.C. and Nguyen, G.N. and Spinnler, C. and Ranasinghe, L. and Warburton, R.J. and Heyn, C. and Wieck, A.D. and Ludwig, Ar .
NANOMATERIALS. Volume: 11 (2021) - 2021 • 6818
An assessment of the structural resolution of various fingerprints commonly used in machine learning
Parsaeifard, B. and Sankar De, D. and Christensen, A.S. and Faber, F.A. and Kocer, E. and De, S. and Behler, J. and von Lilienfeld, O.A. and Goedecker, S.
MACHINE LEARNING: SCIENCE AND TECHNOLOGY. Volume: 2 (2021) - 2021 • 6817
Gate voltage dependence of noise distribution in radio-frequency reflectometry in gallium arsenide quantum dots
Shinozaki, M. and Muto, Y. and Kitada, T. and Nakajima, T. and Delbecq, M.R. and Yoneda, J. and Takeda, K. and Noiri, A. and Ito, T. and Ludwig, Ar. and Wieck, A.D. and Tarucha, S. and Otsuka, T.
APPLIED PHYSICS EXPRESS. Volume: 14 (2021) - 2021 • 6816
Laboratory-Scale Processing and Performance Assessment of Ti–Ta High-Temperature Shape Memory Spring Actuators
Paulsen, A. and Dumlu, H. and Piorunek, D. and Langenkämper, D. and Frenzel, J. and Eggeler, G.
SHAPE MEMORY AND SUPERELASTICITY. Volume: 7 (2021) - 2021 • 6815
Crystalline ytterbium disilicate environmental barrier coatings made by high velocity oxygen fuel spraying
Wolf, M. and Mack, D.E. and Mauer, G. and Guillon, O. and Vaßen, R.
INTERNATIONAL JOURNAL OF APPLIED CERAMIC TECHNOLOGY. Volume: (2021) - 2021 • 6814
Synthesis, sintering, and effect of surface roughness on oxidation of submicron Ti2AlC ceramics
Badie, S. and Dash, A. and Sohn, Y.J. and Vaßen, R. and Guillon, O. and Gonzalez-Julian, J.
JOURNAL OF THE AMERICAN CERAMIC SOCIETY. Volume: 104 (2021) - 2021 • 6813
Combinatorial exploration of B2/L21 precipitation strengthened AlCrFeNiTi compositionally complex alloys
Wolff-Goodrich, S. and Marshal, A. and Pradeep, K.G. and Dehm, G. and Schneider, J.M. and Liebscher, C.H.
JOURNAL OF ALLOYS AND COMPOUNDS. Volume: 853 (2021) - 2021 • 6812
Mechanism for breakaway oxidation of the Ti2AlC MAX phase
Badie, S. and Sebold, D. and Vaßen, R. and Guillon, O. and Gonzalez-Julian, J.
ACTA MATERIALIA. Volume: 215 (2021) - 2021 • 6811
Partitioning of Solutes at Crystal Defects in Borides After Creep and Annealing in a Polycrystalline Superalloy
Lilensten, L. and Kostka, A. and Lartigue-Korinek, S. and Gault, B. and Tin, S. and Antonov, S. and Kontis, P.
JOM. Volume: (2021) - 2021 • 6810
Sensing and electrocatalytic activity of tungsten disulphide thin films fabricated via metal-organic chemical vapour deposition
Wree, J.-L. and Glauber, J.-P. and Öhl, D. and Niesen, A. and Kostka, A. and Rogalla, D. and Schuhmann, W. and Devi, A.
JOURNAL OF MATERIALS CHEMISTRY C. Volume: 9 (2021) - 2021 • 6809
Injection molding and near-complete densification of monolithic and al2o3 fiber-reinforced ti2alc max phase composites
Badie, S. and Gabriel, R. and Sebold, D. and Vaßen, R. and Guillon, O. and Gonzalez-Julian, J.
MATERIALS. Volume: 14 (2021) - 2021 • 6808
The Role of Nitrogen-doping in the Catalytic Transfer Hydrogenation of Phenol to Cyclohexanone with Formic Acid over Pd supported on Carbon Nanotubes
Hu, B. and Li, X. and Busser, W. and Schmidt, S. and Xia, W. and Li, G. and Li, X. and Peng, B.
CHEMISTRY - A EUROPEAN JOURNAL. Volume: 27 (2021) - 2021 • 6807
Experimental validation of formula for calculation thermal diffusivity in superlattices performed using a combination of two frequency-domain methods: Photothermal infrared radiometry and thermoreflectance
Pawlak, M. and Kruck, T. and Spitzer, N. and Dziczek, D. and Ludwig, Ar. and Wieck, A.D.
APPLIED SCIENCES (SWITZERLAND). Volume: 11 (2021) - 2021 • 6806
MD simulation study on defect evolution and doping efficiency of p-type doping of 3C-SiC by Al ion implantation with subsequent annealing
Wu, J. and Xu, Z. and Liu, L. and Hartmaier, A. and Rommel, M. and Nordlund, K. and Wang, T. and Janisch, R. and Zhao, J.
JOURNAL OF MATERIALS CHEMISTRY C. Volume: 9 (2021) - 2021 • 6805
Solvent Effects on Photocatalytic Anaerobic Oxidation of Benzyl Alcohol over Pt-Loaded Defective SrTiO3Nanoparticles
Hu, Y. and Shen, Z. and Li, B. and Li, S. and Yue, J. and Zhao, G. and Muhler, M. and Wang, X.
ACS APPLIED NANO MATERIALS. Volume: 4 (2021) - 2021 • 6804
Wquantitative shape-classification of misfitting precipitates during cubic to tetragonal transformations: Phase-field simulations and experiments
Lin, Y.-Y. and Schleifer, F. and Holzinger, M. and Ta, N. and Skrotzki, B. and Kamachali, R.D. and Glatzel, U. and Fleck, M.
MATERIALS. Volume: 14 (2021) - 2021 • 6803
Rapid Interchangeable Hydrogen, Hydride, and Proton Species at the Interface of Transition Metal Atom on Oxide Surface
Wu, S. and Tseng, K.-Y. and Kato, R. and Wu, T.-S. and Large, A. and Peng, Y.-K. and Xiang, W. and Fang, H. and Mo, J. and Wilkinson, I. and Soo, Y.-L. and Held, G. and Suenaga, K. and Li, T. and Chen, H.-Y.T. and Tsang, S.C.E.
JOURNAL OF THE AMERICAN CHEMICAL SOCIETY. Volume: 143 (2021) - 2021 • 6802
Mechanism of amorphous phase stabilization in ultrathin films of monoatomic phase change material
Dragoni, D. and Behler, J. and Bernasconi, M.
NANOSCALE. Volume: 13 (2021) - 2021 • 6801
Role of pH in the synthesis and growth of gold nanoparticles using L-asparagine: A combined experimental and simulation study
Baez-Cruz, R. and Baptista, L.A. and Ntim, S. and Manidurai, P. and Espinoza, S. and Ramanan, C. and Cortes-Huerto, R. and Sulpizi, M.
JOURNAL OF PHYSICS CONDENSED MATTER. Volume: 33 (2021) - 2021 • 6800
The role of combustion science and technology in low and zero impact energy transformation processes
Dreizler, A. and Pitsch, H. and Scherer, V. and Schulz, C. and Janicka, J.
APPLICATIONS IN ENERGY AND COMBUSTION SCIENCE. Volume: 7 (2021) - 2021 • 6799
Symbiotic crystal-glass alloys via dynamic chemical partitioning
Wu, G. and Liu, C. and Brognara, A. and Ghidelli, M. and Bao, Y. and Liu, S. and Wu, X. and Xia, W. and Zhao, H. and Rao, J. and Ponge, D. and Devulapalli, V. and Lu, W. and Dehm, G. and Raabe, D. and Li, Z.
MATERIALS TODAY. Volume: (2021) - 2021 • 6798
Design Strategies for Electrocatalysts from an Electrochemist's Perspective
Linnemann, J. and Kanokkanchana, K. and Tschulik, K.
ACS CATALYSIS. Volume: 11 (2021) - 2021 • 6797
Bayesian Optimization of High-Entropy Alloy Compositions for Electrocatalytic Oxygen Reduction**
Pedersen, J.K. and Clausen, C.M. and Krysiak, O.A. and Xiao, B. and Batchelor, T.A.A. and Löffler, T. and Mints, V.A. and Banko, L. and Arenz, M. and Savan, A. and Schuhmann, W. and Ludwig, Al. and Rossmeisl, J.
ANGEWANDTE CHEMIE - INTERNATIONAL EDITION. Volume: 60 (2021) - 2021 • 6796
Substantially enhanced plasticity of bulk metallic glasses by densifying local atomic packing
Wu, Y. and Cao, D. and Yao, Y. and Zhang, G. and Wang, J. and Liu, L. and Li, F. and Fan, H. and Liu, X. and Wang, H. and Wang, X. and Zhu, H. and Jiang, S. and Kontis, P. and Raabe, D. and Gault, B. and Lu, Z.
NATURE COMMUNICATIONS. Volume: 12 (2021) - 2021 • 6795
Ceria-Based Materials for Thermocatalytic and Photocatalytic Organic Synthesis
Huang, X. and Zhang, K. and Peng, B. and Wang, G. and Muhler, M. and Wang, F.
ACS CATALYSIS. Volume: 11 (2021) - 2021 • 6794
Optical spin control and coherence properties of acceptor bound holes in strained GaAs
Linpeng, X. and Karin, T. and Durnev, M.V. and Glazov, M.M. and Schott, R. and Wieck, A.D. and Ludwig, Ar. and Fu, K.-M.C.
PHYSICAL REVIEW B. Volume: 103 (2021) - 2021 • 6793
Quantum polyspectra for modeling and evaluating quantum transport measurements: A unifying approach to the strong and weak measurement regime
Sifft, M. and Kurzmann, A. and Kerski, J. and Schott, R. and Ludwig, A. and Wieck, A.D. and Lorke, A. and Geller, M. and Hagele, D.
PHYSICAL REVIEW RESEARCH. Volume: 3 (2021) - 2021 • 6792
A numerical investigation on the effects of porosity on the plastic anisotropy of additive manufactured stainless steel with various crystallographic textures
Wu, J. and Liu, W. and Vajragupta, N. and Hartmaier, A. and Lian, J.
ESAFORM 2021 - 24TH INTERNATIONAL CONFERENCE ON MATERIAL FORMING. Volume: (2021) - 2021 • 6791
Life cycle strengthening of high-strength steels by nanosecond laser shock
Bai, Y. and Wang, H. and Wang, S. and Huang, Y. and Chen, Y. and Zhang, W. and Ostendorf, A. and Zhou, X.
APPLIED SURFACE SCIENCE. Volume: 569 (2021) - 2021 • 6790
Trace Metal Loading of B-N-Co-doped Graphitic Carbon for Active and Stable Bifunctional Oxygen Reduction and Oxygen Evolution Electrocatalysts
Sikdar, N. and Schwiderowski, P. and Medina, D. and Dieckhöfer, S. and Quast, T. and Brix, A.C. and Cychy, S. and Muhler, M. and Masa, J. and Schuhmann, W.
CHEMELECTROCHEM. Volume: 8 (2021) - 2021 • 6789
Beyond the Scholl reaction-one-step planarization and edge chlorination of nanographenes by mechanochemistry
Baier, D.M. and Grätz, S. and Jahromi, B.F. and Hellmann, S. and Bergheim, K. and Pickhardt, W. and Schmid, R. and Borchardt, L.
RSC ADVANCES. Volume: 11 (2021) - 2021 • 6788
New signatures of the spin gap in quantum point contacts
Hudson, K.L. and Srinivasan, A. and Goulko, O. and Adam, J. and Wang, Q. and Yeoh, L.A. and Klochan, O. and Farrer, I. and Ritchie, D.A. and Ludwig, Ar. and Wieck, A.D. and von Delft, J. and Hamilton, A.R.
NATURE COMMUNICATIONS. Volume: 12 (2021) - 2021 • 6787
Electron heating mode transitions in radio-frequency driven micro atmospheric pressure plasma jets in He/O2: A fluid dynamics approach
Liu, Y. and Korolov, I. and Hemke, T. and Bischoff, L. and Hübner, G. and Schulze, J. and Mussenbrock, T.
JOURNAL OF PHYSICS D: APPLIED PHYSICS. Volume: 54 (2021) - 2021 • 6786
Estimating cardiomyofiber strain in vivo by solving a computational model
Perotti, L.E. and Verzhbinsky, I.A. and Moulin, K. and Cork, T.E. and Loecher, M. and Balzani, D. and Ennis, D.B.
MEDICAL IMAGE ANALYSIS. Volume: 68 (2021) - 2021 • 6785
A Metal–Organic Framework derived CuxOyCz Catalyst for Electrochemical CO2 Reduction and Impact of Local pH Change
Sikdar, N. and Junqueira, J.R.C. and Dieckhöfer, S. and Quast, T. and Braun, M. and Song, Y. and Aiyappa, H.B. and Seisel, S. and Weidner, J. and Öhl, D. and Andronescu, C. and Schuhmann, W.
ANGEWANDTE CHEMIE - INTERNATIONAL EDITION. Volume: 60 (2021) - 2021 • 6784
Microstructure and phase composition evolution of silicon-hafnia feedstock during plasma spraying and following cyclic oxidation
Bakan, E. and Sohn, Y.J. and Vaßen, R.
ACTA MATERIALIA. Volume: 214 (2021) - 2021 • 6783
In situ nanoindentation during electrochemical hydrogen charging: a comparison between front-side and a novel back-side charging approach
Duarte, M.J. and Fang, X. and Rao, J. and Krieger, W. and Brinckmann, S. and Dehm, G.
JOURNAL OF MATERIALS SCIENCE. Volume: 56 (2021) - 2021 • 6782
Non-linear effects and electron heating dynamics in radio-frequency capacitively coupled plasmas with a non-uniform transverse magnetic field
Liu, Y. and Trieschmann, J. and Berger, B. and Schulze, J. and Mussenbrock, T.
PHYSICS OF PLASMAS. Volume: 28 (2021) - 2021 • 6781
Faceting diagram for Ag segregation induced nanofaceting at an asymmetric Cu tilt grain boundary
Peter, N.J. and Duarte, M.J. and Kirchlechner, C. and Liebscher, C.H. and Dehm, G.
ACTA MATERIALIA. Volume: 214 (2021) - 2021 • 6780
Mesoporous NiFe2O4 with Tunable Pore Morphology for Electrocatalytic Water Oxidation
Simon, C. and Timm, J. and Tetzlaff, D. and Jungmann, J. and Apfel, U.-P. and Marschall, R.
CHEMELECTROCHEM. Volume: 8 (2021) - 2021 • 6779
Analysis of THz generation using the tilted-pulse-front geometry in the limit of small pulse energies and beam sizes
Wulf, F. and Hoffmann, M. and Saraceno, C.J.
OPTICS EXPRESS. Volume: 29 (2021) - 2021 • 6778
Direct Detection of Surface Species Formed on Iridium Electrocatalysts during the Oxygen Evolution Reaction
BalaKrishnan, A. and Blanc, N. and Hagemann, U. and Gemagami, P. and Wonner, K. and Tschulik, K. and Li, T.
ANGEWANDTE CHEMIE - INTERNATIONAL EDITION. Volume: 60 (2021) - 2021 • 6777
Magnetic NiFe2O4 Nanoparticles Prepared via Non-Aqueous Microwave-Assisted Synthesis for Application in Electrocatalytic Water Oxidation
Simon, C. and Zakaria, M.B. and Kurz, H. and Tetzlaff, D. and Blösser, A. and Weiss, M. and Timm, J. and Weber, B. and Apfel, U.-P. and Marschall, R.
CHEMISTRY - A EUROPEAN JOURNAL. Volume: (2021) - 2021 • 6776
High Power THz Generation Using Tilted Pulse Fronts with Low Pump Pulse Energies
Wulf, F. and Vogel, T. and Mansourzadeh, S. and Hoffmann, M. and Saraceno, C.J.
2021 CONFERENCE ON LASERS AND ELECTRO-OPTICS EUROPE AND EUROPEAN QUANTUM ELECTRONICS CONFERENCE, CLEO/EUROPE-EQEC 2021. Volume: (2021) - 2021 • 6775
Visible light-induced controlled surface grafting polymerization of hydroxyethyl methacrylate from isopropylthioxanthone semipinacol-terminated organic monolayers
Balasubramaniam, A. and Manderfeld, E. and Krause, L.M.K. and Wanka, R. and Schwarze, J. and Beyer, C.D. and Rosenhahn, A.
POLYMER CHEMISTRY. Volume: 12 (2021) - 2021 • 6774
Design of a new wrought CrCoNi-based medium-entropy superalloy C-264 for high-temperature applications
Hunfeld, J. and Sommer, H. and Kiese, J. and Wang, H. and Riyahi khorasgani, A. and Li, T. and Somsen, C. and Kostka, A. and Laplanche, G.
MATERIALS AND DESIGN. Volume: 211 (2021) - 2021 • 6773
Catalyst-enhanced plasma oxidation of n-butane over α-MnO2 in a temperature-controlled twin surface dielectric barrier discharge reactor
Peters, N. and Schücke, L. and Ollegott, K. and Oberste-Beulmann, C. and Awakowicz, P. and Muhler, M.
PLASMA PROCESSES AND POLYMERS. Volume: (2021) - 2021 • 6772
Molecular Insight into the Swelling of a MOF: A Force-Field Investigation of Methanol Uptake in MIL-88B(Fe)-Cl
Siwaipram, S. and Bopp, P.A. and Keupp, J. and Pukdeejorhor, L. and Soetens, J.-C. and Bureekaew, S. and Schmid, R.
JOURNAL OF PHYSICAL CHEMISTRY C. Volume: (2021) - 2021 • 6771
Deep learning for visualization and novelty detection in large X-ray diffraction datasets
Banko, L. and Maffettone, P.M. and Naujoks, D. and Olds, D. and Ludwig, Al.
NPJ COMPUTATIONAL MATERIALS. Volume: 7 (2021) - 2021 • 6770
Micro atmospheric pressure plasma jets excited in He/O2by voltage waveform tailoring: A study based on a numerical hybrid model and experiments
Liu, Y. and Korolov, I. and Trieschmann, J. and Steuer, D. and Schulz-Von Der Gathen, V. and Böke, M. and Bischoff, L. and Hübner, G. and Schulze, J. and Mussenbrock, T.
PLASMA SOURCES SCIENCE AND TECHNOLOGY. Volume: 30 (2021) - 2021 • 6769
Evaluation of antithrombogenic pHPC on CoCr substrates for biomedical applications
Bannewitz, C. and Lenz-Habijan, T. and Lentz, J. and Peters, M. and Trösken, V. and Siebert, S. and Weber, S. and Theisen, W. and Henkes, H. and Monstadt, H.
COATINGS. Volume: 11 (2021) - 2021 • 6768
A mechanical analysis of chemically stimulated linear shape memory polymer actuation
Dumlu, H. and Marquardt, A. and Zirdehi, E.M. and Varnik, F. and Shen, Y. and Neuking, K. and Eggeler, G.
MATERIALS. Volume: 14 (2021) - 2021 • 6767
Reactive wear protection through strong and deformable oxide nanocomposite surfaces
Liu, C. and Li, Z. and Lu, W. and Bao, Y. and Xia, W. and Wu, X. and Zhao, H. and Gault, B. and Liu, C. and Herbig, M. and Fischer, A. and Dehm, G. and Wu, G. and Raabe, D.
NATURE COMMUNICATIONS. Volume: 12 (2021) - 2021 • 6766
Phase constitution of the noble metal thin-film complex solid solution system Ag-Ir-Pd-Pt-Ru in dependence of elemental compositions and annealing temperatures
Xiao, B. and Wang, X. and Savan, A. and Ludwig, Al.
NANO RESEARCH. Volume: (2021) - 2021 • 6765
A Career in Catalysis: Robert Schlögl
Bao, X. and Behrens, M. and Ertl, G. and Fu, Q. and Knop-Gericke, A. and Lunkenbein, T. and Muhler, M. and Schmidt, C.M. and Trunschke, A.
ACS CATALYSIS. Volume: 11 (2021) - 2021 • 6764
Reinforcing bars modelling using a rod–solid interface element without the need for mesh compatibility
Durand, R. and Farias, M.M. and Pedroso, D.M. and Meschke, G.
FINITE ELEMENTS IN ANALYSIS AND DESIGN. Volume: 197 (2021) - 2021 • 6763
A Low-Temperature Structural Transition in Canfieldite, Ag8SnS6, Single Crystals
Slade, T.J. and Gvozdetskyi, V. and Wilde, J.M. and Kreyssig, A. and Gati, E. and Wang, L.-L. and Mudryk, Y. and Ribeiro, R.A. and Pecharsky, V.K. and Zaikina, J.V. and Bud’ko, S.L. and Canfield, P.C.
INORGANIC CHEMISTRY. Volume: 60 (2021) - 2021 • 6762
Enzyme-Inspired Iron Porphyrins for Improved Electrocatalytic Oxygen Reduction and Evolution Reactions
Xie, L. and Zhang, X.-P. and Zhao, B. and Li, P. and Qi, J. and Guo, X. and Wang, B. and Lei, H. and Zhang, W. and Apfel, U.-P. and Cao, R.
ANGEWANDTE CHEMIE - INTERNATIONAL EDITION. Volume: 60 (2021) - 2021 • 6761
Revealing the Impact of Hierarchical Pore Organization in Supercapacitor Electrodes by Coupling Ionic Dynamics at Micro- and Macroscales
Dvoyashkin, M. and Leistenschneider, D. and Evans, J.D. and Sander, M. and Borchardt, L.
ADVANCED ENERGY MATERIALS. Volume: 11 (2021) - 2021 • 6760
Configuration of a MEMS-based Terahertz Reflectarray Using a Genetic Algorithm
Liu, X. and Schmitt, L. and Kolpatzeck, K. and Hoffmann, M. and Balzer, J.C. and Czylwik, A.
INTERNATIONAL CONFERENCE ON INFRARED, MILLIMETER, AND TERAHERTZ WAVES, IRMMW-THZ. Volume: 2021-August (2021) - 2021 • 6759
CALPHAD-informed phase-field modeling of grain boundary microchemistry and precipitation in Al-Zn-Mg-Cu alloys
Liu, C. and Garner, A. and Zhao, H. and Prangnell, P.B. and Gault, B. and Raabe, D. and Shanthraj, P.
ACTA MATERIALIA. Volume: 214 (2021) - 2021 • 6758
Insights into lithium manganese oxide-water interfaces using machine learning potentials
Eckhoff, M. and Behler, J.
JOURNAL OF CHEMICAL PHYSICS. Volume: 155 (2021) - 2021 • 6757
Single co3o4 nanocubes electrocatalyzing the oxygen evolution reaction: Nano-impact insights into intrinsic activity and support effects
Liu, Z. and Corva, M. and Amin, H.M.A. and Blanc, N. and Linnemann, J. and Tschulik, K.
INTERNATIONAL JOURNAL OF MOLECULAR SCIENCES. Volume: 22 (2021) - 2021 • 6756
Catalytic influence of mineral compounds on the reactivity of cellulose-derived char in O2-, CO2-, and H2O-containing atmospheres
Pflieger, C. and Lotz, K. and Hilse, N. and Berger, C.M. and Schiemann, M. and Debiagi, P. and Hasse, C. and Scherer, V. and Muhler, M.
FUEL. Volume: 287 (2021) - 2021 • 6755
Fe/Co and Ni/Co-pentlandite type electrocatalysts for the hydrogen evolution reaction
Smialkowski, M. and Tetzlaff, D. and Hensgen, L. and Siegmund, D. and Apfel, U.-P.
CHINESE JOURNAL OF CATALYSIS. Volume: 42 (2021) - 2021 • 6754
Sintering Activated Atomic Palladium Catalysts with High-Temperature Tolerance of ∼1,000°C
Yang, N. and Zhao, Y. and Zhang, H. and Xiang, W. and Sun, Y. and Yang, S. and Sun, Y. and Zeng, G. and Kato, K. and Li, X. and Yamauchi, M. and Jiang, Z. and Li, T.
CELL REPORTS PHYSICAL SCIENCE. Volume: 2 (2021) - 2021 • 6753
High-dimensional neural network potentials for magnetic systems using spin-dependent atom-centered symmetry functions
Eckhoff, M. and Behler, J.
NPJ COMPUTATIONAL MATERIALS. Volume: 7 (2021) - 2021 • 6752
Mixed Chromate and Molybdate Additives for Cathodic Enhancement in the Chlorate Process
Smulders, V. and Gomes, A.S.O. and Simic, N. and Mei, B. and Mul, G.
ELECTROCATALYSIS. Volume: 12 (2021) - 2021 • 6751
In-flight distribution of an electron within a surface acoustic wave
Edlbauer, H. and Wang, J. and Ota, S. and Richard, A. and Jadot, B. and Mortemousque, P.-A. and Okazaki, Y. and Nakamura, S. and Kodera, T. and Kaneko, N.-H. and Ludwig, A. and Wieck, A.D. and Urdampilleta, M. and Meunier, T. and Bäuerle, C. and Takada, S.
APPLIED PHYSICS LETTERS. Volume: 119 (2021) - 2021 • 6750
Mechanochemically Assisted Synthesis of Hexaazatriphenylenehexacarbonitrile
Pickhardt, W. and Wohlgemuth, M. and Grätz, S. and Borchardt, L.
JOURNAL OF ORGANIC CHEMISTRY. Volume: 86 (2021) - 2021 • 6749
Numerical investigation of the impact of coating layers on RDF combustion and clinker properties in rotary cement kilns
Pieper, C. and Wirtz, S. and Schaefer, S. and Scherer, V.
FUEL. Volume: 283 (2021) - 2021 • 6748
Impact of test temperature on functional degradation in Fe-Ni-Co-Al-Ta shape memory alloy single crystals
Sobrero, C. and Lauhoff, C. and Langenkämper, D. and Somsen, C. and Eggeler, G. and Chumlyakov, Y.I. and Niendorf, T. and Krooß, P.
MATERIALS LETTERS. Volume: 291 (2021) - 2021 • 6747
Magnetoelectric Tuning of Pinning-Type Permanent Magnets through Atomic-Scale Engineering of Grain Boundaries
Ye, X. and Yan, F. and Schäfer, L. and Wang, D. and Geßwein, H. and Wang, W. and Chellali, M.R. and Stephenson, L.T. and Skokov, K. and Gutfleisch, O. and Raabe, D. and Hahn, H. and Gault, B. and Kruk, R.
ADVANCED MATERIALS. Volume: 33 (2021) - 2021 • 6746
Ab initio study of the structural response to magnetic disorder and van der Waals interactions in FeSe
Lochner, F. and Eremin, I.M. and Hickel, T. and Neugebauer, J.
PHYSICAL REVIEW B. Volume: 103 (2021) - 2021 • 6745
Internal photoeffect from a single quantum emitter
Lochner, P. and Kerski, J. and Kurzmann, A. and Wieck, A.D. and Ludwig, A. and Geller, M. and Lorke, A.
PHYSICAL REVIEW B. Volume: 103 (2021) - 2021 • 6744
Finite-size correction for slab supercell calculations of materials with spontaneous polarization
Yoo, S.-H. and Todorova, M. and Wickramaratne, D. and Weston, L. and Walle, C.G.V. and Neugebauer, J.
NPJ COMPUTATIONAL MATERIALS. Volume: 7 (2021) - 2021 • 6743
Comparing the Activity of Complex Solid Solution Electrocatalysts Using Inflection Points of Voltammetric Activity Curves as Activity Descriptors
Löffler, T. and Waag, F. and Gökce, B. and Ludwig, Al. and Barcikowski, S. and Schuhmann, W.
ACS CATALYSIS. Volume: 11 (2021) - 2021 • 6742
Efficient electronic passivation scheme for computing low-symmetry compound semiconductor surfaces in density-functional theory slab calculations
Yoo, S.-H. and Lymperakis, L. and Neugebauer, J.
PHYSICAL REVIEW MATERIALS. Volume: 5 (2021) - 2021 • 6741
What Makes High-Entropy Alloys Exceptional Electrocatalysts?
Löffler, T. and Ludwig, Al. and Rossmeisl, J. and Schuhmann, W.
ANGEWANDTE CHEMIE - INTERNATIONAL EDITION. Volume: (2021) - 2021 • 6740
Round robin test to compare flexural strength test methods for steel fiber-reinforced sprayed concretes
Youn-Čale, B.-Y. and Plückelmann, S. and Breitenbücher, R.
STRUCTURAL CONCRETE. Volume: (2021) - 2021 • 6739
Bearable Local Stress of High-Strength SFRC
Plückelmann, S. and Breitenbücher, R. and Smarslik, M. and Mark, P.
RILEM BOOKSERIES. Volume: 30 (2021) - 2021 • 6738
Complex-Solid-Solution Electrocatalyst Discovery by Computational Prediction and High-Throughput Experimentation**
Batchelor, T.A.A. and Löffler, T. and Xiao, B. and Krysiak, O.A. and Strotkötter, V. and Pedersen, J.K. and Clausen, C.M. and Savan, A. and Li, Y. and Schuhmann, W. and Rossmeisl, J. and Ludwig, Al.
ANGEWANDTE CHEMIE - INTERNATIONAL EDITION. Volume: (2021) - 2021 • 6737
Chemically induced local lattice distortions versus structural phase transformations in compositionally complex alloys
Ikeda, Y. and Gubaev, K. and Neugebauer, J. and Grabowski, B. and Körmann, F.
NPJ COMPUTATIONAL MATERIALS. Volume: 7 (2021) - 2021 • 6736
ConvNet Fine-Tuning Investigation for GPR Images Classification
Elsaadouny, M. and Barowski, J. and Rolfes, I.
2021 34TH GENERAL ASSEMBLY AND SCIENTIFIC SYMPOSIUM OF THE INTERNATIONAL UNION OF RADIO SCIENCE, URSI GASS 2021. Volume: (2021) - 2021 • 6735
A Minimally Invasive Monitoring Concept for Plasma-Assisted Surface Treatments in PET Bottles
Pohle, D. and Mitschker, F. and Jenderny, J. and Rudolph, M. and Schulz, C. and Awakowicz, P. and Rolfes, I.
2020 50TH EUROPEAN MICROWAVE CONFERENCE, EUMC 2020. Volume: (2021) - 2021 • 6734
B-Cu-Zn Gas Diffusion Electrodes for CO2 Electroreduction to C2+ Products at High Current Densities
Song, Y. and Junqueira, J.R.C. and Sikdar, N. and Öhl, D. and Dieckhöfer, S. and Quast, T. and Seisel, S. and Masa, J. and Andronescu, C. and Schuhmann, W.
ANGEWANDTE CHEMIE - INTERNATIONAL EDITION. Volume: 60 (2021) - 2021 • 6733
Fabrication of Gd: XFeyOzfilms using an atomic layer deposition-type approach
Yu, P. and Beer, S.M.J. and Devi, A. and Coll, M.
CRYSTENGCOMM. Volume: 23 (2021) - 2021 • 6732
Unsupervised Learning Implementation for SAR Images Clustering
Elsaadouny, M. and Barowski, J. and Rolfes, I.
2021 INTERNATIONAL CONFERENCE ON ELECTROMAGNETICS IN ADVANCED APPLICATIONS, ICEAA 2021. Volume: (2021) - 2021 • 6731
Nano Security: From Nano-Electronics to Secure Systems
Polian, I. and Altmann, F. and Arul, T. and Boit, C. and Brederlow, R. and Davi, L. and Drechsler, R. and Du, N. and Eisenbarth, T. and Guneysu, T. and Hermann, S. and Hiller, M. and Leupers, R. and Merchant, F. and Mussenbrock, T. and Katzenbeisser, S. and Kumar, A. and Kunz, W. and Mikolajick, T. and Pachauri, V. and Seifert, J.-P. and Torres, F.S. and Trommer, J.
PROCEEDINGS -DESIGN, AUTOMATION AND TEST IN EUROPE, DATE. Volume: 2021-February (2021) - 2021 • 6730
Layer-by-Layer Deposited Hybrid Polymer Coatings Based on Polysaccharides and Zwitterionic Silanes with Marine Antifouling Properties
Yu, W. and Wang, Y. and Gnutt, P. and Wanka, R. and Krause, L.M.K. and Finlay, J.A. and Clare, A.S. and Rosenhahn, A.
ACS APPLIED BIO MATERIALS. Volume: 4 (2021) - 2021 • 6729
Synergy of ferroelectric polarization and oxygen vacancy to promote CO2 photoreduction
Yu, H. and Chen, F. and Li, X. and Huang, H. and Zhang, Q. and Su, S. and Wang, K. and Mao, E. and Mei, B. and Mul, G. and Ma, T. and Zhang, Y.
NATURE COMMUNICATIONS. Volume: 12 (2021) - 2021 • 6728
Superior low-cycle fatigue properties of CoCrNi compared to CoCrFeMnNi
Lu, K. and Chauhan, A. and Walter, M. and Tirunilai, A.S. and Schneider, M. and Laplanche, G. and Freudenberger, J. and Kauffmann, A. and Heilmaier, M. and Aktaa, J.
SCRIPTA MATERIALIA. Volume: 194 (2021) - 2021 • 6727
Sustainable steel through hydrogen plasma reduction of iron ore: Process, kinetics, microstructure, chemistry
Souza Filho, I.R. and Ma, Y. and Kulse, M. and Ponge, D. and Gault, B. and Springer, H. and Raabe, D.
ACTA MATERIALIA. Volume: 213 (2021) - 2021 • 6726
Deterioration of concrete due to ASR: Experiments and multiscale modeling
Iskhakov, T. and Giebson, C. and Timothy, J.J. and Ludwig, H.M. and Meschke, G.
CEMENT AND CONCRETE RESEARCH. Volume: 149 (2021) - 2021 • 6725
DRIE Si Nanowire Arrays Supported Nano-Carbon Film for Deriving High Specific Energy Supercapacitors On-Chip
Lu, P. and Chen, X. and Ohlckers, P. and Halvorsen, E. and Hoffmann, M. and Müller, L.
JOURNAL OF PHYSICS: CONFERENCE SERIES. Volume: 1837 (2021) - 2021 • 6724
On the Formation Mechanism of Column Damage Within Modular Taper Junctions
Zachariah, Z. and Balachandran, S. and Liu, Z. and Pourzal, R. and McCarthy, S.M. and Hall, D.J. and Fischer, A. and Raabe, D. and Herbig, M.
JOURNAL OF ARTHROPLASTY. Volume: 36 (2021) - 2021 • 6723
Twins – A weak link in the magnetic hardening of ThMn12-type permanent magnets
Ener, S. and Skokov, K.P. and Palanisamy, D. and Devillers, T. and Fischbacher, J. and Eslava, G.G. and Maccari, F. and Schäfer, L. and Diop, L.V.B. and Radulov, I. and Gault, B. and Hrkac, G. and Dempsey, N.M. and Schrefl, T. and Raabe, D. and Gutfleisch, O.
ACTA MATERIALIA. Volume: 214 (2021) - 2021 • 6722
Specific heat and gap structure of a nematic superconductor: Application to FeSe
Islam, K.R. and Böker, J. and Eremin, I.M. and Chubukov, A.V.
PHYSICAL REVIEW B. Volume: 104 (2021) - 2021 • 6721
Coherent Beam Splitting of Flying Electrons Driven by a Surface Acoustic Wave
Ito, R. and Takada, S. and Ludwig, Ar. and Wieck, A.D. and Tarucha, S. and Yamamoto, M.
PHYSICAL REVIEW LETTERS. Volume: 126 (2021) - 2021 • 6720
Optically driving the radiative Auger transition
Spinnler, C. and Zhai, L. and Nguyen, G.N. and Ritzmann, J. and Wieck, A.D. and Ludwig, Ar. and Javadi, A. and Reiter, D.E. and Machnikowski, P. and Warburton, R.J. and Löbl, M.C.
NATURE COMMUNICATIONS. Volume: 12 (2021) - 2021 • 6719
Cobalt Metal ALD: Understanding the Mechanism and Role of Zinc Alkyl Precursors as Reductants for Low-Resistivity Co Thin Films
Zanders, D. and Liu, J. and Obenlüneschloß, J. and Bock, C. and Rogalla, D. and Mai, L. and Nolan, M. and Barry, S.T. and Devi, A.
CHEMISTRY OF MATERIALS. Volume: (2021) - 2021 • 6718
Co(II) Amide, Pyrrolate, and Aminopyridinate Complexes: Assessment of their Manifold Structural Chemistry and Thermal Properties**
Zanders, D. and Boysen, N. and Land, M.A. and Obenlüneschloß, J. and Masuda, J.D. and Mallick, B. and Barry, S.T. and Devi, A.
EUROPEAN JOURNAL OF INORGANIC CHEMISTRY. Volume: (2021) - 2021 • 6717
Polymorphic arrangement of an organic molecule in its hydration environment
Lucht, K. and Morgenstern, K.
JOURNAL OF CHEMICAL PHYSICS. Volume: 154 (2021) - 2021 • 6716
Influence of temperature on void collapse in single crystal nickel under hydrostatic compression
Prasad, M.R.G. and Neogi, A. and Vajragupta, N. and Janisch, R. and Hartmaier, A.
MATERIALS. Volume: 14 (2021) - 2021 • 6715
Maximize mixing in highly polyelemental solid solution alloy nanoparticles
Ludwig, Al.
MATTER. Volume: 4 (2021) - 2021 • 6714
Toward electrocatalytic chemoenzymatic hydrogen evolution and beyond
Prechtl, M.H.G. and Apfel, U.-P.
CELL REPORTS PHYSICAL SCIENCE. Volume: 2 (2021) - 2021 • 6713
Influence of the Lattice Structure of Copper Surfaces on Ammonia Dimer Formation
Srivastava, P. and Miller, D.P. and Morgenstern, K.
JOURNAL OF PHYSICAL CHEMISTRY C. Volume: 125 (2021) - 2021 • 6712
Comparison of Hilbert Transform and Complex Demodulation for Defect Identification in Cutting Discs using Vibration-Based Feature Extraction
Priebe, S. and Brackmann, L. and Alabd-Allah, A. and Butt, S. and Röttger, A. and Meschke, G. and Mueller, I.
LECTURE NOTES IN CIVIL ENGINEERING. Volume: 127 (2021) - 2021 • 6711
Angular-dependent interatomic potential for large-scale atomistic simulation of iron: Development and comprehensive comparison with existing interatomic models
Starikov, S. and Smirnova, D. and Pradhan, T. and Lysogorskiy, Y. and Chapman, H. and Mrovec, M. and Drautz, R.
PHYSICAL REVIEW MATERIALS. Volume: 5 (2021) - 2021 • 6710
Powder properties and flowability measurements of tailored nanocomposites for powder bed fusion applications
Lüddecke, A. and Pannitz, O. and Zetzener, H. and Sehrt, J.T. and Kwade, A.
MATERIALS AND DESIGN. Volume: 202 (2021) - 2021 • 6709
Distant spin entanglement via fast and coherent electron shuttling
Jadot, B. and Mortemousque, P.-A. and Chanrion, E. and Thiney, V. and Ludwig, Ar. and Wieck, A.D. and Urdampilleta, M. and Bäuerle, C. and Meunier, T.
NATURE NANOTECHNOLOGY. Volume: 16 (2021) - 2021 • 6708
Reduction of surface morphology influence on THz reflection time domain spectroscopy for material classification by using multiple observation angles
Becke, L. and Gerling, A. and Hofmann, M.R. and Brenner, C.
PROCEEDINGS OF SPIE - THE INTERNATIONAL SOCIETY FOR OPTICAL ENGINEERING. Volume: 11685 (2021) - 2021 • 6707
Tracer diffusion in the σ phase of the CoCrFeMnNi system
Zhang, J. and Muralikrishna, G.M. and Asabre, A. and Kalchev, Y. and Müller, J. and Butz, B. and Hilke, S. and Rösner, H. and Laplanche, G. and Divinski, S.V. and Wilde, G.
ACTA MATERIALIA. Volume: 203 (2021) - 2021 • 6706
Processing of a newly developed nitrogen-alloyed ferritic-austenitic stainless steel by laser powder bed fusion – Microstructure and properties
Becker, L. and Röttger, A. and Boes, J. and Weber, S. and Theisen, W.
ADDITIVE MANUFACTURING. Volume: 46 (2021) - 2021 • 6705
Dopant-segregation to grain boundaries controls electrical conductivity of n-type NbCo(Pt)Sn half-Heusler alloy mediating thermoelectric performance
Luo, T. and Serrano-Sánchez, F. and Bishara, H. and Zhang, S. and Villoro, B. and Kuo, J.J. and Felser, C. and Scheu, C. and Snyder, G.J. and Best, J.P. and Dehm, G. and Yu, Y. and Raabe, D. and Fu, C. and Gault, B.
ACTA MATERIALIA. Volume: 217 (2021) - 2021 • 6704
Tensile creep properties of a CrMnFeCoNi high-entropy alloy
Zhang, M. and George, E.P. and Gibeling, J.C.
SCRIPTA MATERIALIA. Volume: 194 (2021) - 2021 • 6703
Potential of the Recycling of Grinding Sludge by various Powder Metallurgical Processes
Jäger, S. and Weber, S. and Röttger, A.
PROCEDIA CIRP. Volume: 104 (2021) - 2021 • 6702
Truncated hierarchical B-spline material point method for large deformation geotechnical problems
Zhang, K. and Shen, S.-L. and Zhou, A. and Balzani, D.
COMPUTERS AND GEOTECHNICS. Volume: 134 (2021) - 2021 • 6701
A study on the influence of ligand variation on formamidinate complexes of yttrium: New precursors for atomic layer deposition of yttrium oxide
Beer, S.M.J. and Boysen, N. and Muriqi, A. and Zanders, D. and Berning, T. and Rogalla, D. and Bock, C. and Nolan, M. and Devi, A.
DALTON TRANSACTIONS. Volume: 50 (2021) - 2021 • 6700
Radiative heat transfer with a blocked-off approach for application in the discrete element method
Jaeger, B. and Schlag, M. and Scherer, V. and Wirtz, S. and Schiemann, M.
POWDER TECHNOLOGY. Volume: 392 (2021) - 2021 • 6699
Computational study of simultaneous positive and negative streamer propagation in a twin surface dielectric barrier discharge via 2D PIC simulations
Zhang, Q.-Z. and Nguyen-Smith, R.T. and Beckfeld, F. and Liu, Y. and Mussenbrock, T. and Awakowicz, P. and Schulze, J.
PLASMA SOURCES SCIENCE AND TECHNOLOGY. Volume: 30 (2021) - 2021 • 6698
DEM-CFD simulation of wood pellet degradation by particle-wall impact during pneumatic conveying
Jägers, J. and Brömmer, M. and Illana, E. and Wirtz, S. and Scherer, V.
POWDER TECHNOLOGY. Volume: 391 (2021) - 2021 • 6697
Controlling Oxygen Reduction Selectivity through Steric Effects: Electrocatalytic Two-Electron and Four-Electron Oxygen Reduction with Cobalt Porphyrin Atropisomers
Lv, B. and Li, X. and Guo, K. and Ma, J. and Wang, Y. and Lei, H. and Wang, F. and Jin, X. and Zhang, Q. and Zhang, W. and Long, R. and Xiong, Y. and Apfel, U.-P. and Cao, R.
ANGEWANDTE CHEMIE - INTERNATIONAL EDITION. Volume: 60 (2021) - 2021 • 6696
Hollow CeO2@Co2N Nanosheets Derived from Co-ZIF-L for Boosting the Oxygen Evolution Reaction
Zhang, J. and He, W. and Aiyappa, H.B. and Quast, T. and Dieckhöfer, S. and Öhl, D. and Junqueira, J.R.C. and Chen, Y.-T. and Masa, J. and Schuhmann, W.
ADVANCED MATERIALS INTERFACES. Volume: 8 (2021) - 2021 • 6695
Suppression of nuclear spin fluctuations in an InGaAs quantum dot ensemble by GHz-pulsed optical excitation
Evers, E. and Kopteva, N.E. and Yugova, I.A. and Yakovlev, D.R. and Reuter, D. and Wieck, A.D. and Bayer, M. and Greilich, A.
NPJ QUANTUM INFORMATION. Volume: 7 (2021) - 2021 • 6694
Analysis of wood pellet degradation characteristics based on single particle impact tests
Jägers, J. and Spatz, P. and Wirtz, S. and Scherer, V.
POWDER TECHNOLOGY. Volume: 378 (2021) - 2021 • 6693
Synthetic ferripyrophyllite: Preparation, characterization and catalytic application
Qiao, Y. and Theyssen, N. and Spliethoff, B. and Folke, J. and Weidenthaler, C. and Schmidt, W. and Prieto, G. and Ochoa-Hernández, C. and Bill, E. and Ye, S. and Ruland, H. and Schüth, F. and Leitner, W.
DALTON TRANSACTIONS. Volume: 50 (2021) - 2021 • 6692
Fundamentals: Alloy thermodynamics and kinetics of diffusion
Steinbach, I. and Roslyakova, I. and Abrahams, K.
NICKEL BASE SINGLE CRYSTALS ACROSS LENGTH SCALES. Volume: (2021) - 2021 • 6691
Performant implementation of the atomic cluster expansion (PACE) and application to copper and silicon
Lysogorskiy, Y. and Oord, C. and Bochkarev, A. and Menon, S. and Rinaldi, M. and Hammerschmidt, T. and Mrovec, M. and Thompson, A. and Csányi, G. and Ortner, C. and Drautz, R.
NPJ COMPUTATIONAL MATERIALS. Volume: 7 (2021) - 2021 • 6690
Four Generations of High-Dimensional Neural Network Potentials
Behler, J.
CHEMICAL REVIEWS. Volume: 121 (2021) - 2021 • 6689
Combining Switchable Phase-Change Materials and Phase-Transition Materials for Thermally Regulated Smart Mid-Infrared Modulators
Lyu, X. and Heßler, A. and Wang, X. and Cao, Y. and Song, L. and Ludwig, Al. and Wuttig, M. and Taubner, T.
ADVANCED OPTICAL MATERIALS. Volume: (2021) - 2021 • 6688
High-Temperature Oxidation in Dry and Humid Atmospheres of the Equiatomic CrMnFeCoNi and CrCoNi High- and Medium-Entropy Alloys
Stephan-Scherb, C. and Schulz, W. and Schneider, M. and Karafiludis, S. and Laplanche, G.
OXIDATION OF METALS. Volume: 95 (2021) - 2021 • 6687
Machine learning potentials for extended systems: a perspective
Behler, J. and Csányi, G.
EUROPEAN PHYSICAL JOURNAL B. Volume: 94 (2021) - 2021 • 6686
The Impact of Antimony on the Performance of Antimony Doped Tin Oxide Supported Platinum for the Oxygen Reduction Reaction
Jalalpoor, D. and Göhl, D. and Paciok, P. and Heggen, M. and Knossalla, J. and Radev, I. and Peinecke, V. and Weidenthaler, C. and Mayrhofer, K.J.J. and Ledendecker, M. and Schüth, F.
JOURNAL OF THE ELECTROCHEMICAL SOCIETY. Volume: 168 (2021) - 2021 • 6685
Single-Entity Electrocatalysis of Individual “Picked-and-Dropped” Co3O4 Nanoparticles on the Tip of a Carbon Nanoelectrode
Quast, T. and Aiyappa, H.B. and Saddeler, S. and Wilde, P. and Chen, Y.-T. and Schulz, S. and Schuhmann, W.
ANGEWANDTE CHEMIE - INTERNATIONAL EDITION. Volume: 60 (2021) - 2021 • 6684
Single Particle Nanoelectrochemistry Reveals the Catalytic Oxygen Evolution Reaction Activity of Co3O4 Nanocubes
Quast, T. and Varhade, S. and Saddeler, S. and Chen, Y.-T. and Andronescu, C. and Schulz, S. and Schuhmann, W.
ANGEWANDTE CHEMIE - INTERNATIONAL EDITION. Volume: 60 (2021) - 2021 • 6683
Dedicated setup to isolate plasma catalysis mechanisms
Stewig, C. and Urbanietz, T. and Chauvet, L. and Böke, M. and Von Keudell, A.
JOURNAL OF PHYSICS D: APPLIED PHYSICS. Volume: 54 (2021) - 2021 • 6682
Multi-wall carbon nanotubes electrochemically modified with phosphorus and nitrogen functionalities as a basis for bioelectrodes with improved performance
Quintero-Jaime, A.F. and Conzuelo, F. and Schuhmann, W. and Cazorla-Amorós, D. and Morallón, E.
ELECTROCHIMICA ACTA. Volume: 387 (2021) - 2021 • 6681
Atomistic investigation of machinability of monocrystalline 3C–SiC in elliptical vibration-assisted diamond cutting
Zhao, L. and Zhang, J. and Zhang, J. and Hartmaier, A.
CERAMICS INTERNATIONAL. Volume: 47 (2021) - 2021 • 6680
Uncovering process-structure relationships associated to the hot isostatic pressing of the high-speed steel PMHS 3-3-4 through novel microstructural characterization methods
Benito, S. and Boes, J. and Matsuo, M. and Weber, S. and Theisen, W.
MATERIALS AND DESIGN. Volume: 208 (2021) - 2021 • 6679
The Roles of Composition and Mesostructure of Cobalt-Based Spinel Catalysts in Oxygen Evolution Reactions
Rabe, A. and Büker, J. and Salamon, S. and Koul, A. and Hagemann, U. and Landers, J. and Friedel Ortega, K. and Peng, B. and Muhler, M. and Wende, H. and Schuhmann, W. and Behrens, M.
CHEMISTRY - A EUROPEAN JOURNAL. Volume: (2021) - 2021 • 6678
Energy based three-dimensional damage index for monitoring and damage detection of concrete structures [Energetski baziran trodimenzionalni indeks oštećenja za praćenje i otkrivanje oštećenja na betonskim građevinama]
Stojić, N. and Nestorović, T. and Stojić, D. and Marković, N. and Stojković, N. and Velimirović, N.
GRADJEVINAR. Volume: 73 (2021) - 2021 • 6677
Depth-sensing ductile and brittle deformation in 3C-SiC under Berkovich nanoindentation
Zhao, L. and Zhang, J. and Pfetzing, J. and Alam, M. and Hartmaier, A.
MATERIALS AND DESIGN. Volume: 197 (2021) - 2021 • 6676
Highly Efficient and Selective Aerobic Oxidation of Cinnamyl Alcohol under Visible Light over Pt-Loaded NaNbO3Enriched with Oxygen Vacancies by Ni Doping
Zhao, G. and Bonke, S.A. and Schmidt, S. and Wang, Z. and Hu, B. and Falk, T. and Hu, Y. and Rath, T. and Xia, W. and Peng, B. and Schnegg, A. and Weng, Y. and Muhler, M.
ACS SUSTAINABLE CHEMISTRY AND ENGINEERING. Volume: 9 (2021) - 2021 • 6675
Novel computational tools: General discussion
Bennett, T.D. and Brammer, L. and Coudert, F.-X. and Evans, J.D. and Fischer, M. and Goodwin, A.L. and Jiang, J. and Kaskel, S. and Kitagawa, S. and Krause, S. and Lee, J.-S.M. and Matsuda, R. and Rogge, S.M.J. and Ryder, M.R. and Schmid, R. and Tarzia, A. and Van Der Veen, M.A. and Van Speybroeck, V.
FARADAY DISCUSSIONS. Volume: 225 (2021) - 2021 • 6674
Ultrastructural changes of bovine tooth surfaces under erosion in presence of biomimetic hydroxyapatite
Fabritius-Vilpoux, K. and Enax, J. and Mayweg, D. and Meyer, F. and Herbig, M. and Raabe, D. and Fabritius, H.-O.
BIOINSPIRED, BIOMIMETIC AND NANOBIOMATERIALS. Volume: 10 (2021) - 2021 • 6673
Elementary deformation processes in high temperature plasticity of Ni- and Co-base single-crystal superalloys with γ/γ' microstructures
Rae, C.M.F. and Eggeler, G. and Strudel, J.-L.
NICKEL BASE SINGLE CRYSTALS ACROSS LENGTH SCALES. Volume: (2021) - 2021 • 6672
Achieving ultra-low friction with diamond/metal systems in extreme environments
Stoyanov, P. and Merz, R. and Stricker, M. and Kopnarski, M. and Dienwiebel, M.
MATERIALS. Volume: 14 (2021) - 2021 • 6671
Intercritical annealing to achieve a positive strain-rate sensitivity of mechanical properties and suppression of macroscopic plastic instabilities in multi-phase medium-Mn steels
Benzing, J.T. and Luecke, W.E. and Mates, S.P. and Ponge, D. and Raabe, D. and Wittig, J.E.
MATERIALS SCIENCE AND ENGINEERING A. Volume: 803 (2021) - 2021 • 6670
Identification of Active Sites in the Catalytic Oxidation of 2-Propanol over Co1+xFe2–xO4 Spinel Oxides at Solid/Liquid and Solid/Gas Interfaces
Falk, T. and Budiyanto, E. and Dreyer, M. and Pflieger, C. and Waffel, D. and Büker, J. and Weidenthaler, C. and Ortega, K.F. and Behrens, M. and Tüysüz, H. and Muhler, M. and Peng, B.
CHEMCATCHEM. Volume: 13 (2021) - 2021 • 6669
Aperture Synthesis Method to Investigate on the Reflection Properties of Typical Road Surfaces
Jebramcik, J. and Rolfes, I. and Barowski, J.
2020 50TH EUROPEAN MICROWAVE CONFERENCE, EUMC 2020. Volume: (2021) - 2021 • 6668
Synchronising optical emission spectroscopy to spokes in magnetron sputtering discharges
Maaß, P.A. and Schulz-Von Der Gathen, V. and Von Keudell, A. and Held, J.
PLASMA SOURCES SCIENCE AND TECHNOLOGY. Volume: 30 (2021) - 2021 • 6667
Spinodal Decomposition in Nanocrystalline Alloys
Zhou, X. and Darvishi Kamachali, R. and Boyce, B.L. and Clark, B.G. and Raabe, D. and Thompson, G.B.
ACTA MATERIALIA. Volume: 215 (2021) - 2021 • 6666
Influence of the particle size on selective 2-propanol gas-phase oxidation over Co3O4 nanospheres
Falk, T. and Anke, S. and Hajiyani, H. and Saddeler, S. and Schulz, S. and Pentcheva, R. and Peng, B. and Muhler, M.
CATALYSIS SCIENCE AND TECHNOLOGY. Volume: 11 (2021) - 2021 • 6665
Millimeter Wave Material Measurements for Building Entry Loss Models above 100 GHz
Jebramcik, J. and Wagner, J. and Pohl, N. and Rolfes, I. and Barowski, J.
15TH EUROPEAN CONFERENCE ON ANTENNAS AND PROPAGATION, EUCAP 2021. Volume: (2021) - 2021 • 6664
Promising Membrane for Polymer Electrolyte Fuel Cells Shows Remarkable Proton Conduction over Wide Temperature and Humidity Ranges
Berber, M.R. and Ismail, M.S. and Pourkashanian, M. and Zakaria Hegazy, M.B. and Apfel, U.-P.
ACS APPLIED POLYMER MATERIALS. Volume: 3 (2021) - 2021 • 6663
Tight-binding bond parameters for dimers across the periodic table from density-functional theory
Jenke, J. and Ladines, A.N. and Hammerschmidt, T. and Pettifor, D.G. and Drautz, R.
PHYSICAL REVIEW MATERIALS. Volume: 5 (2021) - 2021 • 6662
The hidden structure dependence of the chemical life of dislocations
Zhou, X. and Mianroodi, J.R. and Da Silva, A.K. and Koenig, T. and Thompson, G.B. and Shanthraj, P. and Ponge, D. and Gault, B. and Svendsen, B. and Raabe, D.
SCIENCE ADVANCES. Volume: 7 (2021) - 2021 • 6661
Strain rate dependency of dislocation plasticity
Fan, H. and Wang, Q. and El-Awady, J.A. and Raabe, D. and Zaiser, M.
NATURE COMMUNICATIONS. Volume: 12 (2021) - 2021 • 6660
Crystallographic Orientation Analysis of Nanocrystalline Tungsten Thin Film Using TEM Precession Electron Diffraction and SEM Transmission Kikuchi Diffraction
Jeong, J. and Jang, W.-S. and Kim, K.H. and Kostka, A. and Gu, G. and Kim, Y.-M. and Oh, S.H.
MICROSCOPY AND MICROANALYSIS. Volume: 27 (2021) - 2021 • 6659
Crystallography companion agent for high-throughput materials discovery
Maffettone, P.M. and Banko, L. and Cui, P. and Lysogorskiy, Y. and Little, M.A. and Olds, D. and Ludwig, Al. and Cooper, A.I.
NATURE COMPUTATIONAL SCIENCE. Volume: 1 (2021) - 2021 • 6658
A fully automated approach to calculate the melting temperature of elemental crystals
Zhu, L.-F. and Janssen, J. and Ishibashi, S. and Körmann, F. and Grabowski, B. and Neugebauer, J.
COMPUTATIONAL MATERIALS SCIENCE. Volume: 187 (2021) - 2021 • 6657
Nanoindentation pop-in in oxides at room temperature: Dislocation activation or crack formation?
Fang, X. and Bishara, H. and Ding, K. and Tsybenko, H. and Porz, L. and Höfling, M. and Bruder, E. and Li, Y. and Dehm, G. and Durst, K.
JOURNAL OF THE AMERICAN CERAMIC SOCIETY. Volume: (2021) - 2021 • 6656
Automated Crystal Orientation Mapping by Precession Electron Diffraction-Assisted Four-Dimensional Scanning Transmission Electron Microscopy Using a Scintillator-Based CMOS Detector
Jeong, J. and Cautaerts, N. and Dehm, G. and Liebscher, C.H.
MICROSCOPY AND MICROANALYSIS. Volume: 27 (2021) - 2021 • 6655
Experimental Evaluation of a Novel Sensor-Based Sorting Approach Featuring Predictive Real-Time Multiobject Tracking
Maier, G. and Pfaff, F. and Pieper, C. and Gruna, R. and Noack, B. and Kruggel-Emden, H. and Langle, T. and Hanebeck, U.D. and Wirtz, S. and Scherer, V. and Beyerer, J.
IEEE TRANSACTIONS ON INDUSTRIAL ELECTRONICS. Volume: 68 (2021) - 2021 • 6654
3d transition-metal high-entropy Invar alloy developed by adjusting the valence-electron concentration
Rao, Z. and Cąklr, A. and Özgün, Ö. and Ponge, D. and Raabe, D. and Li, Z. and Acet, M.
PHYSICAL REVIEW MATERIALS. Volume: 5 (2021) - 2021 • 6653
Hot wear of single phase fcc materials—influence of temperature, alloy composition and stacking fault energy
Berger, A. and Walter, M. and Benito, S.M. and Weber, S.
METALS. Volume: 11 (2021) - 2021 • 6652
Forward: Information modelling of tunnelling and underground infrastructure: Technology and applications
Fang, Y. and Ninic, J. and Meschke, G. and König, M. and Zhang, Q.
TUNNELLING AND UNDERGROUND SPACE TECHNOLOGY. Volume: 116 (2021) - 2021 • 6651
Photocatalytic Deacon Reaction over SrTiO3
Rath, T. and Deitermann, M. and Zhao, G. and Wilma Busser, G. and Jansen, H. and Schwiderowski, P. and Xia, W. and Muhler, M.
CHEMPHOTOCHEM. Volume: 5 (2021) - 2021 • 6650
Superior mechanical properties of a selective-laser-melted AlZnMgCuScZr alloy enabled by a tunable hierarchical microstructure and dual-nanoprecipitation
Zhu, Z. and Ng, F.L. and Seet, H.L. and Lu, W. and Liebscher, C.H. and Rao, Z. and Raabe, D. and Mui Ling Nai, S.
MATERIALS TODAY. Volume: (2021) - 2021 • 6649
Prediction of vibration caused by dynamic compactors considering soil-dependent force emission [Erschütterungsprognose für dynamische verdichtungsgeräte unter berücksichtigung der bodenabhängigen kraftemission]
Berg-Jahnke, R. and Meschke, G. and Heiland, D.
BAUINGENIEUR. Volume: 96 (2021) - 2021 • 6648
Influence of nitrogen uptake and heat treatment on the microstructural characteristics and corrosion performance of X190CrVMo20-4-1 steel produced by supersolidus liquid-phase sintering
Farayibi, P.K. and van gen Hassend, F. and Blüm, M. and Weber, S.
MATERIALS AND CORROSION. Volume: 72 (2021) - 2021 • 6647
Unveiling the interface characteristics and their influence on the heat transfer behavior of hot-forged Cu–Cr/Diamond composites
Jia, S.Q. and Bolzoni, L. and Li, T. and Yang, F.
CARBON. Volume: 172 (2021) - 2021 • 6646
An asymmetric cryptand for the site-specific coordination of 3d metals in multiple oxidation states
Jökel, J. and Nyßen, F. and Siegmund, D. and Apfel, U.-P.
DALTON TRANSACTIONS. Volume: 50 (2021) - 2021 • 6645
Electrochemically activated laser-induced graphene coatings against marine biofouling
Manderfeld, E. and Kleinberg, M.N. and Thamaraiselvan, C. and Koschitzki, F. and Gnutt, P. and Plumere, N. and Arnusch, C.J. and Rosenhahn, A.
APPLIED SURFACE SCIENCE. Volume: 569 (2021) - 2021 • 6644
Facet-Dependent Surface Charge and Hydration of Semiconducting Nanoparticles at Variable pH
Su, S. and Siretanu, I. and van den Ende, D. and Mei, B. and Mul, G. and Mugele, F.
ADVANCED MATERIALS. Volume: 33 (2021) - 2021 • 6643
On the size effect of additives in amorphous shape memory polymers
Zirdehi, E.M. and Dumlu, H. and Eggeler, G. and Varnik, F.
MATERIALS. Volume: 14 (2021) - 2021 • 6642
A dithiacyclam-coordinated silver(i) polymer with anti-cancer stem cell activity
Johnson, A. and Iffland, L. and Singh, K. and Apfel, U.-P. and Suntharalingam, K.
DALTON TRANSACTIONS. Volume: 50 (2021) - 2021 • 6641
Mechanocatalytic Room-Temperature Synthesis of Ammonia from Its Elements Down to Atmospheric Pressure
Reichle, S. and Felderhoff, M. and Schüth, F.
ANGEWANDTE CHEMIE - INTERNATIONAL EDITION. Volume: (2021) - 2021 • 6640
A mixed u–p edge-based smoothed particle finite element formulation for viscous flow simulations
Reinold, J. and Meschke, G.
COMPUTATIONAL MECHANICS. Volume: (2021) - 2021 • 6639
Determining Chemical Reaction Systems in Plasma-Assisted Conversion of Methane Using Genetic Algorithms
Reiser, D. and von Keudell, A. and Urbanietz, T.
PLASMA CHEMISTRY AND PLASMA PROCESSING. Volume: 41 (2021) - 2021 • 6638
Reducing hot tearing by grain boundary segregation engineering in additive manufacturing: example of an AlxCoCrFeNi high-entropy alloy
Sun, Z. and Tan, X. and Wang, C. and Descoins, M. and Mangelinck, D. and Tor, S.B. and Jägle, E.A. and Zaefferer, S. and Raabe, D.
ACTA MATERIALIA. Volume: 204 (2021) - 2021 • 6637
Steering accessible oxygen vacancies for alcohol oxidation over defective Nb2O5 under visible light illumination
Zou, Y. and Hu, Y. and Uhrich, A. and Shen, Z. and Peng, B. and Ji, Z. and Muhler, M. and Zhao, G. and Wang, X. and Xu, X.
APPLIED CATALYSIS B: ENVIRONMENTAL. Volume: 298 (2021) - 2021 • 6636
High-Power Lensless THz Imaging of Hidden Objects
Mansourzadeh, S. and Damyanov, D. and Vogel, T. and Wulf, F. and Kohlhaas, R.B. and Globisch, B. and Schultze, T. and Hoffmann, M. and Balzer, J.C. and Saraceno, C.J.
IEEE ACCESS. Volume: 9 (2021) - 2021 • 6635
Investigations on polarization dynamics of birefringent spin-VCSELs
Jung, N. and Lindemann, M. and Pusch, T. and Hashib, M.F. and Hoti, P. and Debernardi, P. and Michalzik, R. and Hofmann, M.R. and Gerhardt, N.C.
PROCEEDINGS OF SPIE - THE INTERNATIONAL SOCIETY FOR OPTICAL ENGINEERING. Volume: 11704 (2021) - 2021 • 6634
Welding of high-entropy alloys and compositionally complex alloys—an overview
Rhode, M. and Richter, T. and Schroepfer, D. and Manzoni, A.M. and Schneider, M. and Laplanche, G.
WELDING IN THE WORLD. Volume: (2021) - 2021 • 6633
Chemical heterogeneity enhances hydrogen resistance in high-strength steels
Sun, B. and Lu, W. and Gault, B. and Ding, R. and Makineni, S.K. and Wan, D. and Wu, C.-H. and Chen, H. and Ponge, D. and Raabe, D.
NATURE MATERIALS. Volume: (2021) - 2021 • 6632
Digital holography for spatially resolved analysis of the semiconductor optical response
Besaga, V.R. and Gerhardt, N.C. and Hofmann, M.R.
APPLIED OPTICS. Volume: 60 (2021) - 2021 • 6631
Integrated spin-lasers for ultrafast polarization modulation
Jung, N. and Lindemann, M. and Pusch, T. and Michalzik, R. and Hofmann, M.R. and Gerhardt, N.C.
PROCEEDINGS OF SPIE - THE INTERNATIONAL SOCIETY FOR OPTICAL ENGINEERING. Volume: 11805 (2021) - 2021 • 6630
Non-local dx y nematicity and the missing electron pocket in FeSe
Rhodes, L.C. and Böker, J. and Müller, M.A. and Eschrig, M. and Eremin, I.M.
NPJ QUANTUM MATERIALS. Volume: 6 (2021) - 2021 • 6629
Development and Implementation of a Rotating Nanoimprint Lithography Tool for Orthogonal Imprinting on Edges of Curved Surfaces
Supreeti, S. and Schienbein, R. and Feßer, P. and Fern, F. and Hoffmann, M. and Sinzinger, S.
NANOMANUFACTURING AND METROLOGY. Volume: 4 (2021) - 2021 • 6628
CVD grown GaSbxN1-xfilms as visible-light active photoanodes
Zywitzki, D. and Mitoraj, D. and Vilk, Y. and Mendoza Reyes, O. and Schleuning, M. and Friedrich, D. and Sadlo, A. and Rogalla, D. and Eichberger, R. and Beranek, R. and Devi, A.
DALTON TRANSACTIONS. Volume: 50 (2021) - 2021 • 6627
Electrochemical CO2 Reduction: Tailoring Catalyst Layers in Gas Diffusion Electrodes
Junge Puring, K. and Siegmund, D. and Timm, J. and Möllenbruck, F. and Schemme, S. and Marschall, R. and Apfel, U.-P.
ADVANCED SUSTAINABLE SYSTEMS. Volume: 5 (2021) - 2021 • 6626
Magnetic crystalline-symmetry-protected axion electrodynamics and field-tunable unpinned Dirac cones in EuIn2As2
Riberolles, S.X.M. and Trevisan, T.V. and Kuthanazhi, B. and Heitmann, T.W. and Ye, F. and Johnston, D.C. and Bud’ko, S.L. and Ryan, D.H. and Canfield, P.C. and Kreyssig, A. and Vishwanath, A. and McQueeney, R.J. and Wang, L.-L. and Orth, P.P. and Ueland, B.G.
NATURE COMMUNICATIONS. Volume: 12 (2021) - 2021 • 6625
Impact of Water Coadsorption on the Electrode Potential of H-Pt(1 1 1)-Liquid Water Interfaces
Surendralal, S. and Todorova, M. and Neugebauer, J.
PHYSICAL REVIEW LETTERS. Volume: 126 (2021) - 2021 • 6624
Chemical Vapor Deposition of Cobalt and Nickel Ferrite Thin Films: Investigation of Structure and Pseudocapacitive Properties
Zywitzki, D. and Schaper, R. and Ciftyürek, E. and Wree, J.-L. and Taffa, D.H. and Baier, D.M. and Rogalla, D. and Li, Y. and Meischein, M. and Ludwig, A. and Li, Z. and Schierbaum, K. and Wark, M. and Devi, A.
ADVANCED MATERIALS INTERFACES. Volume: 8 (2021) - 2021 • 6623
An extended Hamilton principle as unifying theory for coupled problems and dissipative microstructure evolution
Junker, P. and Balzani, D.
CONTINUUM MECHANICS AND THERMODYNAMICS. Volume: (2021) - 2021 • 6622
Current tuned slotted Y-branch laser for wafer thickness measurements with THz radiation
Surkamp, N. and Gerling, A. and O'Gorman, J. and Honsberg, M. and Schmidtmann, S. and Nandi, U. and Preu, S. and Sacher, J. and Brenner, C. and Hofmann, M.R.
ELECTRONICS LETTERS. Volume: 57 (2021) - 2021 • 6621
Combining Nanoconfinement in Ag Core/Porous Cu Shell Nanoparticles with Gas Diffusion Electrodes for Improved Electrocatalytic Carbon Dioxide Reduction
Junqueira, J.R.C. and O'Mara, P.B. and Wilde, P. and Dieckhöfer, S. and Benedetti, T.M. and Andronescu, C. and Tilley, R.D. and Gooding, J.J. and Schuhmann, W.
CHEMELECTROCHEM. Volume: 8 (2021) - 2021 • 6620
Design of refractory compositionally complex alloys with optimal mechanical properties
Ferrari, A. and Lysogorskiy, Y. and Drautz, R.
PHYSICAL REVIEW MATERIALS. Volume: 5 (2021) - 2021 • 6619
Object-oriented framework for 3D bending and free vibration analysis of multilayer plates: Application to cross-laminated timber and soft-core sandwich panels
Marjanović, M. and Meschke, G. and Damnjanović, E.
COMPOSITE STRUCTURES. Volume: 255 (2021) - 2021 • 6618
Zwitterionic Peptides Reduce Accumulation of Marine and Freshwater Biofilm Formers
Beyer, C.D. and Thavalingam, S. and Guseva, T. and Schardt, L. and Zimmermann, R. and Werner, C. and Dietze, P. and Bandow, J.E. and Metzler-Nolte, N. and Rosenhahn, A.
ACS APPLIED MATERIALS AND INTERFACES. Volume: 13 (2021) - 2021 • 6617
Frequency Coded Retroreflective Landmark for 230 GHz Indoor Self-Localization Systems
Kadera, P. and Jimenez-Saez, A. and Schmitt, L. and Schusler, M. and Hoffmann, M. and Lacik, J. and Jakoby, R.
15TH EUROPEAN CONFERENCE ON ANTENNAS AND PROPAGATION, EUCAP 2021. Volume: (2021) - 2021 • 6616
A Tandem Solar Biofuel Cell: Harnessing Energy from Light and Biofuels
Riedel, M. and Höfs, S. and Ruff, A. and Schuhmann, W. and Lisdat, F.
ANGEWANDTE CHEMIE - INTERNATIONAL EDITION. Volume: 60 (2021) - 2021 • 6615
Internal stress-induced recrystallization and diffusive transport in CaTiO3-PbTiO3solid solutions: A new transport mechanism in geomaterials and its implications for thermobarometry, geochronology, and geospeedometry
Beyer, C. and Chakraborty, S.
AMERICAN MINERALOGIST. Volume: 106 (2021) - 2021 • 6614
Effect of synthesis temperature on the phase formation of NiTiAlFeCr compositionally complex alloy thin films
Marshal, A. and Singh, P. and Music, D. and Wolff-Goodrich, S. and Evertz, S. and Schökel, A. and Johnson, D.D. and Dehm, G. and Liebscher, C.H. and Schneider, J.M.
JOURNAL OF ALLOYS AND COMPOUNDS. Volume: 854 (2021) - 2021 • 6613
Acoustic waveform inversion in frequency domain: Application to a tunnel environment
Riedel, C. and Musayev, K. and Baitsch, M. and Zhu, H. and Hackl, K.
UNDERGROUND SPACE (CHINA). Volume: (2021) - 2021 • 6612
Simulation of the θ’ precipitation process with interfacial anisotropy effects in Al-Cu alloys
Ta, N. and Bilal, M.U. and Häusler, I. and Saxena, A. and Lin, Y.-Y. and Schleifer, F. and Fleck, M. and Glatzel, U. and Skrotzki, B. and Kamachali, R.D.
MATERIALS. Volume: 14 (2021) - 2021 • 6611
Asymmetric pressure distribution in EPB shields: Evaluation of measurements and numerical simulations
Bezuijen, A. and Dang, T.S. and Meschke, G.
GEOTECHNICAL ASPECTS OF UNDERGROUND CONSTRUCTION IN SOFT GROUND - PROCEEDINGS OF THE 10TH INTERNATIONAL SYMPOSIUM ON GEOTECHNICAL ASPECTS OF UNDERGROUND CONSTRUCTION IN SOFT GROUND, IS-CAMBRIDGE 2022. Volume: (2021) - 2021 • 6610
Sensitivity of ultrasonic coda wave interferometry to material damage-observations from a virtual concrete lab
Finger, C. and Saydak, L. and Vu, G. and Timothy, J.J. and Meschke, G. and Saenger, E.H.
MATERIALS. Volume: 14 (2021) - 2021 • 6609
A general, implicit, finite-strain FE 2 framework for the simulation of dynamic problems on two scales
Tamsen, E. and Balzani, D.
COMPUTATIONAL MECHANICS. Volume: 67 (2021) - 2021 • 6608
On-chip electrocatalytic NO sensing using ruthenium oxide nanorods
Tanumihardja, E. and Paradelo Rodríguez, A. and Loessberg-Zahl, J.T. and Mei, B. and Olthuis, W. and van den Berg, A.
SENSORS AND ACTUATORS, B: CHEMICAL. Volume: 334 (2021) - 2021 • 6607
Structural forces in segmental linings: process-oriented tunnel advance simulations vs. conventional structural analysis
Marwan, A. and Gall, V.E. and Alsahly, A. and Meschke, G.
TUNNELLING AND UNDERGROUND SPACE TECHNOLOGY. Volume: 111 (2021) - 2021 • 6606
Relative calibration of a retarding field energy analyzer sensor array for spatially resolved measurements of the ion flux and ion energy in low temperature plasmas
Ries, S. and Schroeder, M. and Woestefeld, M. and Corbella, C. and Korolov, I. and Awakowicz, P. and Schulze, J.
REVIEW OF SCIENTIFIC INSTRUMENTS. Volume: 92 (2021) - 2021 • 6605
Noise-robust classification of single-shot electron spin readouts using a deep neural network
Matsumoto, Y. and Fujita, T. and Ludwig, A. and Wieck, A.D. and Komatani, K. and Oiwa, A.
NPJ QUANTUM INFORMATION. Volume: 7 (2021) - 2021 • 6604
Calibrating SECCM measurements by means of a nanoelectrode ruler. The intrinsic oxygen reduction activity of PtNi catalyst nanoparticles
Tetteh, E.B. and Löffler, T. and Tarnev, T. and Quast, T. and Wilde, P. and Aiyappa, H.B. and Schumacher, S. and Andronescu, C. and Tilley, R.D. and Chen, X. and Schuhmann, W.
NANO RESEARCH. Volume: (2021) - 2021 • 6603
A High-Pressure High-Temperature Column for the Simulation of Hydrothermal Water Circulation at Laboratory Scale
Frank, S. and Zuber, P. and Pollak, S. and Heinze, T. and Schreuer, J. and Wohnlich, S.
GEOTECHNICAL TESTING JOURNAL. Volume: 44 (2021) - 2021 • 6602
Influence of the Fe : Ni Ratio in FexNi9-xS8 (x=3–6) on the CO2 Electroreduction
Tetzlaff, D. and Pellumbi, K. and Puring, K.J. and Siegmund, D. and Polet, W.S.K. and Checinski, M.P. and Apfel, U.-P.
CHEMELECTROCHEM. Volume: 8 (2021) - 2021 • 6601
Path to single-crystalline repair and manufacture of Ni-based superalloy using directional annealing
Kalfhaus, T. and Schaar, H. and Thaler, F. and Ruttert, B. and Sebold, D. and Frenzel, J. and Steinbach, I. and Theisen, W. and Guillon, O. and Clyne, T.W. and Vassen, R.
SURFACE AND COATINGS TECHNOLOGY. Volume: 405 (2021) - 2021 • 6600
Rot-free mixed finite elements for gradient elasticity at finite strains
Riesselmann, J. and Ketteler, J.W. and Schedensack, M. and Balzani, D.
INTERNATIONAL JOURNAL FOR NUMERICAL METHODS IN ENGINEERING. Volume: 122 (2021) - 2021 • 6599
Superhydrophobic Candle Soot as a Low Fouling Stable Coating on Water Treatment Membrane Feed Spacers
Thamaraiselvan, C. and Manderfeld, E. and Kleinberg, M.N. and Rosenhahn, A. and Arnusch, C.J.
ACS APPLIED BIO MATERIALS. Volume: 4 (2021) - 2021 • 6598
Understanding Grain Boundary Electrical Resistivity in Cu: The Effect of Boundary Structure
Bishara, H. and Lee, S. and Brink, T. and Ghidelli, M. and Dehm, G.
ACS NANO. Volume: 15 (2021) - 2021 • 6597
Coated single crystal superalloys: Processing, characterization, and modeling of protective coatings
Maurel, V. and Bartsch, M. and Vidal-Sétif, M.-H. and Vaßen, R. and Guipont, V.
NICKEL BASE SINGLE CRYSTALS ACROSS LENGTH SCALES. Volume: (2021) - 2021 • 6596
Determination of spin-wave stiffness in the Fe-Si system using first-principles calculations
Rinaldi, M. and Mrovec, M. and Fähnle, M. and Drautz, R.
PHYSICAL REVIEW B. Volume: 104 (2021) - 2021 • 6595
Design and characterization of an electrostatic constant-force actuator based on a non-linear spring system
Thewes, A.C. and Schmitt, P. and Löhler, P. and Hoffmann, M.
ACTUATORS. Volume: 10 (2021) - 2021 • 6594
Novel approach to study diffusion of hydrogen bearing species in silicate glasses at low temperatures
Bissbort, T. and Becker, H.-W. and Fanara, S. and Chakraborty, S.
CHEMICAL GEOLOGY. Volume: 562 (2021) - 2021 • 6593
Direct generation of 3D structures by laser polymer deposition
Thiele, M. and Kutlu, Y. and Dobbelstein, H. and Petermann, M. and Esen, C. and Ostendorf, A.
JOURNAL OF LASER APPLICATIONS. Volume: 33 (2021) - 2021 • 6592
One-Step Synthesis of Core-Shell-Structured Mixed-Metal CPO-27(Cu,Co) and Investigations on Its Controlled Thermal Transformation
Bitzer, J. and Göbel, C. and Muhamad Ismail, A. and Fu, Q. and Muhler, M. and Kleist, W.
EUROPEAN JOURNAL OF INORGANIC CHEMISTRY. Volume: 2021 (2021) - 2021 • 6591
The effective equations for the ultrasonic response of wet cortical bone
Blaszczyk, M. and Pertsch Gilbert, R. and Hackl, K.
MATHEMATICAL METHODS IN THE APPLIED SCIENCES. Volume: 44 (2021) - 2021 • 6590
Synergistic Effects of Co and Fe on the Oxygen Evolution Reaction Activity of LaCoxFe1−xO3
Füngerlings, A. and Koul, A. and Dreyer, M. and Rabe, A. and Morales, D.M. and Schuhmann, W. and Behrens, M. and Pentcheva, R.
CHEMISTRY - A EUROPEAN JOURNAL. Volume: (2021) - 2021 • 6589
Correlation between grain size and carbon content in white etching areas in bearings
Mayweg, D. and Morsdorf, L. and Li, Y. and Herbig, M.
ACTA MATERIALIA. Volume: 215 (2021) - 2021 • 6588
Competition between Coulomb and van der Waals Interactions in Xe-Cs+ Aggregates on Cu(111) Surfaces
Thomas, J. and Bertram, C. and Daru, J. and Patwari, J. and Langguth, I. and Zhou, P. and Marx, D. and Morgenstern, K. and Bovensiepen, U.
PHYSICAL REVIEW LETTERS. Volume: 127 (2021) - 2021 • 6587
Multiscale modeling of cancellous bone considering full coupling of mechanical, electric and magnetic effects
Blaszczyk, M. and Hackl, K.
BIOMECHANICS AND MODELING IN MECHANOBIOLOGY. Volume: (2021) - 2021 • 6586
Distinguishing persistent effects in an undoped GaAs/AlGaAs quantum well by top-gate-dependent illumination
Fujita, T. and Hayashi, R. and Kohda, M. and Ritzmann, J. and Ludwig, Ar. and Nitta, J. and Wieck, A.D. and Oiwa, A.
JOURNAL OF APPLIED PHYSICS. Volume: 129 (2021) - 2021 • 6585
Towards blood flow in the virtual human: Efficient self-coupling of HemeLB: Virtual Human Blood Flow with HemeLB
McCullough, J.W.S. and Richardson, R.A. and Patronis, A. and Halver, R. and Marshall, R. and Ruefenacht, M. and Wylie, B.J.N. and Odaker, T. and Wiedemann, M. and Lloyd, B. and Neufeld, E. and Sutmann, G. and Skjellum, A. and Kranzlmüller, D. and Coveney, P.V.
INTERFACE FOCUS. Volume: 11 (2021) - 2021 • 6584
An effective model for cancellous bone with a viscous interstitial fluid *
Blaszczyk, M. and Pertsch Gilbert, R. and Hackl, K.
APPLICABLE ANALYSIS. Volume: (2021) - 2021 • 6583
Characterization of a surface plasmon antenna fabricated on a gate-defined lateral quantum dot
Fukai, R. and Sakai, Y. and Nakagawa, T. and Fujita, T. and Kiyama, H. and Ludwig, Ar. and Wieck, A.D. and Oiwa, A.
JAPANESE JOURNAL OF APPLIED PHYSICS. Volume: 60 (2021) - 2021 • 6582
Link between Structural and Optical Properties of CoxFe3- xO4Nanoparticles and Thin Films with Different Co/Fe Ratios
Kampermann, L. and Klein, J. and Korte, J. and Kowollik, O. and Pfingsten, O. and Smola, T. and Saddeler, S. and Piotrowiak, T.H. and Salamon, S. and Landers, J. and Wende, H. and Ludwig, A. and Schulz, S. and Bacher, G.
JOURNAL OF PHYSICAL CHEMISTRY C. Volume: (2021) - 2021 • 6581
Influence of strain rate on the activation of {110}, {112}, {123} slip in ferrite of DP800
Tian, C. and Dehm, G. and Kirchlechner, C.
MATERIALIA. Volume: 15 (2021) - 2021 • 6580
Detection of photogenerated single electrons in a lateral quantum dot with a surface plasmon antenna
Fukai, R. and Sakai, Y. and Fujita, T. and Kiyama, H. and Ludwig, Ar. and Wieck, A.D. and Oiwa, A.
APPLIED PHYSICS EXPRESS. Volume: 14 (2021) - 2021 • 6579
Link between Structural and Optical Properties of CoxFe3-xO4Nanoparticles and Thin Films with Different Co/Fe Ratios
Kampermann, L. and Klein, J. and Korte, J. and Kowollik, O. and Pfingsten, O. and Smola, T. and Saddeler, S. and Piotrowiak, T.H. and Salamon, S. and Landers, J. and Wende, H. and Ludwig, A. and Schulz, S. and Bacher, G.
JOURNAL OF PHYSICAL CHEMISTRY C. Volume: 125 (2021) - 2021 • 6578
Recovering activity of anodically challenged oxygen reduction electrocatalysts by means of reductive potential pulses
Medina, D. and Löffler, T. and Morales, D.M. and Masa, J. and Bobrowski, T. and Barwe, S. and Andronescu, C. and Schuhmann, W.
ELECTROCHEMISTRY COMMUNICATIONS. Volume: 124 (2021) - 2021 • 6577
In situ investigation of nanometric cutting of 3C-SiC using scanning electron microscope
Tian, D. and Xu, Z. and Liu, L. and Zhou, Z. and Zhang, J. and Zhao, X. and Hartmaier, A. and Liu, B. and Song, L. and Luo, X.
INTERNATIONAL JOURNAL OF ADVANCED MANUFACTURING TECHNOLOGY. Volume: (2021) - 2021 • 6576
Stacking fault energy in relation to hydrogen environment embrittlement of metastable austenitic stainless crni‐steels
Fussik, R. and Egels, G. and Theisen, W. and Weber, S.
METALS. Volume: 11 (2021) - 2021 • 6575
On the origin of controlled anisotropic growth of monodisperse gold nanobipyramids
Meena, S.K. and Lerouge, F. and Baldeck, P. and Andraud, C. and Garavelli, M. and Parola, S. and Sulpizi, M. and Rivalta, I.
NANOSCALE. Volume: 13 (2021) - 2021 • 6574
On the long-term aging of S-phase in aluminum alloy 2618A
Rockenhäuser, C. and Rowolt, C. and Milkereit, B. and Darvishi Kamachali, R. and Kessler, O. and Skrotzki, B.
JOURNAL OF MATERIALS SCIENCE. Volume: (2021) - 2021 • 6573
Microstructure analyses and phase-field simulation of partially divorced eutectic solidification in hypoeutectic Mg-Al Alloys
Kang, J.-H. and Park, J. and Song, K. and Oh, C.-S. and Shchyglo, O. and Steinbach, I.
JOURNAL OF MAGNESIUM AND ALLOYS. Volume: (2021) - 2021 • 6572
Reducing cohesion of metal powders for additive manufacturing by nanoparticle dry-coating
Gärtner, E. and Jung, H.Y. and Peter, N.J. and Dehm, G. and Jägle, E.A. and Uhlenwinkel, V. and Mädler, L.
POWDER TECHNOLOGY. Volume: 379 (2021) - 2021 • 6571
Facilitation of adhesion and spreading of endothelial cells on silicone oxide-coated dacron material by microwave-excited low-pressure plasma
Tilkorn, D.J. and Sorg, H. and Sanders, A. and Köller, M. and Awakowicz, P. and Hauser, J.
INNOVATIVE SURGICAL SCIENCES. Volume: 6 (2021) - 2021 • 6570
Sampling distributions of optimal portfolio weights and characteristics in small and large dimensions
Bodnar, T. and Dette, H. and Parolya, N. and Thorsén, E.
RANDOM MATRICES: THEORY AND APPLICATION. Volume: (2021) - 2021 • 6569
Reaction coordinates in complex systems-a perspective
Rogal, J.
EUROPEAN PHYSICAL JOURNAL B. Volume: 94 (2021) - 2021 • 6568
Principles and Limitations of Photoelectrochemical Fuel Generation
Mei, B. and Wenderich, K.
HETEROGENEOUS PHOTOCATALYSIS: FROM FUNDAMENTALS TO APPLICATIONS IN ENERGY CONVERSION AND DEPOLLUTION. Volume: (2021) - 2021 • 6567
Local Latin hypercube refinement for multi-objective design uncertainty optimization[Formula presented]
Bogoclu, C. and Roos, D. and Nestorović, T.
APPLIED SOFT COMPUTING. Volume: 112 (2021) - 2021 • 6566
Theoretical investigation of the 70.5° mixed dislocations in body-centered cubic transition metals
Romaner, L. and Pradhan, T. and Kholtobina, A. and Drautz, R. and Mrovec, M.
ACTA MATERIALIA. Volume: 217 (2021) - 2021 • 6565
Conjugates of Ciprofloxacin and Amphiphilic Block Copoly(2-alkyl-2-oxazolines)s Overcome Efflux Pumps and Are Active against CIP-Resistant Bacteria
Romanovska, A. and Keil, J. and Tophoven, J. and Oruc, M.F. and Schmidt, M. and Breisch, M. and Sengstock, C. and Weidlich, D. and Klostermeier, D. and Tiller, J.C.
MOLECULAR PHARMACEUTICS. Volume: 18 (2021) - 2021 • 6564
Mn-induced Fermi-surface reconstruction in the SmFeAsO parent compound
Meinero, M. and Bonfà, P. and Onuorah, I.J. and Sanna, S. and De Renzi, R. and Eremin, I. and Müller, M.A. and Orain, J.-C. and Martinelli, A. and Provino, A. and Manfrinetti, P. and Putti, M. and Shiroka, T. and Lamura, G.
SCIENTIFIC REPORTS. Volume: 11 (2021) - 2021 • 6563
Mechanical characterization at high temperature
Bonnand, V. and Le Graverend, J.-B. and Bartsch, M.
NICKEL BASE SINGLE CRYSTALS ACROSS LENGTH SCALES. Volume: (2021) - 2021 • 6562
Stabilization of an iridium oxygen evolution catalyst by titanium oxides
Kasian, O. and Li, T. and Mingers, A.M. and Schweinar, K. and Savan, A. and Ludwig, A. and Mayrhofer, K.
JPHYS ENERGY. Volume: 3 (2021) - 2021 • 6561
Upscaling nanoparticle synthesis by sputter deposition in ionic liquids
Meischein, M. and Ludwig, Al.
JOURNAL OF NANOPARTICLE RESEARCH. Volume: 23 (2021) - 2021 • 6560
Parallel hybrid Monte Carlo / Molecular Statics for Simulation of Solute Segregation in Solids
Ganesan, H. and Longsworth, M. and Sutmann, G.
JOURNAL OF PHYSICS: CONFERENCE SERIES. Volume: 1740 (2021) - 2021 • 6559
Rational Development of Guanidinate and Amidinate Based Cerium and Ytterbium Complexes as Atomic Layer Deposition Precursors: Synthesis, Modeling, and Application
Kaur, P. and Mai, L. and Muriqi, A. and Zanders, D. and Ghiyasi, R. and Safdar, M. and Boysen, N. and Winter, M. and Nolan, M. and Karppinen, M. and Devi, A.
CHEMISTRY - A EUROPEAN JOURNAL. Volume: 27 (2021) - 2021 • 6558
Unraveling the Formation Mechanism of Nanoparticles Sputtered in Ionic Liquid
Meischein, M. and Wang, X. and Ludwig, Al.
JOURNAL OF PHYSICAL CHEMISTRY C. Volume: (2021) - 2021 • 6557
Plastic deformation of single crystals of the equiatomic Cr−Mn−Fe−Co−Ni high-entropy alloy in tension and compression from 10 K to 1273 K
Kawamura, M. and Asakura, M. and Okamoto, N.L. and Kishida, K. and Inui, H. and George, E.P.
ACTA MATERIALIA. Volume: 203 (2021) - 2021 • 6556
Comparable environmental stability and disinfection profiles of the currently circulating SARS-CoV-2 variants of concern B.1.1.7 and B.1.351
Meister, T.L. and Fortmann, J. and Todt, D. and Heinen, N. and Ludwig, Al. and Brüggemann, Y. and Elsner, C. and Dittmer, U. and Steinmann, J. and Pfaender, S. and Steinmann, E.
JOURNAL OF INFECTIOUS DISEASES. Volume: 224 (2021) - 2021 • 6555
Nitrogen and Oxygen Functionalization of Multi-walled Carbon Nanotubes for Tuning the Bifunctional Oxygen Reduction/Oxygen Evolution Performance of Supported FeCo Oxide Nanoparticles
Kazakova, M.A. and Koul, A. and Golubtsov, G.V. and Selyutin, A.G. and Ishchenko, A.V. and Kvon, R.I. and Kolesov, B.A. and Schuhmann, W. and Morales, D.M.
CHEMELECTROCHEM. Volume: (2021) - 2021 • 6554
Atomic layer deposition of dielectric Y2O3thin films from a homoleptic yttrium formamidinate precursor and water
Boysen, N. and Zanders, D. and Berning, T. and Beer, S.M.J. and Rogalla, D. and Bock, C. and Devi, A.
RSC ADVANCES. Volume: 11 (2021) - 2021 • 6553
Automated free-energy calculation from atomistic simulations
Menon, S. and Lysogorskiy, Y. and Rogal, J. and Drautz, R.
PHYSICAL REVIEW MATERIALS. Volume: 5 (2021) - 2021 • 6552
Modifications of an electrolytic aluminum oxide film under the treatment with microdischarges during plasma electrolytic oxidation, a self-organized dielectric barrier discharge (DBD) and a DBD-like plasma jet
Bracht, V. and Kogelheide, F. and Gröger, S. and Hermanns, P. and Böddeker, S. and Bibinov, N. and Awakowicz, P.
PLASMA RESEARCH EXPRESS. Volume: 3 (2021) - 2021 • 6551
Subcritical crack growth in hard alloys under cyclic loading
Brackmann, L. and Röttger, A. and Weber, S. and Theisen, W.
FATIGUE AND FRACTURE OF ENGINEERING MATERIALS AND STRUCTURES. Volume: 44 (2021) - 2021 • 6550
Considering Non-Surface Scattering in Physical Optics Approximations
Garten, O. and Statz, C. and Gerling, S. and Jebramcik, J. and Barowski, J. and Plettemeier, D. and Rolfes, I.
IEEE TRANSACTIONS ON ANTENNAS AND PROPAGATION. Volume: (2021) - 2021 • 6549
Advanced characterisation techniques: Multi-scale,: In situ, and time-resolved: General discussion
Brammer, L. and Burrows, A.D. and Chong, S.Y.-L. and Craig, G. and Evans, J. and Farha, O. and Farrusseng, D. and Fischer, M. and Goodwin, A. and Huang, Z. and Johnson, B. and Kaskel, S. and Kitagawa, S. and Lavenn, C. and Lee, A.Y. and Lee, J.-S.M. and Matsuda, R. and Phillips, A.E. and Rainer, D.N. and Ryder, M.R. and Schmid, R. and Shivanna, M. and Sumby, C. and Taddei, M. and Terry, L. and Ting, V.P. and Van Der Veen, M.A. and West, N.G.
FARADAY DISCUSSIONS. Volume: 225 (2021) - 2021 • 6548
Quantum Sensor for Nanoscale Defect Characterization
Kerski, J. and Lochner, P. and Ludwig, Ar. and Wieck, A.D. and Kurzmann, A. and Lorke, A. and Geller, M.
PHYSICAL REVIEW APPLIED. Volume: 15 (2021) - 2021 • 6547
Formation of short-range magnetic order and avoided ferromagnetic quantum criticality in pressurized LaCrGe3
Gati, E. and Wilde, J.M. and Khasanov, R. and Xiang, L. and Dissanayake, S. and Gupta, R. and Matsuda, M. and Ye, F. and Haberl, B. and Kaluarachchi, U. and McQueeney, R.J. and Kreyssig, A. and Bud'Ko, S.L. and Canfield, P.C.
PHYSICAL REVIEW B. Volume: 103 (2021) - 2021 • 6546
Influence of flexible side-chains on the breathing phase transition of pillared layer MOFs: A force field investigation
Keupp, J. and Dürholt, J.P. and Schmid, R.
FARADAY DISCUSSIONS. Volume: 225 (2021) - 2021 • 6545
On the possible influence of the Fermi–Dirac statistics on the potential and entropy of galvanic cells
Mertin, G.K. and Richter, E. and Oldenburger, M. and Hofmann, M.H. and Wycisk, D. and Wieck, A.D. and Birke, K.P.
JOURNAL OF POWER SOURCES. Volume: 498 (2021) - 2021 • 6544
Atom probe tomography
Gault, B. and Chiaramonti, A. and Cojocaru-Mirédin, O. and Stender, P. and Dubosq, R. and Freysoldt, C. and Makineni, S.K. and Li, T. and Moody, M. and Cairney, J.M.
NATURE REVIEWS METHODS PRIMERS. Volume: 1 (2021) - 2021 • 6543
Benchmark for the coupled magneto-mechanical boundary value problem in magneto-active elastomers
Metsch, P. and Schiedung, R. and Steinbach, I. and Kästner, M.
MATERIALS. Volume: 14 (2021) - 2021 • 6542
Surface metal matrix nano-composite of magnesium/hydroxyapatite produced by stir-centrifugal casting
Khalili, V. and Moslemi, S. and Ruttert, B. and Frenzel, J. and Theisen, W. and Eggeler, G.
SURFACE AND COATINGS TECHNOLOGY. Volume: 406 (2021) - 2021 • 6541
Oscillating combustion—primary measure to reduce nitrogen oxide in a grate furnace–experiments and simulations
Gehrmann, H.-J. and Jaeger, B. and Wirtz, S. and Scherer, V. and Aleksandrov, K. and Hauser, M. and Stapf, D. and Pollmeier, G. and Danz, P.
PROCESSES. Volume: 9 (2021) - 2021 • 6540
Degradation behavior of the MgO/HA surface ceramic nano-composites in the simulated body fluid and its use as a potential bone implant
Khalili, V. and Frenzel, J. and Eggeler, G.
MATERIALS CHEMISTRY AND PHYSICS. Volume: 258 (2021) - 2021 • 6539
Electrocatalytic Oxidation of Glycerol Using Solid-State Synthesised Nickel Boride: Impact of Key Electrolysis Parameters on Product Selectivity
Brix, A.C. and Morales, D.M. and Braun, M. and Jambrec, D. and Junqueira, J.R.C. and Cychy, S. and Seisel, S. and Masa, J. and Muhler, M. and Andronescu, C. and Schuhmann, W.
CHEMELECTROCHEM. Volume: 8 (2021) - 2021 • 6538
40 W SESAM-modelocked Ho:YAG thin-disk laser at 2090 nm
Tomilov, S. and Hoffmann, M. and Heidrich, J. and Alaydin, B.O. and Golling, M. and Wang, Y. and Keller, U. and Saraceno, C.J.
2021 CONFERENCE ON LASERS AND ELECTRO-OPTICS EUROPE AND EUROPEAN QUANTUM ELECTRONICS CONFERENCE, CLEO/EUROPE-EQEC 2021. Volume: (2021) - 2021 • 6537
The nanoscale structure of the Pt-water double layer under bias revealed
Khatib, R. and Kumar, A. and Sanvito, S. and Sulpizi, M. and Cucinotta, C.S.
ELECTROCHIMICA ACTA. Volume: 391 (2021) - 2021 • 6536
Moving towards high-power thin-disk lasers in the 2 µm wavelength range
Tomilov, S. and Hoffmann, M. and Wang, Y. and Saraceno, C.J.
JPHYS PHOTONICS. Volume: 3 (2021) - 2021 • 6535
Variational interface element model for 2D and 3D hydraulic fracturing simulations
Khisamitov, I. and Meschke, G.
COMPUTER METHODS IN APPLIED MECHANICS AND ENGINEERING. Volume: 373 (2021) - 2021 • 6534
Integrated Whispering-Gallery-Mode Resonator for Solid-State Coherent Quantum Photonics
Brooks, A. and Chu, X.-L. and Liu, Z. and Schott, R. and Ludwig, Ar. and Wieck, A.D. and Midolo, L. and Lodahl, P. and Rotenberg, N.
NANO LETTERS. Volume: 21 (2021) - 2021 • 6533
Generalized Method for Charge-Transfer Equilibration in Reactive Molecular Dynamics
Gergs, T. and Schmidt, F. and Mussenbrock, T. and Trieschmann, J.
JOURNAL OF CHEMICAL THEORY AND COMPUTATION. Volume: (2021) - 2021 • 6532
A bright and fast source of coherent single photons
Tomm, N. and Javadi, A. and Antoniadis, N.O. and Najer, D. and Löbl, M.C. and Korsch, A.R. and Schott, R. and Valentin, S.R. and Wieck, A.D. and Ludwig, Ar. and Warburton, R.J.
NATURE NANOTECHNOLOGY. Volume: (2021) - 2021 • 6531
Micro x-ray fluorescence analysis of trace element distribution in frozen hydrated HeLa cells at the P06 beamline at Petra III
Rumancev, C. and Vöpel, T. and Stuhr, S. and Von Gundlach, A. and Senkbeil, T. and Ebbinghaus, S. and Garrevoet, J. and Falkenberg, G. and De Samber, B. and Vincze, L. and Rosenhahn, A. and Schroeder, W.
BIOINTERPHASES. Volume: 16 (2021) - 2021 • 6530
Tuning the Mode Splitting of a Semiconductor Microcavity with Uniaxial Stress
Tomm, N. and Korsch, A.R. and Javadi, A. and Najer, D. and Schott, R. and Valentin, S.R. and Wieck, A.D. and Ludwig, Ar. and Warburton, R.J.
PHYSICAL REVIEW APPLIED. Volume: 15 (2021) - 2021 • 6529
Electrochemical preparation of defect-engineered titania: Bulk doping versus surface contamination
Brüninghoff, R. and Paradelo Rodríguez, A. and Jong, R.P.H. and Sturm, J.M. and Breuer, U. and Lievens, C. and Jeremiasse, A.W. and Mul, G. and Mei, B.
APPLIED SURFACE SCIENCE. Volume: 539 (2021) - 2021 • 6528
Teaching solid mechanics to artificial intelligence—a fast solver for heterogeneous materials
Mianroodi, J.R. and H. Siboni, N. and Raabe, D.
NPJ COMPUTATIONAL MATERIALS. Volume: 7 (2021) - 2021 • 6527
Cutting path-dependent machinability of SiCp/Al composite under multi-step ultra-precision diamond cutting
LU, S. and ZHANG, J. and LI, Z. and ZHANG, J. and WANG, X. and HARTMAIER, A. and XU, J. and YAN, Y. and SUN, T.
CHINESE JOURNAL OF AERONAUTICS. Volume: 34 (2021) - 2021 • 6526
Topological aspects responsible for recrystallization evolution in an IF-steel sheet – Investigation with cellular-automaton simulations
Traka, K. and Sedighiani, K. and Bos, C. and Galan Lopez, J. and Angenendt, K. and Raabe, D. and Sietsma, J.
COMPUTATIONAL MATERIALS SCIENCE. Volume: 198 (2021) - 2021 • 6525
Decelerated aging in metallic glasses by low temperature thermal cycling
Bruns, M. and Hassani, M. and Varnik, F. and Hassanpour, A. and Divinski, S. and Wilde, G.
PHYSICAL REVIEW RESEARCH. Volume: 3 (2021) - 2021 • 6524
Investigation of Cyclam Based Re-Complexes as Potential Electrocatalysts for the CO2 Reduction Reaction
Gerschel, P. and Cordes, A.L. and Bimmermann, S. and Siegmund, D. and Metzler-Nolte, N. and Apfel, U.-P.
ZEITSCHRIFT FUR ANORGANISCHE UND ALLGEMEINE CHEMIE. Volume: 647 (2021) - 2021 • 6523
Influence of microstructure and atomic-scale chemistry on the direct reduction of iron ore with hydrogen at 700°C
Kim, S.-H. and Zhang, X. and Ma, Y. and Souza Filho, I.R. and Schweinar, K. and Angenendt, K. and Vogel, D. and Stephenson, L.T. and El-Zoka, A.A. and Mianroodi, J.R. and Rohwerder, M. and Gault, B. and Raabe, D.
ACTA MATERIALIA. Volume: 212 (2021) - 2021 • 6522
Phase-field modeling of chemoelastic binodal/spinodal relations and solute segregation to defects in binary alloys
Mianroodi, J.R. and Shanthraj, P. and Svendsen, B. and Raabe, D.
MATERIALS. Volume: 14 (2021) - 2021 • 6521
A hysteresis model for the unfrozen liquid content in freezing porous media
Saberi, P.S. and Meschke, G.
COMPUTERS AND GEOTECHNICS. Volume: 134 (2021) - 2021 • 6520
Magnetic ordering and structural distortion in a PrFeAsO single crystal studied by neutron and X-ray scattering
Kim, M.G. and Ratcliff, W. and Pajerowski, D.M. and Kim, J.-W. and Yan, J.-Q. and Lynn, J.W. and Goldman, A.I. and Kreyssig, A.
PHYSICAL REVIEW B. Volume: 103 (2021) - 2021 • 6519
Creep feed grinding induced gradient microstructures in the superficial layer of turbine blade root of single crystal nickel-based superalloy
Miao, Q. and Ding, W. and Xu, J. and Cao, L. and Wang, H. and Yin, Z. and Dai, C. and Kuang, W.
INTERNATIONAL JOURNAL OF EXTREME MANUFACTURING. Volume: 3 (2021) - 2021 • 6518
Kinetic simulation of electron cyclotron resonance assisted gas breakdown in split-biased waveguides for ITER collective Thomson scattering diagnostic
Trieschmann, J. and Larsen, A.W. and Mussenbrock, T. and Korsholm, Sø.B.
PHYSICS OF PLASMAS. Volume: 28 (2021) - 2021 • 6517
Titanium Niobium Oxide Ti2Nb10O29/Carbon Hybrid Electrodes Derived by Mechanochemically Synthesized Carbide for High-Performance Lithium-Ion Batteries
Budak, Ö. and Srimuk, P. and Aslan, M. and Shim, H. and Borchardt, L. and Presser, V.
CHEMSUSCHEM. Volume: 14 (2021) - 2021 • 6516
Nanocrystalline equiatomic CoCrFeNi alloy thin films: Are they single phase fcc?
Kini, M.K. and Lee, S. and Savan, A. and Breitbach, B. and Addab, Y. and Lu, W. and Ghidelli, M. and Ludwig, Al. and Bozzolo, N. and Scheu, C. and Chatain, D. and Dehm, G.
SURFACE AND COATINGS TECHNOLOGY. Volume: 410 (2021) - 2021 • 6515
Homogeneous optical anisotropy in an ensemble of InGaAs quantum dots induced by strong enhancement of the heavy-hole band Landé parameter q
Trifonov, A.V. and Akimov, I.A. and Golub, L.E. and Ivchenko, E.L. and Yugova, I.A. and Kosarev, A.N. and Scholz, S.E. and Sgroi, C. and Ludwig, Ar. and Wieck, A.D. and Yakovlev, D.R. and Bayer, M.
PHYSICAL REVIEW B. Volume: 104 (2021) - 2021 • 6514
Atomic Layer Deposition of Copper Metal Films from Cu(acac)2 and Hydroquinone Reductant
Tripathi, T.S. and Wilken, M. and Hoppe, C. and de los Arcos, T. and Grundmeier, G. and Devi, A. and Karppinen, M.
ADVANCED ENGINEERING MATERIALS. Volume: 23 (2021) - 2021 • 6513
ARE DEVIATIONS in A GRADUALLY VARYING MEAN RELEVANT? A TESTING APPROACH BASED on SUP-NORM ESTIMATORS
Bücher, A. and Dette, H. and Heinrichs, F.
ANNALS OF STATISTICS. Volume: 49 (2021) - 2021 • 6512
Synthesis of Cu Single Atoms Supported on Mesoporous Graphitic Carbon Nitride and Their Application in Liquid-Phase Aerobic Oxidation of Cyclohexene
Büker, J. and Huang, X. and Bitzer, J. and Kleist, W. and Muhler, M. and Peng, B.
ACS CATALYSIS. Volume: 11 (2021) - 2021 • 6511
Self-learning-based detection via multiple microresonator imaging
Saetchnikov, A. and Tcherniavskaia, E. and Saetchnikov, V. and Ostendorf, A.
PROCEEDINGS OF SPIE - THE INTERNATIONAL SOCIETY FOR OPTICAL ENGINEERING. Volume: 11782 (2021) - 2021 • 6510
Scratch hardness at a small scale: Experimental methods and correlation to nanoindentation hardness
Tsybenko, H. and Farzam, F. and Dehm, G. and Brinckmann, S.
TRIBOLOGY INTERNATIONAL. Volume: 163 (2021) - 2021 • 6509
Liquid-Phase Cyclohexene Oxidation with O2 over Spray-Flame-Synthesized La1−xSrxCoO3 Perovskite Nanoparticles
Büker, J. and Alkan, B. and Chabbra, S. and Kochetov, N. and Falk, T. and Schnegg, A. and Schulz, C. and Wiggers, H. and Muhler, M. and Peng, B.
CHEMISTRY - A EUROPEAN JOURNAL. Volume: (2021) - 2021 • 6508
Intelligent imaging sensor out of two-photon polymerized microcavities with self-sensing boosting
Saetchnikov, A. and Tcherniavskaia, E. and Saetchnikov, V. and Ostendorf, A.
2021 CONFERENCE ON LASERS AND ELECTRO-OPTICS EUROPE AND EUROPEAN QUANTUM ELECTRONICS CONFERENCE, CLEO/EUROPE-EQEC 2021. Volume: (2021) - 2021 • 6507
Applications of thermodynamic calculations to practical TEG design: Mg2(Si0.3Sn0.7)/Cu interconnections
Tumminello, S. and Ayachi, S. and Fries, S.G. and Müller, E. and de Boor, J.
JOURNAL OF MATERIALS CHEMISTRY A. Volume: 9 (2021) - 2021 • 6506
Atomic-Precision Tailoring of Au–Ag Core–Shell Composite Nanoparticles for Direct Electrochemical-Plasmonic Hydrogen Evolution in Water Splitting
Mo, J. and Barbosa, E.C.M. and Wu, S. and Li, Y. and Sun, Y. and Xiang, W. and Li, T. and Pu, S. and Robertson, A. and Wu, T.-S. and Soo, Y.-L. and Alves, T.V. and Camargo, P.H.C. and Kuo, W. and Tsang, S.C.E.
ADVANCED FUNCTIONAL MATERIALS. Volume: (2021) - 2021 • 6505
Bendable Polycrystalline and Magnetic CoFe2O4Membranes by Chemical Methods
Salles, P. and Guzmán, R. and Zanders, D. and Quintana, A. and Fina, I. and Sánchez, F. and Zhou, W. and Devi, A. and Coll, M.
ACS APPLIED MATERIALS AND INTERFACES. Volume: (2021) - 2021 • 6504
Effect of Multilayer Termination on Nonspecific Protein Adsorption and Antifouling Activity of Alginate-Based Layer-by-Layer Coatings
Gnanasampanthan, T. and Beyer, C.D. and Yu, W. and Karthäuser, J.F. and Wanka, R. and Spöllmann, S. and Becker, H.-W. and Aldred, N. and Clare, A.S. and Rosenhahn, A.
LANGMUIR. Volume: 37 (2021) - 2021 • 6503
Applying machine learning to optical coherence tomography images for automated tissue classification in brain metastases
Möller, J. and Bartsch, A. and Lenz, M. and Tischoff, I. and Krug, R. and Welp, H. and Hofmann, M.R. and Schmieder, K. and Miller, D.
INTERNATIONAL JOURNAL OF COMPUTER ASSISTED RADIOLOGY AND SURGERY. Volume: (2021) - 2021 • 6502
Processing and oxidation response of Cr2AlC MAX-phase composites containing ceramic fibers
Go, T. and Vaßen, R. and Guillon, O. and Gonzalez-Julian, J.
OPEN CERAMICS. Volume: 6 (2021) - 2021 • 6501
Surface reactions during temperature-programmed desorption and reduction experiments with oxygen-functionalized carbon blacks
Göckeler, M. and Berger, C.M. and Purcel, M. and Bergsträßer, R. and Schinkel, A.-P. and Muhler, M.
APPLIED SURFACE SCIENCE. Volume: 561 (2021) - 2021 • 6500
Mo-doped ZnV2O6/reduced graphene oxide photoanodes for solar hydrogen production
Sameie, H. and Alvani, A.A.S. and Mei, B.T. and Salimi, R. and Poelman, D. and Rosei, F.
ELECTROCHIMICA ACTA. Volume: 382 (2021) - 2021 • 6499
A variationally consistent hyperstatic reaction method for tunnel lining design
Bui, H.-G. and Ninić, J. and Do, N.-A. and Dias, D. and Meschke, G.
INTERNATIONAL JOURNAL FOR NUMERICAL AND ANALYTICAL METHODS IN GEOMECHANICS. Volume: (2021) - 2021 • 6498
Nickel nanoparticles supported on nitrogen–doped carbon nanotubes are a highly active, selective and stable CO2 methanation catalyst
Gödde, J. and Merko, M. and Xia, W. and Muhler, M.
JOURNAL OF ENERGY CHEMISTRY. Volume: 54 (2021) - 2021 • 6497
-Hydrogenases: Maturation and reactivity of enzymatic systems and overview of biomimetic models
Kleinhaus, J.T. and Wittkamp, F. and Yadav, S. and Siegmund, D. and Apfel, U.-P.
CHEMICAL SOCIETY REVIEWS. Volume: 50 (2021) - 2021 • 6496
X-ray-Based Techniques to Study the Nano-Bio Interface
Sanchez-Cano, C. and Alvarez-Puebla, R.A. and Abendroth, J.M. and Beck, T. and Blick, R. and Cao, Y. and Caruso, F. and Chakraborty, I. and Chapman, H.N. and Chen, C. and Cohen, B.E. and Conceição, A.L.C. and Cormode, D.P. and Cui, D. and Dawson, K.A. and Falkenberg, G. and Fan, C. and Feliu, N. and Gao, M. and Gargioni, E. and Glüer, C.-C. and Grüner, F. and Hassan, M. and Hu, Y. and Huang, Y. and Huber, S. and Huse, N. and Kang, Y. and Khademhosseini, A. and Keller, T.F. and Körnig, C. and Kotov, N.A. and Koziej, D. and Liang, X.-J. and Liu, B. and Liu, S. and Liu, Y. and Liu, Z. and Liz-Marzán, L.M. and Ma, X. and Machicote, A. and Maison, W. and Mancuso, A.P. and Megahed, S. and Nickel, B. and Otto, F. and Palencia, C. and Pascarelli, S. and Pearson, A. and Peñate-Medina, O. and Qi, B. and Rädler, J. and Richardson, J.J. and Rosenhahn, A. and Rothkamm, K. and Rübhausen, M. and Sanyal, M.K. and Schaak, R.E. and Schlemmer, H.-P. and Schmidt, M. and Schmutzler, O. and Schotten, T. and Schulz, F. and Sood, A.K. and Spiers, K.M. and Staufer, T. and Stemer, D.M. and Stierle, A. and Sun, X. and Tsakanova, G. and Weiss, P.S. and Weller, H. and Westermeier, F. and Xu, M. and Yan, H. and Zeng, Y. and Zhao, Y. and Zhao, Y. and Zhu, D. and Zhu, Y. and Parak, W.J.
ACS NANO. Volume: 15 (2021) - 2021 • 6495
A new approach for open-end sequential change point monitoring
Gösmann, J. and Kley, T. and Dette, H.
JOURNAL OF TIME SERIES ANALYSIS. Volume: 42 (2021) - 2021 • 6494
S-PEEK as a Catalyst for Gas Phase OME Synthesis
Kley, K.S. and Grünert, A. and Schmidt, W. and Schüth, F.
CHEMCATCHEM. Volume: 13 (2021) - 2021 • 6493
Ion dynamics in capacitively coupled argon-xenon discharges
Klich, M. and Wilczek, S. and Janssen, J.F.J. and Brinkmann, R.P. and Mussenbrock, T. and Trieschmann, J.
PLASMA SOURCES SCIENCE AND TECHNOLOGY. Volume: 30 (2021) - 2021 • 6492
Probing the local activity of CO2reduction on gold gas diffusion electrodes: Effect of the catalyst loading and CO2pressure
Monteiro, M.C.O. and Dieckhöfer, S. and Bobrowski, T. and Quast, T. and Pavesi, D. and Koper, M.T.M. and Schuhmann, W.
CHEMICAL SCIENCE. Volume: 12 (2021) - 2021 • 6491
Ti-bearing lightweight steel with large high temperature ductility via thermally stable multi-phase microstructure
Moon, J. and Jo, H.-H. and Park, S.-J. and Kim, S.-D. and Lee, T.-H. and Lee, C.-H. and Lee, M.-G. and Hong, H.-U. and Suh, D.-W. and Raabe, D.
MATERIALS SCIENCE AND ENGINEERING A. Volume: 808 (2021) - 2021 • 6490
Assessment of a Dual Kalman Filter-Based Approach for Input/Output Estimation in an Aluminum Plate
Sattarifar, A. and Nestorović, T.
LECTURE NOTES IN CIVIL ENGINEERING. Volume: 127 (2021) - 2021 • 6489
Peridynamic analysis of dynamic fracture: influence of peridynamic horizon, dimensionality and specimen size
Butt, S.N. and Meschke, G.
COMPUTATIONAL MECHANICS. Volume: 67 (2021) - 2021 • 6488
Revealing atomic-scale vacancy-solute interaction in nickel
Morgado, F.F. and Katnagallu, S. and Freysoldt, C. and Klaes, B. and Vurpillot, F. and Neugebauer, J. and Raabe, D. and Neumeier, S. and Gault, B. and Stephenson, L.T.
SCRIPTA MATERIALIA. Volume: 203 (2021) - 2021 • 6487
Numerical study of epitaxial growth after partial remelting during selective electron beam melting in the context of ni–al
Schaar, H. and Steinbach, I. and Tegeler, M.
METALS. Volume: 11 (2021) - 2021 • 6486
Oxygen Removal from a Hydrocarbon Containing Gas Stream by Plasma Catalysis
Urbanietz, T. and Stewig, C. and Böke, M. and von Keudell, A.
PLASMA CHEMISTRY AND PLASMA PROCESSING. Volume: 41 (2021) - 2021 • 6485
Foreword: Ni-base superalloy single crystals, a fascinating class of high temperature engineering materials
Cailletaud, G. and Eggeler, G.
NICKEL BASE SINGLE CRYSTALS ACROSS LENGTH SCALES. Volume: (2021) - 2021 • 6484
Modelling of a miniature microwave driven nitrogen plasma jet and comparison to measurements
Klute, M. and Kemaneci, E. and Porteanu, H.-E. and Stefanović, I. and Heinrich, W. and Awakowicz, P. and Brinkmann, R.P.
PLASMA SOURCES SCIENCE AND TECHNOLOGY. Volume: 30 (2021) - 2021 • 6483
Workflow Engineering in Materials Design within the BATTERY 2030+ Project
Schaarschmidt, J. and Yuan, J. and Strunk, T. and Kondov, I. and Huber, S.P. and Pizzi, G. and Kahle, L. and Bölle, F.T. and Castelli, I.E. and Vegge, T. and Hanke, F. and Hickel, T. and Neugebauer, J. and Rêgo, C.R.C. and Wenzel, W.
ADVANCED ENERGY MATERIALS. Volume: (2021) - 2021 • 6482
Nickel Base Single Crystals Across Length Scales
Cailletaud, G. and Cormier, J. and Eggeler, G. and Maurel, V. and Nazé, L.
NICKEL BASE SINGLE CRYSTALS ACROSS LENGTH SCALES. Volume: (2021) - 2021 • 6481
Enhanced spin coherence while displacing electron in a two-dimensional array of quantum dots
Mortemousque, P.-A. and Jadot, B. and Chanrion, E. and Thiney, V. and Bäuerle, C. and Ludwig, Ar. and Wieck, A.D. and Urdampilleta, M. and Meunier, T.
PRX QUANTUM. Volume: 2 (2021) - 2021 • 6480
Experimental and numerical investigations on high performance sfrc: Cyclic tensile loading and fatigue
Schäfer, N. and Gudžulić, V. and Breitenbücher, R. and Meschke, G.
MATERIALS. Volume: 14 (2021) - 2021 • 6479
Link between plasma properties with morphological, structural and mechanical properties of thin Ti films deposited by high power impulse magnetron sputtering
Moskovkin, P. and Maszl, C. and Schierholz, R. and Breilmann, W. and Petersen, J. and Pflug, A. and Muller, J. and Raza, M. and Konstantinidis, S. and von Keudell, A. and Lucas, S.
SURFACE AND COATINGS TECHNOLOGY. Volume: 418 (2021) - 2021 • 6478
Thermal Detection of Glucose in Urine Using a Molecularly Imprinted Polymer as a Recognition Element
Caldara, M. and Lowdon, J.W. and Rogosic, R. and Arreguin-Campos, R. and Jimenez-Monroy, K.L. and Heidt, B. and Tschulik, K. and Cleij, T.J. and Diliën, H. and Eersels, K. and van Grinsven, B.
ACS SENSORS. Volume: 6 (2021) - 2021 • 6477
(tBuN)SiMe2NMe2-A new N,N ′-κ 2-monoanionic ligand for atomic layer deposition precursors
Griffiths, M.B.E. and Zanders, D. and Land, M.A. and Masuda, J.D. and Devi, A. and Barry, S.T.
JOURNAL OF VACUUM SCIENCE AND TECHNOLOGY A: VACUUM, SURFACES AND FILMS. Volume: 39 (2021) - 2021 • 6476
Chemical Vapor Deposition of Hollow Graphitic Spheres for Improved Electrochemical Durability
Knossalla, J. and Mielby, J. and Göhl, D. and Wang, F.R. and Jalalpoor, D. and Hopf, A. and Mayrhofer, K.J.J. and Ledendecker, M. and Schüth, F.
ACS APPLIED ENERGY MATERIALS. Volume: 4 (2021) - 2021 • 6475
Effect of Heat Treatment on the Microstructure of Cast Martensitic Stainless Steel Einfluss der Wärmebehandlung auf die Mikrostruktur von nichtrostendem martensitischen Stahlguss
Van Gen Hassend, F. and Weber, S.
PRAKTISCHE METALLOGRAPHIE/PRACTICAL METALLOGRAPHY. Volume: 58 (2021) - 2021 • 6474
Interface Sensitivity in Electron/Ion Yield X-ray Absorption Spectroscopy: The TiO2-H2O Interface
Van Spronsen, M.A. and Zhao, X. and Jaugstetter, M. and Escudero, C. and Duchoň, T. and Hunt, A. and Waluyo, I. and Yang, P. and Tschulik, K. and Salmeron, M.B.
JOURNAL OF PHYSICAL CHEMISTRY LETTERS. Volume: 12 (2021) - 2021 • 6473
Flexibilization of Biorefineries: Tuning Lignin Hydrogenation by Hydrogen Partial Pressure
Cao, Z. and Xu, Y. and Lyu, P. and Dierks, M. and Morales-García, Á. and Schrader, W. and Nachtigall, P. and Schüth, F.
CHEMSUSCHEM. Volume: 14 (2021) - 2021 • 6472
A fourth-generation high-dimensional neural network potential with accurate electrostatics including non-local charge transfer
Ko, T.W. and Finkler, J.A. and Goedecker, S. and Behler, J.
NATURE COMMUNICATIONS. Volume: 12 (2021) - 2021 • 6471
Bright Electrically Controllable Quantum-Dot-Molecule Devices Fabricated by In Situ Electron-Beam Lithography
Schall, J. and Deconinck, M. and Bart, N. and Florian, M. and von Helversen, M. and Dangel, C. and Schmidt, R. and Bremer, L. and Bopp, F. and Hüllen, I. and Gies, C. and Reuter, D. and Wieck, A.D. and Rodt, S. and Finley, J.J. and Jahnke, F. and Ludwig, Ar. and Reitzenstein, S.
ADVANCED QUANTUM TECHNOLOGIES. Volume: 4 (2021) - 2021 • 6470
Unique performance of thermal barrier coatings made of yttria-stabilized zirconia at extreme temperatures (>1500°C)
Vaßen, R. and Mack, D.E. and Tandler, M. and Sohn, Y.J. and Sebold, D. and Guillon, O.
JOURNAL OF THE AMERICAN CERAMIC SOCIETY. Volume: 104 (2021) - 2021 • 6469
General-Purpose Machine Learning Potentials Capturing Nonlocal Charge Transfer
Ko, T.W. and Finkler, J.A. and Goedecker, S. and Behler, J.
ACCOUNTS OF CHEMICAL RESEARCH. Volume: 54 (2021) - 2021 • 6468
Sulfobetaine Methacrylate Polymers of Unconventional Polyzwitterion Architecture and Their Antifouling Properties
SchAnemann, E. and Koc, J. and Karthaüser, J.F. and Ozcan, O. and Schanzenbach, D. and Schardt, L. and Rosenhahn, A. and Laschewsky, A.
BIOMACROMOLECULES. Volume: 22 (2021) - 2021 • 6467
Influence of substrate removal method on the properties of free-standing ysz coatings
Vaßen, R. and Bakan, E. and Schwartz-Lückge, S.
COATINGS. Volume: 11 (2021) - 2020 • 6466
Steels in additive manufacturing: A review of their microstructure and properties
Bajaj, P. and Hariharan, A. and Kini, A. and Kürnsteiner, P. and Raabe, D. and Jägle, E.A.
MATERIALS SCIENCE AND ENGINEERING A. Volume: 772 (2020) - 2020 • 6465
Phonons in magnetically disordered materials: Magnetic versus phononic time scales
Dutta, B. and Körmann, F. and Ghosh, S. and Sanyal, B. and Neugebauer, J. and Hickel, T.
PHYSICAL REVIEW B. Volume: 101 (2020) - 2020 • 6464
Detection of squeezed phonons in pump-probe spectroscopy
Lakehal, M. and Schiró, M. and Eremin, I.M. and Paul, I.
PHYSICAL REVIEW B. Volume: 102 (2020) - 2020 • 6463
Near Transform-Limited Quantum Dot Linewidths in a Broadband Photonic Crystal Waveguide
Pedersen, F.T. and Wang, Y. and Olesen, C.T. and Scholz, S. and Wieck, A.D. and Ludwig, Ar. and Löbl, M.C. and Warburton, R.J. and Midolo, L. and Uppu, R. and Lodahl, P.
ACS PHOTONICS. Volume: 7 (2020) - 2020 • 6462
Quantitative STEM Imaging and Multislice Simulation of Stacking Fault Defects for Exciton Trapping in GaAs
Spurgeon, S. and Matthews, B. and Sushko, P. and Linpeng, X. and Viitaniemi, M. and Durnev, M. and Glazov, M. and Wieck, A. and Ludwig, Ar. and Fu, K.-M.
MICROSCOPY AND MICROANALYSIS. Volume: (2020) - 2020 • 6461
Unveiling the Re effect in Ni-based single crystal superalloys
Wu, X. and Makineni, S.K. and Liebscher, C.H. and Dehm, G. and Rezaei Mianroodi, J. and Shanthraj, P. and Svendsen, B. and Bürger, D. and Eggeler, G. and Raabe, D. and Gault, B.
NATURE COMMUNICATIONS. Volume: 11 (2020) - 2020 • 6460
High-velocity water vapor corrosion of Yb-silicate: Sprayed vs. sintered body
Bakan, E. and Kindelmann, M. and Kunz, W. and Klemm, H. and Vaßen, R.
SCRIPTA MATERIALIA. Volume: 178 (2020) - 2020 • 6459
Fast diffusion mechanism in Li4P2S6: Via a concerted process of interstitial Li ions
Stamminger, A.R. and Ziebarth, B. and Mrovec, M. and Hammerschmidt, T. and Drautz, R.
RSC ADVANCES. Volume: 10 (2020) - 2020 • 6458
Role of magnetic ordering for the design of quinary TWIP-TRIP high entropy alloys
Wu, X. and Li, Z. and Rao, Z. and Ikeda, Y. and Dutta, B. and Körmann, F. and Neugebauer, J. and Raabe, D.
PHYSICAL REVIEW MATERIALS. Volume: 4 (2020) - 2020 • 6457
An investigation on burner rig testing of environmental barrier coatings for aerospace applications
Bakan, E. and Mack, D.E. and Lobe, S. and Koch, D. and Vaßen, R.
JOURNAL OF THE EUROPEAN CERAMIC SOCIETY. Volume: (2020) - 2020 • 6456
A 3D particle finite element model for the simulation of soft soil excavation using hypoplasticity
Bal, A.R.L. and Dang, T.S. and Meschke, G.
COMPUTATIONAL PARTICLE MECHANICS. Volume: 7 (2020) - 2020 • 6455
Study of grain boundary self-diffusion in iron with different atomistic models
Starikov, S. and Mrovec, M. and Drautz, R.
ACTA MATERIALIA. Volume: 188 (2020) - 2020 • 6454
Crystal–Glass High-Entropy Nanocomposites with Near Theoretical Compressive Strength and Large Deformability
Wu, G. and Balachandran, S. and Gault, B. and Xia, W. and Liu, C. and Rao, Z. and Wei, Y. and Liu, S. and Lu, J. and Herbig, M. and Lu, W. and Dehm, G. and Li, Z. and Raabe, D.
ADVANCED MATERIALS. Volume: 32 (2020) - 2020 • 6453
Atomic Scale Origin of Metal Ion Release from Hip Implant Taper Junctions
Balachandran, S. and Zachariah, Z. and Fischer, A. and Mayweg, D. and Wimmer, M.A. and Raabe, D. and Herbig, M.
ADVANCED SCIENCE. Volume: 7 (2020) - 2020 • 6452
Electrical detection of excitonic states by time-resolved conductance measurements
Ebler, C. and Labud, P.A. and Rai, A.K. and Reuter, D. and Wieck, A.D. and Ludwig, Ar.
PHYSICAL REVIEW B. Volume: 101 (2020) - 2020 • 6451
Enhancing the CO2 Electroreduction of Fe/Ni-Pentlandite Catalysts by S/Se Exchange
Pellumbi, K. and Smialkowski, M. and Siegmund, D. and Apfel, U.-P.
CHEMISTRY - A EUROPEAN JOURNAL. Volume: 26 (2020) - 2020 • 6450
Optimized interatomic potential for study of structure and phase transitions in Si-Au and Si-Al systems
Starikov, S. and Gordeev, I. and Lysogorskiy, Y. and Kolotova, L. and Makarov, S.
COMPUTATIONAL MATERIALS SCIENCE. Volume: 184 (2020) - 2020 • 6449
Fast-Track to Research Data Management in Experimental Material Science-Setting the Ground for Research Group Level Materials Digitalization
Banko, L. and Ludwig, Al.
ACS COMBINATORIAL SCIENCE. Volume: 22 (2020) - 2020 • 6448
Simultaneous analysis of light gases and heavy pyrolyzates evolved from lignite and hard coal by pyrolysis–GC/MS–GC/TCD
Eckhard, T. and Wütscher, A. and Muhler, M.
JOURNAL OF ANALYTICAL AND APPLIED PYROLYSIS. Volume: 149 (2020) - 2020 • 6447
Closing the gap between theory and experiment for lithium manganese oxide spinels using a high-dimensional neural network potential
Eckhoff, M. and Schönewald, F. and Risch, M. and Volkert, C.A. and Blöchl, P.E. and Behler, J.
PHYSICAL REVIEW B. Volume: 102 (2020) - 2020 • 6446
Early stage phase separation of AlCoCr0.75Cu0.5FeNi high-entropy powder at the nanoscale
Peter, N.J. and Duarte, M.J. and Liebscher, C.H. and Srivastava, V.C. and Uhlenwinkel, V. and Jägle, E.A. and Dehm, G.
JOURNAL OF ALLOYS AND COMPOUNDS. Volume: 820 (2020) - 2020 • 6445
Investigation of single asperity wear at the microscale in an austenitic steel
Xia, W. and Dehm, G. and Brinckmann, S.
WEAR. Volume: 452-453 (2020) - 2020 • 6444
Predicting oxidation and spin states by high-dimensional neural networks: Applications to lithium manganese oxide spinels
Eckhoff, M. and Lausch, K.N. and Blöchl, P.E. and Behler, J.
JOURNAL OF CHEMICAL PHYSICS. Volume: 153 (2020) - 2020 • 6443
Coupling electrochemistry with a fluorescence reporting reaction enabled by bipolar electrochemistry
Stefano, J.S. and Conzuelo, F. and Masa, J. and Munoz, R.A.A. and Schuhmann, W.
JOURNAL OF ELECTROANALYTICAL CHEMISTRY. Volume: 872 (2020) - 2020 • 6442
Insight into indentation-induced plastic flow in austenitic stainless steel
Xia, W. and Dehm, G. and Brinckmann, S.
JOURNAL OF MATERIALS SCIENCE. Volume: 55 (2020) - 2020 • 6441
Hybrid density functional theory benchmark study on lithium manganese oxides
Eckhoff, M. and Blöchl, P.E. and Behler, J.
PHYSICAL REVIEW B. Volume: 101 (2020) - 2020 • 6440
Determination of plasma parameters by spectral line broadening in an electrosurgical argon plasma
Hillebrand, B. and Iglesias, E. and Gibson, A.R. and Bibinov, N. and Neugebauer, A. and Enderle, M. and Awakowicz, P.
PLASMA SOURCES SCIENCE AND TECHNOLOGY. Volume: 29 (2020) - 2020 • 6439
Processing of a single-crystalline CrCoNi medium-entropy alloy and evolution of its thermal expansion and elastic stiffness coefficients with temperature
Laplanche, G. and Schneider, M. and Scholz, F. and Frenzel, J. and Eggeler, G. and Schreuer, J.
SCRIPTA MATERIALIA. Volume: 177 (2020) - 2020 • 6438
Data related to the growth of σ-phase precipitates in CrMnFeCoNi high-entropy alloys: Temporal evolutions of precipitate dimensions and concentration profiles at interfaces
Laplanche, G.
DATA IN BRIEF. Volume: 33 (2020) - 2020 • 6437
Water-Soluble Polymers with Appending Porphyrins as Bioinspired Catalysts for the Hydrogen Evolution Reaction
Xie, L. and Tian, J. and Ouyang, Y. and Guo, X. and Zhang, W. and Apfel, U.-P. and Zhang, W. and Cao, R.
ANGEWANDTE CHEMIE - INTERNATIONAL EDITION. Volume: 59 (2020) - 2020 • 6436
A Computational Approach to the Microstructural Design of High-Speed Steels
Egels, G. and Wulbieter, N. and Weber, S. and Theisen, W.
STEEL RESEARCH INTERNATIONAL. Volume: 91 (2020) - 2020 • 6435
Pattern-forming nanoprecipitates in NiTi-related high entropy shape memory alloys
Hinte, C. and Barienti, K. and Steinbrücker, J. and Gerstein, G. and Swider, M.A. and Herbst, S. and Eggeler, G. and Maier, H.J.
SCRIPTA MATERIALIA. Volume: 186 (2020) - 2020 • 6434
Growth kinetics of σ-phase precipitates and underlying diffusion processes in CrMnFeCoNi high-entropy alloys
Laplanche, G.
ACTA MATERIALIA. Volume: 199 (2020) - 2020 • 6433
Predictive tracking with improved motion models for optical belt sorting Prädiktives Tracking mit verbesserten Bewegungsmodellen für die optische Schüttgutsortierung
Pfaff, F. and Pieper, C. and Maier, G. and Noack, B. and Gruna, R. and Kruggel-Emden, H. and Hanebeck, U.D. and Wirtz, S. and Scherer, V. and Längle, T. and Beyerer, J.
AT-AUTOMATISIERUNGSTECHNIK. Volume: 68 (2020) - 2020 • 6432
Nanocutting mechanism of 6H-SiC investigated by scanning electron microscope online observation and stress-assisted and ion implant-assisted approaches
Xu, Z. and Liu, L. and He, Z. and Tian, D. and Hartmaier, A. and Zhang, J. and Luo, X. and Rommel, M. and Nordlund, K. and Zhang, G. and Fang, F.
INTERNATIONAL JOURNAL OF ADVANCED MANUFACTURING TECHNOLOGY. Volume: 106 (2020) - 2020 • 6431
Effect of O2/CO2 atmospheres on coal fragmentation
Bareschino, P. and Urciuolo, M. and Scherer, V. and Chirone, R. and Senneca, O.
FUEL. Volume: 267 (2020) - 2020 • 6430
Enantioselective epoxidation by flavoprotein monooxygenases supported by organic solvents
Eggerichs, D. and Mügge, C. and Mayweg, J. and Apfel, U.-P. and Tischler, D.
CATALYSTS. Volume: 10 (2020) - 2020 • 6429
The Effect of Increasing Chemical Complexity on the Mechanical and Functional Behavior of NiTi-Related Shape Memory Alloys
Hinte, C. and Barienti, K. and Steinbrücker, J. and Hartmann, J.-M. and Gerstein, G. and Herbst, S. and Piorunek, D. and Frenzel, J. and Fantin, A. and Maier, H.J.
SHAPE MEMORY AND SUPERELASTICITY. Volume: 6 (2020) - 2020 • 6428
Terahertz Fano resonances induced by combining metamaterial modes of the same symmetry
Xu, R. and Zhang, Z. and Wieck, A.D. and Jukam, N.
OPTICS EXPRESS. Volume: 28 (2020) - 2020 • 6427
Blind competition on the numerical simulation of steel-fiber-reinforced concrete beams failing in shear
Barros, J. and Sanz, B. and Kabele, P. and Yu, R.C. and Meschke, G. and Planas, J. and Cunha, V. and Caggiano, A. and Ozyurt, N. and Gouveia, V. and van den Bos, A. and Poveda, E. and Gal, E. and Cervenka, J. and Neu, G.E. and Rossi, P. and Dias-da-Costa, D. and Juhasz, P.K. and Cendon, D. and Ruiz, G. and Valente, T.
STRUCTURAL CONCRETE. Volume: (2020) - 2020 • 6426
Thermoelastic anisotropy in NdScO3 and NdGaO3 perovskites
Hirschle, C. and Schreuer, J. and Ganschow, S. and Peters, L.
MATERIALS CHEMISTRY AND PHYSICS. Volume: 254 (2020) - 2020 • 6425
Direct Mechanocatalysis: Using Milling Balls as Catalysts
Pickhardt, W. and Grätz, S. and Borchardt, L.
CHEMISTRY - A EUROPEAN JOURNAL. Volume: 26 (2020) - 2020 • 6424
Microscale Atmospheric Pressure Plasma Jet as a Source for Plasma-Driven Biocatalysis
Yayci, A. and Dirks, T. and Kogelheide, F. and Alcalde, M. and Hollmann, F. and Awakowicz, P. and Bandow, J.E.
CHEMCATCHEM. Volume: 12 (2020) - 2020 • 6423
Burst-like reverse martensitic transformation during heating, cooling and under isothermal conditions in stabilized Ni-Ti-Nb
Picornell, C. and Pons, J. and Paulsen, A. and Frenzel, J. and Kaminskii, V. and Sapozhnikov, K. and Van Humbeeck, J. and Kustov, S.
SCRIPTA MATERIALIA. Volume: 180 (2020) - 2020 • 6422
Protection strategies for biocatalytic proteins under plasma treatment
Yayci, A. and Dirks, T. and Kogelheide, F. and Alcalde, M. and Hollmann, F. and Awakowicz, P. and Bandow, J.E.
JOURNAL OF PHYSICS D: APPLIED PHYSICS. Volume: 54 (2020) - 2020 • 6421
Adaptive Concurrent Topology Optimization of Coated Structures with Nonperiodic Infill for Additive Manufacturing
Hoang, V.-N. and Tran, P. and Nguyen, N.-L. and Hackl, K. and Nguyen-Xuan, H.
CAD COMPUTER AIDED DESIGN. Volume: 129 (2020) - 2020 • 6420
Identifying the nature of the active sites in methanol synthesis over Cu/ZnO/Al2O3 catalysts
Laudenschleger, D. and Ruland, H. and Muhler, M.
NATURE COMMUNICATIONS. Volume: 11 (2020) - 2020 • 6419
Interaction of the combustion of refuse derived fuel with the clinker bed in rotary cement kilns: A numerical study
Pieper, C. and Liedmann, B. and Wirtz, S. and Scherer, V. and Bodendiek, N. and Schaefer, S.
FUEL. Volume: 266 (2020) - 2020 • 6418
Excitation and dissociation of CO2 heavily diluted in noble gas atmospheric pressure plasma
Stewig, C. and Schüttler, S. and Urbanietz, T. and Böke, M. and Von Keudell, A.
JOURNAL OF PHYSICS D: APPLIED PHYSICS. Volume: 53 (2020) - 2020 • 6417
Can small polyaromatics describe their larger counterparts for local reactions? A computational study on the H-abstraction reaction by an H-atom from polyaromatics
Yonder, Ö. and Schmitz, G. and Hättig, C. and Schmid, R. and Debiagi, P. and Hasse, C. and Locaspi, A. and Faravelli, T.
JOURNAL OF PHYSICAL CHEMISTRY A. Volume: 124 (2020) - 2020 • 6416
Influence of Ti3Ni4 precipitates on the indentation-induced two-way shape-memory effect in Nickel-Titanium
Laursen, C.M. and Peter, N.J. and Gerstein, G. and Maier, H.J. and Dehm, G. and Frick, C.P.
MATERIALS SCIENCE AND ENGINEERING A. Volume: 792 (2020) - 2020 • 6415
Chemical complexity, microstructure and martensitic transformation in high entropy shape memory alloys
Piorunek, D. and Frenzel, J. and Jöns, N. and Somsen, C. and Eggeler, G.
INTERMETALLICS. Volume: 122 (2020) - 2020 • 6414
Effect of off-stoichiometric compositions on microstructures and phase transformation behavior in Ni-Cu-Pd-Ti-Zr-Hf high entropy shape memory alloys
Piorunek, D. and Oluwabi, O. and Frenzel, J. and Kostka, A. and Maier, H.J. and Somsen, C. and Eggeler, G.
JOURNAL OF ALLOYS AND COMPOUNDS. Volume: (2020) - 2020 • 6413
Studies on ultra-short pulsed laser shock peening of stainless-steel in different confinement media
Elango, K. and Hoppius, J.S. and Kukreja, L.M. and Ostendorf, A. and Gurevich, E.L.
SURFACE AND COATINGS TECHNOLOGY. Volume: 397 (2020) - 2020 • 6412
Dislocation plasticity in FeCoCrMnNi high-entropy alloy: quantitative insights from in situ transmission electron microscopy deformation
Lee, S. and Duarte, M.J. and Feuerbacher, M. and Soler, R. and Kirchlechner, C. and Liebscher, C.H. and Oh, S.H. and Dehm, G.
MATERIALS RESEARCH LETTERS. Volume: 8 (2020) - 2020 • 6411
High-Throughput Characterization of (FexCo1- x)3O4Thin-Film Composition Spreads
Piotrowiak, T.H. and Wang, X. and Banko, L. and Kumari, S. and Sarker, S. and Mehta, A. and Ludwig, Al.
ACS COMBINATORIAL SCIENCE. Volume: 22 (2020) - 2020 • 6410
Revealing nano-chemistry at lattice defects in thermoelectric materials using atom probe tomography
Yu, Y. and Zhou, C. and Zhang, S. and Zhu, M. and Wuttig, M. and Scheu, C. and Raabe, D. and Snyder, G.J. and Gault, B. and Cojocaru-Mirédin, O.
MATERIALS TODAY. Volume: 32 (2020) - 2020 • 6409
Degradable hyaluronic acid/chitosan polyelectrolyte multilayers with marine fouling-release properties
Yu, W. and Wanka, R. and Finlay, J.A. and Clarke, J.L. and Clare, A.S. and Rosenhahn, A.
BIOFOULING. Volume: 36 (2020) - 2020 • 6408
Time-dependent plasticity in silicon microbeams mediated by dislocation nucleation
Elhebeary, M. and Harzer, T. and Dehm, G. and Saif, M.T.A.
PROCEEDINGS OF THE NATIONAL ACADEMY OF SCIENCES OF THE UNITED STATES OF AMERICA. Volume: 117 (2020) - 2020 • 6407
Bulk and surface low temperature phase transitions in the mg-alloy ez33a
Straumal, A. and Mazilkin, I. and Tzoy, K. and Straumal, B. and Bryła, K. and Baranchikov, A. and Eggeler, G.
METALS. Volume: 10 (2020) - 2020 • 6406
Comparison of Spectra of Grain Boundaries Spontaneously Formed in Cu-Ag and Cu-In Systems
Straumal, A.B. and Tsoi, K.V. and Mazilkin, I.A. and Rodin, A.O. and Eggeler, G.
JETP LETTERS. Volume: 111 (2020) - 2020 • 6405
Electroenzymatic Nitrogen Fixation Using a MoFe Protein System Immobilized in an Organic Redox Polymer
Lee, Y.S. and Ruff, A. and Cai, R. and Lim, K. and Schuhmann, W. and Minteer, S.D.
ANGEWANDTE CHEMIE - INTERNATIONAL EDITION. Volume: 59 (2020) - 2020 • 6404
Deformation behavior of concretes with high plastic compressibility [Verformungsverhalten von Betonen mit ausgeprägtem plastischen Stauchvermögen]
Plückelmann, S. and Breitenbücher, R.
BETON- UND STAHLBETONBAU. Volume: 115 (2020) - 2020 • 6403
Prismatic Slip in Magnesium
Stricker, M. and Curtin, W.A.
JOURNAL OF PHYSICAL CHEMISTRY C. Volume: 124 (2020) - 2020 • 6402
Investigation on the scattering characteristics and unsupervised clustering of 3D printed samples
Elsaadouny, M. and Barowski, J. and Jebramcik, J. and Rolfes, I.
INTERNATIONAL JOURNAL OF MICROWAVE AND WIRELESS TECHNOLOGIES. Volume: 12 (2020) - 2020 • 6401
Characterisation of micropores in plasma deposited SiO xfilms by means of positron annihilation lifetime spectroscopy
Hoppe, C. and Mitschker, F. and Butterling, M. and Liedke, M.O. and De Los Arcos, T. and Awakowicz, P. and Wagner, A. and Grundmeier, G.
JOURNAL OF PHYSICS D: APPLIED PHYSICS. Volume: 53 (2020) - 2020 • 6400
Round-robin test on various test-methods for flexural behavior of steel fiber reinforced sprayed concretes
Plückelmann, S. and Breitenbücher, R.
AMERICAN CONCRETE INSTITUTE, ACI SPECIAL PUBLICATION. Volume: SP-343 (2020) - 2020 • 6399
Machine learning for metallurgy II. A neural-network potential for magnesium
Stricker, M. and Yin, B. and Mak, E. and Curtin, W.A.
PHYSICAL REVIEW MATERIALS. Volume: 4 (2020) - 2020 • 6398
Study on Structural and Thermal Characteristics of Heteroleptic Yttrium Complexes as Potential Precursors for Vapor Phase Deposition
Beer, S.M.J. and Krusenbaum, A. and Winter, M. and Vahlas, C. and Devi, A.
EUROPEAN JOURNAL OF INORGANIC CHEMISTRY. Volume: 2020 (2020) - 2020 • 6397
FPGA Based Accelerator for Buried Objects Identification
Elsaadouny, M. and Barowski, J. and Rolfes, I.
2020 43RD INTERNATIONAL CONFERENCE ON TELECOMMUNICATIONS AND SIGNAL PROCESSING, TSP 2020. Volume: (2020) - 2020 • 6396
Experimental investigation of hybrid concrete elements with varying fiber reinforcement under concentrated load
Plückelmann, S. and Breitenbücher, R.
AMERICAN CONCRETE INSTITUTE, ACI SPECIAL PUBLICATION. Volume: SP-343 (2020) - 2020 • 6395
Dual-Heteroatom-Doped Reduced Graphene Oxide Sheets Conjoined CoNi-Based Carbide and Sulfide Nanoparticles for Efficient Oxygen Evolution Reaction
Zakaria, M.B. and Zheng, D. and Apfel, U.-P. and Nagata, T. and Kenawy, E.-R.S. and Lin, J.
ACS APPLIED MATERIALS AND INTERFACES. Volume: 12 (2020) - 2020 • 6394
Extracting the Features of the Shallowly Buried Objects using LeNet Convolutional Network
Elsaadouny, M. and Barowski, J. and Rolfes, I.
14TH EUROPEAN CONFERENCE ON ANTENNAS AND PROPAGATION, EUCAP 2020. Volume: (2020) - 2020 • 6393
Exploring the fundamentals of Ni-based superalloy single crystal (SX) alloy design: Chemical composition vs. microstructure
Horst, O.M. and Adler, D. and Git, P. and Wang, H. and Streitberger, J. and Holtkamp, M. and Jöns, N. and Singer, R.F. and Körner, C. and Eggeler, G.
MATERIALS AND DESIGN. Volume: 195 (2020) - 2020 • 6392
The effect of flue gas contaminants on the CO2electroreduction to formic acid
Legrand, U. and Apfel, U.-P. and Boffito, D.C. and Tavares, J.R.
JOURNAL OF CO2 UTILIZATION. Volume: 42 (2020) - 2020 • 6391
Orientation-dependent deformation behavior of 316L steel manufactured by laser metal deposition and casting under local scratch and indentation load
Pöhl, F. and Hardes, C. and Scholz, F. and Frenzel, J.
MATERIALS. Volume: 13 (2020) - 2020 • 6390
A Rare Low-Spin CoIV Bis(β-silyldiamide) with High Thermal Stability: Steric Enforcement of a Doublet Configuration
Zanders, D. and Bačić, G. and Leckie, D. and Odegbesan, O. and Rawson, J. and Masuda, J.D. and Devi, A. and Barry, S.T.
ANGEWANDTE CHEMIE - INTERNATIONAL EDITION. Volume: 59 (2020) - 2020 • 6389
On the Influence of Alloy Composition on Creep Behavior of Ni-Based Single-Crystal Superalloys (SXs)
Horst, O.M. and Ibrahimkhel, S. and Streitberger, J. and Wochmjakow, N. and Git, P. and Scholz, F. and Thome, P. and Singer, R.F. and Körner, C. and Frenzel, J. and Eggeler, G.
MINERALS, METALS AND MATERIALS SERIES. Volume: (2020) - 2020 • 6388
Snoek-type damping performance in strong and ductile high-entropy alloys
Lei, Z. and Wu, Y. and He, J. and Liu, X. and Wang, H. and Jiang, S. and Gu, L. and Zhang, Q. and Gault, B. and Raabe, D. and Lu, Z.
SCIENCE ADVANCES. Volume: 6 (2020) - 2020 • 6387
Faceted Branched Nickel Nanoparticles with Tunable Branch Length for High-Activity Electrocatalytic Oxidation of Biomass
Poerwoprajitno, A.R. and Gloag, L. and Watt, J. and Cychy, S. and Cheong, S. and Kumar, P.V. and Benedetti, T.M. and Deng, C. and Wu, K.-H. and Marjo, C.E. and Huber, D.L. and Muhler, M. and Gooding, J.J. and Schuhmann, W. and Wang, D.-W. and Tilley, R.D.
ANGEWANDTE CHEMIE - INTERNATIONAL EDITION. Volume: 59 (2020) - 2020 • 6386
Thermal fatigue behavior of functionally graded W/EUROFER-layer systems using a new test apparatus
Emmerich, T. and Vaßen, R. and Aktaa, J.
FUSION ENGINEERING AND DESIGN. Volume: 154 (2020) - 2020 • 6385
The Planar Multipole Resonance Probe: A Minimally Invasive Monitoring Concept for Plasma-Assisted Dielectric Deposition Processes
Pohle, D. and Schulz, C. and Oberberg, M. and Awakowicz, P. and Rolfes, I.
IEEE TRANSACTIONS ON MICROWAVE THEORY AND TECHNIQUES. Volume: 68 (2020) - 2020 • 6384
In Situ X-ray Microscopy Reveals Particle Dynamics in a NiCo Dry Methane Reforming Catalyst under Operating Conditions
Beheshti Askari, A. and Al Samarai, M. and Morana, B. and Tillmann, L. and Pfänder, N. and Wandzilak, A. and Watts, B. and Belkhou, R. and Muhler, M. and Muhler, M. and Debeer, S.
ACS CATALYSIS. Volume: 10 (2020) - 2020 • 6383
Solid-state transformation of aqueous to organic electrolyte - Enhancing the operating voltage window of ‘in situelectrolyte’ supercapacitors
Leistenschneider, D. and Heß, L.H. and Balducci, A. and Borchardt, L.
SUSTAINABLE ENERGY AND FUELS. Volume: 4 (2020) - 2020 • 6382
Deformation mechanisms in a superelastic NiTi alloy: An in-situ high resolution digital image correlation study
Polatidis, E. and Šmíd, M. and Kuběna, I. and Hsu, W.-N. and Laplanche, G. and Van Swygenhoven, H.
MATERIALS AND DESIGN. Volume: 191 (2020) - 2020 • 6381
In situ X-ray emission and high-resolution X-ray absorption spectroscopy applied to Ni-based bimetallic dry methane reforming catalysts
Beheshti Askari, A. and Al Samarai, M. and Hiraoka, N. and Ishii, H. and Tillmann, L. and Muhler, M. and Debeer, S.
NANOSCALE. Volume: 12 (2020) - 2020 • 6380
Development progress of coating first wall components with functionally graded W/EUROFER layers on laboratory scale
Emmerich, T. and Qu, D. and Ghidersa, B.-E. and Lux, M. and Rey, J. and Vaßen, R. and Aktaa, J.
NUCLEAR FUSION. Volume: 60 (2020) - 2020 • 6379
Formic Acid-Assisted Selective Hydrogenolysis of 5-Hydroxymethylfurfural to 2,5-Dimethylfuran over Bifunctional Pd Nanoparticles Supported on N-Doped Mesoporous Carbon
Hu, B. and Warczinski, L. and Li, X. and Lu, M. and Bitzer, J. and Heidelmann, M. and Eckhard, T. and Fu, Q. and Schulwitz, J. and Merko, M. and Li, M. and Kleist, W. and Hättig, C. and Muhler, M. and Peng, B.
ANGEWANDTE CHEMIE - INTERNATIONAL EDITION. Volume: (2020) - 2020 • 6378
Tailoring grain growth and solid solution strengthening of single-phase CrCoNi medium-entropy alloys by solute selection
Hu, G.W. and Zeng, L.C. and Du, H. and Liu, X.W. and Wu, Y. and Gong, P. and Fan, Z.T. and Hu, Q. and George, E.P.
JOURNAL OF MATERIALS SCIENCE AND TECHNOLOGY. Volume: 54 (2020) - 2020 • 6377
Microstructures, Heat Treatment, and Properties of Boron-Alloyed Tool Steels
Lentz, J. and Röttger, A. and Theisen, W.
STEEL RESEARCH INTERNATIONAL. Volume: 91 (2020) - 2020 • 6376
Atomic Structure and Chemical Composition of Planar Fault Structures in Co-Base Superalloys
Lenz, M. and Wu, M. and He, J. and Makineni, S.K. and Gault, B. and Raabe, D. and Neumeier, S. and Spiecker, E.
MINERALS, METALS AND MATERIALS SERIES. Volume: (2020) - 2020 • 6375
Thin interface limit of the double-sided phase-field model with convection
Subhedar, A. and Galenko, P.K. and Varnik, F.
PHILOSOPHICAL TRANSACTIONS OF THE ROYAL SOCIETY A: MATHEMATICAL, PHYSICAL AND ENGINEERING SCIENCES. Volume: 378 (2020) - 2020 • 6374
Surface Charges at the CaF2/Water Interface Allow Very Fast Intermolecular Vibrational-Energy Transfer
Lesnicki, D. and Zhang, Z. and Bonn, M. and Sulpizi, M. and Backus, E.H.G.
ANGEWANDTE CHEMIE - INTERNATIONAL EDITION. Volume: 59 (2020) - 2020 • 6373
Diffuse interface models of solidification with convection: The choice of a finite interface thickness
Subhedar, A. and Galenko, P.K. and Varnik, F.
EUROPEAN PHYSICAL JOURNAL: SPECIAL TOPICS. Volume: 229 (2020) - 2020 • 6372
Generalized stability criterion for exploiting optimized mechanical properties by a general correlation between phase transformations and plastic deformations
Huang, L. and Lin, W. and Zhang, Y. and Feng, D. and Li, Y. and Chen, X. and Niu, K. and Liu, F.
ACTA MATERIALIA. Volume: 201 (2020) - 2020 • 6371
Correlative chemical and structural investigations of accelerated phase evolution in a nanocrystalline high entropy alloy
Li, Y.J. and Kostka, A. and Savan, A. and Ludwig, Al.
SCRIPTA MATERIALIA. Volume: 183 (2020) - 2020 • 6370
Interface tracking characteristics of color-gradient lattice Boltzmann model for immiscible fluids
Subhedar, A. and Reiter, A. and Selzer, M. and Varnik, F. and Nestler, B.
PHYSICAL REVIEW E. Volume: 101 (2020) - 2020 • 6369
Morphology, microstructure, coordinative unsaturation, and hydrogenation activity of unsupported MoS2: How idealized models fail to describe a real sulfide material
Bekx-Schürmann, S. and Mangelsen, S. and Breuninger, P. and Antoni, H. and Schürmann, U. and Kienle, L. and Muhler, M. and Bensch, W. and Grünert, W.
APPLIED CATALYSIS B: ENVIRONMENTAL. Volume: 266 (2020) - 2020 • 6368
Diffusion, defects and understanding the growth of a multicomponent interdiffusion zone between Pt-modified B2 NiAl bond coat and single crystal superalloy
Esakkiraja, N. and Gupta, A. and Jayaram, V. and Hickel, T. and Divinski, S.V. and Paul, A.
ACTA MATERIALIA. Volume: 195 (2020) - 2020 • 6367
Low-noise GaAs quantum dots for quantum photonics
Zhai, L. and Löbl, M.C. and Nguyen, G.N. and Ritzmann, J. and Javadi, A. and Spinnler, C. and Wieck, A.D. and Ludwig, Ar. and Warburton, R.J.
NATURE COMMUNICATIONS. Volume: 11 (2020) - 2020 • 6366
Compatible deformation and extra strengthening by heterogeneous nanolayer composites
Li, J. and Lu, W. and Gibson, J. and Zhang, S. and Korte-Kerzel, S. and Raabe, D.
SCRIPTA MATERIALIA. Volume: 179 (2020) - 2020 • 6365
Mapping the mechanical properties in nitride coatings at the nanometer scale
Zhang, Z. and Chen, Z. and Holec, D. and Liebscher, C.H. and Koutná, N. and Bartosik, M. and Zheng, Y. and Dehm, G. and Mayrhofer, P.H.
ACTA MATERIALIA. Volume: 194 (2020) - 2020 • 6364
Temperature-independent giant dielectric response in transitional BaTiO3 thin films
Everhardt, A.S. and Denneulin, T. and Grünebohm, A. and Shao, Y.-T. and Ondrejkovic, P. and Zhou, S. and Domingo, N. and Catalan, G. and Hlinka, J. and Zuo, J.-M. and Matzen, S. and Noheda, B.
APPLIED PHYSICS REVIEWS. Volume: 7 (2020) - 2020 • 6363
Catalytically Active Iron(IV)oxo Species Based on a Bis(pyridinyl)phenanthrolinylmethane
Hüppe, H.M. and Keisers, K. and Fink, F. and Mürtz, S.D. and Hoffmann, A. and Iffland, L. and Apfel, U.-P. and Herres-Pawlis, S.
ISRAEL JOURNAL OF CHEMISTRY. Volume: 60 (2020) - 2020 • 6362
On the assessment of creep damage evolution in nickel-based superalloys through correlative HR-EBSD and cECCI studies
Sulzer, S. and Li, Z. and Zaefferer, S. and Hafez Haghighat, S.M. and Wilkinson, A. and Raabe, D. and Reed, R.
ACTA MATERIALIA. Volume: 185 (2020) - 2020 • 6361
Electronic structure based design of thin film metallic glasses with superior fracture toughness
Evertz, S. and Kirchlechner, I. and Soler, R. and Kirchlechner, C. and Kontis, P. and Bednarcik, J. and Gault, B. and Dehm, G. and Raabe, D. and Schneider, J.M.
MATERIALS AND DESIGN. Volume: 186 (2020) - 2020 • 6360
Phase decomposition in a nanocrystalline CrCoNi alloy
Li, Y.J. and Kostka, A. and Savan, A. and Ludwig, Al.
SCRIPTA MATERIALIA. Volume: 188 (2020) - 2020 • 6359
Dependence of hydrogen embrittlement mechanisms on microstructure-driven hydrogen distribution in medium Mn steels
Sun, B. and Krieger, W. and Rohwerder, M. and Ponge, D. and Raabe, D.
ACTA MATERIALIA. Volume: 183 (2020) - 2020 • 6358
Review on Quantum Mechanically Guided Design of Ultra-Strong Metallic Glasses
Evertz, S. and Schnabel, V. and Köhler, M. and Kirchlechner, I. and Kontis, P. and Chen, Y.-T. and Soler, R. and Jaya, B.N. and Kirchlechner, C. and Music, D. and Gault, B. and Schneider, J.M. and Raabe, D. and Dehm, G.
FRONTIERS IN MATERIALS. Volume: 7 (2020) - 2020 • 6357
Mode-locked diode laser-based two-photon polymerisation
Surkamp, N. and Zyla, G. and Gurevich, E.L. and Klehr, A. and Knigge, A. and Ostendorf, A. and Hofmann, M.R.
ELECTRONICS LETTERS. Volume: 56 (2020) - 2020 • 6356
Mechanism of collective interstitial ordering in Fe–C alloys
Zhang, X. and Wang, H. and Hickel, T. and Rogal, J. and Li, Y. and Neugebauer, J.
NATURE MATERIALS. Volume: 19 (2020) - 2020 • 6355
Multicomponent diffusion of F, Cl and OH in apatite with application to magma ascent rates
Li, W. and Chakraborty, S. and Nagashima, K. and Costa, F.
EARTH AND PLANETARY SCIENCE LETTERS. Volume: 550 (2020) - 2020 • 6354
Mode-locked diode lasers as sources for two-photon polymerization
Surkamp, N. and Zyla, G. and Gurevich, E.L. and Esen, C. and Klehr, A. and Knigge, A. and Ostendorf, A. and Hofmann, M.R.
PROCEEDINGS OF SPIE - THE INTERNATIONAL SOCIETY FOR OPTICAL ENGINEERING. Volume: 11349 (2020) - 2020 • 6353
Grain boundary energy effect on grain boundary segregation in an equiatomic high-entropy alloy
Li, L. and Kamachali, R.D. and Li, Z. and Zhang, Z.
PHYSICAL REVIEW MATERIALS. Volume: 4 (2020) - 2020 • 6352
Influence of trapped gas on pore healing under hot isostatic pressing in nickel-base superalloys
Prasad, M.R.G. and Gao, S. and Vajragupta, N. and Hartmaier, A.
CRYSTALS. Volume: 10 (2020) - 2020 • 6351
Continuous wave THz system based on dual wavelength monolithic Y-branch laser diode
Surkamp, N. and Gerling, A. and O'Gorman, J. and Honsberg, M. and Schmidtmann, S. and Nandi, U. and Preu, S. and Sacher, J. and Brenner, C. and Hofmann, M.R.
INTERNATIONAL CONFERENCE ON TRANSPARENT OPTICAL NETWORKS. Volume: 2020-July (2020) - 2020 • 6350
Interplay of Chemistry and Faceting at Grain Boundaries in a Model Al Alloy
Zhao, H. and Huber, L. and Lu, W. and Peter, N.J. and An, D. and De Geuser, F. and Dehm, G. and Ponge, D. and Neugebauer, J. and Gault, B. and Raabe, D.
PHYSICAL REVIEW LETTERS. Volume: 124 (2020) - 2020 • 6349
Effect of aspect ratio on the deformation behavior of dislocation-free Ni3 Al nanocubes
Li, P. and Wang, X. and Zhou, Y. and Pfetzing-Micklich, J. and Somsen, C. and Eggeler, G.
NANOMATERIALS. Volume: 10 (2020) - 2020 • 6348
Deterministic positioning of nanophotonic waveguides around single self-assembled quantum dots
Pregnolato, T. and Chu, X.-L. and Schröder, T. and Schott, R. and Wieck, A.D. and Ludwig, Ar. and Lodahl, P. and Rotenberg, N.
APL PHOTONICS. Volume: 5 (2020) - 2020 • 6347
Amorphization-governed elasto-plastic deformation under nanoindentation in cubic (3C) silicon carbide
Zhao, L. and Alam, M. and Zhang, J. and Janisch, R. and Hartmaier, A.
CERAMICS INTERNATIONAL. Volume: 46 (2020) - 2020 • 6346
Effect of ozone stress on the intracellular metabolites from Cobetia marina
Li, J. and Rumancev, C. and Lutze, H.V. and Schmidt, T.C. and Rosenhahn, A. and Schmitz, O.J.
ANALYTICAL AND BIOANALYTICAL CHEMISTRY. Volume: 412 (2020) - 2020 • 6345
(Al, Zn)3Zr dispersoids assisted η′ precipitation in anAl-Zn-Mg-Cu-Zr alloy
Zhao, H. and Chen, Y. and Gault, B. and Makineni, S.K. and Ponge, D. and Raabe, D.
MATERIALIA. Volume: 10 (2020) - 2020 • 6344
An observer for partially obstructed wood particles in industrial drying processes
Berner, M.O. and Scherer, V. and Mönnigmann, M.
COMPUTERS AND CHEMICAL ENGINEERING. Volume: 141 (2020) - 2020 • 6343
Reversion and re-aging of a peak aged Al-Zn-Mg-Cu alloy
Zhao, H. and Gault, B. and Ponge, D. and Raabe, D.
SCRIPTA MATERIALIA. Volume: 188 (2020) - 2020 • 6342
Controllability analysis and optimal control of biomass drying with reduced order models
Berner, M.O. and Scherer, V. and Mönnigmann, M.
JOURNAL OF PROCESS CONTROL. Volume: 89 (2020) - 2020 • 6341
Induced C–C coupling in CO2 photocatalytic reduction via carbothermally reduced nonstoichiometric tungsten oxide
Li, T. and Dong, X. and Chen, W. and Zhao, X. and Li, G. and Feng, G. and Song, Y. and Wei, W. and Sun, Y.
APPLIED SURFACE SCIENCE. Volume: 526 (2020) - 2020 • 6340
Thermal cycling performances of multilayered yttria-stabilized zirconia/gadolinium zirconate thermal barrier coatings
Zhou, D. and Mack, D.E. and Bakan, E. and Mauer, G. and Sebold, D. and Guillon, O. and Vaßen, R.
JOURNAL OF THE AMERICAN CERAMIC SOCIETY. Volume: 103 (2020) - 2020 • 6339
Erratum: A critical comparison of neural network potentials for molecular reaction dynamics with exact permutation symmetry (Phys. Chem. Chem. Phys. (2019) 21 (9672-9682) DOI: 10.1039/C8CP06919K)
Li, J. and Song, K. and Behler, J.
PHYSICAL CHEMISTRY CHEMICAL PHYSICS. Volume: 22 (2020) - 2020 • 6338
Impact of electron solvation on ice structures at the molecular scale
Bertram, C. and Auburger, P. and Bockstedte, M. and Stähler, J. and Bovensiepen, U. and Morgenstern, K.
JOURNAL OF PHYSICAL CHEMISTRY LETTERS. Volume: 11 (2020) - 2020 • 6337
Identification of a multi-dimensional reaction coordinate for crystal nucleation in Ni3Al
Liang, Y. and Díaz Leines, G. and Drautz, R. and Rogal, J.
THE JOURNAL OF CHEMICAL PHYSICS. Volume: 152 (2020) - 2020 • 6336
On-Chip Nanomechanical Filtering of Quantum-Dot Single-Photon Sources
Zhou, X. and Uppu, R. and Liu, Z. and Papon, C. and Schott, R. and Wieck, A.D. and Ludwig, Ar. and Lodahl, P. and Midolo, L.
LASER AND PHOTONICS REVIEWS. Volume: 14 (2020) - 2020 • 6335
Additive-free spin coating of tin oxide thin films: Synthesis, characterization and evaluation of tin β-ketoiminates as a new precursor class for solution deposition processes
Huster, N. and Zanders, D. and Karle, S. and Rogalla, D. and Devi, A.
DALTON TRANSACTIONS. Volume: 49 (2020) - 2020 • 6334
Integrated surface gratings in VCSELs for high birefringence splitting
Pusch, T. and Debernardi, P. and Lindemann, M. and Jung, N. and Gerhardt, N.C. and Hofmann, M.R. and Michalzik, R.
PROCEEDINGS OF SPIE - THE INTERNATIONAL SOCIETY FOR OPTICAL ENGINEERING. Volume: 11356 (2020) - 2020 • 6333
Manipulation of birefringence in spin-VCSELs
Pusch, T. and Lindemann, M. and Jung, N. and Gerhardt, N.C. and Hofmann, M.R. and Michalzik, R.
PROCEEDINGS OF SPIE - THE INTERNATIONAL SOCIETY FOR OPTICAL ENGINEERING. Volume: 11470 (2020) - 2020 • 6332
Anharmonic free energy of lattice vibrations in fcc crystals from a mean-field bond
Swinburne, T.D. and Janssen, J. and Todorova, M. and Simpson, G. and Plechac, P. and Luskin, M. and Neugebauer, J.
PHYSICAL REVIEW B. Volume: 102 (2020) - 2020 • 6331
Hierarchical highly ordered SnO2 nanobowl branched ZnO nanowires for ultrasensitive and selective hydrogen sulfide gas sensing
Zhu, L.-Y. and Yuan, K.-P. and Yang, J.-H. and Hang, C.-Z. and Ma, H.-P. and Ji, X.-M. and Devi, A. and Lu, H.-L. and Zhang, D.W.
MICROSYSTEMS AND NANOENGINEERING. Volume: 6 (2020) - 2020 • 6330
Performance of the standard exchange-correlation functionals in predicting melting properties fully from first principles: Application to Al and magnetic Ni
Zhu, L.-F. and Körmann, F. and Ruban, A.V. and Neugebauer, J. and Grabowski, B.
PHYSICAL REVIEW B. Volume: 101 (2020) - 2020 • 6329
Digital holography for evaluation of the refractive index distribution externally induced in semiconductors
Besaga, V.R. and Gerhardt, N.C. and Hofmann, M.R.
PROCEEDINGS OF SPIE - THE INTERNATIONAL SOCIETY FOR OPTICAL ENGINEERING. Volume: 11306 (2020) - 2020 • 6328
Reduced-graphene-oxide-based needle-type field-effect transistor for dopamine sensing
Quast, T. and Mariani, F. and Scavetta, E. and Schuhmann, W. and Andronescu, C.
CHEMELECTROCHEM. Volume: 7 (2020) - 2020 • 6327
Electroenzymatic CO2 Fixation Using Redox Polymer/Enzyme-Modified Gas Diffusion Electrodes
Szczesny, J. and Ruff, A. and Oliveira, A.R. and Pita, M. and Pereira, I.A.C. and De Lacey, A.L. and Schuhmann, W.
ACS ENERGY LETTERS. Volume: 5 (2020) - 2020 • 6326
Origin of Laser-Induced Colloidal Gold Surface Oxidation and Charge Density, and Its Role in Oxidation Catalysis
Ziefuß, A.R. and Haxhiaj, I. and Müller, S. and Gharib, M. and Gridina, O. and Rehbock, C. and Chakraborty, I. and Peng, B. and Muhler, M. and Parak, W.J. and Barcikowski, S. and Reichenberger, S.
JOURNAL OF PHYSICAL CHEMISTRY C. Volume: 124 (2020) - 2020 • 6325
Inspection of semiconductor-based planar wave-guiding structures with a near-infrared transmission digital holographic microscopy
Besaga, V.R. and Gerhardt, N.C. and Hofmann, M.R.
PROCEEDINGS OF SPIE - THE INTERNATIONAL SOCIETY FOR OPTICAL ENGINEERING. Volume: 11369 (2020) - 2020 • 6324
Automated simulation of voxel-based microstructures based on enhanced finite cell approach
Fangye, Y.F. and Miska, N. and Balzani, D.
ARCHIVE OF APPLIED MECHANICS. Volume: 90 (2020) - 2020 • 6323
Redox-Polymer-Based High-Current-Density Gas-Diffusion H2-Oxidation Bioanode Using [FeFe] Hydrogenase from Desulfovibrio desulfuricans in a Membrane-free Biofuel Cell
Szczesny, J. and Birrell, J.A. and Conzuelo, F. and Lubitz, W. and Ruff, A. and Schuhmann, W.
ANGEWANDTE CHEMIE - INTERNATIONAL EDITION. Volume: 59 (2020) - 2020 • 6322
Measurement of Backaction from Electron Spins in a Gate-Defined GaAs Double Quantum dot Coupled to a Mesoscopic Nuclear Spin Bath
Bethke, P. and McNeil, R.P.G. and Ritzmann, J. and Botzem, T. and Ludwig, Ar. and Wieck, A.D. and Bluhm, H.
PHYSICAL REVIEW LETTERS. Volume: 125 (2020) - 2020 • 6321
Hard Cladding by Supersolidus Liquid Phase Sintering: An Experimental and Simulation Study on Martensitic Stainless Steels
Farayibi, P.K. and Blüm, M. and Weber, S.
METALLURGICAL AND MATERIALS TRANSACTIONS A: PHYSICAL METALLURGY AND MATERIALS SCIENCE. Volume: 51 (2020) - 2020 • 6320
Irreversible Structural Changes of Copper Hexacyanoferrate Used as a Cathode in Zn-Ion Batteries
Lim, J. and Kasiri, G. and Sahu, R. and Schweinar, K. and Hengge, K. and Raabe, D. and La Mantia, F. and Scheu, C.
CHEMISTRY - A EUROPEAN JOURNAL. Volume: 26 (2020) - 2020 • 6319
Influence of Pore Characteristics on Anisotropic Mechanical Behavior of Laser Powder Bed Fusion–Manufactured Metal by Micromechanical Modeling
R. G. Prasad, M. and Biswas, A. and Geenen, K. and Amin, W. and Gao, S. and Lian, J. and Röttger, A. and Vajragupta, N. and Hartmaier, A.
ADVANCED ENGINEERING MATERIALS. Volume: 22 (2020) - 2020 • 6318
Fabrication and optical characterization of photonic crystal nanocavities with electrodes for gate-defined quantum dots
Tajiri, T. and Sakai, Y. and Kuruma, K. and Ji, S.M. and Kiyama, H. and Oiwa, A. and Ritzmann, J. and Ludwig, Ar. and Wieck, A.D. and Ota, Y. and Arakawa, Y. and Iwamoto, S.
JAPANESE JOURNAL OF APPLIED PHYSICS. Volume: 59 (2020) - 2020 • 6317
Multiple character of non-monotonic size-dependence for relaxation dynamics in polymer-particle and binary mixtures
Zirdehi, E.M. and Voigtmann, T. and Varnik, F.
JOURNAL OF PHYSICS CONDENSED MATTER. Volume: 32 (2020) - 2020 • 6316
Densification of a high chromium cold work tool steel powder in different atmospheres by SLPS: Microstructure, heat treatment and micromechanical properties
Farayibi, P.K. and Blüm, M. and Weber, S.
MATERIALS SCIENCE AND ENGINEERING A. Volume: 777 (2020) - 2020 • 6315
Elasto-plastic large deformation analysis of multi-patch thin shells by isogeometric approach
Huynh, G.D. and Zhuang, X. and Bui, H.G. and Meschke, G. and Nguyen-Xuan, H.
FINITE ELEMENTS IN ANALYSIS AND DESIGN. Volume: 173 (2020) - 2020 • 6314
Computational micro-macro analysis of impact on strain-hardening cementitious composites (Shcc) including microscopic inertia
Tamsen, E. and Curosu, I. and Mechtcherine, V. and Balzani, D.
MATERIALS. Volume: 13 (2020) - 2020 • 6313
Heat treatment optimisation of supersolidus sintered steel compounds [Optimierung der Wärmebehandlung von supersolidus gesinterten Schichtverbunden]
Farayibi, P.K. and Blüm, M. and Weber, S.
HTM - JOURNAL OF HEAT TREATMENT AND MATERIALS. Volume: 75 (2020) - 2020 • 6312
Current Challenges and Opportunities in Microstructure-Related Properties of Advanced High-Strength Steels
Raabe, D. and Sun, B. and Kwiatkowski Da Silva, A. and Gault, B. and Yen, H.-W. and Sedighiani, K. and Thoudden Sukumar, P. and Souza Filho, I.R. and Katnagallu, S. and Jägle, E. and Kürnsteiner, P. and Kusampudi, N. and Stephenson, L. and Herbig, M. and Liebscher, C.H. and Springer, H. and Zaefferer, S. and Shah, V. and Wong, S.-L. and Baron, C. and Diehl, M. and Roters, F. and Ponge, D.
METALLURGICAL AND MATERIALS TRANSACTIONS A: PHYSICAL METALLURGY AND MATERIALS SCIENCE. Volume: 51 (2020) - 2020 • 6311
Joint investigation of strain partitioning and chemical partitioning in ferrite-containing TRIP-assisted steels
Tan, X. and Ponge, D. and Lu, W. and Xu, Y. and He, H. and Yan, J. and Wu, D. and Raabe, D.
ACTA MATERIALIA. Volume: 186 (2020) - 2020 • 6310
Three-dimensional numerical simulation of soft-tissue wound healing using constrained-mixture anisotropic hyperelasticity and gradient-enhanced damage mechanics
Zuo, D. and Avril, S. and Yang, H. and Jamaleddin Mousavi, S. and Hackl, K. and He, Y.
JOURNAL OF THE ROYAL SOCIETY INTERFACE. Volume: 17 (2020) - 2020 • 6309
α-Aminoisobutyric acid-stabilized peptide sams with low nonspecific protein adsorption and resistance against marine biofouling
Beyer, C.D. and Reback, M.L. and Gopal, S.M. and Nolte, K.A. and Finlay, J.A. and Clare, A.S. and Schäfer, L.V. and Metzler-Nolte, N. and Rosenhahn, A.
ACS SUSTAINABLE CHEMISTRY AND ENGINEERING. Volume: 8 (2020) - 2020 • 6308
Electrochemical CO2 and Proton Reduction by a Co(dithiacyclam) Complex
Iffland, L. and Siegmund, D. and Apfel, U.-P.
ZEITSCHRIFT FUR ANORGANISCHE UND ALLGEMEINE CHEMIE. Volume: 646 (2020) - 2020 • 6307
Performance and Cost Assessment of Machine Learning Interatomic Potentials
Zuo, Y. and Chen, C. and Li, X. and Deng, Z. and Chen, Y. and Behler, J. and Csányi, G. and Shapeev, A.V. and Thompson, A.P. and Wood, M.A. and Ong, S.P.
JOURNAL OF PHYSICAL CHEMISTRY A. Volume: 124 (2020) - 2020 • 6306
Low Fouling Peptides with an All (d) Amino Acid Sequence Provide Enhanced Stability against Proteolytic Degradation while Maintaining Low Antifouling Properties
Beyer, C.D. and Reback, M.L. and Heinen, N. and Thavalingam, S. and Rosenhahn, A. and Metzler-Nolte, N.
LANGMUIR. Volume: 36 (2020) - 2020 • 6305
Bias current and temperature dependence of polarization dynamics in spin-lasers with electrically tunable birefringence
Lindemann, M. and Jung, N. and Stadler, P. and Pusch, T. and Michalzik, R. and Hofmann, M.R. and Gerhardt, N.C.
AIP ADVANCES. Volume: 10 (2020) - 2020 • 6304
Effects of cryogenic temperature and grain size on fatigue-crack propagation in the medium-entropy CrCoNi alloy
Rackwitz, J. and Yu, Q. and Yang, Y. and Laplanche, G. and George, E.P. and Minor, A.M. and Ritchie, R.O.
ACTA MATERIALIA. Volume: 200 (2020) - 2020 • 6303
A Universal Nano-capillary Based Method of Catalyst Immobilization for Liquid-Cell Transmission Electron Microscopy
Tarnev, T. and Cychy, S. and Andronescu, C. and Muhler, M. and Schuhmann, W. and Chen, Y.-T.
ANGEWANDTE CHEMIE - INTERNATIONAL EDITION. Volume: 59 (2020) - 2020 • 6302
Sensitivity analysis of non-local damage in soft biological tissues
Zuo, D. and Avril, S. and Ran, C. and Yang, H. and Mousavi, S.J. and Hackl, K. and He, Y.
INTERNATIONAL JOURNAL FOR NUMERICAL METHODS IN BIOMEDICAL ENGINEERING. Volume: (2020) - 2020 • 6301
Ultraviolet/vacuum-ultraviolet emission from a high power magnetron sputtering plasma with an aluminum target
Iglesias, E.J. and Hecimovic, A. and Mitschker, F. and Fiebrandt, M. and Bibinov, N. and Awakowicz, P.
JOURNAL OF PHYSICS D: APPLIED PHYSICS. Volume: 53 (2020) - 2020 • 6300
Intensity and polarization dynamics in ultrafast birefringent spin-VCSELs
Lindemann, M. and Jung, N. and Burghard, M. and Pusch, T. and Xu, G. and Žutić, I. and Birkedal, D. and Michalzik, R. and Hofmann, M.R. and Gerhardt, N.C.
PROCEEDINGS OF SPIE - THE INTERNATIONAL SOCIETY FOR OPTICAL ENGINEERING. Volume: 11470 (2020) - 2020 • 6299
Surface Properties of Battery Materials Elucidated Using Scanning Electrochemical Microscopy: The Case of Type I Silicon Clathrate
Tarnev, T. and Wilde, P. and Dopilka, A. and Schuhmann, W. and Chan, C.K. and Ventosa, E.
CHEMELECTROCHEM. Volume: 7 (2020) - 2020 • 6298
Spin-lasers: spintronics beyond magnetoresistance
Žutić, I. and Xu, G. and Lindemann, M. and Faria Junior, P.E. and Lee, J. and Labinac, V. and Stojšić, K. and Sipahi, G.M. and Hofmann, M.R. and Gerhardt, N.C.
SOLID STATE COMMUNICATIONS. Volume: 316-317 (2020) - 2020 • 6297
Insight into Electron Transfer from a Redox Polymer to a Photoactive Protein
Białek, R. and Thakur, K. and Ruff, A. and Jones, M.R. and Schuhmann, W. and Ramanan, C. and Gibasiewicz, K.
JOURNAL OF PHYSICAL CHEMISTRY B. Volume: 124 (2020) - 2020 • 6296
Repair of concrete traffic areas with slim carbon reinforced concrete layers [Instandsetzung von gefugten Betonflächen mit einer dünnen Schicht aus Carbonbeton]
Farwig, K. and Neumann, J. and Schneider, R. and Breitenbücher, R. and Curbach, M.
BETON- UND STAHLBETONBAU. Volume: 115 (2020) - 2020 • 6295
Effects of Mo on the mechanical behavior of γ/γʹ-strengthened Co-Ti-based alloys
Im, H.J. and Lee, S. and Choi, W.S. and Makineni, S.K. and Raabe, D. and Ko, W.-S. and Choi, P.-P.
ACTA MATERIALIA. Volume: 197 (2020) - 2020 • 6294
Impact of nematicity on the relationship between antiferromagnetic fluctuations and superconductivity in FeSe0.91 S0.09 under pressure
Rana, K. and Xiang, L. and Wiecki, P. and Ribeiro, R.A. and Lesseux, G.G. and Böhmer, A.E. and Bud'ko, S.L. and Canfield, P.C. and Furukawa, Y.
PHYSICAL REVIEW B. Volume: 101 (2020) - 2020 • 6293
Two-photon polymerization with diode lasers emitting ultrashort pulses with high repetition rate
Zyla, G. and Surkamp, N. and Gurevich, E.L. and Esen, C. and Klehr, A. and Knigge, A. and Hofmann, M.R. and Ostendorf, A.
OPTICS LETTERS. Volume: 45 (2020) - 2020 • 6292
Unveiling the mechanism of abnormal magnetic behavior of FeNiCoMnCu high-entropy alloys through a joint experimental-theoretical study
Rao, Z. and Dutta, B. and Körmann, F. and Ponge, D. and Li, L. and He, J. and Stephenson, L. and Schäfer, L. and Skokov, K. and Gutfleisch, O. and Raabe, D. and Li, Z.
PHYSICAL REVIEW MATERIALS. Volume: 4 (2020) - 2020 • 6291
Structural colors with angle-insensitive optical properties generated by Morpho-inspired 2PP structures
Zyla, G. and Kovalev, A. and Gurevich, E.L. and Esen, C. and Liu, Y. and Lu, Y. and Gorb, S. and Ostendorf, A.
APPLIED PHYSICS A: MATERIALS SCIENCE AND PROCESSING. Volume: 126 (2020) - 2020 • 6290
Beyond Solid Solution High-Entropy Alloys: Tailoring Magnetic Properties via Spinodal Decomposition
Rao, Z. and Dutta, B. and Körmann, F. and Lu, W. and Zhou, X. and Liu, C. and da Silva, A.K. and Wiedwald, U. and Spasova, M. and Farle, M. and Ponge, D. and Gault, B. and Neugebauer, J. and Raabe, D. and Li, Z.
ADVANCED FUNCTIONAL MATERIALS. Volume: (2020) - 2020 • 6289
Tuning Coordination Geometry of Nickel Ketoiminates and Its Influence on Thermal Characteristics for Chemical Vapor Deposition of Nanostructured NiO Electrocatalysts
Zywitzki, D. and Taffa, D.H. and Lamkowski, L. and Winter, M. and Rogalla, D. and Wark, M. and Devi, A.
INORGANIC CHEMISTRY. Volume: 59 (2020) - 2020 • 6288
Micro-, macromechanical and aeroelastic investigation of glass - fiber based, lightweight turbomachinery components
Iseni, S. and Prasad, M.R.G. and Hartmaier, A. and Holeczek, K. and Modeler, N. and di Mare, F.
PROCEEDINGS OF THE ASME TURBO EXPO. Volume: 10A-2020 (2020) - 2020 • 6287
Terahertz beam steering concept based on a MEMS-reconfigurable reflection grating
Liu, X. and Samfaß, L. and Kolpatzeck, K. and Häring, L. and Balzer, J.C. and Hoffmann, M. and Czylwik, A.
SENSORS (SWITZERLAND). Volume: 20 (2020) - 2020 • 6286
Model-Based Analysis of the Photocatalytic HCl Oxidation Kinetics over TiO2
Rath, T. and Bloh, J.Z. and Lüken, A. and Ollegott, K. and Muhler, M.
INDUSTRIAL AND ENGINEERING CHEMISTRY RESEARCH. Volume: 59 (2020) - 2020 • 6285
Advanced broadband MEMS infrared emitter based on high-temperature-resistant nanostructured surfaces and packaging solutions for harsh environments
Biermann, S. and Magi, A. and Sachse, P. and Hoffmann, M. and Wedrich, K. and Müller, L. and Koppert, R. and Ortlepp, T. and Baldauf, J.
PROCEEDINGS OF SPIE - THE INTERNATIONAL SOCIETY FOR OPTICAL ENGINEERING. Volume: 11279 (2020) - 2020 • 6284
Correlation analysis of strongly fluctuating atomic volumes, charges, and stresses in body-centered cubic refractory high-entropy alloys
Ishibashi, S. and Ikeda, Y. and Körmann, F. and Grabowski, B. and Neugebauer, J.
PHYSICAL REVIEW MATERIALS. Volume: 4 (2020) - 2020 • 6283
On the role of chemical heterogeneity in phase transformations and mechanical behavior of flash annealed quenching & partitioning steels
Liu, G. and Li, T. and Yang, Z. and Zhang, C. and Li, J. and Chen, H.
ACTA MATERIALIA. Volume: 201 (2020) - 2020 • 6282
Iodine-Catalyzed Selective Functionalization of Ethane in Oleum: Toward a Direct Process for the Production of Ethylene Glycol from Shale Gas
Bilke, M. and Zimmermann, T. and Schüth, F.
JOURNAL OF THE AMERICAN CHEMICAL SOCIETY. Volume: 142 (2020) - 2020 • 6281
Ultrafast Construction of Oxygen-Containing Scaffold over Graphite for Trapping Ni2+into Single Atom Catalysts
Liu, Z. and Li, S. and Yang, J. and Tan, X. and Yu, C. and Zhao, C. and Han, X. and Huang, H. and Wan, G. and Liu, Y. and Tschulik, K. and Qiu, J.
ACS NANO. Volume: 14 (2020) - 2020 • 6280
Real-Time Detection of Single Auger Recombination Events in a Self-Assembled Quantum Dot
Lochner, P. and Kurzmann, A. and Kerski, J. and Stegmann, P. and König, J. and Wieck, A.D. and Ludwig, Ar. and Lorke, A. and Geller, M.
NANO LETTERS. Volume: 20 (2020) - 2020 • 6279
Approaches to Measure the Resistivity of Grain Boundaries in Metals with High Sensitivity and Spatial Resolution: A Case Study Employing Cu
Bishara, H. and Ghidelli, M. and Dehm, G.
ACS APPLIED ELECTRONIC MATERIALS. Volume: 2 (2020) - 2020 • 6278
Radiative Auger process in the single-photon limit
Löbl, M.C. and Spinnler, C. and Javadi, A. and Zhai, L. and Nguyen, G.N. and Ritzmann, J. and Midolo, L. and Lodahl, P. and Wieck, A.D. and Ludwig, Ar. and Warburton, R.J.
NATURE NANOTECHNOLOGY. Volume: 15 (2020) - 2020 • 6277
Regularization parameter selection in indirect regression by residual based bootstrap
Bissantz, N. and Chown, J. and Dette, H.
STATISTICA SINICA. Volume: 30 (2020) - 2020 • 6276
Design of Complex Solid-Solution Electrocatalysts by Correlating Configuration, Adsorption Energy Distribution Patterns, and Activity Curves
Löffler, T. and Savan, A. and Meyer, H. and Meischein, M. and Strotkötter, V. and Ludwig, Al. and Schuhmann, W.
ANGEWANDTE CHEMIE - INTERNATIONAL EDITION. Volume: 59 (2020) - 2020 • 6275
Effect of Grain Statistics on Micromechanical Modeling: The Example of Additively Manufactured Materials Examined by Electron Backscatter Diffraction
Biswas, A. and Prasad, M.R.G. and Vajragupta, N. and Kostka, A. and Niendorf, T. and Hartmaier, A.
ADVANCED ENGINEERING MATERIALS. Volume: 22 (2020) - 2020 • 6274
Thermal Spray Processes for the Repair of Gas Turbine Components
Fiebig, J. and Bakan, E. and Kalfhaus, T. and Mauer, G. and Guillon, O. and Vaßen, R.
ADVANCED ENGINEERING MATERIALS. Volume: 22 (2020) - 2020 • 6273
New Model–Based Bioequivalence Statistical Approaches for Pharmacokinetic Studies with Sparse Sampling
Loingeville, F. and Bertrand, J. and Nguyen, T.T. and Sharan, S. and Feng, K. and Sun, W. and Han, J. and Grosser, S. and Zhao, L. and Fang, L. and Möllenhoff, K. and Dette, H. and Mentré, F.
AAPS JOURNAL. Volume: 22 (2020) - 2020 • 6272
Elucidating the dual role of grain boundaries as dislocation sources and obstacles and its impact on toughness and brittle-to-ductile transition
Reiser, J. and Hartmaier, A.
SCIENTIFIC REPORTS. Volume: 10 (2020) - 2020 • 6271
Optimized reconstruction of the crystallographic orientation density function based on a reduced set of orientations Reconstruction of the orientation density function
Biswas, A. and Vajragupta, N. and Hielscher, R. and Hartmaier, A.
JOURNAL OF APPLIED CRYSTALLOGRAPHY. Volume: 53 (2020) - 2020 • 6270
A simple Peltier cold trap aperture for protection of vacuum UV optics against hydrocarbons and reliable calibration of VUV spectrometers using D2 lamps
Fiebrandt, M. and Awakowicz, P.
MEASUREMENT SCIENCE AND TECHNOLOGY. Volume: 31 (2020) - 2020 • 6269
Experimental Evidence for the Incorporation of Two Metals at Equivalent Lattice Positions in Mixed-Metal Metal–Organic Frameworks
Bitzer, J. and Otterbach, S. and Thangavel, K. and Kultaeva, A. and Schmid, R. and Pöppl, A. and Kleist, W.
CHEMISTRY - A EUROPEAN JOURNAL. Volume: 26 (2020) - 2020 • 6268
Determination of atomic oxygen state densities in a double inductively coupled plasma using optical emission and absorption spectroscopy and probe measurements
Fiebrandt, M. and Bibinov, N. and Awakowicz, P.
PLASMA SOURCES SCIENCE AND TECHNOLOGY. Volume: 29 (2020) - 2020 • 6267
Light-controlled imaging of biocatalytic reactionsviascanning photoelectrochemical microscopy for multiplexed sensing
Riedel, M. and Ruff, A. and Schuhmann, W. and Lisdat, F. and Conzuelo, F.
CHEMICAL COMMUNICATIONS. Volume: 56 (2020) - 2020 • 6266
Sustainable and rapid preparation of nanosized Fe/Ni-pentlandite particles by mechanochemistry
Tetzlaff, D. and Pellumbi, K. and Baier, D.M. and Hoof, L. and Shastry Barkur, H. and Smialkowski, M. and Amin, H.M.A. and Grätz, S. and Siegmund, D. and Borchardt, L. and Apfel, U.-P.
CHEMICAL SCIENCE. Volume: 11 (2020) - 2020 • 6265
Upcycling strategy of grinding swarf by supersolidus liquid phase sintering
Jäger, S. and Weber, S.
PROCEDIA CIRP. Volume: 90 (2020) - 2020 • 6264
Shedding Light on Proton and Electron Dynamics in [FeFe] Hydrogenases
Lorent, C. and Katz, S. and Duan, J. and Kulka, C.J. and Caserta, G. and Teutloff, C. and Yadav, S. and Apfel, U.-P. and Winkler, M. and Happe, T. and Horch, M. and Zebger, I.
JOURNAL OF THE AMERICAN CHEMICAL SOCIETY. Volume: 142 (2020) - 2020 • 6263
Characterization of Dielectric Materials by Sparse Signal Processing with Iterative Dictionary Updates
Thanthrige, U.S.K.P.M. and Barowski, J. and Rolfes, I. and Erni, D. and Kaiser, T. and Sezgin, A.
IEEE SENSORS LETTERS. Volume: 4 (2020) - 2020 • 6262
An automated and continuous method for the optical measurement of wood pellet size distribution and the gravimetric determination of fines
Jägers, J. and Wirtz, S. and Scherer, V.
POWDER TECHNOLOGY. Volume: 367 (2020) - 2020 • 6261
Experimental analysis of wood pellet degradation during pneumatic conveying processes
Jägers, J. and Wirtz, S. and Scherer, V. and Behr, M.
POWDER TECHNOLOGY. Volume: 359 (2020) - 2020 • 6260
Three-field mixed finite element formulations for gradient elasticity at finite strains
Riesselmann, J. and Ketteler, J.W. and Schedensack, M. and Balzani, D.
GAMM MITTEILUNGEN. Volume: 43 (2020) - 2020 • 6259
Implications of resistance and mass transport limitations on the common Tafel approach at composite catalyst thin-film electrodes
Blanc, N. and Rurainsky, C. and Tschulik, K.
JOURNAL OF ELECTROANALYTICAL CHEMISTRY. Volume: 872 (2020) - 2020 • 6258
Excess noise in Al x Ga 1 - X As/GaAs based quantum rings
Riha, C. and Buchholz, S.S. and Chiatti, O. and Wieck, A.D. and Reuter, D. and Fischer, S.F.
APPLIED PHYSICS LETTERS. Volume: 117 (2020) - 2020 • 6257
Thermodynamic modelling of the Ni–Zr system
Jana, A. and Sridar, S. and Fries, S.G. and Hammerschmidt, T. and Kumar, K.C.H.
INTERMETALLICS. Volume: 116 (2020) - 2020 • 6256
Enhancing Electrogenerated Chemiluminescence on Platinum Electrodes through Surface Modification
Fiorani, A. and Eßmann, V. and Santos, C.S. and Schuhmann, W.
CHEMELECTROCHEM. Volume: 7 (2020) - 2020 • 6255
Interfacial nanophases stabilize nanotwins in high-entropy alloys
Lu, W. and Liebscher, C.H. and Yan, F. and Fang, X. and Li, L. and Li, J. and Guo, W. and Dehm, G. and Raabe, D. and Li, Z.
ACTA MATERIALIA. Volume: 185 (2020) - 2020 • 6254
Theory of strain-induced magnetic order and splitting of Tc and TTRSB in Sr2Ru O4
Rømer, A.T. and Kreisel, A. and Müller, M.A. and Hirschfeld, P.J. and Eremin, I.M. and Andersen, B.M.
PHYSICAL REVIEW B. Volume: 102 (2020) - 2020 • 6253
Tailoring the Size, Inversion Parameter, and Absorption of Phase-Pure Magnetic MgFe2O4Nanoparticles for Photocatalytic Degradations
Bloesser, A. and Kurz, H. and Timm, J. and Wittkamp, F. and Simon, C. and Hayama, S. and Weber, B. and Apfel, U.-P. and Marschall, R.
ACS APPLIED NANO MATERIALS. Volume: 3 (2020) - 2020 • 6252
Accurate Global Potential Energy Surfaces for the H + CH3OH Reaction by Neural Network Fitting with Permutation Invariance
Lu, D. and Behler, J. and Li, J.
JOURNAL OF PHYSICAL CHEMISTRY A. Volume: 124 (2020) - 2020 • 6251
Linking Fluid Densimetry and Molecular Simulation: Adsorption Behavior of Carbon Dioxide on Planar Gold Surfaces
Tietz, C. and Sekulla, M. and Yang, X. and Schmid, R. and Richter, M.
INDUSTRIAL AND ENGINEERING CHEMISTRY RESEARCH. Volume: 59 (2020) - 2020 • 6250
Frontiers in molecular simulation of solvated ions, molecules and interfaces
Blumberger, J. and Gaigeot, M.-P. and Sulpizi, M. and Vuilleumier, R.
PHYSICAL CHEMISTRY CHEMICAL PHYSICS. Volume: 22 (2020) - 2020 • 6249
Topology optimization with anisotropic materials, including a filter to smooth fiber pathways
Jantos, D.R. and Hackl, K. and Junker, P.
STRUCTURAL AND MULTIDISCIPLINARY OPTIMIZATION. Volume: 61 (2020) - 2020 • 6248
Spin-glass phase transition revealed in transport measurements
Forestier, G. and Solana, M. and Naud, C. and Wieck, A.D. and Lefloch, F. and Whitney, R. and Carpentier, D. and Lévy, L.P. and Saminadayar, L.
PHYSICAL REVIEW B. Volume: 102 (2020) - 2020 • 6247
Comparative study of the residual stress development in HMDSN-based organosilicon and silicon oxide coatings
Jaritz, M. and Hopmann, C. and Wilski, S. and Kleines, L. and Banko, L. and Grochla, D. and Ludwig, Al. and Dahlmann, R.
JOURNAL OF PHYSICS D: APPLIED PHYSICS. Volume: 53 (2020) - 2020 • 6246
Scalable Fabrication of Biophotoelectrodes by Means of Automated Airbrush Spray-Coating
Bobrowski, T. and Conzuelo, F. and Ruff, A. and Hartmann, V. and Frank, A. and Erichsen, T. and Nowaczyk, M.M. and Schuhmann, W.
CHEMPLUSCHEM. Volume: 85 (2020) - 2020 • 6245
HMDSO-Based Thin Plasma Polymers as Corrosion Barrier Against NaOH Solution
Jaritz, M. and Hopmann, C. and Wilski, S. and Kleines, L. and Rudolph, M. and Awakowicz, P. and Dahlmann, R.
JOURNAL OF MATERIALS ENGINEERING AND PERFORMANCE. Volume: 29 (2020) - 2020 • 6244
Guest Editorial
Rolfes, I. and Pohl, N.
IEEE TRANSACTIONS ON MICROWAVE THEORY AND TECHNIQUES. Volume: 68 (2020) - 2020 • 6243
Nanocrystalline Ga-Zn Oxynitride Materials: Minimized Defect Density for Improved Photocatalytic Activity?
Lukic, S. and Busser, G.W. and Zhang, S. and Menze, J. and Muhler, M. and Scheu, C. and Winterer, M.
ZEITSCHRIFT FUR PHYSIKALISCHE CHEMIE. Volume: 234 (2020) - 2020 • 6242
The 2019 IEEE MTT-S International Microwave Workshop Series on Advanced Materials and Processes for RF and THz Applications [Conference Reports]
Rolfes, I.
IEEE MICROWAVE MAGAZINE. Volume: 21 (2020) - 2020 • 6241
Crystal structure and composition dependence of mechanical properties of single-crystalline NbCo2 Laves phase
Luo, W. and Kirchlechner, C. and Zavašnik, J. and Lu, W. and Dehm, G. and Stein, F.
ACTA MATERIALIA. Volume: 184 (2020) - 2020 • 6240
Anode spots of low current gliding arc plasmatron
Böddeker, S. and Bracht, V. and Hermanns, P. and Gröger, S. and Kogelheide, F. and Bibinov, N. and Awakowicz, P.
PLASMA SOURCES SCIENCE AND TECHNOLOGY. Volume: 29 (2020) - 2020 • 6239
Multilevel surrogate modeling approach for optimization problems with polymorphic uncertain parameters
Freitag, S. and Edler, P. and Kremer, K. and Meschke, G.
INTERNATIONAL JOURNAL OF APPROXIMATE REASONING. Volume: 119 (2020) - 2020 • 6238
Ultra-bright source of indistinguishable single photons
Javadi, A. and Tomm, N. and Antoniadis, N.O. and Najer, D. and Löbl, M.C. and Korsch, A. and Schott, R. and Valentin, S.R. and Wieck, A.D. and Ludwig, Ar. and Warburton, R.J.
OPTICS INFOBASE CONFERENCE PAPERS. Volume: (2020) - 2020 • 6237
Composition dependence of hardness and elastic modulus of the cubic and hexagonal NbCo2 Laves phase polytypes studied by nanoindentation
Luo, W. and Kirchlechner, C. and Li, J. and Dehm, G. and Stein, F.
JOURNAL OF MATERIALS RESEARCH. Volume: 35 (2020) - 2020 • 6236
Structural reliability and durability assessment of reinforced concrete structures
Freitag, S. and Kremer, K. and Edler, P. and Hofmann, M. and Meschke, G.
PROCEEDINGS OF THE 29TH EUROPEAN SAFETY AND RELIABILITY CONFERENCE, ESREL 2019. Volume: (2020) - 2020 • 6235
Characterization of low-resistance ohmic contacts to a two-dimensional electron gas in a GaAs/AlGaAs heterostructure
Javaid Iqbal, M. and Reuter, D. and Wieck, A.D. and Van Der Wal, C.
EPJ APPLIED PHYSICS. Volume: 89 (2020) - 2020 • 6234
A strong and ductile medium-entropy alloy resists hydrogen embrittlement and corrosion
Luo, H. and Sohn, S.S. and Lu, W. and Li, L. and Li, X. and Soundararajan, C.K. and Krieger, W. and Li, Z. and Raabe, D.
NATURE COMMUNICATIONS. Volume: 11 (2020) - 2020 • 6233
On the Importance of Structural and Functional Fatigue in Shape Memory Technology
Frenzel, J.
SHAPE MEMORY AND SUPERELASTICITY. Volume: (2020) - 2020 • 6232
Millimeterwave Radar Systems for In-Line Thickness Monitoring in Pipe Extrusion Production Lines
Jebramcik, J. and Rolfes, I. and Pohl, N. and Barowski, J.
IEEE SENSORS LETTERS. Volume: 4 (2020) - 2020 • 6231
Generalized dipole correction for charged surfaces in the repeated-slab approach
Freysoldt, C. and Mishra, A. and Ashton, M. and Neugebauer, J.
PHYSICAL REVIEW B. Volume: 102 (2020) - 2020 • 6230
Sequencing of metals in multivariate metal-organic frameworks
Ji, Z. and Li, T. and Yaghi, O.M.
SCIENCE. Volume: 369 (2020) - 2020 • 6229
Phase-sensitive determination of nodal d-wave order parameter in single-band and multiband superconductors
Böker, J. and Sulangi, M.A. and Akbari, A. and Davis, J.C.S. and Hirschfeld, P.J. and Eremin, I.M.
PHYSICAL REVIEW B. Volume: 101 (2020) - 2020 • 6228
Phase boundary segregation-induced strengthening and discontinuous yielding in ultrafine-grained duplex medium-Mn steels
Ma, Y. and Sun, B. and Schökel, A. and Song, W. and Ponge, D. and Raabe, D. and Bleck, W.
ACTA MATERIALIA. Volume: 200 (2020) - 2020 • 6227
Synergistic Effect of Molybdenum and Tungsten in Highly Mixed Carbide Nanoparticles as Effective Catalysts in the Hydrogen Evolution Reaction under Alkaline and Acidic Conditions
Fu, Q. and Peng, B. and Masa, J. and Chen, Y.-T. and Xia, W. and Schuhmann, W. and Muhler, M.
CHEMELECTROCHEM. Volume: 7 (2020) - 2020 • 6226
Method for conducting in situ high-temperature digital image correlation with simultaneous synchrotron measurements under thermomechanical conditions
Rossmann, L. and Sarley, B. and Hernandez, J. and Kenesei, P. and Köster, A. and Wischek, J. and Almer, J. and Maurel, V. and Bartsch, M. and Raghavan, S.
REVIEW OF SCIENTIFIC INSTRUMENTS. Volume: 91 (2020) - 2020 • 6225
Walter Thiel (1949-2019)
Fürstner, A. and List, B. and Ritter, T. and Schüth, F. and Neese, F.
ANGEWANDTE CHEMIE (INTERNATIONAL ED. IN ENGLISH). Volume: 59 (2020) - 2020 • 6224
Performance of wear resistant MCrAlY coatings with oxide dispersion strengthening
Bolelli, G. and Vorkötter, C. and Lusvarghi, L. and Morelli, S. and Testa, V. and Vaßen, R.
WEAR. Volume: 444-445 (2020) - 2020 • 6223
Frequency-Coded mm-Wave Tags for Self-Localization System Using Dielectric Resonators
Jiménez-Sáez, A. and Alhaj-Abbas, A. and Schüßler, M. and Abuelhaija, A. and El-Absi, M. and Sakaki, M. and Samfaß, L. and Benson, N. and Hoffmann, M. and Jakoby, R. and Kaiser, T. and Solbach, K.
JOURNAL OF INFRARED, MILLIMETER, AND TERAHERTZ WAVES. Volume: 41 (2020) - 2020 • 6222
Drug Release from Polymer Thin Films and Gel Pellets: Insights from Programmed Microplate Electroanalysis
Ruff, A. and Jaikaew, W. and Khunkaewla, P. and Schuhmann, W. and Schulte, A.
CHEMPLUSCHEM. Volume: 85 (2020) - 2020 • 6221
Estimation of the relative permittivity from the molecular structure of polymers used in automotive industries
Bonfig, T. and Körner, E. and Jebramcik, J. and Kroll, L. and Rolfes, I. and Jan Barowski
MATERIALS RESEARCH EXPRESS. Volume: 7 (2020) - 2020 • 6220
From Precursor Chemistry to Gas Sensors: Plasma-Enhanced Atomic Layer Deposition Process Engineering for Zinc Oxide Layers from a Nonpyrophoric Zinc Precursor for Gas Barrier and Sensor Applications
Mai, L. and Mitschker, F. and Bock, C. and Niesen, A. and Ciftyurek, E. and Rogalla, D. and Mickler, J. and Erig, M. and Li, Z. and Awakowicz, P. and Schierbaum, K. and Devi, A.
SMALL. Volume: 16 (2020) - 2020 • 6219
Bioelectrocatalysis as the basis for the design of enzyme-based biofuel cells and semi-artificial biophotoelectrodes
Ruff, A. and Conzuelo, F. and Schuhmann, W.
NATURE CATALYSIS. Volume: 3 (2020) - 2020 • 6218
The brittle-to-ductile transition in cold-rolled tungsten sheets: the rate-limiting mechanism of plasticity controlling the BDT in ultrafine-grained tungsten
Bonnekoh, C. and Reiser, J. and Hartmaier, A. and Bonk, S. and Hoffmann, A. and Rieth, M.
JOURNAL OF MATERIALS SCIENCE. Volume: 55 (2020) - 2020 • 6217
Impermeable Charge Transport Layers Enable Aqueous Processing on Top of Perovskite Solar Cells
Gahlmann, T. and Brinkmann, K.O. and Becker, T. and Tückmantel, C. and Kreusel, C. and van gen Hassend, F. and Weber, S. and Riedl, T.
ADVANCED ENERGY MATERIALS. Volume: 10 (2020) - 2020 • 6216
A dinuclear porphyrin-macrocycle as efficient catalyst for the hydrogen evolution reaction
Jökel, J. and Schwer, F. and Von Delius, M. and Apfel, U.-P.
CHEMICAL COMMUNICATIONS. Volume: 56 (2020) - 2020 • 6215
Redox-Polymer-Wired [NiFeSe] Hydrogenase Variants with Enhanced O2 Stability for Triple-Protected High-Current-Density H2-Oxidation Bioanodes
Ruff, A. and Szczesny, J. and Vega, M. and Zacarias, S. and Matias, P.M. and Gounel, S. and Mano, N. and Pereira, I.A.C. and Schuhmann, W.
CHEMSUSCHEM. Volume: 13 (2020) - 2020 • 6214
Deciphering the complex crystallography of a strained two-phase alloy using high-resolution STEM and molecular dynamics calculations
Borisevich, A. and Zarkadoula, E. and Yang, Y. and George, E.
MICROSCOPY AND MICROANALYSIS. Volume: (2020) - 2020 • 6213
Structure and dynamics of solid/liquid interfaces
Gaigeot, M.-P. and Sulpizi, M.
SURFACE AND INTERFACE SCIENCE, VOLUME 7: SOLID-LIQUID AND BIOLOGICAL INTERFACES. Volume: (2020) - 2020 • 6212
Soft X-ray diffraction patterns measured by a LiF detector with sub-micrometre resolution and an ultimate dynamic range
Makarov, S. and Pikuz, S. and Ryazantsev, S. and Pikuz, T. and Buzmakov, A. and Rose, M. and Lazarev, S. and Senkbeil, T. and Von Gundlach, A. and Stuhr, S. and Rumancev, C. and Dzhigaev, D. and Skopintsev, P. and Zaluzhnyy, I. and Viefhaus, J. and Rosenhahn, A. and Kodama, R. and Vartanyants, I.A.
JOURNAL OF SYNCHROTRON RADIATION. Volume: 27 (2020) - 2020 • 6211
Atomistic simulations of thermal conductivity in GeTe nanowires
Bosoni, E. and Campi, D. and Donadio, D. and Sosso, G.C. and Behler, J. and Bernasconi, M.
JOURNAL OF PHYSICS D: APPLIED PHYSICS. Volume: 53 (2020) - 2020 • 6210
Insights into the mechanochemical synthesis of Sn-β: Solid-state metal incorporation in beta zeolite
Joshi, H. and Ochoa-Hernández, C. and Nürenberg, E. and Kang, L. and Wang, F.R. and Weidenthaler, C. and Schmidt, W. and Schüth, F.
MICROPOROUS AND MESOPOROUS MATERIALS. Volume: 309 (2020) - 2020 • 6209
CO2 Hydrogenation with Cu/ZnO/Al2O3: A Benchmark Study
Ruland, H. and Song, H. and Laudenschleger, D. and Stürmer, S. and Schmidt, S. and He, J. and Kähler, K. and Muhler, M. and Schlögl, R.
CHEMCATCHEM. Volume: 12 (2020) - 2020 • 6208
Electrochemical dealloying as a tool to tune the porosity, composition and catalytic activity of nanomaterials
Rurainsky, C. and Manjón, A.G. and Hiege, F. and Chen, Y.-T. and Scheu, C. and Tschulik, K.
JOURNAL OF MATERIALS CHEMISTRY A. Volume: 8 (2020) - 2020 • 6207
A carbene stabilized precursor for the spatial atomic layer deposition of copper thin films
Boysen, N. and Misimi, B. and Muriqi, A. and Wree, J.-L. and Hasselmann, T. and Rogalla, D. and Haeger, T. and Theirich, D. and Nolan, M. and Riedl, T. and Devi, A.
CHEMICAL COMMUNICATIONS. Volume: 56 (2020) - 2020 • 6206
Parallel finite cell method with adaptive geometric multigrid
Saberi, S. and Vogel, A. and Meschke, G.
LECTURE NOTES IN COMPUTER SCIENCE (INCLUDING SUBSERIES LECTURE NOTES IN ARTIFICIAL INTELLIGENCE AND LECTURE NOTES IN BIOINFORMATICS). Volume: 12247 LNCS (2020) - 2020 • 6205
Bulk nanostructured AlCoCrFeMnNi chemically complex alloy synthesized by laser-powder bed fusion
Jung, H.Y. and Peter, N.J. and Gärtner, E. and Dehm, G. and Uhlenwinkel, V. and Jägle, E.A.
ADDITIVE MANUFACTURING. Volume: 35 (2020) - 2020 • 6204
Influence of rafted microstructures on creep in Ni-base single crystal superalloys: A 3D discrete dislocation dynamics study
Gao, S. and Ali, M.A. and Hartmaier, A.
MODELLING AND SIMULATION IN MATERIALS SCIENCE AND ENGINEERING. Volume: 28 (2020) - 2020 • 6203
Investigation of the polarization state in spin-VCSELs with thermally tuned birefringence
Jung, N. and Lindemann, M. and Stadler, P. and Pusch, T. and Michalzik, R. and Hofmann, M.R. and Gerhardt, N.C.
PROCEEDINGS OF SPIE - THE INTERNATIONAL SOCIETY FOR OPTICAL ENGINEERING. Volume: 11356 (2020) - 2020 • 6202
Transferability of neural network potentials for varying stoichiometry: Phonons and thermal conductivity of MnxGeycompounds
Mangold, C. and Chen, S. and Barbalinardo, G. and Behler, J. and Pochet, P. and Termentzidis, K. and Han, Y. and Chaput, L. and Lacroix, D. and Donadio, D.
JOURNAL OF APPLIED PHYSICS. Volume: 127 (2020) - 2020 • 6201
Solute hydrogen and deuterium observed at the near atomic scale in high-strength steel
Breen, A.J. and Stephenson, L.T. and Sun, B. and Li, Y. and Kasian, O. and Raabe, D. and Herbig, M. and Gault, B.
ACTA MATERIALIA. Volume: 188 (2020) - 2020 • 6200
Assessing the Influence of Supercritical Carbon Dioxide on the Electrochemical Reduction to Formic Acid Using Carbon-Supported Copper Catalysts
Junge Puring, K. and Evers, O. and Prokein, M. and Siegmund, D. and Scholten, F. and Mölders, N. and Renner, M. and Roldan Cuenya, B. and Petermann, M. and Weidner, E. and Apfel, U.-P.
ACS CATALYSIS. Volume: 10 (2020) - 2020 • 6199
Sputter deposition of highly active complex solid solution electrocatalysts into an ionic liquid library: Effect of structure and composition on oxygen reduction activity
Manjón, A.G. and Löffler, T. and Meischein, M. and Meyer, H. and Lim, J. and Strotkötter, V. and Schuhmann, W. and Ludwig, Al. and Scheu, C.
NANOSCALE. Volume: 12 (2020) - 2020 • 6198
Enhanced dissolution of silver nanoparticles in a physical mixture with platinum nanoparticles based on the sacrificial anode effect
Breisch, M. and Loza, K. and Pappert, K. and Rostek, A. and Rurainsky, C. and Tschulik, K. and Heggen, M. and Epple, M. and Tiller, J.C. and Schildhauer, T.A. and Köller, M. and Sengstock, C.
NANOTECHNOLOGY. Volume: 31 (2020) - 2020 • 6197
A Laser Written 4D Optical Microcavity for Advanced Biochemical Sensing in Aqueous Environment
Saetchnikov, A.V. and Tcherniavskaia, E.A. and Saetchnikov, V.A. and Ostendorf, A.
JOURNAL OF LIGHTWAVE TECHNOLOGY. Volume: 38 (2020) - 2020 • 6196
A new variational approach for the thermodynamic topology optimization of hyperelastic structures
Junker, P. and Balzani, D.
COMPUTATIONAL MECHANICS. Volume: (2020) - 2020 • 6195
Application of dispersed microresonator based sensor for aerospace-related tasks
Saetchnikov, A. and Tcherniavskaia, E. and Saetchnikov, V. and Ostendorf, A.
2020 IEEE INTERNATIONAL WORKSHOP ON METROLOGY FOR AEROSPACE, METROAEROSPACE 2020 - PROCEEDINGS. Volume: (2020) - 2020 • 6194
Comparison of cryogenic deformation of the concentrated solid solutions CoCrFeMnNi, CoCrNi and CoNi
Tirunilai, A.S. and Hanemann, T. and Reinhart, C. and Tschan, V. and Weiss, K.-P. and Laplanche, G. and Freudenberger, J. and Heilmaier, M. and Kauffmann, A.
MATERIALS SCIENCE AND ENGINEERING A. Volume: 783 (2020) - 2020 • 6193
Simulation and optimization of the design of focusing dielectric lenses based on cartesian ovals with physical optics
Garten, O. and Barowski, J. and Rolfes, I.
2020 INTERNATIONAL WORKSHOP ON ANTENNA TECHNOLOGY, IWAT 2020. Volume: (2020) - 2020 • 6192
Needle-type organic electrochemical transistor for spatially resolved detection of dopamine
Mariani, F. and Quast, T. and Andronescu, C. and Gualandi, I. and Fraboni, B. and Tonelli, D. and Scavetta, E. and Schuhmann, W.
MICROCHIMICA ACTA. Volume: 187 (2020) - 2020 • 6191
Design and application of distributed microresonator-based systems for biochemical sensing
Saetchnikov, A. and Tcherniavskaia, E. and Saetchnikov, V. and Ostendorf, A.
PROCEEDINGS OF SPIE - THE INTERNATIONAL SOCIETY FOR OPTICAL ENGINEERING. Volume: 11354 (2020) - 2020 • 6190
German Catalysis Society Meeting: Exploring the Various Facets of Catalysis
Titus, J. and Harth, F. and Eckert, R. and Mei, B.T. and Franken, T.
CHEMCATCHEM. Volume: 12 (2020) - 2020 • 6189
Considerations regarding simulator design for electromagnetic measurement systems
Garten, O. and Barowski, J. and Rolfes, I.
PROCEEDINGS OF THE 2020 IEEE INTERNATIONAL CONFERENCE ON COMPUTATIONAL ELECTROMAGNETICS, ICCEM 2020. Volume: (2020) - 2020 • 6188
Ab initio investigation of electronic and magnetic properties of antiferromagnetic/ferroelectric LaMnO3/BaTiO3interface
Kabanov, V.V. and Piyanzina, I.I. and Lysogorskiy, Yu.V. and Tayurskii, D.A. and Mamin, R.F.
MATERIALS RESEARCH EXPRESS. Volume: 7 (2020) - 2020 • 6187
Deep-learning powered whispering gallery mode sensor based on multiplexed imaging at fixed frequency
Saetchnikov, A.V. and Tcherniavskaia, E.A. and Saetchnikov, V.A. and Ostendorf, A.
OPTO-ELECTRONIC ADVANCES. Volume: 3 (2020) - 2020 • 6186
Improved Adhesion of Different Environmental Barrier Coatings on Al2O3/Al2O3-Ceramic Matrix Composites
Gatzen, C. and Mack, D.E. and Guillon, O. and Vaßen, R.
ADVANCED ENGINEERING MATERIALS. Volume: 22 (2020) - 2020 • 6185
Polymer/enzyme-modified HF-etched carbon nanoelectrodes for single-cell analysis
Marquitan, M. and Ruff, A. and Bramini, M. and Herlitze, S. and Mark, M.D. and Schuhmann, W.
BIOELECTROCHEMISTRY. Volume: 133 (2020) - 2020 • 6184
Reconstruction of structural anomalies out of seismic measurements by means of a non-deterministic full waveform inversion approach for application in mechanized tunneling
Trapp, M. and Nestorović, T.
JOURNAL OF APPLIED GEOPHYSICS. Volume: 182 (2020) - 2020 • 6183
Glutamate detection at the cellular level by means of polymer/enzyme multilayer modified carbon nanoelectrodes
Marquitan, M. and Mark, M.D. and Ernst, A. and Muhs, A. and Herlitze, S. and Ruff, A. and Schuhmann, W.
JOURNAL OF MATERIALS CHEMISTRY B. Volume: 8 (2020) - 2020 • 6182
Detecting deviations from second-order stationarity in locally stationary functional time series
Bücher, A. and Dette, H. and Heinrichs, F.
ANNALS OF THE INSTITUTE OF STATISTICAL MATHEMATICS. Volume: 72 (2020) - 2020 • 6181
Inverse method to determine fatigue properties of materials by combining cyclic indentation and numerical simulation
Sajjad, H.M. and ul Hassan, H. and Kuntz, M. and Schäfer, B.J. and Sonnweber-Ribic, P. and Hartmaier, A.
MATERIALS. Volume: 13 (2020) - 2020 • 6180
Electrostatic potential shape of gate-defined quantum point contacts
Geier, M. and Freudenfeld, J. and Silva, J.T. and Umansky, V. and Reuter, D. and Wieck, A.D. and Brouwer, P.W. and Ludwig, S.
PHYSICAL REVIEW B. Volume: 101 (2020) - 2020 • 6179
Role of inclination dependence of grain boundary energy on the microstructure evolution during grain growth
Salama, H. and Kundin, J. and Shchyglo, O. and Mohles, V. and Marquardt, K. and Steinbach, I.
ACTA MATERIALIA. Volume: 188 (2020) - 2020 • 6178
In Situ Generation of Electrolyte inside Pyridine-Based Covalent Triazine Frameworks for Direct Supercapacitor Integration
Troschke, E. and Leistenschneider, D. and Rensch, T. and Grätz, S. and Maschita, J. and Ehrling, S. and Klemmed, B. and Lotsch, B.V. and Eychmüller, A. and Borchardt, L. and Kaskel, S.
CHEMSUSCHEM. Volume: 13 (2020) - 2020 • 6177
Selective cyclohexene oxidation with O2, H2O2and: Tert -butyl hydroperoxide over spray-flame synthesized LaCo1- xFexO3nanoparticles
Büker, J. and Alkan, B. and Fu, Q. and Xia, W. and Schulwitz, J. and Waffel, D. and Falk, T. and Schulz, C. and Wiggers, H. and Muhler, M. and Peng, B.
CATALYSIS SCIENCE AND TECHNOLOGY. Volume: 10 (2020) - 2020 • 6176
On the commensuration of plastic plowing at the microscale
Tsybenko, H. and Xia, W. and Dehm, G. and Brinckmann, S.
TRIBOLOGY INTERNATIONAL. Volume: 151 (2020) - 2020 • 6175
Hardware/Software Co-design for the Signal Processing of Dielectric Materials Characterization
Kamaleldin, A. and Wagner, J. and Rolfes, I. and Barowski, J. and Goehringer, D.
2020 3RD INTERNATIONAL WORKSHOP ON MOBILE TERAHERTZ SYSTEMS, IWMTS 2020. Volume: (2020) - 2020 • 6174
Concept development for the generation of support structures in the laser metal deposition process
Marx, J. and Thiele, M. and Esen, C. and Ostendorf, A.
PROCEDIA CIRP. Volume: 94 (2020) - 2020 • 6173
How nanoscale dislocation reactions govern low-temperature and high-stress creep of ni-base single crystal superalloys
Bürger, D. and Dlouhý, A. and Yoshimi, K. and Eggeler, G.
CRYSTALS. Volume: 10 (2020) - 2020 • 6172
Multistep stochastic mechanism of polarization reversal in rhombohedral ferroelectrics
Genenko, Y.A. and Khachaturyan, R. and Vorotiahin, I.S. and Schultheiß, J. and Daniels, J.E. and Grünebohm, A. and Koruza, J.
PHYSICAL REVIEW B. Volume: 102 (2020) - 2020 • 6171
Evaluating Computational Shortcuts in Supercell-Based Phonon Calculations of Molecular Crystals: The Instructive Case of Naphthalene
Kamencek, T. and Wieser, S. and Kojima, H. and Bedoya-Martínez, N. and Dürholt, J.P. and Schmid, R. and Zojer, E.
JOURNAL OF CHEMICAL THEORY AND COMPUTATION. Volume: 16 (2020) - 2020 • 6170
Electrocatalysis as the Nexus for Sustainable Renewable Energy: The Gordian Knot of Activity, Stability, and Selectivity
Masa, J. and Andronescu, C. and Schuhmann, W.
ANGEWANDTE CHEMIE - INTERNATIONAL EDITION. Volume: 59 (2020) - 2020 • 6169
Dft-cef approach for the thermodynamic properties and volume of stable and metastable al–ni compounds
Tumminello, S. and Palumbo, M. and Koßmann, J. and Hammerschmidt, T. and Alonso, P.R. and Sommadossi, S. and Fries, S.G.
METALS. Volume: 10 (2020) - 2020 • 6168
On the stress and temperature dependence of low temperature and high stress shear creep in Ni-base single crystal superalloys
Bürger, D. and Dlouhý, A. and Yoshimi, K. and Eggeler, G.
MATERIALS SCIENCE AND ENGINEERING A. Volume: 795 (2020) - 2020 • 6167
High entropy alloys: A focused review of mechanical properties and deformation mechanisms
George, E.P. and Curtin, W.A. and Tasan, C.C.
ACTA MATERIALIA. Volume: 188 (2020) - 2020 • 6166
Detection and amplification of spin noise using scattered laser light in a quantum-dot microcavity
Kamenskii, A.N. and Petrov, M.Y. and Kozlov, G.G. and Zapasskii, V.S. and Scholz, S.E. and Sgroi, C. and Ludwig, Ar. and Wieck, A.D. and Bayer, M. and Greilich, A.
PHYSICAL REVIEW B. Volume: 101 (2020) - 2020 • 6165
Breaking scaling relations in electrocatalysis
Masa, J. and Schuhmann, W.
JOURNAL OF SOLID STATE ELECTROCHEMISTRY. Volume: 24 (2020) - 2020 • 6164
Numerical material testing for discontinuous fiber composites using statistically similar representative volume elements
Sasagawa, T. and Tanaka, M. and Omote, R. and Balzani, D.
SCIENTIFIC REPORTS. Volume: 10 (2020) - 2020 • 6163
Efficient cut-cell quadrature based on moment fitting for materially nonlinear analysis
Bui, H.-G. and Schillinger, D. and Meschke, G.
COMPUTER METHODS IN APPLIED MECHANICS AND ENGINEERING. Volume: 366 (2020) - 2020 • 6162
Electrochemical CO2 Reduction-The Effect of Chalcogenide Exchange in Ni-Isocyclam Complexes
Gerschel, P. and Battistella, B. and Siegmund, D. and Ray, K. and Apfel, U.-P.
ORGANOMETALLICS. Volume: 39 (2020) - 2020 • 6161
Effect of size and domain orientation on strength of Barium Titanate
Mathews, N.G. and Saxena, A.K. and Kirchlechner, C. and Dehm, G. and Jaya, B.N.
SCRIPTA MATERIALIA. Volume: 182 (2020) - 2020 • 6160
A parallelization strategy for hydro-mechanically coupled mechanized tunneling simulations
Bui, H.-G. and Meschke, G.
COMPUTERS AND GEOTECHNICS. Volume: 120 (2020) - 2020 • 6159
Structure zone investigation of multiple principle element alloy thin films as optimization for nanoindentation measurements
Savan, A. and Allermann, T. and Wang, X. and Grochla, D. and Banko, L. and Kalchev, Y. and Kostka, A. and Pfetzing-Micklich, J. and Ludwig, Al.
MATERIALS. Volume: 13 (2020) - 2020 • 6158
Thermal barrier coatings with novel architectures for diesel engine applications
Uczak de Goes, W. and Markocsan, N. and Gupta, M. and Vaßen, R. and Matsushita, T. and Illkova, K.
SURFACE AND COATINGS TECHNOLOGY. Volume: 396 (2020) - 2020 • 6157
Full counting statistics of spin-flip and spin-conserving charge transitions in Pauli-spin blockade
Matsuo, S. and Kuroyama, K. and Yabunaka, S. and Valentin, S.R. and Ludwig, Ar. and Wieck, A.D. and Tarucha, S.
PHYSICAL REVIEW RESEARCH. Volume: 2 (2020) - 2020 • 6156
Experimental conditions affecting the measured fracture toughness at the microscale: Notch geometry and crack extension measurement
Saxena, A.K. and Brinckmann, S. and Völker, B. and Dehm, G. and Kirchlechner, C.
MATERIALS AND DESIGN. Volume: 191 (2020) - 2020 • 6155
Columnar-equiaxed transition in continuous casting based on a micro-macro solidification model with long-range solutal mixing
Uddagiri, M. and Spee, J. and Hubig, S. and Steinbach, I.
IOP CONFERENCE SERIES: MATERIALS SCIENCE AND ENGINEERING. Volume: 861 (2020) - 2020 • 6154
Coatings with Columnar Microstructures for Thermal Barrier Applications
Mauer, G. and Vaßen, R.
ADVANCED ENGINEERING MATERIALS. Volume: 22 (2020) - 2020 • 6153
Suspended Spot-Size Converters for Scalable Single-Photon Devices
Uğurlu, A.D. and Thyrrestrup, H. and Uppu, R. and Ouellet-Plamondon, C. and Schott, R. and Wieck, A.D. and Ludwig, Ar. and Lodahl, P. and Midolo, L.
ADVANCED QUANTUM TECHNOLOGIES. Volume: 3 (2020) - 2020 • 6152
Insights into Water Permeation through hBN Nanocapillaries by Ab Initio Machine Learning Molecular Dynamics Simulations
Ghorbanfekr, H. and Behler, J. and Peeters, F.M.
JOURNAL OF PHYSICAL CHEMISTRY LETTERS. Volume: 11 (2020) - 2020 • 6151
Selective photocatalytic oxidation of cyclohexanol to cyclohexanone: A spectroscopic and kinetic study
Karimi Estahbanati, M.R. and Feilizadeh, M. and Babin, A. and Mei, B. and Mul, G. and Iliuta, M.C.
CHEMICAL ENGINEERING JOURNAL. Volume: 382 (2020) - 2020 • 6150
Saturation of the stress-strain behaviour of architectural fabrics
Uhlemann, J. and Surholt, F. and Westerhoff, A. and Stranghöner, N. and Motevalli, M. and Balzani, D.
MATERIALS AND DESIGN. Volume: 191 (2020) - 2020 • 6149
Evidence for an Fulde-Ferrell-Larkin-Ovchinnikov State with Segmented Vortices in the BCS-BEC-Crossover Superconductor FeSe
Kasahara, S. and Sato, Y. and Licciardello, S. and Čulo, M. and Arsenijević, S. and Ottenbros, T. and Tominaga, T. and Böker, J. and Eremin, I. and Shibauchi, T. and Wosnitza, J. and Hussey, N.E. and Matsuda, Y.
PHYSICAL REVIEW LETTERS. Volume: 124 (2020) - 2020 • 6148
Recent Progress in Local Characterization of Damage Evolution in Thermal Barrier Coating Under Thermal Cycling
Maurel, V. and Mahfouz, L. and Guipont, V. and Marchand, B. and Gaslain, F. and Koster, A. and Dennstedt, A. and Bartsch, M. and Coudon, F.
MINERALS, METALS AND MATERIALS SERIES. Volume: (2020) - 2020 • 6147
Nanoglass–Nanocrystal Composite—a Novel Material Class for Enhanced Strength–Plasticity Synergy
Katnagallu, S. and Wu, G. and Singh, S.P. and Nandam, S.H. and Xia, W. and Stephenson, L.T. and Gleiter, H. and Schwaiger, R. and Hahn, H. and Herbig, M. and Raabe, D. and Gault, B. and Balachandran, S.
SMALL. Volume: 16 (2020) - 2020 • 6146
Architectural woven polyester fabrics: examination of possible classification of stiffness values in correlation with strength values
Uhlemann, J. and Stranghöner, N. and Motevalli, M. and Balzani, D.
ARCHITECTURAL ENGINEERING AND DESIGN MANAGEMENT. Volume: (2020) - 2020 • 6145
Defect Segregation and Its Effect on the Photoelectrochemical Properties of Ti-Doped Hematite Photoanodes for Solar Water Splitting
Scherrer, B. and Li, T. and Tsyganok, A. and Döbeli, M. and Gupta, B. and Malviya, K.D. and Kasian, O. and Maman, N. and Gault, B. and Grave, D.A. and Mehlman, A. and Visoly-Fisher, I. and Raabe, D. and Rothschild, A.
CHEMISTRY OF MATERIALS. Volume: 32 (2020) - 2020 • 6144
Direct Atomic-Level Imaging of Zeolites: Oxygen, Sodium in Na-LTA and Iron in Fe-MFI
Mayoral, A. and Zhang, Q. and Zhou, Y. and Chen, P. and Ma, Y. and Monji, T. and Losch, P. and Schmidt, W. and Schüth, F. and Hirao, H. and Yu, J. and Terasaki, O.
ANGEWANDTE CHEMIE - INTERNATIONAL EDITION. Volume: 59 (2020) - 2020 • 6143
Erratum: Anisotropic thermodynamic and transport properties of single-crystalline CaKFe4As4 (Physical Review B (2016) 94 (064501) DOI: 10.1103/PhysRevB.94.064501)
Meier, W.R. and Kong, T. and Kaluarachchi, U.S. and Taufour, V. and Jo, N.H. and Drachuck, G. and Böhmer, A.E. and Saunders, S.M. and Sapkota, A. and Kreyssig, A. and Tanatar, M.A. and Prozorov, R. and Goldman, A.I. and Balakirev, F.F. and Gurevich, A. and Bud'ko, S.L. and Canfield, P.C.
PHYSICAL REVIEW B. Volume: 102 (2020) - 2020 • 6142
Simulation of capillary-driven kinetics with multi-phase-field and lattice Boltzmann method
Schiedung, R. and Tegeler, M. and Medvedev, D. and Varnik, F.
MODELLING AND SIMULATION IN MATERIALS SCIENCE AND ENGINEERING. Volume: 28 (2020) - 2020 • 6141
On-chip deterministic operation of quantum dots in dual-mode waveguides for a plug-and-play single-photon source
Uppu, R. and Eriksen, H.T. and Thyrrestrup, H. and Uğurlu, A.D. and Wang, Y. and Scholz, S. and Wieck, A.D. and Ludwig, Ar. and Löbl, M.C. and Warburton, R.J. and Lodahl, P. and Midolo, L.
NATURE COMMUNICATIONS. Volume: 11 (2020) - 2020 • 6140
Tantalum and zirconium induced structural transitions at complex [111] tilt grain boundaries in copper
Meiners, T. and Duarte, J.M. and Richter, G. and Dehm, G. and Liebscher, C.H.
ACTA MATERIALIA. Volume: 190 (2020) - 2020 • 6139
Scalable integrated single-photon source
Uppu, R. and Pedersen, F.T. and Wang, Y. and Olesen, C.T. and Papon, C. and Zhou, X. and Midolo, L. and Scholz, S. and Wieck, A.D. and Ludwig, Ar. and Lodahl, P.
SCIENCE ADVANCES. Volume: 6 (2020) - 2020 • 6138
Observations of grain-boundary phase transformations in an elemental metal
Meiners, T. and Frolov, T. and Rudd, R.E. and Dehm, G. and Liebscher, C.H.
NATURE. Volume: 579 (2020) - 2020 • 6137
On the agglomeration tendency of carbonaceous fuels in fluidized beds
Urciuolo, M. and Solimene, R. and Ammendola, P. and Krusch, S. and Scherer, V. and Salatino, P. and Chirone, R. and Senneca, O.
FUEL. Volume: 277 (2020) - 2020 • 6136
Simulation of a reacting agitated bed of straw pellets by a resolved coupled DEM/CFD method using a blocked-off approach
Buss, F. and Wirtz, S. and Scherer, V.
INTERNATIONAL JOURNAL OF THERMAL SCIENCES. Volume: 152 (2020) - 2020 • 6135
Structural evolution of bimetallic Co-Cu catalysts in CO hydrogenation to higher alcohols at high pressure
Göbel, C. and Schmidt, S. and Froese, C. and Fu, Q. and Chen, Y.-T. and Pan, Q. and Muhler, M.
JOURNAL OF CATALYSIS. Volume: 383 (2020) - 2020 • 6134
Interplay of Spin Crossover and Coordination-Induced Spin State Switch for Iron Bis(pyrazolyl)methanes in Solution
Keisers, K. and Hüppe, H.M. and Iffland-Mühlhaus, L. and Hoffmann, A. and Göbel, C. and Apfel, U.-P. and Weber, B. and Herres-Pawlis, S.
INORGANIC CHEMISTRY. Volume: 59 (2020) - 2020 • 6133
On the effects of diluted and mixed ionic liquids as liquid substrates for the sputter synthesis of nanoparticles
Meischein, M. and Fork, M. and Ludwig, Al.
NANOMATERIALS. Volume: 10 (2020) - 2020 • 6132
Engineering a Compliant Mechanical Amplifier for MEMS Sensor Applications
Schmitt, P. and Hoffmann, M.
JOURNAL OF MICROELECTROMECHANICAL SYSTEMS. Volume: 29 (2020) - 2020 • 6131
Observation of the Kondo screening cloud
V. Borzenets, I. and Shim, J. and Chen, J.C.H. and Ludwig, Ar. and Wieck, A.D. and Tarucha, S. and Sim, H.-S. and Yamamoto, M.
NATURE. Volume: 579 (2020) - 2020 • 6130
Direct Binary Encoding of Displacements on the Nano-Scale
Schmitt, P. and Hoffmann, M.
PROCEEDINGS OF THE IEEE INTERNATIONAL CONFERENCE ON MICRO ELECTRO MECHANICAL SYSTEMS (MEMS). Volume: 2020-January (2020) - 2020 • 6129
Micromechanical modeling of DP600 steel: From microstructure to the sheet metal forming process
Vajragupta, N. and Maassen, S. and Clausmeyer, T. and Brands, D. and Schröder, J. and Hartmaier, A.
PROCEDIA MANUFACTURING. Volume: 47 (2020) - 2020 • 6128
The steady-state kinetics of CO hydrogenation to higher alcohols over a bulk Co-Cu catalyst
Göbel, C. and Schmidt, S. and Froese, C. and Bujara, T. and Viktor Scherer and Muhler, M.
JOURNAL OF CATALYSIS. Volume: (2020) - 2020 • 6127
Role of pre-ordered liquid in the selection mechanism of crystal polymorphs during nucleation
Menon, S. and Díaz Leines, G. and Drautz, R. and Rogal, J.
JOURNAL OF CHEMICAL PHYSICS. Volume: 153 (2020) - 2020 • 6126
Controlling bubble coalescence in metallic foams: A simple phase field-based approach
Vakili, S. and Steinbach, I. and Varnik, F.
COMPUTATIONAL MATERIALS SCIENCE. Volume: 173 (2020) - 2020 • 6125
Multi-phase-field simulation of microstructure evolution in metallic foams
Vakili, S. and Steinbach, I. and Varnik, F.
SCIENTIFIC REPORTS. Volume: 10 (2020) - 2020 • 6124
Photocatalytic oxidation of propane using hydrothermally prepared anatase-brookite-rutile tio2 samples. An in situ drifts study
Cano-Casanova, L. and Mei, B. and Mul, G. and Lillo-Ródenas, M.Á. and Román-Martínez, M.C.
NANOMATERIALS. Volume: 10 (2020) - 2020 • 6123
Effect of electric current on the optical orientation of interface electrons in AlGaAs/GaAs heterostructures
Ken, O.S. and Zhukov, E.A. and Akimov, I.A. and Korenev, V.L. and Kopteva, N.E. and Kalitukha, I.V. and Sapega, V.F. and Wieck, A.D. and Ludwig, Ar. and Schott, R. and Kusrayev, Y.G. and Yakovlev, D.R. and Bayer, M.
PHYSICAL REVIEW B. Volume: 102 (2020) - 2020 • 6122
Synthesis of plasmonic Fe/Al nanoparticles in ionic liquids
Schmitz, A. and Meyer, H. and Meischein, M. and Garzón Manjón, A. and Schmolke, L. and Giesen, B. and Schlüsener, C. and Simon, P. and Grin, Y. and Fischer, R.A. and Scheu, C. and Ludwig, Al. and Janiak, C.
RSC ADVANCES. Volume: 10 (2020) - 2020 • 6121
On the influence of crystallography on creep of circular notched single crystal superalloy specimens
Cao, L. and Thome, P. and Agudo Jácome, L. and Somsen, C. and Cailletaud, G. and Eggeler, G.
MATERIALS SCIENCE AND ENGINEERING A. Volume: 782 (2020) - 2020 • 6120
Cr2AlC MAX phase as bond coat for thermal barrier coatings: Processing, testing under thermal gradient loading, and future challenges
Gonzalez-Julian, J. and Mauer, G. and Sebold, D. and Mack, D.E. and Vassen, R.
JOURNAL OF THE AMERICAN CERAMIC SOCIETY. Volume: 103 (2020) - 2020 • 6119
Subtoxic cell responses to silica particles with different size and shape
Kersting, M. and Olejnik, M. and Rosenkranz, N. and Loza, K. and Breisch, M. and Rostek, A. and Westphal, G. and Bünger, J. and Ziegler, N. and Ludwig, Al. and Köller, M. and Sengstock, C. and Epple, M.
SCIENTIFIC REPORTS. Volume: 10 (2020) - 2020 • 6118
Roadmap on multiscale materials modeling
Van Der Giessen, E. and Schultz, P.A. and Bertin, N. and Bulatov, V.V. and Cai, W. and Csányi, G. and Foiles, S.M. and Geers, M.G.D. and González, C. and Hütter, M. and Kim, W.K. and Kochmann, D.M. and Llorca, J. and Mattsson, A.E. and Rottler, J. and Shluger, A. and Sills, R.B. and Steinbach, I. and Strachan, A. and Tadmor, E.B.
MODELLING AND SIMULATION IN MATERIALS SCIENCE AND ENGINEERING. Volume: 28 (2020) - 2020 • 6117
Artificial neural network surrogate modelling for real-time predictions and control of building damage during mechanised tunnelling
Cao, B.T. and Obel, M. and Freitag, S. and Mark, P. and Meschke, G.
ADVANCES IN ENGINEERING SOFTWARE. Volume: 149 (2020) - 2020 • 6116
Analysis of strengthening due to grain boundaries and annealing twin boundaries in the CrCoNi medium-entropy alloy
Schneider, M. and George, E.P. and Manescau, T.J. and Záležák, T. and Hunfeld, J. and Dlouhý, A. and Eggeler, G. and Laplanche, G.
INTERNATIONAL JOURNAL OF PLASTICITY. Volume: 124 (2020) - 2020 • 6115
Degradation of zirconia in moisture
Cao, X. and Vassen, R. and Wang, J. and Zou, B. and Li, S. and Hui, Y. and Yuan, J. and Song, W. and Cao, Q. and Jiang, J. and Deng, L. and Dong, S.
CORROSION SCIENCE. Volume: 176 (2020) - 2020 • 6114
Data compilation on the effect of grain size, temperature, and texture on the strength of a single-phase FCC MnFeNi medium-entropy alloy
Schneider, M. and Werner, F. and Langenkämper, D. and Reinhart, C. and Laplanche, G.
DATA IN BRIEF. Volume: 28 (2020) - 2020 • 6113
Performance of YSZ and Gd2Zr2O7/YSZ double layer thermal barrier coatings in burner rig tests
Vaßen, R. and Bakan, E. and Mack, D. and Schwartz-Lückge, S. and Sebold, D. and Jung Sohn, Y. and Zhou, D. and Guillon, O.
JOURNAL OF THE EUROPEAN CERAMIC SOCIETY. Volume: 40 (2020) - 2020 • 6112
A comprehensive approach for the characterization of porous polymers using 13C and 15N dynamic nuclear polarization NMR spectroscopy
Grätz, S. and De Olivera Junior, M. and Gutmann, T. and Borchardt, L.
PHYSICAL CHEMISTRY CHEMICAL PHYSICS. Volume: 22 (2020) - 2020 • 6111
Correlation of Microstructure and Properties of Cold Gas Sprayed INCONEL 718 Coatings
Vaßen, R. and Fiebig, J. and Kalfhaus, T. and Gibmeier, J. and Kostka, A. and Schrüfer, S.
JOURNAL OF THERMAL SPRAY TECHNOLOGY. Volume: (2020) - 2020 • 6110
High-resolution in vivo imaging of peripheral nerves using optical coherence tomography: A feasibility study
Carolus, A.E. and Lenz, M. and Hofmann, M. and Welp, H. and Schmieder, K. and Brenke, C.
JOURNAL OF NEUROSURGERY. Volume: 132 (2020) - 2020 • 6109
Mechanochemical Cyclodehydrogenation with Elemental Copper: An Alternative Pathway toward Nanographenes
Grätz, S. and Oltermann, M. and Vogt, C.G. and Borchardt, L.
ACS SUSTAINABLE CHEMISTRY AND ENGINEERING. Volume: 8 (2020) - 2020 • 6108
Development of a Method for Non-Invasive Measurement of Absolute Pressure in Partially Transparent Containers with Carbonated Beverages [Entwicklung einer Methode zur nichtinvasiven Messung des Absolutdrucks in teiltransparenten Gebinden mit karbonisierten Getränken]
Grafen, M. and Falkenstein, M. and Ostendorf, A. and Esen, C.
CHEMIE-INGENIEUR-TECHNIK. Volume: 92 (2020) - 2020 • 6107
Influence of Process Parameters on the Aerosol Deposition (AD) of Yttria-Stabilized Zirconia Particles
Mishra, T.P. and Singh, R. and Mücke, R. and Malzbender, J. and Bram, M. and Guillon, O. and Vaßen, R.
JOURNAL OF THERMAL SPRAY TECHNOLOGY. Volume: (2020) - 2020 • 6106
Non-porous organic crystals and their interaction with guest molecules from the gas phase
Casco, M.E. and Krupp, F. and Grätz, S. and Schwenger, A. and Damakoudi, V. and Richert, C. and Frey, W. and Borchardt, L.
ADSORPTION. Volume: 26 (2020) - 2020 • 6105
Size dependent strength, slip transfer and slip compatibility in nanotwinned silver
Kini, M.K. and Dehm, G. and Kirchlechner, C.
ACTA MATERIALIA. Volume: 184 (2020) - 2020 • 6104
Holographic imaging of particles with Lloyd’s mirror interferometer
Schnitzler, L. and Kleemann, N. and Neutsch, K. and Hofmann, M.R. and Gerhardt, N.C.
OPTICS INFOBASE CONFERENCE PAPERS. Volume: (2020) - 2020 • 6103
Dislocation plasticity and detwinning under thermal stresses in nanotwinned Ag thin films
Kini, M.K. and Merola, C. and Breitbach, B. and Klapproth, D. and Philippi, B. and Molin, J.-B. and Kirchlechner, C. and Dehm, G.
ACTA MATERIALIA. Volume: 198 (2020) - 2020 • 6102
Synthesis of Novel Sulfobetaine Polymers with Differing Dipole Orientations in Their Side Chains, and Their Effects on the Antifouling Properties
Schönemann, E. and Koc, J. and Aldred, N. and Clare, A.S. and Laschewsky, A. and Rosenhahn, A. and Wischerhoff, E.
MACROMOLECULAR RAPID COMMUNICATIONS. Volume: 41 (2020) - 2020 • 6101
Closed-loop control of a GaAs-based singlet-triplet spin qubit with 99.5% gate fidelity and low leakage
Cerfontaine, P. and Botzem, T. and Ritzmann, J. and Humpohl, S.S. and Ludwig, Ar. and Schuh, D. and Bougeard, D. and Wieck, A.D. and Bluhm, H.
NATURE COMMUNICATIONS. Volume: 11 (2020) - 2020 • 6100
Superconductivity with broken time-reversal symmetry inside a superconducting s-wave state
Grinenko, V. and Sarkar, R. and Kihou, K. and Lee, C.H. and Morozov, I. and Aswartham, S. and Büchner, B. and Chekhonin, P. and Skrotzki, W. and Nenkov, K. and Hühne, R. and Nielsch, K. and Drechsler, S.-L. and Vadimov, V.L. and Silaev, M.A. and Volkov, P.A. and Eremin, I. and Luetkens, H. and Klauss, H.-H.
NATURE PHYSICS. Volume: 16 (2020) - 2020 • 6099
In-situ synthesis via laser metal deposition of a lean Cu–3.4Cr–0.6Nb (at%) conductive alloy hardened by Cr nano-scale precipitates and by Laves phase micro-particles
Kini, A.R. and Maischner, D. and Weisheit, A. and Ponge, D. and Gault, B. and Jägle, E.A. and Raabe, D.
ACTA MATERIALIA. Volume: 197 (2020) - 2020 • 6098
Equivalence of regression curves sharing common parameters
Möllenhoff, K. and Bretz, F. and Dette, H.
BIOMETRICS. Volume: 76 (2020) - 2020 • 6097
Nanocrystalline Sm-based 1:12 magnets
Schönhöbel, A.M. and Madugundo, R. and Barandiarán, J.M. and Hadjipanayis, G.C. and Palanisamy, D. and Schwarz, T. and Gault, B. and Raabe, D. and Skokov, K. and Gutfleisch, O. and Fischbacher, J. and Schrefl, T.
ACTA MATERIALIA. Volume: 200 (2020) - 2020 • 6096
Characterization of a transient spark micro-discharge in nitrogen using simultaneous two-wavelength diagnostics
Gröger, S. and Fiebrandt, M. and Hamme, M. and Bibinov, N. and Awakowicz, P.
MEASUREMENT SCIENCE AND TECHNOLOGY. Volume: 31 (2020) - 2020 • 6095
In vivo imaging of human peripheral nerves using optical coherence tomography compared to histopathology slices
Möller, J. and Carolus, A.E. and Van De Nes, J.A.P. and Lenz, M. and Brenke, C. and Schmieder, K. and Welp, H. and Gerhardt, N.C. and Hofmann, M.R.
PROGRESS IN BIOMEDICAL OPTICS AND IMAGING - PROCEEDINGS OF SPIE. Volume: 11228 (2020) - 2020 • 6094
Trends in elastic properties of Ti-Ta alloys from first-principles calculations
Chakraborty, T. and Rogal, J.
JOURNAL OF PHYSICS CONDENSED MATTER. Volume: 33 (2020) - 2020 • 6093
How tensile tests allow a screening of the fracture toughness of hard coatings
Völker, B. and Du, C. and Fager, H. and Rueß, H. and Soler, R. and Kirchlechner, C. and Dehm, G. and Schneider, J.M.
SURFACE AND COATINGS TECHNOLOGY. Volume: 390 (2020) - 2020 • 6092
Could face-centered cubic titanium in cold-rolled commercially-pure titanium only be a Ti-hydride?
Chang, Y. and Zhang, S. and Liebscher, C.H. and Dye, D. and Ponge, D. and Scheu, C. and Dehm, G. and Raabe, D. and Gault, B. and Lu, W.
SCRIPTA MATERIALIA. Volume: 178 (2020) - 2020 • 6091
Differentiation between Carbon Corrosion and Oxygen Evolution Catalyzed by NixB/C Hybrid Electrocatalysts in Alkaline Solution using Differential Electrochemical Mass Spectrometry
Möller, S. and Barwe, S. and Dieckhöfer, S. and Masa, J. and Andronescu, C. and Schuhmann, W.
CHEMELECTROCHEM. Volume: 7 (2020) - 2020 • 6090
Influence of lattice misfit on the deformation behaviour of α2/γ lamellae in TiAl alloys
Chauniyal, A. and Janisch, R.
MATERIALS SCIENCE AND ENGINEERING A. Volume: 796 (2020) - 2020 • 6089
Nanosecond pulsed discharges in distilled water: I. Continuum radiation and plasma ignition
Grosse, K. and Schulz-Von Der Gathen, V. and Von Keudell, A.
PLASMA SOURCES SCIENCE AND TECHNOLOGY. Volume: 29 (2020) - 2020 • 6088
Online Monitoring of Electrochemical Carbon Corrosion in Alkaline Electrolytes by Differential Electrochemical Mass Spectrometry
Möller, S. and Barwe, S. and Masa, J. and Wintrich, D. and Seisel, S. and Baltruschat, H. and Schuhmann, W.
ANGEWANDTE CHEMIE - INTERNATIONAL EDITION. Volume: 59 (2020) - 2020 • 6087
Automated Fitting of Neural Network Potentials at Coupled Cluster Accuracy: Protonated Water Clusters as Testing Ground
Schran, C. and Behler, J. and Marx, D.
JOURNAL OF CHEMICAL THEORY AND COMPUTATION. Volume: 16 (2020) - 2020 • 6086
Chemistry in nanosecond plasmas in water
Chauvet, L. and Nenbangkaeo, C. and Grosse, K. and von Keudell, A.
PLASMA PROCESSES AND POLYMERS. Volume: 17 (2020) - 2020 • 6085
Carbon Supported Phosphoric Acid Catalysts for Gas-Phase Synthesis of Diesel Additives
Grünert, A. and Schmidt, W. and Schüth, F.
CATALYSIS LETTERS. Volume: (2020) - 2020 • 6084
In situ investigation of atmospheric plasma-sprayed Mn–Co–Fe–O by synchrotron X-ray nano-tomography
Grünwald, N. and Lhuissier, P. and Salvo, L. and Villanova, J. and Menzler, N.H. and Guillon, O. and Martin, C.L. and Vaßen, R.
JOURNAL OF MATERIALS SCIENCE. Volume: 55 (2020) - 2020 • 6083
Sintering and biocompatibility of blended elemental Ti-xNb alloys
Chen, Y. and Han, P. and Dehghan-Manshadi, A. and Kent, D. and Ehtemam-Haghighi, S. and Jowers, C. and Bermingham, M. and Li, T. and Cooper-White, J. and Dargusch, M.S.
JOURNAL OF THE MECHANICAL BEHAVIOR OF BIOMEDICAL MATERIALS. Volume: 104 (2020) - 2020 • 6082
Exasteel: Towards a virtual laboratory for the multiscale simulation of dual-phase steel using high-performance computing
Klawonn, A. and Lanser, M. and Uran, M. and Rheinbach, O. and Köhler, S. and Schröder, J. and Scheunemann, L. and Brands, D. and Balzani, D. and Gandhi, A. and Wellein, G. and Wittmann, M. and Schenk, O. and Janalík, R.
LECTURE NOTES IN COMPUTATIONAL SCIENCE AND ENGINEERING. Volume: 136 (2020) - 2020 • 6081
A new class of lightweight, stainless steels with ultra-high strength and large ductility
Moon, J. and Ha, H.-Y. and Kim, K.-W. and Park, S.-J. and Lee, T.-H. and Kim, S.-D. and Jang, J.H. and Jo, H.-H. and Hong, H.-U. and Lee, B.H. and Lee, Y.-J. and Lee, C. and Suh, D.-W. and Han, H.N. and Raabe, D. and Lee, C.-H.
SCIENTIFIC REPORTS. Volume: 10 (2020) - 2020 • 6080
Trimetallic Mn-Fe-Ni Oxide Nanoparticles Supported on Multi-Walled Carbon Nanotubes as High-Performance Bifunctional ORR/OER Electrocatalyst in Alkaline Media
Morales, D.M. and Kazakova, M.A. and Dieckhöfer, S. and Selyutin, A.G. and Golubtsov, G.V. and Schuhmann, W. and Masa, J.
ADVANCED FUNCTIONAL MATERIALS. Volume: 30 (2020) - 2020 • 6079
Conversion of volatile organic compounds in a twin surface dielectric barrier discharge
Schücke, L. and Gembus, J.-L. and Peters, N. and Kogelheide, F. and Nguyen-Smith, R.T. and Gibson, A.R. and Schulze, J. and Muhler, M. and Awakowicz, P.
PLASMA SOURCES SCIENCE AND TECHNOLOGY. Volume: 29 (2020) - 2020 • 6078
Nanosecond pulsed discharges in distilled water-Part II: Line emission and plasma propagation
Von Keudell, A. and Grosse, K. and Schulz-Von Der Gathen, V.
PLASMA SOURCES SCIENCE AND TECHNOLOGY. Volume: 29 (2020) - 2020 • 6077
Numerical multi-level model for fiber-reinforced concrete - Multi-level validation based on an experimental study on high-strength concrete [Numerisches Mehrebenen-Modell für Stahlfaserbeton: Von der Faser- zur Bauteilebene: Mehrstufige Validierung anhand einer experimentellen Studie an hochfestem Faserbeton]
Gudžulic, V. and Neu, G.E. and Gebuhr, G. and Anders, S. and Meschke, G.
BETON- UND STAHLBETONBAU. Volume: 115 (2020) - 2020 • 6076
The sum is more than its parts: stability of MnFe oxide nanoparticles supported on oxygen-functionalized multi-walled carbon nanotubes at alternating oxygen reduction reaction and oxygen evolution reaction conditions
Morales, D.M. and Kazakova, M.A. and Purcel, M. and Masa, J. and Schuhmann, W.
JOURNAL OF SOLID STATE ELECTROCHEMISTRY. Volume: 24 (2020) - 2020 • 6075
Interstitial doping enhances the strength-ductility synergy in a CoCrNi medium entropy alloy
Moravcik, I. and Hornik, V. and Minárik, P. and Li, L. and Dlouhy, I. and Janovska, M. and Raabe, D. and Li, Z.
MATERIALS SCIENCE AND ENGINEERING A. Volume: 781 (2020) - 2020 • 6074
Cementitious composites with high compaction potential: Modeling and calibration
Vu, G. and Iskhakov, T. and Timothy, J.J. and Schulte-Schrepping, C. and Breitenbücher, R. and Meschke, G.
MATERIALS. Volume: 13 (2020) - 2020 • 6073
Yield strength increase of a CoCrNi medium entropy alloy by interstitial nitrogen doping at maintained ductility
Moravcik, I. and Hadraba, H. and Li, L. and Dlouhy, I. and Raabe, D. and Li, Z.
SCRIPTA MATERIALIA. Volume: 178 (2020) - 2020 • 6072
Solidification of Two-Component Grouts by the Use of Superabsorbent Polymers as Activator
Schulte-Schrepping, C. and Ov, D. and Breitenbücher, R.
RILEM BOOKSERIES. Volume: 24 (2020) - 2020 • 6071
Transversally travelling ultrasound for light guiding deep into scattering media
Cherkashin, M.N. and Brenner, C. and Schmitz, G. and Hofmann, M.R.
COMMUNICATIONS PHYSICS. Volume: 3 (2020) - 2020 • 6070
Moving cracks form white etching areas during rolling contact fatigue in bearings
Morsdorf, L. and Mayweg, D. and Li, Y. and Diederichs, A. and Raabe, D. and Herbig, M.
MATERIALS SCIENCE AND ENGINEERING A. Volume: 771 (2020) - 2020 • 6069
Common structures of CO2 on structurally different coin metal surfaces
Vyshnepolsky, M. and Morgenstern, K.
PHYSICAL CHEMISTRY CHEMICAL PHYSICS. Volume: 22 (2020) - 2020 • 6068
Homolytic versus Heterolytic Hydrogen Evolution Reaction Steered by a Steric Effect
Guo, X. and Wang, N. and Li, X. and Zhang, Z. and Zhao, J. and Ren, W. and Ding, S. and Xu, G. and Li, J. and Apfel, U.-P. and Zhang, W. and Cao, R.
ANGEWANDTE CHEMIE - INTERNATIONAL EDITION. Volume: 59 (2020) - 2020 • 6067
Coherent control of individual electron spins in a two-dimensional quantum dot array
Mortemousque, P.-A. and Chanrion, E. and Jadot, B. and Flentje, H. and Ludwig, Ar. and Wieck, A.D. and Urdampilleta, M. and Bäuerle, C. and Meunier, T.
NATURE NANOTECHNOLOGY. Volume: (2020) - 2020 • 6066
Two-dimensional electron bound hole photoluminescence in GaAs in perpendicular magnetic fields
Schuster, J. and Kim, T.Y. and Batke, E. and Reuter, D. and Wieck, A.D.
SEMICONDUCTOR SCIENCE AND TECHNOLOGY. Volume: 35 (2020) - 2020 • 6065
Investigation of Synergistic Effects between Co and Fe in Co3-xFexO4 Spinel Catalysts for the Liquid-Phase Oxidation of Aromatic Alcohols and Styrene
Waffel, D. and Budiyanto, E. and Porske, T. and Büker, J. and Falk, T. and Fu, Q. and Schmidt, S. and Tüysüz, H. and Muhler, M. and Peng, B.
MOLECULAR CATALYSIS. Volume: 498 (2020) - 2020 • 6064
Dislocation-induced breakthrough of strength and ductility trade-off in a non-equiatomic high-entropy alloy
Guo, W. and Su, J. and Lu, W. and Liebscher, C.H. and Kirchlechner, C. and Ikeda, Y. and Körmann, F. and Liu, X. and Xue, Y. and Dehm, G.
ACTA MATERIALIA. Volume: 185 (2020) - 2020 • 6063
The elastic share of inelastic stress-strain paths of woven fabrics
Motevalli, M. and Uhlemann, J. and Stranghöner, N. and Balzani, D.
MATERIALS. Volume: 13 (2020) - 2020 • 6062
Variational Modeling and Finite-Element Simulation of Functional Fatigue in Polycrystalline Shape Memory Alloys
Waimann, J. and Hackl, K. and Junker, P.
JOURNAL OF OPTIMIZATION THEORY AND APPLICATIONS. Volume: 184 (2020) - 2020 • 6061
Dictionary learning in Fourier-transform scanning tunneling spectroscopy
Cheung, S.C. and Shin, J.Y. and Lau, Y. and Chen, Z. and Sun, J. and Zhang, Y. and Müller, M.A. and Eremin, I.M. and Wright, J.N. and Pasupathy, A.N.
NATURE COMMUNICATIONS. Volume: 11 (2020) - 2020 • 6060
Theoretical investigation of a miniature microwave driven plasma jet
Klute, M. and Porteanu, H.-E. and Stefanović, I. and Heinrich, W. and Awakowicz, P. and Brinkmann, R.P.
PLASMA SOURCES SCIENCE AND TECHNOLOGY. Volume: 29 (2020) - 2020 • 6059
Unfolding the complexity of phonon quasi-particle physics in disordered materials
Mu, S. and Olsen, R.J. and Dutta, B. and Lindsay, L. and Samolyuk, G.D. and Berlijn, T. and Specht, E.D. and Jin, K. and Bei, H. and Hickel, T. and Larson, B.C. and Stocks, G.M.
NPJ COMPUTATIONAL MATERIALS. Volume: 6 (2020) - 2020 • 6058
Variational regularization of damage models based on the emulated RVE
Schwarz, S. and Junker, P. and Hackl, K.
CONTINUUM MECHANICS AND THERMODYNAMICS. Volume: (2020) - 2020 • 6057
Hydrogen embrittlement at cleavage planes and grain boundaries in bcc iron—revisiting the first-principles cohesive zone model
Guzmán, A.A. and Jeon, J. and Hartmaier, A. and Janisch, R.
MATERIALS. Volume: 13 (2020) - 2020 • 6056
Laser scanning microscopy for the evaluation of the solid particle erosion resistance of different hardpaint wear protection layers [Laserscanningmikroskopie zur Beurteilung der Strahlverschleißbeständigkeit unterschiedlicher Hardpaint-Verschleißschutzschichten]
Schwarz, P. and Weber, S. and Deuerler, F.
PRAKTISCHE METALLOGRAPHIE/PRACTICAL METALLOGRAPHY. Volume: 57 (2020) - 2020 • 6055
XRD measurement of stacking fault energy of Cr–Ni austenitic steels: influence of temperature and alloying elements
Walter, M. and Mujica Roncery, L. and Weber, S. and Leich, L. and Theisen, W.
JOURNAL OF MATERIALS SCIENCE. Volume: 55 (2020) - 2020 • 6054
Continuous Wave THz System Based on an Electrically Tunable Monolithic Dual Wavelength Y-Branch DBR Diode Laser
Gwaro, J.O. and Brenner, C. and Theurer, L.S. and Maiwald, M. and Sumpf, B. and Hofmann, M.R.
JOURNAL OF INFRARED, MILLIMETER, AND TERAHERTZ WAVES. Volume: 41 (2020) - 2020 • 6053
Influence of particle reinforcement and heat treatment on the wear resistance of inductively melted hardpaint coatings
Schwarz, P. and Weber, S. and Deuerler, F.
METALS. Volume: 10 (2020) - 2020 • 6052
Peel-back controlled lithospheric convergence explains the secular transitions in Archean metamorphism and magmatism
Chowdhury, P. and Chakraborty, S. and Gerya, T.V. and Cawood, P.A. and Capitanio, F.A.
EARTH AND PLANETARY SCIENCE LETTERS. Volume: 538 (2020) - 2020 • 6051
Confinement of a three-dimensional organic molecule to two dimensions on a surface
Müller, M. and Henzl, J. and Morgenstern, K.
CHEMICAL PHYSICS LETTERS. Volume: 738 (2020) - 2020 • 6050
Microfluidic accumulation assay to quantify the attachment of the marine bacterium Cobetia marina on fouling-release coatings
Schwarze, J. and Wanka, R. and Rosenhahn, A.
BIOINTERPHASES. Volume: 15 (2020) - 2020 • 6049
Corrosion behavior of NiTi alloy subjected to femtosecond laser shock peening without protective coating in air environment
Wang, H. and Jürgensen, J. and Decker, P. and Hu, Z. and Yan, K. and Gurevich, E.L. and Ostendorf, A.
APPLIED SURFACE SCIENCE. Volume: 501 (2020) - 2020 • 6048
Atomistic deformation behavior of single and twin crystalline Cu nanopillars with preexisting dislocations
Ko, W.-S. and Stukowski, A. and Hadian, R. and Nematollahi, A. and Jeon, J.B. and Choi, W.S. and Dehm, G. and Neugebauer, J. and Kirchlechner, C. and Grabowski, B.
ACTA MATERIALIA. Volume: 197 (2020) - 2020 • 6047
Benchmarking and validation of a combined CFD-optics solver for micro-scale problems
Münster, R. and Mierka, O. and Turek, S. and Weigel, T. and Ostendorf, A.
OSA CONTINUUM. Volume: 3 (2020) - 2020 • 6046
Geometrical-optics analysis of whispering-gallery modes in the layer of a coated spherical resonator
Schweiger, G. and Weigel, T. and Ostendorf, A.
PHYSICAL REVIEW A. Volume: 102 (2020) - 2020 • 6045
Influences of Cr Content on the Phase Transformation Properties and Stress Change in V-Cr-O Thin-Film Libraries
Wang, X. and Suhr, E. and Banko, L. and Salomon, S. and Ludwig, Al.
ACS APPLIED ELECTRONIC MATERIALS. Volume: 2 (2020) - 2020 • 6044
Photocatalytic hydrogen production by photo-reforming of methanol with one-pot synthesized Pt-containing TiO2 photocatalysts
Chung, Y.-H. and Han, K. and Lin, C.-Y. and O'Neill, D. and Mul, G. and Mei, B. and Yang, C.-M.
CATALYSIS TODAY. Volume: 356 (2020) - 2020 • 6043
Formation of a 2D Meta-stable Oxide by Differential Oxidation of AgCu Alloys
Schweinar, K. and Beeg, S. and Hartwig, C. and Rajamathi, C.R. and Kasian, O. and Piccinin, S. and Prieto, M.J. and Tanase, L.C. and Gottlob, D.M. and Schmidt, T. and Raabe, D. and Schlögl, R. and Gault, B. and Jones, T.E. and Greiner, M.T.
ACS APPLIED MATERIALS AND INTERFACES. Volume: 12 (2020) - 2020 • 6042
Towards an understanding of grain boundary step in diamond cutting of polycrystalline copper
Wang, Z. and Zhang, J. and Zhang, J. and Li, G. and Zhang, H. and ul Hassan, H. and Hartmaier, A. and Yan, Y. and Sun, T.
JOURNAL OF MATERIALS PROCESSING TECHNOLOGY. Volume: 276 (2020) - 2020 • 6041
Are Onsager's reciprocal relations necessary to apply Thermodynamic Extremal Principles?
Hackl, K. and Fischer, F.D. and Zickler, G.A. and Svoboda, J.
JOURNAL OF THE MECHANICS AND PHYSICS OF SOLIDS. Volume: 135 (2020) - 2020 • 6040
Probing catalytic surfaces by correlative scanning photoemission electron microscopy and atom probe tomography
Schweinar, K. and Nicholls, R.L. and Rajamathi, C.R. and Zeller, P. and Amati, M. and Gregoratti, L. and Raabe, D. and Greiner, M. and Gault, B. and Kasian, O.
JOURNAL OF MATERIALS CHEMISTRY A. Volume: 8 (2020) - 2020 • 6039
Interfacial fracture toughness of sintered hybrid silver interconnects
Wang, S. and Kirchlechner, C. and Keer, L. and Dehm, G. and Yao, Y.
JOURNAL OF MATERIALS SCIENCE. Volume: 55 (2020) - 2020 • 6038
On the treatment of non-reciprocal rate-independent kinetics via thermodynamic extremal principles
Hackl, K. and Fischer, F.D. and Svoboda, J.
JOURNAL OF THE MECHANICS AND PHYSICS OF SOLIDS. Volume: 145 (2020) - 2020 • 6037
Effect of Dipole Orientation in Mixed, Charge-Equilibrated Self-assembled Monolayers on Protein Adsorption and Marine Biofouling
Koc, J. and Schardt, L. and Nolte, K. and Beyer, C. and Eckhard, T. and Schwiderowski, P. and Clarke, J.L. and Finlay, J.A. and Clare, A.S. and Muhler, M. and Laschewsky, A. and Rosenhahn, A.
ACS APPLIED MATERIALS AND INTERFACES. Volume: 12 (2020) - 2020 • 6036
Enhanced antibacterial performance of ultrathin silver/platinum nanopatches by a sacrificial anode mechanism
Abuayyash, A. and Ziegler, N. and Meyer, H. and Meischein, M. and Sengstock, C. and Moellenhoff, J. and Rurainsky, C. and Heggen, M. and Garzón-Manjón, A. and Scheu, C. and Tschulik, K. and Ludwig, Al. and Köller, M.
NANOMEDICINE: NANOTECHNOLOGY, BIOLOGY, AND MEDICINE. Volume: 24 (2020) - 2020 • 6035
Constraints in thermodynamic extremal principles for non-local dissipative processes
Hackl, K. and Fischer, F.D. and Svoboda, J.
CONTINUUM MECHANICS AND THERMODYNAMICS. Volume: 32 (2020) - 2020 • 6034
Effects of crosslink density in zwitterionic hydrogel coatings on their antifouling performance and susceptibility to silt uptake
Koc, J. and Schönemann, E. and Wanka, R. and Aldred, N. and Clare, A.S. and Gardner, H. and Swain, G.W. and Hunsucker, K. and Laschewsky, A. and Rosenhahn, A.
BIOFOULING. Volume: 36 (2020) - 2020 • 6033
Surface modification of NiTi alloy by ultrashort pulsed laser shock peening
Wang, H. and Kalchev, Y. and Wang, H. and Yan, K. and Gurevich, E.L. and Ostendorf, A.
SURFACE AND COATINGS TECHNOLOGY. Volume: 394 (2020) - 2020 • 6032
Structural stability of Co–V intermetallic phases and thermodynamic description of the Co–V system
Wang, P. and Hammerschmidt, T. and Kattner, U.R. and Olson, G.B.
CALPHAD: COMPUTER COUPLING OF PHASE DIAGRAMS AND THERMOCHEMISTRY. Volume: 68 (2020) - 2020 • 6031
Microstructure evolution and thermal stability of equiatomic CoCrFeNi films on (0001) α-Al2O3
Addab, Y. and Kini, M.K. and Courtois, B. and Savan, A. and Ludwig, Al. and Bozzolo, N. and Scheu, C. and Dehm, G. and Chatain, D.
ACTA MATERIALIA. Volume: 200 (2020) - 2020 • 6030
Microhardness and microabrasion behaviour of NiTi shape memory alloy after femtosecond laser shock peening without coating in air
Wang, H. and Gurevich, E.L. and Ostendorf, A.
PROCEEDINGS OF SPIE - THE INTERNATIONAL SOCIETY FOR OPTICAL ENGINEERING. Volume: 11273 (2020) - 2020 • 6029
The emergent field of high entropy oxides: Design, prospects, challenges, and opportunities for tailoring material properties
Musicó, B.L. and Gilbert, D. and Ward, T.Z. and Page, K. and George, E. and Yan, J. and Mandrus, D. and Keppens, V.
APL MATERIALS. Volume: 8 (2020) - 2020 • 6028
On the crystallographic anisotropy of plastic zone size in single crystalline copper under Berkovich nanoindentation
Wang, Z. and Zhang, J. and Ma, A. and Hartmaier, A. and Yan, Y. and Sun, T.
MATERIALS TODAY COMMUNICATIONS. Volume: 25 (2020) - 2020 • 6027
Crystal anisotropy-dependent shear angle variation in orthogonal cutting of single crystalline copper
Wang, Z. and Zhang, J. and Xu, Z. and Zhang, J. and Li, G. and Zhang, H. and Li, Z. and Hassan, H.U. and Fang, F. and Hartmaier, A. and Yan, Y. and Sun, T.
PRECISION ENGINEERING. Volume: 63 (2020) - 2020 • 6026
Polymer-Based Batteries—Flexible and Thin Energy Storage Systems
Hager, M.D. and Esser, B. and Feng, X. and Schuhmann, W. and Theato, P. and Schubert, U.S.
ADVANCED MATERIALS. Volume: 32 (2020) - 2020 • 6025
The role of humidity and UV-C emission in the inactivation of B. subtilis spores during atmospheric-pressure dielectric barrier discharge treatment
Kogelheide, F. and Voigt, F. and Hillebrand, B. and Moeller, R. and Fuchs, F. and Gibson, A.R. and Awakowicz, P. and Stapelmann, K. and Fiebrandt, M.
JOURNAL OF PHYSICS D: APPLIED PHYSICS. Volume: 53 (2020) - 2020 • 6024
An efficient and robust approach to determine material parameters of crystal plasticity constitutive laws from macro-scale stress-strain curves
Sedighiani, K. and Diehl, M. and Traka, K. and Roters, F. and Sietsma, J. and Raabe, D.
INTERNATIONAL JOURNAL OF PLASTICITY. Volume: 134 (2020) - 2020 • 6023
Characterisation of volume and surface dielectric barrier discharges in N2–O2 mixtures using optical emission spectroscopy
Kogelheide, F. and Offerhaus, B. and Bibinov, N. and Krajinski, P. and Schücke, L. and Schulze, J. and Stapelmann, K. and Awakowicz, P.
PLASMA PROCESSES AND POLYMERS. Volume: 17 (2020) - 2020 • 6022
Controlling the Number of Branches and Surface Facets of Pd-Core Ru-Branched Nanoparticles to Make Highly Active Oxygen Evolution Reaction Electrocatalysts
Myekhlai, M. and Benedetti, T.M. and Gloag, L. and Poerwoprajitno, A.R. and Cheong, S. and Schuhmann, W. and Gooding, J.J. and Tilley, R.D.
CHEMISTRY - A EUROPEAN JOURNAL. Volume: 26 (2020) - 2020 • 6021
Acidity enhancement through synergy of penta- and tetra-coordinated aluminum species in amorphous silica networks
Wang, Z. and Li, T. and Jiang, Y. and Lafon, O. and Liu, Z. and Trébosc, J. and Baiker, A. and Amoureux, J.-P. and Huang, J.
NATURE COMMUNICATIONS. Volume: 11 (2020) - 2020 • 6020
Spinodal decomposition versus classical γ′ nucleation in a nickel-base superalloy powder: An in-situ neutron diffraction and atomic-scale analysis
Collins, D.M. and D'Souza, N. and Panwisawas, C. and Papadaki, C. and West, G.D. and Kostka, A. and Kontis, P.
ACTA MATERIALIA. Volume: 200 (2020) - 2020 • 6019
Revealing the two-step nucleation and growth mechanism of vanadium carbonitrides in microalloyed steels
Wang, H. and Li, Y. and Detemple, E. and Eggeler, G.
SCRIPTA MATERIALIA. Volume: 187 (2020) - 2020 • 6018
Chemical instability at chalcogenide surfaces impacts chalcopyrite devices well beyond the surface
Colombara, D. and Elanzeery, H. and Nicoara, N. and Sharma, D. and Claro, M. and Schwarz, T. and Koprek, A. and Wolter, M.H. and Melchiorre, M. and Sood, M. and Valle, N. and Bondarchuk, O. and Babbe, F. and Spindler, C. and Cojocaru-Miredin, O. and Raabe, D. and Dale, P.J. and Sadewasser, S. and Siebentritt, S.
NATURE COMMUNICATIONS. Volume: 11 (2020) - 2020 • 6017
Acoustic Testing in the Technology of Production of Construction Ceramics with Reinforcing Additives
Nafikov, R.M. and Faseeva, G.R. and Lysogorskiy, Y.V. and Zakharov, Y.A. and Kabirov, R.R.
TECHNICAL PHYSICS. Volume: 65 (2020) - 2020 • 6016
Reassessing the rationale behind herbicide biosensors: The case of a photosystem II/redox polymer-based bioelectrodefs
Wang, P. and Zhao, F. and Hartmann, V. and Nowaczyk, M.M. and Ruff, A. and Schuhmann, W. and Conzuelo, F.
BIOELECTROCHEMISTRY. Volume: 136 (2020) - 2020 • 6015
Phase diagram of grain boundary facet and line junctions in silicon
Alam, M. and Lymperakis, L. and Neugebauer, J.
PHYSICAL REVIEW MATERIALS. Volume: 4 (2020) - 2020 • 6014
Effects of size and elasticity on the relation between flow velocity and wall shear stress in side-wall aneurysms: A lattice Boltzmann-based computer simulation study
Wang, H. and Krüger, T. and Varnik, F.
PLOS ONE. Volume: 15 (2020) - 2020 • 6013
Coherence of a Driven Electron Spin Qubit Actively Decoupled from Quasistatic Noise
Nakajima, T. and Noiri, A. and Kawasaki, K. and Yoneda, J. and Stano, P. and Amaha, S. and Otsuka, T. and Takeda, K. and Delbecq, M.R. and Allison, G. and Ludwig, Ar. and Wieck, A.D. and Loss, D. and Tarucha, S.
PHYSICAL REVIEW X. Volume: 10 (2020) - 2020 • 6012
Thermal treatment of lignin, cellulose and hemicellulose in nitrogen and carbon dioxide
Senneca, O. and Cerciello, F. and Russo, C. and Wütscher, A. and Muhler, M. and Apicella, B.
FUEL. Volume: 271 (2020) - 2020 • 6011
Extension of the thermal annealing concepts developed for coal combustion to conversion of lignocellulosic biomass
Senneca, O. and Ontyd, C. and Cerciello, F. and Schiemann, M. and Scherer, V.
ENERGY AND FUELS. Volume: 34 (2020) - 2020 • 6010
Two-dimensional lateral surface superlattices in GaAs heterostructures with independent control of carrier density and modulation potential
Wang, D.Q. and Reuter, D. and Wieck, A.D. and Hamilton, A.R. and Klochan, O.
APPLIED PHYSICS LETTERS. Volume: 117 (2020) - 2020 • 6009
Influence of rf plasma jet surface treatment on wetting behavior of yttria stabilized zirconia sps coatings
Komarov, P. and Jech, D. and Čelko, L. and Pijáková, B. and Zhou, D. and Vaßen, R.
DEFECT AND DIFFUSION FORUM. Volume: 405 DDF (2020) - 2020 • 6008
Microscale plastic anisotropy of basal and pyramidal I slip in pure magnesium tested in shear
Seok, M.-Y. and Gopalan, H. and Nandy, S. and Zaefferer, S. and Raabe, D. and Kirchlechner, C. and Dehm, G.
MATERIALIA. Volume: 14 (2020) - 2020 • 6007
Convolutional restricted Boltzmann machine aided Monte Carlo: An application to Ising and Kitaev models
Alcalde Puente, D. and Eremin, I.M.
PHYSICAL REVIEW B. Volume: 102 (2020) - 2020 • 6006
Kokkos implementation of an Ewald Coulomb solver and analysis of performance portability
Halver, R. and Meinke, J.H. and Sutmann, G.
JOURNAL OF PARALLEL AND DISTRIBUTED COMPUTING. Volume: 138 (2020) - 2020 • 6005
Recent Developments in Small-Scale Shape Memory Oxides
Wang, X. and Ludwig, Al.
SHAPE MEMORY AND SUPERELASTICITY. Volume: 6 (2020) - 2020 • 6004
Combinatorial synthesis of Ni–Mn–Ga-(Fe,Co,Cu) high temperature ferromagnetic shape memory alloys thin films
Alexandrakis, V. and Barandiaran, J.M. and Pérez-Checa, A. and Lázpita, P. and Decker, P. and Salomon, S. and Feuchtwanger, J. and Ludwig, Al. and Chernenko, V.
SCRIPTA MATERIALIA. Volume: 178 (2020) - 2020 • 6003
Comparative proteomic analysis of osteogenic differentiated human adipose tissue and bone marrow-derived stromal cells
Dadras, M. and May, C. and Wagner, J.M. and Wallner, C. and Becerikli, M. and Dittfeld, S. and Serschnitzki, B. and Schilde, L. and Guntermann, A. and Sengstock, C. and Köller, M. and Seybold, D. and Geßmann, J. and Schildhauer, T.A. and Lehnhardt, M. and Marcus, K. and Behr, B.
JOURNAL OF CELLULAR AND MOLECULAR MEDICINE. Volume: 24 (2020) - 2020 • 6002
Examining Performance Portability with Kokkos for an Ewald Sum Coulomb Solver
Halver, R. and Meinke, J.H. and Sutmann, G.
LECTURE NOTES IN COMPUTER SCIENCE (INCLUDING SUBSERIES LECTURE NOTES IN ARTIFICIAL INTELLIGENCE AND LECTURE NOTES IN BIOINFORMATICS). Volume: 12044 LNCS (2020) - 2020 • 6001
Silicon Oxide Barrier Films Deposited on Polycarbonate Substrates in Pulsed Plasmas
Shafaei, S. and Yang, L. and Rudolph, M. and Awakowicz, P.
PLASMA CHEMISTRY AND PLASMA PROCESSING. Volume: 40 (2020) - 2020 • 6000
Anisotropy-Related Machining Characteristics in Ultra-Precision Diamond Cutting of Crystalline Copper
Wang, Z. and Zhang, J. and Li, G. and Xu, Z. and Zhang, H. and Zhang, J. and Hartmaier, A. and Fang, F. and Yan, Y. and Sun, T.
NANOMANUFACTURING AND METROLOGY. Volume: 3 (2020) - 2020 • 5999
Meissner currents induced by topological magnetic textures in hybrid superconductor/ferromagnet structures
Dahir, S.M. and Volkov, A.F. and Eremin, I.M.
PHYSICAL REVIEW B. Volume: 102 (2020) - 2020 • 5998
Experimental and Theoretical Investigation on Phase Formation and Mechanical Properties in Cr-Co-Ni Alloys Processed Using a Novel Thin-Film Quenching Technique
Naujoks, D. and Schneider, M. and Salomon, S. and Pfetzing-Micklich, J. and Subramanyam, A.P.A. and Hammerschmidt, T. and Drautz, R. and Frenzel, J. and Kostka, A. and Eggeler, G. and Laplanche, G. and Ludwig, Al.
ACS COMBINATORIAL SCIENCE. Volume: 22 (2020) - 2020 • 5997
Robust optimization scheme for inverse method for crystal plasticity model parametrization
Shahmardani, M. and Vajragupta, N. and Hartmaier, A.
MATERIALS. Volume: 13 (2020) - 2020 • 5996
Femtosecond laser shock peening on the surface of NiTi shape memory alloy
Wang, H. and Gurevich, E.L. and Ostendorf, A.
PROCEDIA CIRP. Volume: 94 (2020) - 2020 • 5995
Combinatorial Synthesis and High-Throughput Characterization of Microstructure and Phase Transformation in Ni–Ti–Cu–V Quaternary Thin-Film Library
Al Hasan, N.M. and Hou, H. and Sarkar, S. and Thienhaus, S. and Mehta, A. and Ludwig, Al. and Takeuchi, I.
ENGINEERING. Volume: 6 (2020) - 2020 • 5994
Ultrastrong lightweight compositionally complex steels via dual-nanoprecipitation
Wang, Z. and Lu, W. and Zhao, H. and Liebscher, C.H. and He, J. and Ponge, D. and Raabe, D. and Li, Z.
SCIENCE ADVANCES. Volume: 6 (2020) - 2020 • 5993
Combinatorial Exploration and Mapping of Phase Transformation in a Ni-Ti-Co Thin Film Library
Al Hasan, N.M. and Hou, H. and Gao, T. and Counsell, J. and Sarker, S. and Thienhaus, S. and Walton, E. and Decker, P. and Mehta, A. and Ludwig, Al. and Takeuchi, I.
ACS COMBINATORIAL SCIENCE. Volume: 22 (2020) - 2020 • 5992
Using spectral-based representative volume element crystal plasticity simulations to predict yield surface evolution during large scale forming simulations
Han, F. and Diehl, M. and Roters, F. and Raabe, D.
JOURNAL OF MATERIALS PROCESSING TECHNOLOGY. Volume: 277 (2020) - 2020 • 5991
High temperature creep resistance of a thermally stable nanocrystalline Fe-5 at.% Zr steel
Shan, G.B. and Chen, Y.Z. and Li, Y.J. and Zhang, C.Y. and Dong, H. and Cong, Y.B. and Zhang, W.X. and Huang, L.K. and Suo, T. and Liu, F.
SCRIPTA MATERIALIA. Volume: 179 (2020) - 2020 • 5990
Laser shock peening on high-strength steel
Wang, H. and Keller, S. and Bai, Y. and Kashaev, N. and Gurevich, E.L. and Ostendorf, A.
PROCEEDINGS OF SPIE - THE INTERNATIONAL SOCIETY FOR OPTICAL ENGINEERING. Volume: 11546 (2020) - 2020 • 5989
Role of coherency loss on rafting behavior of Ni-based superalloys
Ali, M.A. and Görler, J.V. and Steinbach, I.
COMPUTATIONAL MATERIALS SCIENCE. Volume: 171 (2020) - 2020 • 5988
Microstructure-based multiscale modeling of large strain plastic deformation by coupling a full-field crystal plasticity-spectral solver with an implicit finite element solver
Han, F. and Roters, F. and Raabe, D.
INTERNATIONAL JOURNAL OF PLASTICITY. Volume: 125 (2020) - 2020 • 5987
Multi-component chemo-mechanics based on transport relations for the chemical potential
Shanthraj, P. and Liu, C. and Akbarian, A. and Svendsen, B. and Raabe, D.
COMPUTER METHODS IN APPLIED MECHANICS AND ENGINEERING. Volume: 365 (2020) - 2020 • 5986
Formation mechanism of κ-carbides and deformation behavior in Si-alloyed FeMnAlC lightweight steels
Wang, Z. and Lu, W. and Zhao, H. and He, J. and Wang, K. and Zhou, B. and Ponge, D. and Raabe, D. and Li, Z.
ACTA MATERIALIA. Volume: 198 (2020) - 2020 • 5985
Effect of γ′ precipitate size on hardness and creep properties of Ni-base single crystal superalloys: Experiment and simulation
Ali, M.A. and López-Galilea, I. and Gao, S. and Ruttert, B. and Amin, W. and Shchyglo, O. and Hartmaier, A. and Theisen, W. and Steinbach, I.
MATERIALIA. Volume: 12 (2020) - 2020 • 5984
Long-term heat treatment of collector bars for aluminium electrolysis: impact on microstructure and electrical properties
Hankel, J. and Kernebeck, S. and Deuerler, F. and Weber, S.
SN APPLIED SCIENCES. Volume: 2 (2020) - 2020 • 5983
Helium metastable species generation in atmospheric pressure RF plasma jets driven by tailored voltage waveforms in mixtures of He and N2
Korolov, I. and Leimkühler, M. and Böke, M. and Donkó, Z. and Schulz-Von Der Gathen, V. and Bischoff, L. and Hübner, G. and Hartmann, P. and Gans, T. and Liu, Y. and Mussenbrock, T. and Schulze, J.
JOURNAL OF PHYSICS D: APPLIED PHYSICS. Volume: 53 (2020) - 2020 • 5982
Temperature effects on the ionic conductivity in concentrated alkaline electrolyte solutions
Shao, Y. and Hellström, M. and Yllö, A. and Mindemark, J. and Hermansson, K. and Behler, J. and Zhang, C.
PHYSICAL CHEMISTRY CHEMICAL PHYSICS. Volume: 22 (2020) - 2020 • 5981
45-degree rafting in Ni-based superalloys: A combined phase-field and strain gradient crystal plasticity study
Ali, M.A. and Amin, W. and Shchyglo, O. and Steinbach, I.
INTERNATIONAL JOURNAL OF PLASTICITY. Volume: 128 (2020) - 2020 • 5980
Development of a recycling strategy for grinding sludge using supersolidus liquid phase sintering
Hankel, J. and Jäger, S. and Weber, S.
JOURNAL OF CLEANER PRODUCTION. Volume: 263 (2020) - 2020 • 5979
Electron tunneling dynamics between two-dimensional and zero-dimensional quantum systems: Contributions of momentum matching, higher subbands, and phonon-assisted processes
Korsch, A.R. and Ebler, C. and Nguyen, G.N. and Scholz, S. and Wieck, A.D. and Ludwig, Ar.
PHYSICAL REVIEW B. Volume: 102 (2020) - 2020 • 5978
Anisotropic failure behavior of ordered intermetallic TiAl alloys under pure mode-I loading
Neogi, A. and Alam, M. and Hartmaier, A. and Janisch, R.
MODELLING AND SIMULATION IN MATERIALS SCIENCE AND ENGINEERING. Volume: 28 (2020) - 2020 • 5977
Sol-Gel-Based Hybrid Materials as Antifouling and Fouling-Release Coatings for Marine Applications
Wanka, R. and Koc, J. and Clarke, J. and Hunsucker, K.Z. and Swain, G.W. and Aldred, N. and Finlay, J.A. and Clare, A.S. and Rosenhahn, A.
ACS APPLIED MATERIALS AND INTERFACES. Volume: 12 (2020) - 2020 • 5976
Influence of muck properties and chamber design on pressure distribution in EPB pressure chambers – Insights from computational flow simulations
Dang, T.S. and Meschke, G.
TUNNELLING AND UNDERGROUND SPACE TECHNOLOGY. Volume: 99 (2020) - 2020 • 5975
Data-oriented constitutive modeling of plasticity in metals
Hartmaier, A.
MATERIALS. Volume: 13 (2020) - 2020 • 5974
Global Optimization based on Mixed H2and HinfApproach for Placement of Piezoelectric Actuators and Sensors on Curved Surfaces in Actively Controlled Structures
Nestorovic, T. and Oveisi, A. and Arriessa Sukhairi, T. and Hassw, K.
PROCEEDINGS OF THE 15TH IEEE CONFERENCE ON INDUSTRIAL ELECTRONICS AND APPLICATIONS, ICIEA 2020. Volume: (2020) - 2020 • 5973
Anchoring of palladium nanoparticles on N-doped mesoporous carbon
Warczinski, L. and Hu, B. and Eckhard, T. and Peng, B. and Muhler, M. and Hättig, C.
PHYSICAL CHEMISTRY CHEMICAL PHYSICS. Volume: 22 (2020) - 2020 • 5972
Improved quantum efficiency in an engineered light harvesting/photosystem II super-complex for high current density biophotoanodes
Hartmann, V. and Harris, D. and Bobrowski, T. and Ruff, A. and Frank, A. and Günther Pomorski, T. and Rögner, M. and Schuhmann, W. and Adir, N. and Nowaczyk, M.M.
JOURNAL OF MATERIALS CHEMISTRY A. Volume: 8 (2020) - 2020 • 5971
Amphiphilic Dicyclopentenyl/Carboxybetaine-Containing Copolymers for Marine Fouling-Release Applications
Koschitzki, F. and Wanka, R. and Sobota, L. and Koc, J. and Gardner, H. and Hunsucker, K.Z. and Swain, G.W. and Rosenhahn, A.
ACS APPLIED MATERIALS AND INTERFACES. Volume: 12 (2020) - 2020 • 5970
Spray-Flame-Prepared LaCo1–xFexO3 Perovskite Nanoparticles as Active OER Catalysts: Influence of Fe Content and Low-Temperature Heating
Alkan, B. and Medina, D. and Landers, J. and Heidelmann, M. and Hagemann, U. and Salamon, S. and Andronescu, C. and Wende, H. and Schulz, C. and Schuhmann, W. and Wiggers, H.
CHEMELECTROCHEM. Volume: 7 (2020) - 2020 • 5969
Segregation-assisted spinodal and transient spinodal phase separation at grain boundaries
Darvishi Kamachali, R. and Kwiatkowski da Silva, A. and McEniry, E. and Ponge, D. and Gault, B. and Neugebauer, J. and Raabe, D.
NPJ COMPUTATIONAL MATERIALS. Volume: 6 (2020) - 2020 • 5968
Charged particle dynamics and distribution functions in low pressure dual-frequency capacitively coupled plasmas operated at low frequencies and high voltages
Hartmann, P. and Wang, L. and Nösges, K. and Berger, B. and Wilczek, S. and Brinkmann, R.P. and Mussenbrock, T. and Juhasz, Z. and Donkó, Z. and Derzsi, A. and Lee, E. and Schulze, J.
PLASMA SOURCES SCIENCE AND TECHNOLOGY. Volume: 29 (2020) - 2020 • 5967
Short-range order in face-centered cubic VCoNi alloys
Kostiuchenko, T. and Ruban, A.V. and Neugebauer, J. and Shapeev, A. and Körmann, F.
PHYSICAL REVIEW MATERIALS. Volume: 4 (2020) - 2020 • 5966
A model for grain boundary thermodynamics
Darvishi Kamachali, R.
RSC ADVANCES. Volume: 10 (2020) - 2020 • 5965
Influence of Mineral Composition of Chars Derived by Hydrothermal Carbonization on Sorption Behavior of CO2, CH4, and O2
Wedler, C. and Lotz, K. and Arami-Niya, A. and Xiao, G. and Span, R. and Muhler, M. and May, E.F. and Richter, M.
ACS OMEGA. Volume: 5 (2020) - 2020 • 5964
Self-mode-locking and chirp compensation in an external cavity diode laser at 1550 nm
Alloush, A. and Bassal, A. and Brenner, C. and Fortin, C. and Mekhazni, K. and Gamarra, P. and Calò, C. and Hofmann, M.R.
PROCEEDINGS OF SPIE - THE INTERNATIONAL SOCIETY FOR OPTICAL ENGINEERING. Volume: 11356 (2020) - 2020 • 5963
In-depth particle localization with common-path digital holographic microscopy
Neutsch, K. and Schnitzler, L. and Sun, J. and Tranelis, M.J. and Hofmann, M.R. and Gerhardt, N.C.
PROCEEDINGS OF SPIE - THE INTERNATIONAL SOCIETY FOR OPTICAL ENGINEERING. Volume: 11306 (2020) - 2020 • 5962
Comparison of self-mode-locking in monolithic and external cavity diode laser at 1550 nm
Alloush, M.A. and Bassal, A. and Brenner, C. and Fortin, C. and Mekhazni, K. and Gamarra, P. and Calo, C. and Hofmann, M.R.
PROCEEDINGS OF SPIE - THE INTERNATIONAL SOCIETY FOR OPTICAL ENGINEERING. Volume: 11301 (2020) - 2020 • 5961
Short SiC fiber/Ti3SiC2 MAX phase composites: Fabrication and creep evaluation
Dash, A. and Malzbender, J. and Vaßen, R. and Guillon, O. and Gonzalez-Julian, J.
JOURNAL OF THE AMERICAN CERAMIC SOCIETY. Volume: 103 (2020) - 2020 • 5960
A crossover in spatio-temporal correlations of strain fluctuations in glass forming liquids
Hassani, M. and Bruns, M. and Varnik, F.
JOURNAL OF STATISTICAL MECHANICS: THEORY AND EXPERIMENT. Volume: 2020 (2020) - 2020 • 5959
Forward and inverse problems in piezoelectricity using isogeometric symmetric Galerkin boundary element method and level set method
Nguyen, B.H. and Nanthakumar, S.S. and He, Y.Q. and Tran, H.D. and Hackl, K. and Zhuang, X.
ENGINEERING ANALYSIS WITH BOUNDARY ELEMENTS. Volume: 113 (2020) - 2020 • 5958
Amplitude noise and RF response analysis of 1 GHz mode-locked pulses from an InP-based laser chip at 1550 nm
Alloush, A. and Van Delden, M. and Bassal, A. and Brenner, C. and Musch, T. and Lo, M.C. and Augustin, L. and Guzman, R. and Carpintero, G. and Hofmann, M.R.
2020 IEEE PHOTONICS CONFERENCE, IPC 2020 - PROCEEDINGS. Volume: (2020) - 2020 • 5957
Compressive creep of SiC whisker/Ti3SiC2 composites at high temperature in air
Dash, A. and Malzbender, J. and Dash, K. and Rasinski, M. and Vaßen, R. and Guillon, O. and Gonzalez-Julian, J.
JOURNAL OF THE AMERICAN CERAMIC SOCIETY. Volume: 103 (2020) - 2020 • 5956
A comparative study on the tribological properties of a cobalt-free superaustenitic stainless steel at elevated temperature
van gen Hassend, F. and Weber, S.
METALS. Volume: 10 (2020) - 2020 • 5955
Influence of molecular beam effusion cell quality on optical and electrical properties of quantum dots and quantum wells
Nguyen, G.N. and Korsch, A.R. and Schmidt, M. and Ebler, C. and Labud, P.A. and Schott, R. and Lochner, P. and Brinks, F. and Wieck, A.D. and Ludwig, Ar.
JOURNAL OF CRYSTAL GROWTH. Volume: 550 (2020) - 2020 • 5954
Femtosecond RMS timing jitter from 1 GHz InP on-chip mode-locked laser at 1550 nm
Alloush, A. and Bassal, A. and Brenner, C. and Lo, M.C. and Guzman, R. and Augustin, L. and Carpintero, G. and Hofmann, M.R.
2020 CONFERENCE ON LASERS AND ELECTRO-OPTICS PACIFIC RIM, CLEO-PR 2020 - PROCEEDINGS. Volume: (2020) - 2020 • 5953
Influence of Chemical Inhomogeneities on Local Phase Stabilities and Material Properties in Cast Martensitic Stainless Steel
van gen Hassend, F. and Weber, S.
STEEL RESEARCH INTERNATIONAL. Volume: 91 (2020) - 2020 • 5952
Integrated BIM-to-FEM approach in mechanised tunnelling
Alsahly, A. and Hegemann, F. and König, M. and Meschke, G.
GEOMECHANIK UND TUNNELBAU. Volume: 13 (2020) - 2020 • 5951
Electrocatalytic Reduction of CO2 to Acetic Acid by a Molecular Manganese Corrole Complex
De, R. and Gonglach, S. and Paul, S. and Haas, M. and Sreejith, S.S. and Gerschel, P. and Apfel, U.-P. and Vuong, T.H. and Rabeah, J. and Roy, S. and Schöfberger, W.
ANGEWANDTE CHEMIE - INTERNATIONAL EDITION. Volume: 59 (2020) - 2020 • 5950
Powering Artificial Enzymatic Cascades with Electrical Energy
Al-Shameri, A. and Petrich, M.-C. and junge Puring, K. and Apfel, U.-P. and Nestl, B.M. and Lauterbach, L.
ANGEWANDTE CHEMIE - INTERNATIONAL EDITION. Volume: 59 (2020) - 2020 • 5949
Elastic properties of single crystal Bi12SiO20 as a function of pressure and temperature and acoustic attenuation effects in Bi12 MO20 (M = Si, Ge and Ti)
Haussühl, E. and Reichmann, H.J. and Schreuer, J. and Friedrich, A. and Hirschle, C. and Bayarjargal, L. and Winkler, B. and Alencar, I. and Wiehl, L. and Ganschow, S.
MATERIALS RESEARCH EXPRESS. Volume: 7 (2020) - 2020 • 5948
Fully convolutional neural network for detection and counting of diatoms on coatings after short-term field exposure
Krause, L.M.K. and Koc, J. and Rosenhahn, B. and Rosenhahn, A.
ENVIRONMENTAL SCIENCE AND TECHNOLOGY. Volume: 54 (2020) - 2020 • 5947
Does plasma-induced methionine degradation provide alternative reaction paths for cell death?
Deichmöller, J. and Kogelheide, F. and Murke, S. and Hüther, D. and Schwaab, G. and Awakowicz, P. and Havenith, M.
JOURNAL OF PHYSICS D: APPLIED PHYSICS. Volume: 53 (2020) - 2020 • 5946
Properties of α-Brass Nanoparticles. 1. Neural Network Potential Energy Surface
Weinreich, J. and Römer, A. and Paleico, M.L. and Behler, J.
JOURNAL OF PHYSICAL CHEMISTRY C. Volume: 124 (2020) - 2020 • 5945
Trivalent iron rich CoFe layered oxyhydroxides for electrochemical water oxidation
Weiß, S. and Ertl, M. and Varhade, S.D. and Radha, A.V. and Schuhmann, W. and Breu, J. and Andronescu, C.
ELECTROCHIMICA ACTA. Volume: 350 (2020) - 2020 • 5944
On the atomic solute diffusional mechanisms during compressive creep deformation of a Co-Al-W-Ta single crystal superalloy
He, J. and Zenk, C.H. and Zhou, X. and Neumeier, S. and Raabe, D. and Gault, B. and Makineni, S.K.
ACTA MATERIALIA. Volume: 184 (2020) - 2020 • 5943
Metal-Rich Chalcogenides for Electrocatalytic Hydrogen Evolution: Activity of Electrodes and Bulk Materials
Siegmund, D. and Blanc, N. and Smialkowski, M. and Tschulik, K. and Apfel, U.-P.
CHEMELECTROCHEM. Volume: 7 (2020) - 2020 • 5942
On the rhenium segregation at the low angle grain boundary in a single crystal Ni-base superalloy
He, J. and Scholz, F. and Horst, O.M. and Thome, P. and Frenzel, J. and Eggeler, G. and Gault, B.
SCRIPTA MATERIALIA. Volume: 185 (2020) - 2020 • 5941
Metal-Rich Chalcogenides as Sustainable Electrocatalysts for Oxygen Evolution and Reduction: State of the Art and Future Perspectives
Amin, H.M.A. and Apfel, U.-P.
EUROPEAN JOURNAL OF INORGANIC CHEMISTRY. Volume: 2020 (2020) - 2020 • 5940
Response to Comment on "high-surface-area corundum by mechanochemically induced phase transformation of boehmite"
Amrute, A.P. and Lodziana, Z. and Schreyer, H. and Weidenthaler, C. and Schüth, F.
SCIENCE. Volume: 368 (2020) - 2020 • 5939
Optimal designs for estimating individual coefficients in polynomial regression with no intercept
Dette, H. and Melas, V.B. and Shpilev, P.
STATISTICS AND PROBABILITY LETTERS. Volume: 158 (2020) - 2020 • 5938
BIM-to-IGA: A fully automatic design-through-analysis workflow for segmented tunnel linings
Ninić, J. and Bui, H.G. and Meschke, G.
ADVANCED ENGINEERING INFORMATICS. Volume: 46 (2020) - 2020 • 5937
Industrial feasibility of anodic hydrogen peroxide production through photoelectrochemical water splitting: a techno-economic analysis
Wenderich, K. and Kwak, W. and Grimm, A. and Kramer, G.J. and Mul, G. and Mei, B.
SUSTAINABLE ENERGY AND FUELS. Volume: 4 (2020) - 2020 • 5936
Hydrothermal Stability of High-Surface-Area α-Al2O3and Its Use as a Support for Hydrothermally Stable Fischer-Tropsch Synthesis Catalysts
Amrute, A.P. and Jeske, K. and Łodziana, Z. and Prieto, G. and Schüth, F.
CHEMISTRY OF MATERIALS. Volume: 32 (2020) - 2020 • 5935
Likelihood ratio tests for many groups in high dimensions
Dette, H. and Dörnemann, N.
JOURNAL OF MULTIVARIATE ANALYSIS. Volume: 178 (2020) - 2020 • 5934
Influence of Contaminants in Steel Mill Exhaust Gases on Cu/ZnO/Al2O3 Catalysts Applied in Methanol Synthesis
He, J. and Laudenschleger, D. and Schittkowski, J. and Machoke, A. and Song, H. and Muhler, M. and Schlögl, R. and Ruland, H.
CHEMIE-INGENIEUR-TECHNIK. Volume: 92 (2020) - 2020 • 5933
Plasmachemical Trace-Oxygen Removal in a Coke Oven Gas with a Coaxial Packed-Bed-DBD Reactor
Nitsche, T. and Budt, M. and Apfel, U.-P.
CHEMIE-INGENIEUR-TECHNIK. Volume: 92 (2020) - 2020 • 5932
Thermoregeneration of Plastrons on Superhydrophobic Coatings for Sustained Antifouling Properties
Simovich, T. and Rosenhahn, A. and Lamb, R.N.
ADVANCED ENGINEERING MATERIALS. Volume: 22 (2020) - 2020 • 5931
Mechanochemical Grafting: A Solvent-less Highly Efficient Method for the Synthesis of Hybrid Inorganic-Organic Materials
Amrute, A.P. and Zibrowius, B. and Schüth, F.
CHEMISTRY OF MATERIALS. Volume: 32 (2020) - 2020 • 5930
Testing relevant hypotheses in functional time series via self-normalization
Dette, H. and Kokot, K. and Volgushev, S.
JOURNAL OF THE ROYAL STATISTICAL SOCIETY. SERIES B: STATISTICAL METHODOLOGY. Volume: 82 (2020) - 2020 • 5929
Multiscale change point detection for dependent data
Dette, H. and Eckle, T. and Vetter, M.
SCANDINAVIAN JOURNAL OF STATISTICS. Volume: 47 (2020) - 2020 • 5928
The mechanochemical Scholl reaction as a versatile synthesis tool for the solvent-free generation of microporous polymers
Krusenbaum, A. and Grätz, S. and Bimmermann, S. and Hutsch, S. and Borchardt, L.
RSC ADVANCES. Volume: 10 (2020) - 2020 • 5927
A Likelihood Ratio Approach to Sequential Change Point Detection for a General Class of Parameters
Dette, H. and Gösmann, J.
JOURNAL OF THE AMERICAN STATISTICAL ASSOCIATION. Volume: 115 (2020) - 2020 • 5926
Factors Governing the Activity of α-MnO2 Catalysts in the Oxygen Evolution Reaction: Conductivity versus Exposed Surface Area of Cryptomelane
Heese-Gärtlein, J. and Morales, D.M. and Rabe, A. and Bredow, T. and Schuhmann, W. and Behrens, M.
CHEMISTRY - A EUROPEAN JOURNAL. Volume: 26 (2020) - 2020 • 5925
Tuning Light-Driven Water Oxidation Efficiency of Molybdenum-Doped BiVO4 by Means of Multicomposite Catalysts Containing Nickel, Iron, and Chromium Oxides
Krysiak, O.A. and Junqueira, J.R.C. and Conzuelo, F. and Bobrowski, T. and Wilde, P. and Wysmolek, A. and Schuhmann, W.
CHEMPLUSCHEM. Volume: 85 (2020) - 2020 • 5924
High-Throughput Characterization of Structural and Photoelectrochemical Properties of a Bi-Mo-W-O Thin-Film Materials Library
Nowak, M. and Gutkowski, R. and Junqueira, J. and Schuhmann, W. and Ludwig, A.
ZEITSCHRIFT FUR PHYSIKALISCHE CHEMIE. Volume: 234 (2020) - 2020 • 5923
Prediction in Locally Stationary Time Series
Dette, H. and Wu, W.
JOURNAL OF BUSINESS AND ECONOMIC STATISTICS. Volume: (2020) - 2020 • 5922
Atomic relaxation around defects in magnetically disordered materials computed by atomic spin constraints within an efficient Lagrange formalism
Hegde, O. and Grabowski, M. and Zhang, X. and Waseda, O. and Hickel, T. and Freysoldt, C. and Neugebauer, J.
PHYSICAL REVIEW B. Volume: 102 (2020) - 2020 • 5921
Role of image charges in ionic liquid confined between metallic interfaces
Ntim, S. and Sulpizi, M.
PHYSICAL CHEMISTRY CHEMICAL PHYSICS. Volume: 22 (2020) - 2020 • 5920
Dominant In-Plane Symmetric Elastoresistance in CsFe2As2
Wiecki, P. and Haghighirad, A.-A. and Weber, F. and Merz, M. and Heid, R. and Böhmer, A.E.
PHYSICAL REVIEW LETTERS. Volume: 125 (2020) - 2020 • 5919
Functional data analysis in the banach space of continuous functions
Dette, H. and Kokot, K. and Aue, A.
ANNALS OF STATISTICS. Volume: 48 (2020) - 2020 • 5918
Microstructure, texture, and strength development during high-pressure torsion of crmnfeconi high-entropy alloy
Skrotzki, W. and Pukenas, A. and Odor, E. and Joni, B. and Ungar, T. and Völker, B. and Hohenwarter, A. and Pippan, R. and George, E.P.
CRYSTALS. Volume: 10 (2020) - 2020 • 5917
Estimating a Change Point in a Sequence of Very High-Dimensional Covariance Matrices
Dette, H. and Pan, G. and Yang, Q.
JOURNAL OF THE AMERICAN STATISTICAL ASSOCIATION. Volume: (2020) - 2020 • 5916
The magnetic asymmetry effect in geometrically asymmetric capacitively coupled radio frequency discharges operated in Ar/O2
Oberberg, M. and Berger, B. and Buschheuer, M. and Engel, D. and Wölfel, C. and Eremin, D. and Lunze, J. and Brinkmann, R.P. and Awakowicz, P. and Schulze, J.
PLASMA SOURCES SCIENCE AND TECHNOLOGY. Volume: 29 (2020) - 2020 • 5915
Atomic scale configuration of planar defects in the Nb-rich C14 Laves phase NbFe2
Šlapáková, M. and Zendegani, A. and Liebscher, C.H. and Hickel, T. and Neugebauer, J. and Hammerschmidt, T. and Ormeci, A. and Grin, J. and Dehm, G. and Kumar, K.S. and Stein, F.
ACTA MATERIALIA. Volume: 183 (2020) - 2020 • 5914
Study of the transition from self-organised to homogeneous plasma distribution in chromium HiPIMS discharge
Šlapanská, M. and Hecimovic, A. and Gudmundsson, J.T. and Hnilica, J. and Breilmann, W. and Vašina, P. and Von Keudell, A.
JOURNAL OF PHYSICS D: APPLIED PHYSICS. Volume: 53 (2020) - 2020 • 5913
On the reversible deactivation of cobalt ferrite spinel nanoparticles applied in selective 2-propanol oxidation
Anke, S. and Falk, T. and Bendt, G. and Sinev, I. and Hävecker, M. and Antoni, H. and Zegkinoglou, I. and Jeon, H. and Knop-Gericke, A. and Schlögl, R. and Roldan Cuenya, B. and Schulz, S. and Muhler, M.
JOURNAL OF CATALYSIS. Volume: 382 (2020) - 2020 • 5912
High-strength Damascus steel by additive manufacturing
Kürnsteiner, P. and Wilms, M.B. and Weisheit, A. and Gault, B. and Jägle, E.A. and Raabe, D.
NATURE. Volume: 582 (2020) - 2020 • 5911
Thin-Film Microtensile-Test Structures for High-Throughput Characterization of Mechanical Properties
Oellers, T. and Arigela, V.G. and Kirchlechner, C. and Dehm, G. and Ludwig, Al.
ACS COMBINATORIAL SCIENCE. Volume: 22 (2020) - 2020 • 5910
CoFe-OH Double Hydroxide Films Electrodeposited on Ni-Foam as Electrocatalyst for the Oxygen Evolution Reaction
Sliozberg, K. and Aniskevich, Y. and Kayran, U. and Masa, J. and Schuhmann, W.
ZEITSCHRIFT FUR PHYSIKALISCHE CHEMIE. Volume: 234 (2020) - 2020 • 5909
Electron dynamics in low pressure capacitively coupled radio frequency discharges
Wilczek, S. and Schulze, J. and Brinkmann, R.P. and Donkó, Z. and Trieschmann, J. and Mussenbrock, T.
JOURNAL OF APPLIED PHYSICS. Volume: 127 (2020) - 2020 • 5908
Insights on the role of primary and secondary tar reactions in soot inception during fast pyrolysis of coal
Apicella, B. and Russo, C. and Cerciello, F. and Stanzione, F. and Ciajolo, A. and Scherer, V. and Senneca, O.
FUEL. Volume: 275 (2020) - 2020 • 5907
Chemical boundary engineering: A new route toward lean, ultrastrong yet ductile steels
Ding, R. and Yao, Y. and Sun, B. and Liu, G. and He, J. and Li, T. and Wan, X. and Dai, Z. and Ponge, D. and Raabe, D. and Zhang, C. and Godfrey, A. and Miyamoto, G. and Furuhara, T. and Yang, Z. and van der Zwaag, S. and Chen, H.
SCIENCE ADVANCES. Volume: 6 (2020) - 2020 • 5906
Control of thermally stable core-shell nano-precipitates in additively manufactured Al-Sc-Zr alloys
Kürnsteiner, P. and Bajaj, P. and Gupta, A. and Wilms, M.B. and Weisheit, A. and Li, X. and Leinenbach, C. and Gault, B. and Jägle, E.A. and Raabe, D.
ADDITIVE MANUFACTURING. Volume: 32 (2020) - 2020 • 5905
Elevated temperature microstructure evolution of a medium-entropy CrCoNi superalloy containing Al,Ti
Slone, C.E. and George, E.P. and Mills, M.J.
JOURNAL OF ALLOYS AND COMPOUNDS. Volume: 817 (2020) - 2020 • 5904
Insights into the Formation, Chemical Stability, and Activity of Transient NiyP@NiO x Core-Shell Heterostructures for the Oxygen Evolution Reaction
Wilde, P. and Dieckhöfer, S. and Quast, T. and Xiang, W. and Bhatt, A. and Chen, Y.-T. and Seisel, S. and Barwe, S. and Andronescu, C. and Li, T. and Schuhmann, W. and Masa, J.
ACS APPLIED ENERGY MATERIALS. Volume: 3 (2020) - 2020 • 5903
Effect of Oxygen on High-temperature Phase Equilibria in Ternary Ti-Al-Nb Alloys
Distl, B. and Dehm, G. and Stein, F.
ZEITSCHRIFT FUR ANORGANISCHE UND ALLGEMEINE CHEMIE. Volume: 646 (2020) - 2020 • 5902
Deactivating deformation twinning in medium-entropy CrCoNi with small additions of aluminum and titanium
Slone, C.E. and LaRosa, C.R. and Zenk, C.H. and George, E.P. and Ghazisaeidi, M. and Mills, M.J.
SCRIPTA MATERIALIA. Volume: 178 (2020) - 2020 • 5901
Pattern Formation in High Power Impulse Magnetron Sputtering (HiPIMS) Plasmas
Held, J. and von Keudell, A.
PLASMA CHEMISTRY AND PLASMA PROCESSING. Volume: 40 (2020) - 2020 • 5900
Catalytic oxidation of small organic molecules by cold plasma in solution in the presence of molecular iron complexes†
Śmiłowicz, D. and Kogelheide, F. and Schöne, A.L. and Stapelmann, K. and Awakowicz, P. and Metzler-Nolte, N.
SCIENTIFIC REPORTS. Volume: 10 (2020) - 2020 • 5899
High-throughput characterization of Ag–V–O nanostructured thin-film materials libraries for photoelectrochemical solar water splitting
Kumari, S. and Helt, L. and Junqueira, J.R.C. and Kostka, A. and Zhang, S. and Sarker, S. and Mehta, A. and Scheu, C. and Schuhmann, W. and Ludwig, Al.
INTERNATIONAL JOURNAL OF HYDROGEN ENERGY. Volume: 45 (2020) - 2020 • 5898
Cell-biological effects of zinc oxide spheres and rods from the nano- to the microscale at sub-toxic levels
Olejnik, M. and Kersting, M. and Rosenkranz, N. and Loza, K. and Breisch, M. and Rostek, A. and Prymak, O. and Schürmeyer, L. and Westphal, G. and Köller, M. and Bünger, J. and Epple, M. and Sengstock, C.
CELL BIOLOGY AND TOXICOLOGY. Volume: (2020) - 2020 • 5897
An experimentally validated neural-network potential energy surface for H-atom on free-standing graphene in full dimensionality
Wille, S. and Jiang, H. and Bünermann, O. and Wodtke, A.M. and Behler, J. and Kandratsenka, A.
PHYSICAL CHEMISTRY CHEMICAL PHYSICS. Volume: 22 (2020) - 2020 • 5896
Velocity distribution of metal ions in the target region of HiPIMS: The role of Coulomb collisions
Held, J. and Thiemann-Monjé, S. and Von Keudell, A. and Schulz-Von Der Gathen, V.
PLASMA SOURCES SCIENCE AND TECHNOLOGY. Volume: 29 (2020) - 2020 • 5895
Structural and photoelectrochemical properties in the thin film system Cu-Fe-V-O and its ternary subsystems Fe-V-O and Cu-V-O
Kumari, S. and Junqueira, J.R.C. and Sarker, S. and Mehta, A. and Schuhmann, W. and Ludwig, Al.
JOURNAL OF CHEMICAL PHYSICS. Volume: 153 (2020) - 2020 • 5894
Fundamental Properties and Applications of Dielectric Barrier Discharges in Plasma-Catalytic Processes at Atmospheric Pressure
Ollegott, K. and Wirth, P. and Oberste-Beulmann, C. and Awakowicz, P. and Muhler, M.
CHEMIE-INGENIEUR-TECHNIK. Volume: 92 (2020) - 2020 • 5893
Electron density, temperature and the potential structure of spokes in HiPIMS
Held, J. and Maaß, P.A. and Gathen, V.S.-V.D. and Keudell, A.V.
PLASMA SOURCES SCIENCE AND TECHNOLOGY. Volume: 29 (2020) - 2020 • 5892
Combinatorial Search for New Solar Water Splitting Photoanode Materials in the Thin-Film System Fe-Ti-W-O
Kumari, S. and Khare, C. and Xi, F. and Nowak, M. and Sliozberg, K. and Gutkowski, R. and Bassi, P.S. and Fiechter, S. and Schuhmann, W. and Ludwig, Al .
ZEITSCHRIFT FUR PHYSIKALISCHE CHEMIE. Volume: 234 (2020) - 2020 • 5891
Atomistic description of self-diffusion in molybdenum: A comparative theoretical study of non-Arrhenius behavior
Smirnova, D. and Starikov, S. and Leines, G.D. and Liang, Y. and Wang, N. and Popov, M.N. and Abrikosov, I.A. and Sangiovanni, D.G. and Drautz, R. and Mrovec, M.
PHYSICAL REVIEW MATERIALS. Volume: 4 (2020) - 2020 • 5890
Ab initio Description of Bond Breaking in Large Electric Fields
Ashton, M. and Mishra, A. and Neugebauer, J. and Freysoldt, C.
PHYSICAL REVIEW LETTERS. Volume: 124 (2020) - 2020 • 5889
Laser metal deposition of refractory high-entropy alloys for high-throughput synthesis and structure-property characterization
Dobbelstein, H. and George, E.P. and Gurevich, E.L. and Kostka, A. and Ostendorf, A. and Laplanche, G.
INTERNATIONAL JOURNAL OF EXTREME MANUFACTURING. Volume: 3 (2020) - 2020 • 5888
High-Throughput Exploration of Metal Vanadate Thin-Film Systems (M-V-O, M = Cu, Ag, W, Cr, Co, Fe) for Solar Water Splitting: Composition, Structure, Stability, and Photoelectrochemical Properties
Kumari, S. and Junqueira, J.R.C. and Schuhmann, W. and Ludwig, Al.
ACS COMBINATORIAL SCIENCE. Volume: 22 (2020) - 2020 • 5887
Impact of magnetism on the phase stability of rare-earth based hard magnetic materials
Sözen, H.İ. and Hickel, T. and Neugebauer, J.
CALPHAD: COMPUTER COUPLING OF PHASE DIAGRAMS AND THERMOCHEMISTRY. Volume: 68 (2020) - 2020 • 5886
Quantum-phase-field: From de broglie-bohm double-solution program to doublon networks
Kundin, J. and Steinbach, I.
ZEITSCHRIFT FUR NATURFORSCHUNG - SECTION A JOURNAL OF PHYSICAL SCIENCES. Volume: 75 (2020) - 2020 • 5885
Crystal structure analysis and high-temperature phase transitions of complex rare-earth perovskite, La2(Al1/2MgTa1/2)O6
Sohn, Y.J. and Mauer, G. and Roth, G. and Guillon, O. and Vaßen, R.
JOURNAL OF THE AMERICAN CERAMIC SOCIETY. Volume: 103 (2020) - 2020 • 5884
Impact of coating layers in rotary cement kilns: Numerical investigation with a blocked-off region approach for radiation and momentum
Wirtz, S. and Pieper, C. and Buss, F. and Schiemann, M. and Schaefer, S. and Scherer, V.
THERMAL SCIENCE AND ENGINEERING PROGRESS. Volume: 15 (2020) - 2020 • 5883
High-Dimensional Neural Network Potentials for Atomistic Simulations
Hellström, M. and Behler, J.
LECTURE NOTES IN PHYSICS. Volume: 968 (2020) - 2020 • 5882
Directed Energy Deposition of PA12 carbon nanotube composite powder using a fiber laser
Kutlu, Y. and Wencke, Y.L. and Luinstra, G.A. and Esen, C. and Ostendorf, A.
PROCEDIA CIRP. Volume: 94 (2020) - 2020 • 5881
New phosphorous-based [fefe]-hydrogenase models
Wittkamp, F. and Boydas, E.B. and Roemelt, M. and Apfel, U.-P.
CATALYSTS. Volume: 10 (2020) - 2020 • 5880
Atomic cluster expansion of scalar, vectorial, and tensorial properties including magnetism and charge transfer
Drautz, R.
PHYSICAL REVIEW B. Volume: 102 (2020) - 2020 • 5879
Hydrogen resistance of a 1 GPa strong equiatomic CoCrNi medium entropy alloy
Soundararajan, C.K. and Luo, H. and Raabe, D. and Li, Z.
CORROSION SCIENCE. Volume: 167 (2020) - 2020 • 5878
Grain boundary segregation, phase formation, and their influence on the coercivity of rapidly solidified SmF e11Ti hard magnetic alloys
Palanisamy, D. and Ener, S. and Maccari, F. and Schäfer, L. and Skokov, K.P. and Gutfleisch, O. and Raabe, D. and Gault, B.
PHYSICAL REVIEW MATERIALS. Volume: 4 (2020) - 2020 • 5877
The impact of grain-scale strain localization on strain hardening of a high-Mn steel: Real-time tracking of the transition from the γ → ε → α’ transformation to twinning
Souza Filho, I.R. and Dutta, A. and Almeida Junior, D.R. and Lu, W. and Sandim, M.J.R. and Ponge, D. and Sandim, H.R.Z. and Raabe, D.
ACTA MATERIALIA. Volume: 197 (2020) - 2020 • 5876
In-situ control of microdischarge characteristics in unipolar pulsed plasma electrolytic oxidation of aluminum
Hermanns, P. and Boeddeker, S. and Bracht, V. and Bibinov, N. and Awakowicz, P.
JOURNAL OF PHYSICS D: APPLIED PHYSICS. Volume: 53 (2020) - 2020 • 5875
Global optimization of copper clusters at the ZnO(10 1 ¯ 0) surface using a DFT-based neural network potential and genetic algorithms
Paleico, M.L. and Behler, J.
JOURNAL OF CHEMICAL PHYSICS. Volume: 153 (2020) - 2020 • 5874
Formation and behaviour of plasma spots on the surface of titanium film
Hermanns, P. and Kogelheide, F. and Bracht, V. and Ries, S. and Krüger, F. and Böddeker, S. and Bibinov, N. and Awakowicz, P.
JOURNAL OF PHYSICS D: APPLIED PHYSICS. Volume: 54 (2020) - 2020 • 5873
A flexible and adaptive grid algorithm for global optimization utilizing basin hopping Monte Carlo
Paleico, M.L. and Behler, J.
JOURNAL OF CHEMICAL PHYSICS. Volume: 152 (2020) - 2020 • 5872
Electrochemically Induced pH Change: Time-Resolved Confocal Fluorescence Microscopy Measurements and Comparison with Numerical Model
Pande, N. and Chandrasekar, S.K. and Lohse, D. and Mul, G. and Wood, J.A. and Mei, B.T. and Krug, D.
JOURNAL OF PHYSICAL CHEMISTRY LETTERS. Volume: 11 (2020) - 2020 • 5871
Resistance of pure and mixed rare earth silicates against calcium-magnesium-aluminosilicate (CMAS): A comparative study
Wolf, M. and Mack, D.E. and Guillon, O. and Vaßen, R.
JOURNAL OF THE AMERICAN CERAMIC SOCIETY. Volume: 103 (2020) - 2020 • 5870
Phase Transformation-Induced Changes in Microstructure and Residual Stresses in Thermally Sprayed MnCoFeO4 Protective Coatings
Back, H.C. and Gibmeier, J. and Vaßen, R.
JOURNAL OF THERMAL SPRAY TECHNOLOGY. Volume: 29 (2020) - 2020 • 5869
Transferability of process parameters in laser powder bed fusion processes for an energy and cost efficient manufacturing
Pannitz, O. and Sehrt, J.T.
SUSTAINABILITY (SWITZERLAND). Volume: 12 (2020) - 2020 • 5868
Microchemistry-dependent simulation of yield stress and flow stress in non-heat treatable Al sheet alloys
Wong, S.L. and Laptyeva, G. and Brüggemann, T. and Engler, O. and Roters, F. and Raabe, D. and Karhausen, K.-F.
MODELLING AND SIMULATION IN MATERIALS SCIENCE AND ENGINEERING. Volume: 28 (2020) - 2020 • 5867
Operando Studies of the Electrochemical Dissolution of Silver Nanoparticles in Nitrate Solutions Observed With Hyperspectral Dark-Field Microscopy
Wonner, K. and Rurainsky, C. and Tschulik, K.
FRONTIERS IN CHEMISTRY. Volume: 7 (2020) - 2020 • 5866
A test for separability in covariance operators of random surfaces
Bagchi, P. and Dette, H.
ANNALS OF STATISTICS. Volume: 48 (2020) - 2020 • 5865
Interdiffusion in Cr–Fe–Co–Ni medium-entropy alloys
Durand, A. and Peng, L. and Laplanche, G. and Morris, J.R. and George, E.P. and Eggeler, G.
INTERMETALLICS. Volume: 122 (2020) - 2020 • 5864
The importance of nanoscale confinement to electrocatalytic performance
Wordsworth, J. and Benedetti, T.M. and Alinezhad, A. and Tilley, R.D. and Edwards, M.A. and Schuhmann, W. and Gooding, J.J.
CHEMICAL SCIENCE. Volume: 11 (2020) - 2020 • 5863
Microscopic model for the stacking-fault potential and the exciton wave function in GaAs
Durnev, M.V. and Glazov, M.M. and Linpeng, X. and Viitaniemi, M.L.K. and Matthews, B. and Spurgeon, S.R. and Sushko, P.V. and Wieck, A.D. and Ludwig, Ar. and Fu, K.-M.C.
PHYSICAL REVIEW B. Volume: 101 (2020) - 2020 • 5862
BOPcat software package for the construction and testing of tight-binding models and bond-order potentials
Ladines, A.N. and Hammerschmidt, T. and Drautz, R.
COMPUTATIONAL MATERIALS SCIENCE. Volume: 173 (2020) - 2020 • 5861
Measurement of thermal transport properties of selected superlattice and thin films using frequency-domain photothermal infrared radiometry
Pawlak, M. and Jukam, N. and Kruck, T. and Dziczek, D. and Ludwig, Ar. and Wieck, A.D.
MEASUREMENT: JOURNAL OF THE INTERNATIONAL MEASUREMENT CONFEDERATION. Volume: 166 (2020) - 2020 • 5860
Temperature-dependent change of the fractal dimension of Cu dendrites on Cu(111)
Sprodowski, C. and Morgenstern, K.
NEW JOURNAL OF PHYSICS. Volume: 22 (2020) - 2020 • 5859
A new metalorganic chemical vapor deposition process for MoS2with a 1,4-diazabutadienyl stabilized molybdenum precursor and elemental sulfur
Wree, J.-L. and Ciftyurek, E. and Zanders, D. and Boysen, N. and Kostka, A. and Rogalla, D. and Kasischke, M. and Ostendorf, A. and Schierbaum, K. and Devi, A.
DALTON TRANSACTIONS. Volume: 49 (2020) - 2019 • 5858
YAlO3-a novel environmental barrier coating for Al2O3/Al2O3-ceramic matrix composites
Gatzen, C. and Mack, D.E. and Guillon, O. and Vaßen, R.
COATINGS. Volume: 9 (2019) - 2019 • 5857
Stress-induced formation of TCP phases during high temperature low cycle fatigue loading of the single-crystal Ni-base superalloy ERBO/1
Meid, C. and Eggeler, M. and Watermeyer, P. and Kostka, A. and Hammerschmidt, T. and Drautz, R. and Eggeler, G. and Bartsch, M.
ACTA MATERIALIA. Volume: 168 (2019) - 2019 • 5856
On the influence of the heat treatment on microstructure formation and mechanical properties of near-α Ti-Fe alloys
Sandlöbes, S. and Korte-Kerzel, S. and Raabe, D.
MATERIALS SCIENCE AND ENGINEERING A. Volume: 748 (2019) - 2019 • 5855
Combinatorial Synthesis of Binary Nanoparticles in Ionic Liquids by Cosputtering and Mixing of Elemental Nanoparticles
Meischein, M. and Garzón-Manjón, A. and Frohn, T. and Meyer, H. and Salomon, S. and Scheu, C. and Ludwig, Al.
ACS COMBINATORIAL SCIENCE. Volume: (2019) - 2019 • 5854
Superioniclike diffusion in an elemental crystal: Bcc Titanium
Sangiovanni, D.G. and Klarbring, J. and Smirnova, D. and Skripnyak, N.V. and Gambino, D. and Mrovec, M. and Simak, S.I. and Abrikosov, I.A.
PHYSICAL REVIEW LETTERS. Volume: 123 (2019) - 2019 • 5853
Configurational-force interface model for brittle fracture propagation
Khisamitov, I. and Meschke, G.
COMPUTER METHODS IN APPLIED MECHANICS AND ENGINEERING. Volume: 351 (2019) - 2019 • 5852
Enhanced sensitivity of scanning bipolar electrochemical microscopy for O2 detection
Santos, C.S. and Conzuelo, F. and Eßmann, V. and Bertotti, M. and Schuhmann, W.
ANALYTICA CHIMICA ACTA. Volume: 1087 (2019) - 2019 • 5851
Selective Delamination of Thin Films from Ceramic Surfaces upon Femtosecond Laser Ablation
Kiel, F. and Bulgakova, N.M. and Ostendorf, A. and Gurevich, E.L.
2019 CONFERENCE ON LASERS AND ELECTRO-OPTICS, CLEO 2019 - PROCEEDINGS. Volume: (2019) - 2019 • 5850
Electron-phonon coupling and superconductivity-induced distortion of the phonon lineshape in V3Si
Sauer, A. and Zocco, D.A. and Said, A.H. and Heid, R. and Böhmer, A. and Weber, F.
PHYSICAL REVIEW B. Volume: 99 (2019) - 2019 • 5849
Bimetallic silver-platinum nanoparticles with combined osteo-promotive and antimicrobial activity
Breisch, M. and Grasmik, V. and Loza, K. and Pappert, K. and Rostek, A. and Ziegler, N. and Ludwig, Al. and Heggen, M. and Epple, M. and Tiller, J.C. and Schildhauer, T.A. and Köller, M. and Sengstock, C.
NANOTECHNOLOGY. Volume: 30 (2019) - 2019 • 5848
Selective Delamination upon Femtosecond Laser Ablation of Ceramic Surfaces
Kiel, F. and Bulgakova, N.M. and Ostendorf, A. and Gurevich, E.L.
PHYSICAL REVIEW APPLIED. Volume: 11 (2019) - 2019 • 5847
Big data and simulation – a new approach for real-time TBM steering
Meschke, G. and Cao, B.T. and Freitag, S. and Egorov, A. and Saadallah, A. and Morik, K.
TUNNELS AND UNDERGROUND CITIES: ENGINEERING AND INNOVATION MEET ARCHAEOLOGY, ARCHITECTURE AND ART- PROCEEDINGS OF THE WTC 2019 ITA-AITES WORLD TUNNEL CONGRESS. Volume: (2019) - 2019 • 5846
Micro fracture investigations of white etching layers
Saxena, A.K. and Kumar, A. and Herbig, M. and Brinckmann, S. and Dehm, G. and Kirchlechner, C.
MATERIALS AND DESIGN. Volume: 180 (2019) - 2019 • 5845
New challenges in concrete technology [Neue Herausforderungen in der Betontechnik]
Breitenbücher, R.
BETON- UND STAHLBETONBAU. Volume: 114 (2019) - 2019 • 5844
High-entropy alloys
George, E.P. and Raabe, D. and Ritchie, R.O.
NATURE REVIEWS MATERIALS. Volume: 4 (2019) - 2019 • 5843
Robust segmental lining design – Potentials of advanced numerical simulations for the design of TBM driven tunnels
Meschke, G. and Neu, G.E. and Marwan, A.
GEOMECHANIK UND TUNNELBAU. Volume: 12 (2019) - 2019 • 5842
Magnetic structure of Nd in NdFeAsO studied by X-ray resonant magnetic scattering
Kim, M.G. and Kim, J.-W. and Yan, J.-Q. and Goldman, A.I. and Kreyssig, A.
PHYSICAL REVIEW B. Volume: 100 (2019) - 2019 • 5841
Monolithic Mode-Locked Laser Diode for THz Communication
Gerling, A. and Tybussek, K. and Gaimard, Q. and Merghem, K. and Ramdane, A. and Hofmann, M.R. and Brenner, C. and Balzer, J.C.
INTERNATIONAL CONFERENCE ON INFRARED, MILLIMETER, AND TERAHERTZ WAVES, IRMMW-THZ. Volume: 2019-September (2019) - 2019 • 5840
Golomb ruler based discrete frequency multimodal continuous wave THz spectroscopy system
Gerling, A. and Becke, L. and Tonder, S. and Hofmann, M.R. and Balzer, J.C. and Brenner, C.
2019 2ND INTERNATIONAL WORKSHOP ON MOBILE TERAHERTZ SYSTEMS, IWMTS 2019. Volume: (2019) - 2019 • 5839
Accurate Probabilities for Highly Activated Reaction of Polyatomic Molecules on Surfaces Using a High-Dimensional Neural Network Potential: CHD 3 + Cu(111)
Gerrits, N. and Shakouri, K. and Behler, J. and Kroes, G.-J.
JOURNAL OF PHYSICAL CHEMISTRY LETTERS. Volume: 10 (2019) - 2019 • 5838
Micromechanical modelling of the cyclic deformation behavior of martensitic sae 4150-a comparison of different kinematic hardening models
Schäfer, B.J. and Song, X. and Sonnweber-Ribic, P. and Hassan, H.U. and Hartmaier, A.
METALS. Volume: 9 (2019) - 2019 • 5837
Sulfur substitution in a Ni(cyclam) derivative results in lower overpotential for CO2 reduction and enhanced proton reduction
Gerschel, P. and Warm, K. and Farquhar, E.R. and Englert, U. and Reback, M.L. and Siegmund, D. and Ray, K. and Apfel, U.-P.
DALTON TRANSACTIONS. Volume: 48 (2019) - 2019 • 5836
Fatigue behavior of HPC and FRC under cyclic tensile loading: Experiments and modeling
Schäfer, N. and Gudžulić, V. and Timothy, J.J. and Breitenbücher, R. and Meschke, G.
STRUCTURAL CONCRETE. Volume: 20 (2019) - 2019 • 5835
Tribolayer formation during macro- and microscale cyclic contact
Brinckmann, S. and Stratmann, A. and Dehm, G. and Jacobs, G.
TRIBOLOGY INTERNATIONAL. Volume: 129 (2019) - 2019 • 5834
Atomistic phase field chemomechanical modeling of dislocation-solute-precipitate interaction in Ni–Al–Co
Mianroodi, J.R. and Shanthraj, P. and Kontis, P. and Cormier, J. and Gault, B. and Svendsen, B. and Raabe, D.
ACTA MATERIALIA. Volume: 175 (2019) - 2019 • 5833
Micromechanical modelling of the influence of strain ratio on fatigue crack initiation in a martensitic steel-a comparison of different fatigue indicator parameters
Schäfer, B.J. and Sonnweber-Ribic, P. and ul Hassan, H. and Hartmaier, A.
MATERIALS. Volume: 12 (2019) - 2019 • 5832
Towards quantifying the shear delamination of thin films
Brinckmann, S. and Soler, R. and Dehm, G.
MATERIALIA. Volume: 8 (2019) - 2019 • 5831
Mechanical characterisation of the protective Al2O3 scale in Cr2AlC MAX phases
Gibson, J.S.K.-L. and Gonzalez-Julian, J. and Krishnan, S. and Vaßen, R. and Korte-Kerzel, S.
JOURNAL OF THE EUROPEAN CERAMIC SOCIETY. Volume: (2019) - 2019 • 5830
Micromechanical modeling of fatigue crack nucleation around non-metallic inclusions in martensitic high-strength steels
Schäfer, B.J. and Sonnweber-Ribic, P. and Ul-Hassan, H. and Hartmaier, A.
METALS. Volume: 9 (2019) - 2019 • 5829
Disrupting the spatio-temporal symmetry of the electron dynamics in atmospheric pressure plasmas by voltage waveform tailoring
Gibson, A.R. and Donkó, Z. and Alelyani, L. and Bischoff, L. and Hübner, G. and Bredin, J. and Doyle, S. and Korolov, I. and Niemi, K. and Mussenbrock, T. and Hartmann, P. and Dedrick, J.P. and Schulze, J. and Gans, T. and O'Connell, D.
PLASMA SOURCES SCIENCE AND TECHNOLOGY. Volume: 28 (2019) - 2019 • 5828
Extremely Robust Gas-Quenching Deposition of Halide Perovskites on Top of Hydrophobic Hole Transport Materials for Inverted (p-i-n) Solar Cells by Targeting the Precursor Wetting Issue
Brinkmann, K.O. and He, J. and Schubert, F. and Malerczyk, J. and Kreusel, C. and Van Gen Hassend, F. and Weber, S. and Song, J. and Qu, J. and Riedl, T.
ACS APPLIED MATERIALS AND INTERFACES. Volume: 11 (2019) - 2019 • 5827
Understanding the Acidic Properties of the Amorphous Hydroxylated Silica Surface
Gierada, M. and De Proft, F. and Sulpizi, M. and Tielens, F.
JOURNAL OF PHYSICAL CHEMISTRY C. Volume: 123 (2019) - 2019 • 5826
Time-Dependent Photoluminescence of Nanostructured Anatase TiO2 and the Role of Bulk and Surface Processes
Brüninghoff, R. and Wenderich, K. and Korterik, J.P. and Mei, B.T. and Mul, G. and Huijser, A.
JOURNAL OF PHYSICAL CHEMISTRY C. Volume: 123 (2019) - 2019 • 5825
Influence of different annealing atmospheres on the mechanical properties of freestanding MCrALY bond coats investigated by micro-tensile creep tests
Giese, S. and Neumeier, S. and Bergholz, J. and Naumenko, D. and Quadakkers, W.J. and Vaßen, R. and Göken, M.
METALS. Volume: 9 (2019) - 2019 • 5824
Integration of Molybdenum-Doped, Hydrogen-Annealed BiVO 4 with Silicon Microwires for Photoelectrochemical Applications
Milbrat, A. and Vijselaar, W. and Guo, Y. and Mei, B. and Huskens, J. and Mul, G.
ACS SUSTAINABLE CHEMISTRY AND ENGINEERING. Volume: 7 (2019) - 2019 • 5823
Polyacrylamide Bead Sensors for in vivo Quantification of Cell-Scale Stress in Zebrafish Development
Träber, N. and Uhlmann, K. and Girardo, S. and Kesavan, G. and Wagner, K. and Friedrichs, J. and Goswami, R. and Bai, K. and Brand, M. and Werner, C. and Balzani, D. and Guck, J.
SCIENTIFIC REPORTS. Volume: 9 (2019) - 2019 • 5822
Comparative Analysis of Photocatalytic and Electrochemical Degradation of 4-Ethylphenol in Saline Conditions
Brüninghoff, R. and Van Duijne, A.K. and Braakhuis, L. and Saha, P. and Jeremiasse, A.W. and Mei, B. and Mul, G.
ENVIRONMENTAL SCIENCE AND TECHNOLOGY. Volume: 53 (2019) - 2019 • 5821
Nonbasal Slip Systems Enable a Strong and Ductile Hexagonal-Close-Packed High-Entropy Phase
Bu, Y. and Li, Z. and Liu, J. and Wang, H. and Raabe, D. and Yang, W.
PHYSICAL REVIEW LETTERS. Volume: 122 (2019) - 2019 • 5820
Advances in in situ nanomechanical testing
Minor, A.M. and Dehm, G.
MRS BULLETIN. Volume: 44 (2019) - 2019 • 5819
Quantification of uncertain macroscopic material properties resulting from variations of microstructure morphology based on statistically similar volume elements: application to dual-phase steel microstructures
Miska, N. and Balzani, D.
COMPUTATIONAL MECHANICS. Volume: 64 (2019) - 2019 • 5818
A correlation between char emissivity and temperature
Schiemann, M. and Gronarz, T. and Graeser, P. and Gorewoda, J. and Kneer, R. and Scherer, V.
FUEL. Volume: 256 (2019) - 2019 • 5817
Intelligent optimization and machine learning algorithms for structural anomaly detection using seismic signals
Trapp, M. and Bogoclu, C. and Nestorović, T. and Roos, D.
MECHANICAL SYSTEMS AND SIGNAL PROCESSING. Volume: 133 (2019) - 2019 • 5816
Sediment challenge to promising ultra-low fouling hydrophilic surfaces in the marine environment
Koc, J. and Simovich, T. and Schönemann, E. and Chilkoti, A. and Gardner, H. and Swain, G.W. and Hunsucker, K. and Laschewsky, A. and Rosenhahn, A.
BIOFOULING. Volume: 35 (2019) - 2019 • 5815
Influence of the Nature of Boron-Doped Diamond Anodes on the Dehydrogenative Phenol-Phenol Cross-Coupling
Gleede, B. and Yamamoto, T. and Nakahara, K. and Botz, A. and Graßl, T. and Neuber, R. and Matthée, T. and Einaga, Y. and Schuhmann, W. and Waldvogel, S.R.
CHEMELECTROCHEM. Volume: 6 (2019) - 2019 • 5814
Low-Fouling Thin Hydrogel Coatings Made of Photo-Cross-Linked Polyzwitterions
Koc, J. and Schönemann, E. and Amuthalingam, A. and Clarke, J. and Finlay, J.A. and Clare, A.S. and Laschewsky, A. and Rosenhahn, A.
LANGMUIR. Volume: 35 (2019) - 2019 • 5813
Creep properties of single crystal Ni-base superalloys (SX): A comparison between conventionally cast and additive manufactured CMSX-4 materials
Bürger, D. and Parsa, A.B. and Ramsperger, M. and Körner, C. and Eggeler, G.
MATERIALS SCIENCE AND ENGINEERING A. Volume: 762 (2019) - 2019 • 5812
Phonon Lifetimes throughout the Brillouin Zone at Elevated Temperatures from Experiment and Ab Initio
Glensk, A. and Grabowski, B. and Hickel, T. and Neugebauer, J. and Neuhaus, J. and Hradil, K. and Petry, W. and Leitner, M.
PHYSICAL REVIEW LETTERS. Volume: 123 (2019) - 2019 • 5811
Initiation and stagnation of room temperature grain coarsening in cyclically strained gold films
Glushko, O. and Dehm, G.
ACTA MATERIALIA. Volume: 169 (2019) - 2019 • 5810
How water flips at charged titanium dioxide: An SFG-study on the water-TiO 2 interface
Schlegel, S.J. and Hosseinpour, S. and Gebhard, M. and Devi, A. and Bonn, M. and Backus, E.H.G.
PHYSICAL CHEMISTRY CHEMICAL PHYSICS. Volume: 21 (2019) - 2019 • 5809
Cold spray deposition of Cr2AlC MAX phase for coatings and bond-coat layers
Go, T. and Sohn, Y.J. and Mauer, G. and Vaßen, R. and Gonzalez-Julian, J.
JOURNAL OF THE EUROPEAN CERAMIC SOCIETY. Volume: 39 (2019) - 2019 • 5808
Dissipated electrical power and electron density in an RF atmospheric pressure helium plasma jet
Golda, J. and Kogelheide, F. and Awakowicz, P. and Gathen, V.S.-V.D.
PLASMA SOURCES SCIENCE AND TECHNOLOGY. Volume: 28 (2019) - 2019 • 5807
Molecular cobalt corrole complex for the heterogeneous electrocatalytic reduction of carbon dioxide
Gonglach, S. and Paul, S. and Haas, M. and Pillwein, F. and Sreejith, S.S. and Barman, S. and De, R. and Müllegger, S. and Gerschel, P. and Apfel, U.-P. and Coskun, H. and Aljabour, A. and Stadler, P. and Schöfberger, W. and Roy, S.
NATURE COMMUNICATIONS. Volume: 10 (2019) - 2019 • 5806
Up-converting ALD/MLD thin films with Yb3+, Er3+ in amorphous organic framework
Tuomisto, M. and Giedraityte, Z. and Mai, L. and Devi, A. and Boiko, V. and Grzeszkiewicz, K. and Hreniak, D. and Karppinen, M. and Lastusaari, M.
JOURNAL OF LUMINESCENCE. Volume: 213 (2019) - 2019 • 5805
SiO2 microstructure evolution during plasma deposition analyzed via ellipsometric porosimetry
Buschhaus, R. and von Keudell, A.
PLASMA PROCESSES AND POLYMERS. Volume: 16 (2019) - 2019 • 5804
Optical excitation density dependence of spin dynamics in bulk cubic GaN
Buß, J.H. and Schupp, T. and As, D.J. and Hägele, D. and Rudolph, J.
JOURNAL OF APPLIED PHYSICS. Volume: 126 (2019) - 2019 • 5803
Electron spin dynamics in mesoscopic GaN nanowires
Buß, J.H. and Fernández-Garrido, S. and Brandt, O. and Hägele, D. and Rudolph, J.
APPLIED PHYSICS LETTERS. Volume: 114 (2019) - 2019 • 5802
Emittance of typical ash minerals: Influence of particle size and mixtures of carbonates and sulfates
Gorewoda, J. and Maas, P. and Scherer, V.
ENERGY PROCEDIA. Volume: 158 (2019) - 2019 • 5801
Enhancing Electrocatalytic Activity through Liquid-Phase Exfoliation of NiFe Layered Double Hydroxide Intercalated with Metal Phthalocyanines in the Presence of Graphene
Morales, D.M. and Barwe, S. and Vasile, E. and Andronescu, C. and Schuhmann, W.
CHEMPHYSCHEM. Volume: 20 (2019) - 2019 • 5800
A fully-relaxed variationally-consistent framework for inelastic micro-sphere models: Finite viscoelasticity
Govindjee, S. and Zoller, M.J. and Hackl, K.
JOURNAL OF THE MECHANICS AND PHYSICS OF SOLIDS. Volume: 127 (2019) - 2019 • 5799
Magnetic-field effects on the fragile antiferromagnetism in YbBiPt
Ueland, B.G. and Kreyssig, A. and Mun, E.D. and Lynn, J.W. and Harriger, L.W. and Pratt, D.K. and Prokeš, K. and Hüsges, Z. and Toft-Petersen, R. and Sauerbrei, S. and Saunders, S.M. and Furukawa, Y. and Bud'Ko, S.L. and McQueeney, R.J. and Canfield, P.C. and Goldman, A.I.
PHYSICAL REVIEW B. Volume: 99 (2019) - 2019 • 5798
Ferroelasticity, anelasticity and magnetoelastic relaxation in Co-doped iron pnictide: Ba(Fe0.957Co0.043)2As2
Carpenter, M.A. and Evans, D.M. and Schiemer, J.A. and Wolf, T. and Adelmann, P. and Böhmer, A.E. and Meingast, C. and Dutton, S.E. and Mukherjee, P. and Howard, C.J.
JOURNAL OF PHYSICS CONDENSED MATTER. Volume: 31 (2019) - 2019 • 5797
Geometrically nonlinear simulation of textile membrane structures based on orthotropic hyperelastic energy functions
Motevalli, M. and Uhlemann, J. and Stranghöner, N. and Balzani, D.
COMPOSITE STRUCTURES. Volume: 223 (2019) - 2019 • 5796
A generic method for equipping arbitrary rf discharge simulation frameworks with external lumped element circuits
Schmidt, F. and Trieschmann, J. and Gergs, T. and Mussenbrock, T.
JOURNAL OF APPLIED PHYSICS. Volume: 125 (2019) - 2019 • 5795
Suspended epoxy polymer inverted tapers for scalable fibre-coupled single-photon devices
Uǧurlu, A.D. and Thyrrestrup, H. and Uppu, R. and Ouellet-Plamondon, C. and Schott, R. and Wieck, A.D. and Ludwig, Ar. and Lodahl, P. and Midolo, L.
2019 CONFERENCE ON LASERS AND ELECTRO-OPTICS EUROPE AND EUROPEAN QUANTUM ELECTRONICS CONFERENCE, CLEO/EUROPE-EQEC 2019. Volume: (2019) - 2019 • 5794
Experimental Evidence of Confined Methane Hydrate in Hydrophilic and Hydrophobic Model Carbons
Casco, M.E. and Zhang, E. and Grätz, S. and Krause, S. and Bon, V. and Wallacher, D. and Grimm, N. and Többens, D.M. and Hauß, T. and Borchardt, L.
JOURNAL OF PHYSICAL CHEMISTRY C. Volume: 123 (2019) - 2019 • 5793
Ab initio vibrational free energies including anharmonicity for multicomponent alloys
Grabowski, B. and Ikeda, Y. and Srinivasan, P. and Körmann, F. and Freysoldt, C. and Duff, A.I. and Shapeev, A. and Neugebauer, J.
NPJ COMPUTATIONAL MATERIALS. Volume: 5 (2019) - 2019 • 5792
Handling of blackplate in metallographic preparation and heat treatment optimization via quenching dilatometry [Handhabung von Feinstblechen in der metallographischen Präparation und Wärmebehand-lungsoptimierung mittels Abschreckdilatometrie]
Schmidtseifer, N. and Weber, S.
PRAKTISCHE METALLOGRAPHIE/PRACTICAL METALLOGRAPHY. Volume: 56 (2019) - 2019 • 5791
Mechanochemical synthesis of N-doped porous carbon at room temperature
Casco, M.E. and Kirchhoff, S. and Leistenschneider, D. and Rauche, M. and Brunner, E. and Borchardt, L.
NANOSCALE. Volume: 11 (2019) - 2019 • 5790
Mechanochemical synthesis of hyper-crosslinked polymers: Influences on their pore structure and adsorption behaviour for organic vapors
Grätz, S. and Zink, S. and Kraffczyk, H. and Rose, M. and Borchardt, L.
BEILSTEIN JOURNAL OF ORGANIC CHEMISTRY. Volume: 15 (2019) - 2019 • 5789
Calibration of Atom Probe Tomography Reconstructions Through Correlation with Electron Micrographs
Mouton, I. and Katnagallu, S. and Makineni, S.K. and Cojocaru-Mirédin, O. and Schwarz, T. and Stephenson, L.T. and Raabe, D. and Gault, B.
MICROSCOPY AND MICROANALYSIS. Volume: 25 (2019) - 2019 • 5788
High Order Compact Multigrid Solver for Implicit Solvation Models
Castañeda Medina, A. and Schmid, R.
JOURNAL OF CHEMICAL THEORY AND COMPUTATION. Volume: 15 (2019) - 2019 • 5787
Quantification Challenges for Atom Probe Tomography of Hydrogen and Deuterium in Zircaloy-4
Mouton, I. and Breen, A.J. and Wang, S. and Chang, Y. and Szczepaniak, A. and Kontis, P. and Stephenson, L.T. and Raabe, D. and Herbig, M. and Britton, T.B. and Gault, B.
MICROSCOPY AND MICROANALYSIS. Volume: 25 (2019) - 2019 • 5786
A mechanism-based homogenization of a dislocation source model for bending
Schmitt, S. and Stricker, M. and Gumbsch, P. and Schulz, K.
ACTA MATERIALIA. Volume: 164 (2019) - 2019 • 5785
Characterization of a nitrogen gliding arc plasmatron using optical emission spectroscopy and high-speed camera
Gröger, S. and Ramakers, M. and Hamme, M. and Medrano, J.A. and Bibinov, N. and Gallucci, F. and Bogaerts, A. and Awakowicz, P.
JOURNAL OF PHYSICS D: APPLIED PHYSICS. Volume: 52 (2019) - 2019 • 5784
Aggregation control of Ru and Ir nanoparticles by tunable aryl alkyl imidazolium ionic liquids
Schmolke, L. and Lerch, S. and Bülow, M. and Siebels, M. and Schmitz, A. and Thomas, J. and Dehm, G. and Held, C. and Strassner, T. and Janiak, C.
NANOSCALE. Volume: 11 (2019) - 2019 • 5783
Major shear zone within the Greater Himalayan Sequence and sequential evolution of the metamorphic core in Sikkim, India
Chakraborty, S. and Mukul, M. and Mathew, G. and Pande, K.
TECTONOPHYSICS. Volume: 770 (2019) - 2019 • 5782
Collective modes in pumped unconventional superconductors with competing ground states
Müller, M.A. and Volkov, P.A. and Paul, I. and Eremin, I.M.
PHYSICAL REVIEW B. Volume: 100 (2019) - 2019 • 5781
New insights into the position and geometry of the main central thrust from Sikkim, Eastern Himalaya
Chakraborty, S. and Mukul, M.
JOURNAL OF GEOLOGY. Volume: 127 (2019) - 2019 • 5780
Order/disorder processes and electromechanical properties of monoclinic GdCa4O(BO3)3
Münchhalfen, M. and Schreuer, J. and Reuther, C. and Möckel, R. and Götze, J. and Mehner, E. and Stöcker, H. and Meyer, D.
ZEITSCHRIFT FUR KRISTALLOGRAPHIE - CRYSTALLINE MATERIALS. Volume: (2019) - 2019 • 5779
Ti and its alloys as examples of cryogenic focused ion beam milling of environmentally-sensitive materials
Chang, Y. and Lu, W. and Guénolé, J. and Stephenson, L.T. and Szczpaniak, A. and Kontis, P. and Ackerman, A.K. and Dear, F.F. and Mouton, I. and Zhong, X. and Zhang, S. and Dye, D. and Liebscher, C.H. and Ponge, D. and Korte-Kerzel, S. and Raabe, D. and Gault, B.
NATURE COMMUNICATIONS. Volume: 10 (2019) - 2019 • 5778
Nanosecond plasmas in water: Ignition, cavitation and plasma parameters
Grosse, K. and Held, J. and Kai, M. and Von Keudell, A.
PLASMA SOURCES SCIENCE AND TECHNOLOGY. Volume: 28 (2019) - 2019 • 5777
Effect of temperature and texture on Hall–Petch strengthening by grain and annealing twin boundaries in the MnFeNi medium-entropy alloy
Schneider, M. and Werner, F. and Langenkämper, D. and Reinhart, C. and Laplanche, G.
METALS. Volume: 9 (2019) - 2019 • 5776
Quantification of solute deuterium in titanium deuteride by atom probe tomography with both laser pulsing and high-voltage pulsing: Influence of the surface electric field
Chang, Y.H. and Mouton, I. and Stephenson, L. and Ashton, M. and Zhang, G.K. and Szczpaniak, A. and Lu, W.J. and Ponge, D. and Raabe, D. and Gault, B.
NEW JOURNAL OF PHYSICS. Volume: 21 (2019) - 2019 • 5775
Influence of composition and precipitation evolution on damage at grain boundaries in a crept polycrystalline Ni-based superalloy
Kontis, P. and Kostka, A. and Raabe, D. and Gault, B.
ACTA MATERIALIA. Volume: 166 (2019) - 2019 • 5774
Benchmark dataset of the effect of grain size on strength in the single-phase FCC CrCoNi medium entropy alloy
Schneider, M. and George, E.P. and Manescau, T.J. and Záležák, T. and Hunfeld, J. and Dlouhý, A. and Eggeler, G. and Laplanche, G.
DATA IN BRIEF. Volume: 27 (2019) - 2019 • 5773
Atomic-scale grain boundary engineering to overcome hot-cracking in additively-manufactured superalloys
Kontis, P. and Chauvet, E. and Peng, Z. and He, J. and da Silva, A.K. and Raabe, D. and Tassin, C. and Blandin, J.-J. and Abed, S. and Dendievel, R. and Gault, B. and Martin, G.
ACTA MATERIALIA. Volume: 177 (2019) - 2019 • 5772
Microstructure and phase evolution of atmospheric plasma sprayed Mn-Co-Fe oxide protection layers for solid oxide fuel cells
Grünwald, N. and Sohn, Y.J. and Yin, X. and Menzler, N.H. and Guillon, O. and Vaßen, R.
JOURNAL OF THE EUROPEAN CERAMIC SOCIETY. Volume: 39 (2019) - 2019 • 5771
Retrofitting metal-organic frameworks
Schneider, C. and Bodesheim, D. and Keupp, J. and Schmid, R. and Kieslich, G.
NATURE COMMUNICATIONS. Volume: 10 (2019) - 2019 • 5770
Nitrogen-Doped Biomass-Derived Carbon Formed by Mechanochemical Synthesis for Lithium–Sulfur Batteries
Schneidermann, C. and Kensy, C. and Otto, P. and Oswald, S. and Giebeler, L. and Leistenschneider, D. and Grätz, S. and Dörfler, S. and Kaskel, S. and Borchardt, L.
CHEMSUSCHEM. Volume: 12 (2019) - 2019 • 5769
Upcycling of polyurethane waste by mechanochemistry: Synthesis of N-doped porous carbon materials for supercapacitor applications
Schneidermann, C. and Otto, P. and Leistenschneider, D. and Grätz, S. and Eßbach, C. and Borchardt, L.
BEILSTEIN JOURNAL OF NANOTECHNOLOGY. Volume: 10 (2019) - 2019 • 5768
Ab initio thermodynamics of liquid and solid water
Cheng, B. and Engel, E.A. and Behler, J. and Dellago, C. and Ceriotti, M.
PROCEEDINGS OF THE NATIONAL ACADEMY OF SCIENCES OF THE UNITED STATES OF AMERICA. Volume: 116 (2019) - 2019 • 5767
Ab initio based method to study structural phase transitions in dynamically unstable crystals, with new insights on the β to ω transformation in titanium
Korbmacher, D. and Glensk, A. and Duff, A.I. and Finnis, M.W. and Grabowski, B. and Neugebauer, J.
PHYSICAL REVIEW B. Volume: 100 (2019) - 2019 • 5766
High spatial resolution electrochemical biosensing using reflected light microscopy
Munteanu, R.-E. and Ye, R. and Polonschii, C. and Ruff, A. and Gheorghiu, M. and Gheorghiu, E. and Boukherroub, R. and Schuhmann, W. and Melinte, S. and Gáspár, S.
SCIENTIFIC REPORTS. Volume: 9 (2019) - 2019 • 5765
Transducer-matched multipulse excitation for signal-to-noise ratio improvement in diode laser-based photoacoustic systems
Cherkashin, M.N. and Brenner, C. and Hofmann, M.R.
JOURNAL OF BIOMEDICAL OPTICS. Volume: 24 (2019) - 2019 • 5764
Control of electron dynamics, radical and metastable species generation in atmospheric pressure RF plasma jets by Voltage Waveform Tailoring
Korolov, I. and Donkó, Z. and Hübner, G. and Bischoff, L. and Hartmann, P. and Gans, T. and Liu, Y. and Mussenbrock, T. and Schulze, J.
PLASMA SOURCES SCIENCE AND TECHNOLOGY. Volume: 28 (2019) - 2019 • 5763
Temperature and bias anomalies in the photoluminescence of InAs quantum dots coupled to a Fermi reservoir
Korsch, A.R. and Nguyen, G.N. and Schmidt, M. and Ebler, C. and Valentin, S.R. and Lochner, P. and Rothfuchs, C. and Wieck, A.D. and Ludwig, Ar.
PHYSICAL REVIEW B. Volume: 99 (2019) - 2019 • 5762
A gated quantum dot strongly coupled to an optical microcavity
Najer, D. and Söllner, I. and Sekatski, P. and Dolique, V. and Löbl, M.C. and Riedel, D. and Schott, R. and Starosielec, S. and Valentin, S.R. and Wieck, A.D. and Sangouard, N. and Ludwig, Ar. and Warburton, R.J.
NATURE. Volume: 575 (2019) - 2019 • 5761
Oxygen-mediated deformation and grain refinement in Cu-Fe nanocrystalline alloys
Guo, J. and Duarte, M.J. and Zhang, Y. and Bachmaier, A. and Gammer, C. and Dehm, G. and Pippan, R. and Zhang, Z.
ACTA MATERIALIA. Volume: 166 (2019) - 2019 • 5760
Quantum non-demolition measurement of an electron spin qubit
Nakajima, T. and Noiri, A. and Yoneda, J. and Delbecq, M.R. and Stano, P. and Otsuka, T. and Takeda, K. and Amaha, S. and Allison, G. and Kawasaki, K. and Ludwig, Ar. and Wieck, A.D. and Loss, D. and Tarucha, S.
NATURE NANOTECHNOLOGY. Volume: 14 (2019) - 2019 • 5759
Contrast enhancement for topographic imaging in confocal laser scanning microscopy
Schnitzler, L. and Finkeldey, M. and Hofmann, M.R. and Gerhardt, N.C.
APPLIED SCIENCES (SWITZERLAND). Volume: 9 (2019) - 2019 • 5758
Investigation on Optical Methods for Multi Scale Electromagnetic Simulations
Vogt, S. and Jebramcik, J. and Garten, O. and Barowski, J. and Rolfes, I.
GEMIC 2019 - 2019 GERMAN MICROWAVE CONFERENCE. Volume: (2019) - 2019 • 5757
Regulating the size and spatial distribution of Pd nanoparticles supported by the defect engineered metal-organic framework HKUST-1 and applied in the aerobic oxidation of cinnamyl alcohol
Guo, P. and Fu, Q. and Yildiz, C. and Chen, Y.-T. and Ollegott, K. and Froese, C. and Kleist, W. and Fischer, R.A. and Wang, Y. and Muhler, M. and Peng, B.
CATALYSIS SCIENCE AND TECHNOLOGY. Volume: 9 (2019) - 2019 • 5756
Surface modification by polyzwitterions of the sulfabetaine-type, and their resistance to biofouling
Schönemann, E. and Laschewsky, A. and Wischerhoff, E. and Koc, J. and Rosenhahn, A.
POLYMERS. Volume: 11 (2019) - 2019 • 5755
Asymptotic Simulation Methods as Forward Models in Multilayer Material Characterization Applications
Vogt, S. and Garten, O. and Jebramcik, J. and Barowski, J. and Rolfes, I.
IMWS-AMP 2019 - 2019 IEEE MTT-S INTERNATIONAL MICROWAVE WORKSHOP SERIES ON ADVANCED MATERIALS AND PROCESSES FOR RF AND THZ APPLICATIONS. Volume: (2019) - 2019 • 5754
Shape-preserving machining produces gradient nanolaminate medium entropy alloys with high strain hardening capability
Guo, W. and Pei, Z. and Sang, X. and Poplawsky, J.D. and Bruschi, S. and Qu, J. and Raabe, D. and Bei, H.
ACTA MATERIALIA. Volume: 170 (2019) - 2019 • 5753
Direct Mechanocatalysis: Palladium as Milling Media and Catalyst in the Mechanochemical Suzuki Polymerization
Vogt, C.G. and Grätz, S. and Lukin, S. and Halasz, I. and Etter, M. and Evans, J.D. and Borchardt, L.
ANGEWANDTE CHEMIE - INTERNATIONAL EDITION. Volume: 58 (2019) - 2019 • 5752
Slow Cooling at Higher Temperatures Recorded within High- P Mafic Granulites from the Southern Granulite Terrain, India: Implications for the Presence and Style of Plate Tectonics near the Archean-Proterozoic Boundary
Chowdhury, P. and Chakraborty, S.
JOURNAL OF PETROLOGY. Volume: 60 (2019) - 2019 • 5751
A Combinatorial Approach for Optimization of Oxygen Evolution Catalyst Loading on Mo-doped BiVO4 Photoanodes
Gutkowski, R. and Masa, J. and Schuhmann, W.
ELECTROANALYSIS. Volume: 31 (2019) - 2019 • 5750
Additive manufacturing of soft magnetic permalloy from Fe and Ni powders: Control of magnetic anisotropy
Schönrath, H. and Spasova, M. and Kilian, S.O. and Meckenstock, R. and Witt, G. and Sehrt, J.T. and Farle, M.
JOURNAL OF MAGNETISM AND MAGNETIC MATERIALS. Volume: 478 (2019) - 2019 • 5749
Goodness-of-fit testing the error distribution in multivariate indirect regression
Chown, J. and Bissantz, N. and Dette, H.
ELECTRONIC JOURNAL OF STATISTICS. Volume: 13 (2019) - 2019 • 5748
Continuous wave thz source based on an electrically tunable monolithic two-color semiconductor diode laser
Gwaro, J.O. and Brenner, C. and Sumpf, B. and Klehr, A. and Fricke, J. and Hofmann, M.R.
2019 CONFERENCE ON LASERS AND ELECTRO-OPTICS EUROPE AND EUROPEAN QUANTUM ELECTRONICS CONFERENCE, CLEO/EUROPE-EQEC 2019. Volume: (2019) - 2019 • 5747
Impact of lattice relaxations on phase transitions in a high-entropy alloy studied by machine-learning potentials
Kostiuchenko, T. and Körmann, F. and Neugebauer, J. and Shapeev, A.
NPJ COMPUTATIONAL MATERIALS. Volume: 5 (2019) - 2019 • 5746
Milling Down to Nanometers: A General Process for the Direct Dry Synthesis of Supported Metal Catalysts
Schreyer, H. and Eckert, R. and Immohr, S. and de Bellis, J. and Felderhoff, M. and Schüth, F.
ANGEWANDTE CHEMIE - INTERNATIONAL EDITION. Volume: 58 (2019) - 2019 • 5745
BioSAxS measurements reveal that two antimicrobial peptides induce similar molecular changes in Gram-negative and Gram-positive bacteria
Von Gundlach, A. and Ashby, M.P. and Gani, J. and Lopez-Perez, P.M. and Cookson, A.R. and Huws, S.A. and Rumancev, C. and Garamus, V.M. and Mikut, R. and Rosenhahn, A. and Hilpert, K.
FRONTIERS IN PHARMACOLOGY. Volume: 10 (2019) - 2019 • 5744
On the role of cobalt carbidization in higher alcohol synthesis over hydrotalcite-based Co-Cu catalysts
Nebel, J. and Schmidt, S. and Pan, Q. and Lotz, K. and Kaluza, S. and Muhler, M.
CHINESE JOURNAL OF CATALYSIS. Volume: 40 (2019) - 2019 • 5743
Superior cyclic life of thermal barrier coatings with advanced bond coats on single-crystal superalloys
Vorkötter, C. and Mack, D.E. and Guillon, O. and Vaßen, R.
SURFACE AND COATINGS TECHNOLOGY. Volume: 361 (2019) - 2019 • 5742
Oxide Dispersion Strengthened Bond Coats with Higher Alumina Content: Oxidation Resistance and Influence on Thermal Barrier Coating Lifetime
Vorkötter, C. and Hagen, S.P. and Pintsuk, G. and Mack, D.E. and Virtanen, S. and Guillon, O. and Vaßen, R.
OXIDATION OF METALS. Volume: 92 (2019) - 2019 • 5741
Density, distribution and nature of planar faults in silver antimony telluride for thermoelectric applications
Abdellaoui, L. and Zhang, S. and Zaefferer, S. and Bueno-Villoro, R. and Baranovskiy, A. and Cojocaru-Mirédin, O. and Yu, Y. and Amouyal, Y. and Raabe, D. and Snyder, G.J. and Scheu, C.
ACTA MATERIALIA. Volume: 178 (2019) - 2019 • 5740
Influence of bulk energy and triple junction mobility on interface kinetics - A tool for interpretation of experiments
Hackl, K. and Khan, A.U. and Fischer, F.D. and Svoboda, J.
ACTA MATERIALIA. Volume: 174 (2019) - 2019 • 5739
The basics of electronic structure theory for periodic systems
Kratzer, P. and Neugebauer, J.
FRONTIERS IN CHEMISTRY. Volume: 7 (2019) - 2019 • 5738
Robust segmental lining design combining steel fiber reinforced concrete and traditional reinforcement
Neu, G.E. and Gall, V.E. and Meschke, G.
TUNNELS AND UNDERGROUND CITIES: ENGINEERING AND INNOVATION MEET ARCHAEOLOGY, ARCHITECTURE AND ART- PROCEEDINGS OF THE WTC 2019 ITA-AITES WORLD TUNNEL CONGRESS. Volume: (2019) - 2019 • 5737
Towards Mechanistic Understanding of Liquid-Phase Cinnamyl Alcohol Oxidation with tert-Butyl Hydroperoxide over Noble-Metal-Free LaCo1–xFexO3 Perovskites
Waffel, D. and Alkan, B. and Fu, Q. and Chen, Y.-T. and Schmidt, S. and Schulz, C. and Wiggers, H. and Muhler, M. and Peng, B.
CHEMPLUSCHEM. Volume: 84 (2019) - 2019 • 5736
Making more from methane
Schüth, F.
SCIENCE. Volume: 363 (2019) - 2019 • 5735
A 3D printed elliptical mirror for material characterization using FMCW transceivers
Wagner, J. and Barowski, J. and Rolfes, I.
ASIA-PACIFIC MICROWAVE CONFERENCE PROCEEDINGS, APMC. Volume: 2018-November (2019) - 2019 • 5734
Wilhelm Keim (1934-2018)
Schüth, F. and Wasserscheid, P.
ANGEWANDTE CHEMIE (INTERNATIONAL ED. IN ENGLISH). Volume: 58 (2019) - 2019 • 5733
Synthetic Aperture Radar Imaging Using MIMO Frequency Modulated Continuous Wave Sensors
Wagner, J. and Dahl, C. and Rolfes, I. and Barowski, J.
IMWS-AMP 2019 - 2019 IEEE MTT-S INTERNATIONAL MICROWAVE WORKSHOP SERIES ON ADVANCED MATERIALS AND PROCESSES FOR RF AND THZ APPLICATIONS. Volume: (2019) - 2019 • 5732
Modeling of structures with polymorphic uncertainties at different length scales
Kremer, K. and Edler, P. and Miska, N. and Leichsenring, F. and Balzani, D. and Freitag, S. and Graf, W. and Kaliske, M. and Meschke, G.
GAMM MITTEILUNGEN. Volume: 42 (2019) - 2019 • 5731
Synthetic aperture radar surveillance of conveyed materials at 144 GHz
Wagner, J. and Rolfes, I. and Barowski, J.
ASIA-PACIFIC MICROWAVE CONFERENCE PROCEEDINGS, APMC. Volume: 2019-December (2019) - 2019 • 5730
Three-dimensional particle localization with common-path digital holographic microscopy
Neutsch, K. and GöÂ-Ring, L. and Tranelis, M.J. and Hofmann, M.R. and Gerhardt, N.C.
PROCEEDINGS OF SPIE - THE INTERNATIONAL SOCIETY FOR OPTICAL ENGINEERING. Volume: 10944 (2019) - 2019 • 5729
Development of a test setup for the simulation of the annular gap grouting on a semi technical scale
Schulte-Schrepping, C. and Breitenbücher, R.
TUNNELS AND UNDERGROUND CITIES: ENGINEERING AND INNOVATION MEET ARCHAEOLOGY, ARCHITECTURE AND ART- PROCEEDINGS OF THE WTC 2019 ITA-AITES WORLD TUNNEL CONGRESS. Volume: (2019) - 2019 • 5728
Thermo-mechanical properties of mullite ceramics: New data
Krenzel, T.F. and Schreuer, J. and Laubner, D. and Cichocki, M. and Schneider, H.
JOURNAL OF THE AMERICAN CERAMIC SOCIETY. Volume: 102 (2019) - 2019 • 5727
Two-component grouts with alkali-activated binders
Schulte-Schrepping, C. and Breitenbücher, R.
TUNNELS AND UNDERGROUND CITIES: ENGINEERING AND INNOVATION MEET ARCHAEOLOGY, ARCHITECTURE AND ART- PROCEEDINGS OF THE WTC 2019 ITA-AITES WORLD TUNNEL CONGRESS. Volume: (2019) - 2019 • 5726
The molecular chaperone Hsp33 is activated by atmospheric-pressure plasma protecting proteins from aggregation
Krewing, M. and Stepanek, J.J. and Cremers, C. and Lackmann, J.-W. and Schubert, B. and Müller, A. and Awakowicz, P. and Leichert, L.I.O. and Jakob, U. and Bandow, J.E.
JOURNAL OF THE ROYAL SOCIETY INTERFACE. Volume: 16 (2019) - 2019 • 5725
Validation of etching model of polypropylene layers exposed to argon plasmas
Corbella, C. and Pranda, A. and Portal, S. and de los Arcos, T. and Grundmeier, G. and Oehrlein, G.S. and von Keudell, A.
PLASMA PROCESSES AND POLYMERS. Volume: 16 (2019) - 2019 • 5724
Synthetic approaches to artificial photosynthesis: General discussion
Aitchison, C.M. and Andrei, V. and Antón-García, D. and Apfel, U.-P. and Badiani, V. and Beller, M. and Bocarsly, A.B. and Bonnet, S. and Brueggeller, P. and Caputo, C.A. and Cassiola, F. and Clausing, S.T. and Cooper, A.I. and Creissen, C.E. and De La Peña O'Shea, V.A. and Domcke, W. and Durrant, J.R. and Grätzel, M. and Hammarström, L. and Hankin, A. and Hatzell, M.C. and Karadas, F. and König, B. and Kuehnel, M.F. and Lamaison, S. and Lin, C.-Y. and Maneiro, M. and Minteer, S.D. and Paris, A.R. and Pastor, E. and Pornrungroj, C. and Reek, J.N.H. and Reisner, E. and Roy, S. and Sahm, C. and Shankar, R. and Shaw, W.J. and Shylin, S.I. and Smith, W.A. and Sokol, K. and Soo, H.S. and Sprick, R.S. and Viertl, W. and Vogel, A. and Wagner, A. and Wakerley, D. and Wang, Q. and Wielend, D. and Zwijnenburg, M.A.
FARADAY DISCUSSIONS. Volume: 215 (2019) - 2019 • 5723
On crystal mosaicity in single crystal Ni-based superalloys
Hallensleben, P. and Scholz, F. and Thome, P. and Schaar, H. and Steinbach, I. and Eggeler, G. and Frenzel, J.
CRYSTALS. Volume: 9 (2019) - 2019 • 5722
Impact of Heating–Cooling Rates on the Functional Properties of Ti–20Ta–5Al High-Temperature Shape Memory Alloys
Krooß, P. and Lauhoff, C. and Langenkämper, D. and Paulsen, A. and Reul, A. and Degener, S. and Aminforoughi, B. and Frenzel, J. and Somsen, C. and Schmahl, W.W. and Eggeler, G. and Maier, H.J. and Niendorf, T.
SHAPE MEMORY AND SUPERELASTICITY. Volume: 5 (2019) - 2019 • 5721
Computationally Efficient Simulation in Urban Mechanized Tunneling Based on Multilevel BIM Models
Ninić, J. and Bui, H.-G. and Koch, C. and Meschke, G.
JOURNAL OF COMPUTING IN CIVIL ENGINEERING. Volume: 33 (2019) - 2019 • 5720
Luminescent Nd 2 S 3 thin films: A new chemical vapour deposition route towards rare-earth sulphides
Cwik, S. and Beer, S.M.J. and Schmidt, M. and Gerhardt, N.C. and De Los Arcos, T. and Rogalla, D. and Weßing, J. and Giner, I. and Hofmann, M. and Grundmeier, G. and Wieck, A.D. and Devi, A.
DALTON TRANSACTIONS. Volume: 48 (2019) - 2019 • 5719
Variable chemical decoration of extended defects in Cu-poor C u2ZnSnS e4 thin films
Schwarz, T. and Redinger, A. and Siebentritt, S. and Peng, Z. and Gault, B. and Raabe, D. and Choi, P.-P.
PHYSICAL REVIEW MATERIALS. Volume: 3 (2019) - 2019 • 5718
Oxygen Evolution Electrocatalysis of a Single MOF-Derived Composite Nanoparticle on the Tip of a Nanoelectrode
Aiyappa, H.B. and Wilde, P. and Quast, T. and Masa, J. and Andronescu, C. and Chen, Y.-T. and Muhler, M. and Fischer, R.A. and Schuhmann, W.
ANGEWANDTE CHEMIE - INTERNATIONAL EDITION. Volume: 58 (2019) - 2019 • 5717
Operando Thin-Layer ATR-FTIR Spectroelectrochemical Radial Flow Cell with Tilt Correction and Borehole Electrode
Cychy, S. and Hiltrop, D. and Andronescu, C. and Muhler, M. and Schuhmann, W.
ANALYTICAL CHEMISTRY. Volume: (2019) - 2019 • 5716
BOPfox program for tight-binding and analytic bond-order potential calculations
Hammerschmidt, T. and Seiser, B. and Ford, M.E. and Ladines, A.N. and Schreiber, S. and Wang, N. and Jenke, J. and Lysogorskiy, Y. and Teijeiro, C. and Mrovec, M. and Cak, M. and Margine, E.R. and Pettifor, D.G. and Drautz, R.
COMPUTER PHYSICS COMMUNICATIONS. Volume: 235 (2019) - 2019 • 5715
MOFs for Electrocatalysis: From Serendipity to Design Strategies
Aiyappa, H.B. and Masa, J. and Andronescu, C. and Muhler, M. and Fischer, R.A. and Schuhmann, W.
SMALL METHODS. Volume: 3 (2019) - 2019 • 5714
Interaction of Skyrmions and Pearl Vortices in Superconductor-Chiral Ferromagnet Heterostructures
Dahir, S.M. and Volkov, A.F. and Eremin, I.M.
PHYSICAL REVIEW LETTERS. Volume: 122 (2019) - 2019 • 5713
Production and investigation of the wear behavior of inductively melted hardpaint coatings [Herstellung und Untersuchung des Verschleißverhaltens von induktiv eingeschmolzenen Hardpaint-Schutzschichten]
Schwarz, P. and Deuerler, F. and Weber, S.
MATERIALWISSENSCHAFT UND WERKSTOFFTECHNIK. Volume: 50 (2019) - 2019 • 5712
Structural and Functional Properties of the Thin Film System Ti-Ni-Si
Wambach, M. and Ziolkowski, P. and Müller, E. and Ludwig, Al.
ACS COMBINATORIAL SCIENCE. Volume: 21 (2019) - 2019 • 5711
Phase-dependent spin polarization of Cooper pairs in magnetic Josephson junctions
Dahir, S.M. and Volkov, A.F. and Eremin, I.M.
PHYSICAL REVIEW B. Volume: 100 (2019) - 2019 • 5710
Quantum Vortex Core and Missing Pseudogap in the Multiband BCS-BEC Crossover Superconductor FeSe
Hanaguri, T. and Kasahara, S. and Böker, J. and Eremin, I. and Shibauchi, T. and Matsuda, Y.
PHYSICAL REVIEW LETTERS. Volume: 122 (2019) - 2019 • 5709
Voltage waveform tailoring in radio frequency plasmas for surface charge neutralization inside etch trenches
Krüger, F. and Wilczek, S. and Mussenbrock, T. and Schulze, J.
PLASMA SOURCES SCIENCE AND TECHNOLOGY. Volume: 28 (2019) - 2019 • 5708
Electrical and Structural Properties of the Partial Ternary Thin-Film System Ni-Si-B
Wambach, M. and Nguyen, N. and Hamann, S. and Nishio, M. and Yagyu, S. and Chikyow, T. and Ludwig, Al.
ACS COMBINATORIAL SCIENCE. Volume: 21 (2019) - 2019 • 5707
Assessment of coal pyrolysis kinetics for Barracuda or Ansys Fluent
Krusch, S. and Scherer, V. and Solimene, R. and Senneca, O.
ENERGY PROCEDIA. Volume: 158 (2019) - 2019 • 5706
Blending Real World Gasoline with Biofuel in a Direct Conversion Process
Nürenberg, E. and Schulze, P. and Kohler, F. and Zubel, M. and Pischinger, S. and Schüth, F.
ACS SUSTAINABLE CHEMISTRY AND ENGINEERING. Volume: 7 (2019) - 2019 • 5705
Effects of femtosecond laser shock peening in distilled water on the surface characterizations of NiTi shape memory alloy
Wang, H. and Pöhl, F. and Yan, K. and Decker, P. and Gurevich, E.L. and Ostendorf, A.
APPLIED SURFACE SCIENCE. Volume: 471 (2019) - 2019 • 5704
Magnetic control of nonlinear electron resonance heating in a capacitively coupled radio frequency discharge
Oberberg, M. and Engel, D. and Berger, B. and Wölfel, C. and Eremin, D. and Lunze, J. and Brinkmann, R.P. and Awakowicz, P. and Schulze, J.
PLASMA SOURCES SCIENCE AND TECHNOLOGY. Volume: 28 (2019) - 2019 • 5703
Thermodynamic assessment of the Co-Ta system
Wang, P. and Koßmann, J. and Kattner, U.R. and Palumbo, M. and Hammerschmidt, T. and Olson, G.B.
CALPHAD: COMPUTER COUPLING OF PHASE DIAGRAMS AND THERMOCHEMISTRY. Volume: 64 (2019) - 2019 • 5702
Heterodimetallic Ferrocenyl Dithiophosphonate Complexes of Nickel(II), Zinc(II) and Cadmium(II) as Sensitizers for TiO2-Based Dye-Sensitized Solar Cells
Ajayi, T.J. and Ollengo, M. and le Roux, L. and Pillay, M.N. and Staples, R.J. and Biros, S.M. and Wenderich, K. and Mei, B. and van Zyl, W.E.
CHEMISTRYSELECT. Volume: 4 (2019) - 2019 • 5701
Carbon partitioning and microstructure evolution during tempering of an Fe-Ni-C steel
Harding, I. and Mouton, I. and Gault, B. and Raabe, D. and Kumar, K.S.
SCRIPTA MATERIALIA. Volume: 172 (2019) - 2019 • 5700
Application of atom probe tomography to complex microstructures of laser additively manufactured samples
Kürnsteiner, P. and Hariharan, A. and Jung, H.Y. and Peter, N. and Wilms, M.B. and Weisheit, A. and Barriobero-Vila, P. and Gault, B. and Raabe, D. and Jägle, E.A.
MICROSCOPY AND MICROANALYSIS. Volume: 25 (2019) - 2019 • 5699
Catalytic Reactivation of Industrial Oxygen Depolarized Cathodes by in situ Generation of Atomic Hydrogen
Öhl, D. and Franzen, D. and Paulisch, M. and Dieckhöfer, S. and Barwe, S. and Andronescu, C. and Manke, I. and Turek, T. and Schuhmann, W.
CHEMSUSCHEM. Volume: 12 (2019) - 2019 • 5698
Crystal plasticity finite element modeling and simulation of diamond cutting of polycrystalline copper
Wang, Z. and Zhang, J. and Xu, Z. and Zhang, J. and Hassan, H.U. and Li, G. and Zhang, H. and Hartmaier, A. and Fang, F. and Yan, Y. and Sun, T.
JOURNAL OF MANUFACTURING PROCESSES. Volume: 38 (2019) - 2019 • 5697
Misorientation-dependent solute enrichment at interfaces and its contribution to defect formation mechanisms during laser additive manufacturing of superalloys
Hariharan, A. and Lu, L. and Risse, J. and Kostka, A. and Gault, B. and Jägle, E.A. and Raabe, D.
PHYSICAL REVIEW MATERIALS. Volume: 3 (2019) - 2019 • 5696
Microstructure – Property correlations for additively manufactured NiTi based shape memory alloys
Kumar S, S. and Marandi, L. and Balla, V.K. and Bysakh, S. and Piorunek, D. and Eggeler, G. and Das, M. and Sen, I.
MATERIALIA. Volume: 8 (2019) - 2019 • 5695
Accelerating spin-space sampling by auxiliary spin dynamics and temperature-dependent spin-cluster expansion
Wang, N. and Hammerschmidt, T. and Rogal, J. and Drautz, R.
PHYSICAL REVIEW B. Volume: 99 (2019) - 2019 • 5694
Photon Noise Suppression by a Built-in Feedback Loop
Al-Ashouri, A. and Kurzmann, A. and Merkel, B. and Ludwig, Ar. and Wieck, A.D. and Lorke, A. and Geller, M.
NANO LETTERS. Volume: 19 (2019) - 2019 • 5693
Micromechanical modelling of coupled crystal plasticity and hydrogen diffusion
Hassan, H.U. and Govind, K. and Hartmaier, A.
PHILOSOPHICAL MAGAZINE. Volume: 99 (2019) - 2019 • 5692
Effect of Nb on improving the impact toughness of Mo-containing low-alloyed steels
Wang, H.C. and Somsen, C. and Li, Y.J. and Fries, S.G. and Detemple, E. and Eggeler, G.
JOURNAL OF MATERIALS SCIENCE. Volume: 54 (2019) - 2019 • 5691
Probing the Degree of Heterogeneity within a Shear Band of a Model Glass
Hassani, M. and Lagogianni, A.E. and Varnik, F.
PHYSICAL REVIEW LETTERS. Volume: 123 (2019) - 2019 • 5690
Crystal plasticity finite element simulation and experiment investigation of nanoscratching of single crystalline copper
Wang, Z. and Zhang, H. and Li, Z. and Li, G. and Zhang, J. and Zhang, J. and Hassan, H.U. and Yan, Y. and Hartmaier, A. and Sun, T.
WEAR. Volume: 430-431 (2019) - 2019 • 5689
On the mechanism of extraordinary strain hardening in an interstitial high-entropy alloy under cryogenic conditions
Wang, Z. and Lu, W. and Raabe, D. and Li, Z.
JOURNAL OF ALLOYS AND COMPOUNDS. Volume: (2019) - 2019 • 5688
The 2019 materials by design roadmap
Alberi, K. and Nardelli, M.B. and Zakutayev, A. and Mitas, L. and Curtarolo, S. and Jain, A. and Fornari, M. and Marzari, N. and Takeuchi, I. and Green, M.L. and Kanatzidis, M. and Toney, M.F. and Butenko, S. and Meredig, B. and Lany, S. and Kattner, U. and Davydov, A. and Toberer, E.S. and Stevanovic, V. and Walsh, A. and Park, N.-G. and Aspuru-Guzik, A. and Tabor, D.P. and Nelson, J. and Murphy, J. and Setlur, A. and Gregoire, J. and Li, H. and Xiao, R. and Ludwig, Al. and Martin, L.W. and Rappe, A.M. and Wei, S.-H. and Perkins, J.
JOURNAL OF PHYSICS D: APPLIED PHYSICS. Volume: 52 (2019) - 2019 • 5687
Emulation of Spatially Distributed Plasma Density Profiles in 3D Electromagnetic Field Simulations
Hattenhorst, B. and Pohle, D. and Schulz, C. and Rolfes, I. and Musch, T.
IMWS-AMP 2019 - 2019 IEEE MTT-S INTERNATIONAL MICROWAVE WORKSHOP SERIES ON ADVANCED MATERIALS AND PROCESSES FOR RF AND THZ APPLICATIONS. Volume: (2019) - 2019 • 5686
Comparative study of different anisotropy and potential formulations of phase-field models for dendritic solidification
Kundin, J. and Steinbach, I.
COMPUTATIONAL MATERIALS SCIENCE. Volume: 170 (2019) - 2019 • 5685
Determination of NO densities in a surface dielectric barrier discharge using optical emission spectroscopy
Offerhaus, B. and Kogelheide, F. and Jalat, D. and Bibinov, N. and Schulze, J. and Stapelmann, K. and Awakowicz, P.
JOURNAL OF APPLIED PHYSICS. Volume: 126 (2019) - 2019 • 5684
How [FeFe]-Hydrogenase Facilitates Bidirectional Proton Transfer
Senger, M. and Eichmann, V. and Laun, K. and Duan, J. and Wittkamp, F. and Knör, G. and Apfel, U.-P. and Happe, T. and Winkler, M. and Heberle, J. and Stripp, S.T.
JOURNAL OF THE AMERICAN CHEMICAL SOCIETY. Volume: 141 (2019) - 2019 • 5683
Synthesis of Ti3SiC2 MAX phase powder by a molten salt shielded synthesis (MS3) method in air
Dash, A. and Sohn, Y.J. and Vaßen, R. and Guillon, O. and Gonzalez-Julian, J.
JOURNAL OF THE EUROPEAN CERAMIC SOCIETY. Volume: 39 (2019) - 2019 • 5682
The role of lattice defects, element partitioning and intrinsic heat effects on the microstructure in selective laser melted Ti-6Al-4V
Haubrich, J. and Gussone, J. and Barriobero-Vila, P. and Kürnsteiner, P. and Jägle, E.A. and Raabe, D. and Schell, N. and Requena, G.
ACTA MATERIALIA. Volume: 167 (2019) - 2019 • 5681
Assessment of combustion rates of coal chars for oxy-combustion applications
Senneca, O. and Vorobiev, N. and Wütscher, A. and Cerciello, F. and Heuer, S. and Wedler, C. and Span, R. and Schiemann, M. and Muhler, M. and Scherer, V.
FUEL. Volume: 238 (2019) - 2019 • 5680
Combined phase-field crystal plasticity simulation of P- and N-type rafting in Co-based superalloys
Wang, C. and Ali, M.A. and Gao, S. and Goerler, J.V. and Steinbach, I.
ACTA MATERIALIA. Volume: 175 (2019) - 2019 • 5679
Molten salt shielded synthesis of oxidation prone materials in air
Dash, A. and Vaßen, R. and Guillon, O. and Gonzalez-Julian, J.
NATURE MATERIALS. Volume: 18 (2019) - 2019 • 5678
Fragmentation of pulverized coal in a laminar drop tube reactor: Experiments and model
Senneca, O. and Heuer, S. and Bareschino, P. and Urciuolo, M. and Pepe, F. and Schiemann, M. and Chirone, R. and Scherer, V.
PROCEEDINGS OF THE COMBUSTION INSTITUTE. Volume: 37 (2019) - 2019 • 5677
Direct, Selective Production of Aromatic Alcohols from Ethanol Using a Tailored Bifunctional Cobalt-Hydroxyapatite Catalyst
Wang, Q.-N. and Weng, X.-F. and Zhou, B.-C. and Lv, S.-P. and Miao, S. and Zhang, D. and Han, Y. and Scott, S.L. and Schüth, F. and Lu, A.-H.
ACS CATALYSIS. Volume: 9 (2019) - 2019 • 5676
Engineering atomic-level complexity in high-entropy and complex concentrated alloys
Oh, H.S. and Kim, S.J. and Odbadrakh, K. and Ryu, W.H. and Yoon, K.N. and Mu, S. and Körmann, F. and Ikeda, Y. and Tasan, C.C. and Raabe, D. and Egami, T. and Park, E.S.
NATURE COMMUNICATIONS. Volume: 10 (2019) - 2019 • 5675
Hydroxyapatite nanowires rich in [Ca-O-P] sites for ethanol direct coupling showing high C6-12 alcohol yield
Wang, Q.-N. and Zhou, B.-C. and Weng, X.-F. and Lv, S.-P. and Schüth, F. and Lu, A.-H.
CHEMICAL COMMUNICATIONS. Volume: 55 (2019) - 2019 • 5674
Differences in formation and oxidation of Colombian coal chars in air and oxy-fuel atmospheres
Debiagi, P.E.A. and Heuer, S. and Ontyd, C. and Schiemann, M. and Faravelli, T. and Hasse, C. and Scherer, V.
CHEMICAL ENGINEERING TRANSACTIONS. Volume: 74 (2019) - 2019 • 5673
Photogeneration of a single electron from a single Zeeman-resolved light-hole exciton with preserved angular momentum
Kuroyama, K. and Larsson, M. and Chang, C.Y. and Muramoto, J. and Heya, K. and Fujita, T. and Allison, G. and Valentin, S.R. and Ludwig, Ar. and Wieck, A.D. and Matsuo, S. and Oiwa, A. and Tarucha, S.
PHYSICAL REVIEW B. Volume: 99 (2019) - 2019 • 5672
Tungsten carbide as a deoxidation agent for plasma-facing tungsten-based materials
Šestan, A. and Zavašnik, J. and Kržmanc, M.M. and Kocen, M. and Jenuš, P. and Novak, S. and Čeh, M. and Dehm, G.
JOURNAL OF NUCLEAR MATERIALS. Volume: 524 (2019) - 2019 • 5671
Influence of Cr Alloying (1.5 to 5 at.%) on Martensitic Phase Transformation Temperatures in Co-Ni-Ga-Cr Thin Films
Decker, P. and Fortmann, J. and Salomon, S. and Krooß, P. and Niendorf, T. and Ludwig, Al.
SHAPE MEMORY AND SUPERELASTICITY. Volume: 5 (2019) - 2019 • 5670
Investigation on growth mechanisms of columnar structured YSZ coatings in Plasma Spray-Physical Vapor Deposition (PS-PVD)
He, W. and Mauer, G. and Sohn, Y.J. and Schwedt, A. and Guillon, O. and Vaßen, R.
JOURNAL OF THE EUROPEAN CERAMIC SOCIETY. Volume: 39 (2019) - 2019 • 5669
Optical Detection of Single-Electron Tunneling into a Semiconductor Quantum Dot
Kurzmann, A. and Stegmann, P. and Kerski, J. and Schott, R. and Ludwig, Ar. and Wieck, A.D. and König, J. and Lorke, A. and Geller, M.
PHYSICAL REVIEW LETTERS. Volume: 122 (2019) - 2019 • 5668
The interaction between grain boundary and tool geometry in nanocutting of a bi-crystal copper
Wang, Z. and Sun, T. and Zhang, H. and Li, G. and Li, Z. and Zhang, J. and Yan, Y. and Hartmaier, A.
INTERNATIONAL JOURNAL OF EXTREME MANUFACTURING. Volume: 1 (2019) - 2019 • 5667
Gradient-enhanced continuum models of healing in damaged soft tissues
He, Y. and Zuo, D. and Hackl, K. and Yang, H. and Mousavi, S.J. and Avril, S.
BIOMECHANICS AND MODELING IN MECHANOBIOLOGY. Volume: 18 (2019) - 2019 • 5666
Optimal designs for frequentist model averaging
Alhorn, K. and Schorning, K. and Dette, H.
BIOMETRIKA. Volume: 106 (2019) - 2019 • 5665
Joint contribution of transformation and twinning to the high strength-ductility combination of a FeMnCoCr high entropy alloy at cryogenic temperatures
He, Z.F. and Jia, N. and Ma, D. and Yan, H.L. and Li, Z.M. and Raabe, D.
MATERIALS SCIENCE AND ENGINEERING A. Volume: 759 (2019) - 2019 • 5664
The Empirical Process of Residuals from an Inverse Regression
Kutta, T. and Bissantz, N. and Chown, J. and Dette, H.
MATHEMATICAL METHODS OF STATISTICS. Volume: 28 (2019) - 2019 • 5663
Catalytic Carbon Monoxide Oxidation over Potassium-Doped Manganese Dioxide Nanoparticles Synthesized by Spray Drying
Ollegott, K. and Peters, N. and Antoni, H. and Muhler, M.
EMISSION CONTROL SCIENCE AND TECHNOLOGY. Volume: (2019) - 2019 • 5662
Direct Growth of Highly Strained Pt Islands on Branched Ni Nanoparticles for Improved Hydrogen Evolution Reaction Activity
Alinezhad, A. and Gloag, L. and Benedetti, T.M. and Cheong, S. and Webster, R.F. and Roelsgaard, M. and Iversen, B.B. and Schuhmann, W. and Gooding, J.J. and Tilley, R.D.
JOURNAL OF THE AMERICAN CHEMICAL SOCIETY. Volume: 141 (2019) - 2019 • 5661
Thermodynamics of grain boundary segregation, interfacial spinodal and their relevance for nucleation during solid-solid phase transitions
Kwiatkowski da Silva, A. and Kamachali, R.D. and Ponge, D. and Gault, B. and Neugebauer, J. and Raabe, D.
ACTA MATERIALIA. Volume: 168 (2019) - 2019 • 5660
Cascade Reactions in Nanozymes: Spatially Separated Active Sites inside Ag-Core-Porous-Cu-Shell Nanoparticles for Multistep Carbon Dioxide Reduction to Higher Organic Molecules
O'Mara, P.B. and Wilde, P. and Benedetti, T.M. and Andronescu, C. and Cheong, S. and Gooding, J.J. and Tilley, R.D. and Schuhmann, W.
JOURNAL OF THE AMERICAN CHEMICAL SOCIETY. Volume: 141 (2019) - 2019 • 5659
Three-Dimensional Characterization of Cracks in a Columnar Thermal Barrier Coating System for Gas Turbine Applications
Dennstedt, A. and Gaslain, F. and Bartsch, M. and Guipont, V. and Maurel, V.
INTEGRATING MATERIALS AND MANUFACTURING INNOVATION. Volume: 8 (2019) - 2019 • 5658
Spectral solvers for crystal plasticity and multi-physics simulations
Shanthraj, P. and Diehl, M. and Eisenlohr, P. and Roters, F. and Raabe, D.
HANDBOOK OF MECHANICS OF MATERIALS. Volume: (2019) - 2019 • 5657
Antifouling Properties of Dendritic Polyglycerols against Marine Macrofouling Organisms
Wanka, R. and Aldred, N. and Finlay, J.A. and Amuthalingam, A. and Clarke, J.L. and Clare, A.S. and Rosenhahn, A.
LANGMUIR. Volume: 35 (2019) - 2019 • 5656
Spray-Flame-Synthesized LaCo1−xFexO3 Perovskite Nanoparticles as Electrocatalysts for Water and Ethanol Oxidation
Alkan, B. and Cychy, S. and Varhade, S. and Muhler, M. and Schulz, C. and Schuhmann, W. and Wiggers, H. and Andronescu, C.
CHEMELECTROCHEM. Volume: 6 (2019) - 2019 • 5655
Difference in charge and spin dynamics in a quantum dot-lead coupled system
Otsuka, T. and Nakajima, T. and Delbecq, M.R. and Stano, P. and Amaha, S. and Yoneda, J. and Takeda, K. and Allison, G. and Li, S. and Noiri, A. and Ito, T. and Loss, D. and Ludwig, Ar. and Wieck, A.D. and Tarucha, S.
PHYSICAL REVIEW B. Volume: 99 (2019) - 2019 • 5654
Structural Changes to Supported Water Nanoislands Induced by Kosmotropic Ions
Weber, I. and Gerrard, N. and Hodgson, A. and Morgenstern, K.
JOURNAL OF PHYSICAL CHEMISTRY C. Volume: 123 (2019) - 2019 • 5653
Phase-field simulation of martensite microstructure in low-carbon steel
Shchyglo, O. and Du, G. and Engels, J.K. and Steinbach, I.
ACTA MATERIALIA. Volume: 175 (2019) - 2019 • 5652
Rhodium-Catalyzed ortho-Arylation of (Hetero)aromatic Acids
Weber, P. and Rank, C.K. and Yalcinkaya, E. and Dyga, M. and van Lingen, T. and Schmid, R. and Patureau, F.W. and Gooßen, L.J.
ADVANCED SYNTHESIS AND CATALYSIS. Volume: 361 (2019) - 2019 • 5651
Reconstructing the austenite parent microstructure of martensitic steels: A case study for reduced-activation Eurofer steels
Almeida Junior, D.R. and Zilnyk, K.D. and Raabe, D. and Sandim, H.R.Z.
JOURNAL OF NUCLEAR MATERIALS. Volume: 516 (2019) - 2019 • 5650
Optimal designs for regression with spherical data
Dette, H. and Konstantinou, M. and Schorning, K. and Gösmann, J.
ELECTRONIC JOURNAL OF STATISTICS. Volume: 13 (2019) - 2019 • 5649
Determinants of block Hankel matrices for random matrix-valued measures
Dette, H. and Tomecki, D.
STOCHASTIC PROCESSES AND THEIR APPLICATIONS. Volume: 129 (2019) - 2019 • 5648
High-Dimensional Neural Network Potentials for Atomistic Simulations
Hellström, M. and Behler, J.
ACS SYMPOSIUM SERIES. Volume: 1326 (2019) - 2019 • 5647
The blue in continuous-time regression models with correlated errors
Dette, H. and Pepelyshev, A. and Zhigljavsky, A.
ANNALS OF STATISTICS. Volume: 47 (2019) - 2019 • 5646
One-dimensional vs. two-dimensional proton transport processes at solid-liquid zinc-oxide-water interfaces
Hellström, M. and Quaranta, V. and Behler, J.
CHEMICAL SCIENCE. Volume: 10 (2019) - 2019 • 5645
Vibration control subjected to windup problem: An applied view on analysis and synthesis with convex formulation
Oveisi, A. and Nestorović, T.
CONTROL ENGINEERING PRACTICE. Volume: 82 (2019) - 2019 • 5644
Tbm drive along curved alignments: Model based prognosis of shield movement
Alsahly, A. and Marwan, A. and Meschke, G.
TUNNELS AND UNDERGROUND CITIES: ENGINEERING AND INNOVATION MEET ARCHAEOLOGY, ARCHITECTURE AND ART- PROCEEDINGS OF THE WTC 2019 ITA-AITES WORLD TUNNEL CONGRESS. Volume: (2019) - 2019 • 5643
Change point analysis of correlation in non-stationary time series
Dette, H. and Wu, W. and Zhou, Z.
STATISTICA SINICA. Volume: 29 (2019) - 2019 • 5642
Anti-thrombogenic coatings for devices in neurointerventional surgery: Case report and review of the literature
Henkes, H. and Bhogal, P. and Aguilar Pérez, M. and Lenz-Habijan, T. and Bannewitz, C. and Peters, M. and Sengstock, C. and Ganslandt, O. and Lylyk, P. and Monstadt, H.
INTERVENTIONAL NEURORADIOLOGY. Volume: 25 (2019) - 2019 • 5641
Mixed Kalman-fuzzy sliding mode state observer in disturbance rejection control of a vibrating smart structure
Oveisi, A. and Nestorović, T.
INTERNATIONAL JOURNAL OF ACOUSTICS AND VIBRATIONS. Volume: 24 (2019) - 2019 • 5640
Introducing Pseudocapacitive Bioelectrodes into a Biofuel Cell/Biosupercapacitor Hybrid Device for Optimized Open Circuit Voltage
Alsaoub, S. and Conzuelo, F. and Gounel, S. and Mano, N. and Schuhmann, W. and Ruff, A.
CHEMELECTROCHEM. Volume: 6 (2019) - 2019 • 5639
Detecting relevant changes in the mean of nonstationary processes - A mass excess approach1
Dette, H. and Wu, W.
ANNALS OF STATISTICS. Volume: 47 (2019) - 2019 • 5638
On the Oxidation Behavior and Its Influence on the Martensitic Transformation of Ti–Ta High-Temperature Shape Memory Alloys
Langenkämper, D. and Paulsen, A. and Somsen, C. and Frenzel, J. and Eggeler, G.
SHAPE MEMORY AND SUPERELASTICITY. Volume: 5 (2019) - 2019 • 5637
Some explicit solutions of c-optimal design problems for polynomial regression with no intercept
Dette, H. and Melas, V.B. and Shpilev, P.
ANNALS OF THE INSTITUTE OF STATISTICAL MATHEMATICS. Volume: (2019) - 2019 • 5636
Understanding the role of cu and clustering on strain hardening and strain rate sensitivity of al-mg-si-cu alloys
Langille, M. and Diak, B.J. and De Geuser, F. and Guiglionda, G. and Meddeb, S. and Zhao, H. and Gault, B. and Raabe, D. and Deschamps, A.
MINERALS, METALS AND MATERIALS SERIES. Volume: (2019) - 2019 • 5635
On the compositional partitioning during phase transformation in a binary ferromagnetic MnAl alloy
Palanisamy, D. and Raabe, D. and Gault, B.
ACTA MATERIALIA. Volume: 174 (2019) - 2019 • 5634
A sensitive and selective graphene/cobalt tetrasulfonated phthalocyanine sensor for detection of dopamine
Diab, N. and Morales, D.M. and Andronescu, C. and Masoud, M. and Schuhmann, W.
SENSORS AND ACTUATORS, B: CHEMICAL. Volume: 285 (2019) - 2019 • 5633
Temperature dependence of elastic moduli in a refractory HfNbTaTiZr high-entropy alloy
Laplanche, G. and Gadaud, P. and Perrière, L. and Guillot, I. and Couzinié, J.P.
JOURNAL OF ALLOYS AND COMPOUNDS. Volume: 799 (2019) - 2019 • 5632
Iron Aluminides
Palm, M. and Stein, F. and Dehm, G.
ANNUAL REVIEW OF MATERIALS RESEARCH. Volume: 49 (2019) - 2019 • 5631
Photocatalytic one-step synthesis of Ag nanoparticles without reducing agent and their catalytic redox performance supported on carbon
Shui, L. and Zhang, G. and Hu, B. and Chen, X. and Jin, M. and Zhou, G. and Li, N. and Muhler, M. and Peng, B.
JOURNAL OF ENERGY CHEMISTRY. Volume: 36 (2019) - 2019 • 5630
Site-specific quasi in situ investigation of primary static recrystallization in a low carbon steel
Diehl, M. and Kertsch, L. and Traka, K. and Helm, D. and Raabe, D.
MATERIALS SCIENCE AND ENGINEERING A. Volume: 755 (2019) - 2019 • 5629
Mitigation of EC breakdown in the gyrotron transmission line of the ITER Collective Thomson Scattering diagnostic via a Split Biased Waveguide
Larsen, A.W. and Korsholm, S.B. and Gonçalves, B. and Gutierrez, H.E. and Henriques, E. and Infante, V. and Jensen, T. and Jessen, M. and Klinkby, E.B. and NonbØl, E. and Luis, R. and Vale, A. and Lopes, A. and Naulin, V. and Nielsen, S.K. and Salewski, M. and Rasmussen, J. and Taormina, A. and MØllsØe, C. and Mussenbrock, T. and Trieschmann, J.
JOURNAL OF INSTRUMENTATION. Volume: 14 (2019) - 2019 • 5628
Correlating the short-time current response of a hydrogen evolving nickel electrode to bubble growth
Pande, N. and Mul, G. and Lohse, D. and Mei, B.
JOURNAL OF THE ELECTROCHEMICAL SOCIETY. Volume: 166 (2019) - 2019 • 5627
Molecular Design of Zwitterionic Polymer Interfaces: Searching for the Difference
Laschewsky, A. and Rosenhahn, A.
LANGMUIR. Volume: 35 (2019) - 2019 • 5626
Elemental site occupancy in the L12 A3B ordered intermetallic phase in Co-based superalloys and its influence on the microstructure
Pandey, P. and Makineni, S.K. and Samanta, A. and Sharma, A. and Das, S.M. and Nithin, B. and Srivastava, C. and Singh, A.K. and Raabe, D. and Gault, B. and Chattopadhyay, K.
ACTA MATERIALIA. Volume: 163 (2019) - 2019 • 5625
Studying grain boundary strengthening by dislocation-based strain gradient crystal plasticity coupled with a multi-phase-field model
Amin, W. and Ali, M.A. and Vajragupta, N. and Hartmaier, A.
MATERIALS. Volume: 12 (2019) - 2019 • 5624
Tuning the magnetic anisotropy of niptmnga by substitution and epitaxial strain
Herper, H.C. and Grunebohm, A.
IEEE TRANSACTIONS ON MAGNETICS. Volume: 55 (2019) - 2019 • 5623
Strength of hydrogen-free and hydrogen-doped Ni50Ti50 shape memory platelets
Weiser, A. and Buršíková, V. and Jarý, M. and Dymáček, P. and Dugáček, J. and Frenzel, J. and Čermák, J. and Dlouhý, A.
SCRIPTA MATERIALIA. Volume: 162 (2019) - 2019 • 5622
High-surface-area corundum by mechanochemically induced phase transformation of boehmite
Amrute, A.P. and Łodziana, Z. and Schreyer, H. and Weidenthaler, C. and Schüth, F.
SCIENCE. Volume: 366 (2019) - 2019 • 5621
Strength of hydrogen-free and hydrogen-doped Ni 50 Ti 50 shape memory platelets
Weiser, A. and Buršíková, V. and Jarý, M. and Dymáček, P. and Dugáček, J. and Frenzel, J. and Čermák, J. and Dlouhý, A.
SCRIPTA MATERIALIA. Volume: 162 (2019) - 2019 • 5620
Real-time nanoscale observation of deformation mechanisms in CrCoNi-based medium- to high-entropy alloys at cryogenic temperatures
Ding, Q. and Fu, X. and Chen, D. and Bei, H. and Gludovatz, B. and Li, J. and Zhang, Z. and George, E.P. and Yu, Q. and Zhu, T. and Ritchie, R.O.
MATERIALS TODAY. Volume: 25 (2019) - 2019 • 5619
Effect of nanometric γ′-particles on the stress-induced martensitic transformation in 〈001〉-oriented Co49Ni21Ga30 shape memory alloy single crystals
Lauhoff, C. and Reul, A. and Langenkämper, D. and Krooß, P. and Somsen, C. and Gutmann, M.J. and Kireeva, I. and Chumlyakov, Y.I. and Schmahl, W.W. and Niendorf, T.
SCRIPTA MATERIALIA. Volume: 168 (2019) - 2019 • 5618
Nanomechanical single-photon routing
Papon, C. and Zhou, X. and Thyrrestrup, H. and Liu, Z. and Stobbe, S. and Schott, R. and Wieck, A.D. and Ludwig, Ar. and Lodahl, P. and Midolo, L.
OPTICA. Volume: 6 (2019) - 2019 • 5617
Coherent Optical Control of a Quantum-Dot Spin-Qubit in a Waveguide-Based Spin-Photon Interface
Ding, D. and Appel, M.H. and Javadi, A. and Zhou, X. and Löbl, M.C. and Söllner, I. and Schott, R. and Papon, C. and Pregnolato, T. and Midolo, L. and Wieck, A.D. and Ludwig, Ar. and Warburton, R.J. and Schröder, T. and Lodahl, P.
PHYSICAL REVIEW APPLIED. Volume: 11 (2019) - 2019 • 5616
Towards maximized utilization of iridium for the acidic oxygen evolution reaction
Ledendecker, M. and Geiger, S. and Hengge, K. and Lim, J. and Cherevko, S. and Mingers, A.M. and Göhl, D. and Fortunato, G.V. and Jalalpoor, D. and Schüth, F. and Scheu, C. and Mayrhofer, K.J.J.
NANO RESEARCH. Volume: 12 (2019) - 2019 • 5615
First Evidence for Mechanism of Inverse Ripening from In-situ TEM and Phase-Field Study of δ′ Precipitation in an Al-Li Alloy
Park, J. and Kamachali, R.D. and Kim, S.-D. and Kim, S.-H. and Oh, C.-S. and Schwarze, C. and Steinbach, I.
SCIENTIFIC REPORTS. Volume: 9 (2019) - 2019 • 5614
Parallel Multistream Training of High-Dimensional Neural Network Potentials
Singraber, A. and Morawietz, T. and Behler, J. and Dellago, C.
JOURNAL OF CHEMICAL THEORY AND COMPUTATION. Volume: 15 (2019) - 2019 • 5613
Role of hole confinement in the recombination properties of InGaN quantum structures
Anikeeva, M. and Albrecht, M. and Mahler, F. and Tomm, J.W. and Lymperakis, L. and Chèze, C. and Calarco, R. and Neugebauer, J. and Schulz, T.
SCIENTIFIC REPORTS. Volume: 9 (2019) - 2019 • 5612
On the evolution of dislocation cell structures in two Al-alloys (Al-5Mg and Al-11Zn) during reciprocal sliding wear at high homologous temperatures
Parsa, A.B. and Walter, M. and Theisen, W. and Bürger, D. and Eggeler, G.
WEAR. Volume: (2019) - 2019 • 5611
Library-Based LAMMPS Implementation of High-Dimensional Neural Network Potentials
Singraber, A. and Behler, J. and Dellago, C.
JOURNAL OF CHEMICAL THEORY AND COMPUTATION. Volume: 15 (2019) - 2019 • 5610
Selective 2-Propanol Oxidation over Unsupported Co3O4 Spinel Nanoparticles: Mechanistic Insights into Aerobic Oxidation of Alcohols
Anke, S. and Bendt, G. and Sinev, I. and Hajiyani, H. and Antoni, H. and Zegkinoglou, I. and Jeon, H. and Pentcheva, R. and Roldan Cuenya, B. and Schulz, S. and Muhler, M.
ACS CATALYSIS. Volume: 9 (2019) - 2019 • 5609
Enhancing the water splitting performance of cryptomelane-type α-(K)MnO2
Antoni, H. and Morales, D.M. and Bitzer, J. and Fu, Q. and Chen, Y.-T. and Masa, J. and Kleist, W. and Schuhmann, W. and Muhler, M.
JOURNAL OF CATALYSIS. Volume: 374 (2019) - 2019 • 5608
Laser metal deposition of compositionally graded TiZrNbTa refractory high-entropy alloys using elemental powder blends
Dobbelstein, H. and Gurevich, E.L. and George, E.P. and Ostendorf, A. and Laplanche, G.
ADDITIVE MANUFACTURING. Volume: 25 (2019) - 2019 • 5607
Mechanical damage characterization in human femoropopliteal arteries of different ages
Anttila, E. and Balzani, D. and Desyatova, A. and Deegan, P. and MacTaggart, J. and Kamenskiy, A.
ACTA BIOMATERIALIA. Volume: 90 (2019) - 2019 • 5606
A Kinetic Study on the Evolution of Martensitic Transformation Behavior and Microstructures in Ti–Ta High-Temperature Shape-Memory Alloys During Aging
Paulsen, A. and Frenzel, J. and Langenkämper, D. and Rynko, R. and Kadletz, P. and Grossmann, L. and Schmahl, W.W. and Somsen, C. and Eggeler, G.
SHAPE MEMORY AND SUPERELASTICITY. Volume: 5 (2019) - 2019 • 5605
Development of a MOF-FF-compatible interaction model for liquid methanol and Cl− in methanol
Siwaipram, S. and Bopp, P.A. and Soetens, J.-C. and Schmid, R. and Bureekaew, S.
JOURNAL OF MOLECULAR LIQUIDS. Volume: 285 (2019) - 2019 • 5604
Helical magnetic ordering in Sr(Co1-xNix)2As2
Wilde, J.M. and Kreyssig, A. and Vaknin, D. and Sangeetha, N.S. and Li, B. and Tian, W. and Orth, P.P. and Johnston, D.C. and Ueland, B.G. and McQueeney, R.J.
PHYSICAL REVIEW B. Volume: 100 (2019) - 2019 • 5603
Evolution of coal char porosity from CO2-pyrolysis experiments
Heuer, S. and Wedler, C. and Ontyd, C. and Schiemann, M. and Span, R. and Richter, M. and Scherer, V.
FUEL. Volume: 253 (2019) - 2019 • 5602
Tailoring the porosity of a mesoporous carbon by a solvent-free mechanochemical approach
Leistenschneider, D. and Wegner, K. and Eßbach, C. and Sander, M. and Schneidermann, C. and Borchardt, L.
CARBON. Volume: 147 (2019) - 2019 • 5601
The puzzling issue of silica toxicity: Are silanols bridging the gaps between surface states and pathogenicity?
Pavan, C. and Delle Piane, M. and Gullo, M. and Filippi, F. and Fubini, B. and Hoet, P. and Horwell, C.J. and Huaux, F. and Lison, D. and Lo Giudice, C. and Martra, G. and Montfort, E. and Schins, R. and Sulpizi, M. and Wegner, K. and Wyart-Remy, M. and Ziemann, C. and Turci, F.
PARTICLE AND FIBRE TOXICOLOGY. Volume: 16 (2019) - 2019 • 5600
Bioinspired reactivity and coordination chemistry
Apfel, U.-P. and Suntharalingam, K.
DALTON TRANSACTIONS. Volume: 48 (2019) - 2019 • 5599
Tension/Compression asymmetry of a creep deformed single crystal Co-base superalloy
Lenz, M. and Eggeler, Y.M. and Müller, J. and Zenk, C.H. and Volz, N. and Wollgramm, P. and Eggeler, G. and Neumeier, S. and Göken, M. and Spiecker, E.
ACTA MATERIALIA. Volume: 166 (2019) - 2019 • 5598
Achieving ultra-high strength and ductility in equiatomic CrCoNi with partially recrystallized microstructures
Slone, C.E. and Miao, J. and George, E.P. and Mills, M.J.
ACTA MATERIALIA. Volume: 165 (2019) - 2019 • 5597
High temperature pyrolysis of lignite and synthetic carbons
Apicella, B. and Russo, C. and Ciajolo, A. and Cortese, L. and Cerciello, F. and Stanzione, F. and Wuetscher, A. and Muhler, M. and Senneca, O.
FUEL. Volume: (2019) - 2019 • 5596
On pinning-depinning and microkink-flow in solid state dewetting: Insights by in-situ ESEM on Al thin films
Hieke, S.W. and Willinger, M.-G. and Wang, Z.-J. and Richter, G. and Chatain, D. and Dehm, G. and Scheu, C.
ACTA MATERIALIA. Volume: 165 (2019) - 2019 • 5595
Analysis of in vivo optical coherence tomography images of human peripheral nerves using texture analysis
Lenz, M. and Möller, J. and Miemiec, R. and Carolus, A. and Brenke, C. and Schmieder, K. and Welp, H. and Gerhardt, N.C. and Hofmann, M.R.
PROGRESS IN BIOMEDICAL OPTICS AND IMAGING - PROCEEDINGS OF SPIE. Volume: 11073 (2019) - 2019 • 5594
Seleno-analogues of pentlandites (Fe4.5Ni4.5S8-: YSey, y = 1-6): Tuning bulk Fe/Ni sulphoselenides for hydrogen evolution
Smialkowski, M. and Siegmund, D. and Pellumbi, K. and Hensgen, L. and Antoni, H. and Muhler, M. and Apfel, U.-P.
CHEMICAL COMMUNICATIONS. Volume: 55 (2019) - 2019 • 5593
Enhancing the Selectivity between Oxygen and Chlorine towards Chlorine during the Anodic Chlorine Evolution Reaction on a Dimensionally Stable Anode
Wintrich, D. and Öhl, D. and Barwe, S. and Ganassin, A. and Möller, S. and Tarnev, T. and Botz, A. and Ruff, A. and Clausmeyer, J. and Masa, J. and Schuhmann, W.
CHEMELECTROCHEM. Volume: 6 (2019) - 2019 • 5592
Spontaneous Fluctuations in Mesoscopic Simulations of Nematic Liquid Crystals
Híjar, H. and Halver, R. and Sutmann, G.
FLUCTUATION AND NOISE LETTERS. Volume: 18 (2019) - 2019 • 5591
Study on Chemical Modifications of Glutathione by Cold Atmospheric Pressure Plasma (Cap) Operated in Air in the Presence of Fe(II) and Fe(III) Complexes
Śmiłowicz, D. and Kogelheide, F. and Stapelmann, K. and Awakowicz, P. and Metzler-Nolte, N.
SCIENTIFIC REPORTS. Volume: 9 (2019) - 2019 • 5590
Development of a high-temperature micromechanics stage with a novel temperature measurement approach
Arigela, V.G. and Oellers, T. and Ludwig, Al. and Kirchlechner, C. and Dehm, G.
REVIEW OF SCIENTIFIC INSTRUMENTS. Volume: 90 (2019) - 2019 • 5589
Time and Mechanism of Nanoparticle Functionalization by Macromolecular Ligands during Pulsed Laser Ablation in Liquids
Letzel, A. and Reich, S. and Dos Santos Rolo, T. and Kanitz, A. and Hoppius, J. and Rack, A. and Olbinado, M.P. and Ostendorf, A. and Gökce, B. and Plech, A. and Barcikowski, S.
LANGMUIR. Volume: 35 (2019) - 2019 • 5588
Electrochemical formation of Cr(III)-based films on Au electrodes
Smulders, V. and Simic, N. and Gomes, A.S.O. and Mei, B. and Mul, G.
ELECTROCHIMICA ACTA. Volume: 296 (2019) - 2019 • 5587
An Automated Computational Approach for Complete In-Plane Compositional Interface Analysis by Atom Probe Tomography
Peng, Z. and Lu, Y. and Hatzoglou, C. and Kwiatkowski Da Silva, A. and Vurpillot, F. and Ponge, D. and Raabe, D. and Gault, B.
MICROSCOPY AND MICROANALYSIS. Volume: 25 (2019) - 2019 • 5586
Unraveling the Metastability of Cn 2+ (n = 2-4) Clusters
Peng, Z. and Zanuttini, D. and Gervais, B. and Jacquet, E. and Blum, I. and Choi, P.-P. and Raabe, D. and Vurpillot, F. and Gault, B.
JOURNAL OF PHYSICAL CHEMISTRY LETTERS. Volume: 10 (2019) - 2019 • 5585
Atomic cluster expansion for accurate and transferable interatomic potentials
Drautz, R.
PHYSICAL REVIEW B. Volume: 99 (2019) - 2019 • 5584
Thermoelastic properties of rare-earth scandates SmScO3, TbScO3 and DyScO3
Hirschle, C. and Schreuer, J. and Ganschow, S. and Schulze-Jonack, I.
JOURNAL OF APPLIED PHYSICS. Volume: 126 (2019) - 2019 • 5583
Ab initio phase stabilities of Ce-based hard magnetic materials and comparison with experimental phase diagrams
Sözen, H.Ä. and Ener, S. and MacCari, F. and Skokov, K.P. and Gutfleisch, O. and Körmann, F. and Neugebauer, J. and Hickel, T.
PHYSICAL REVIEW MATERIALS. Volume: 3 (2019) - 2019 • 5582
Effect of Al, Ti and C additions on Widmanstätten microstructures and mechanical properties of cast Al0.6CoCrFeNi compositionally complex alloys
Asabre, A. and Kostka, A. and Stryzhyboroda, O. and Pfetzing-Micklich, J. and Hecht, U. and Laplanche, G.
MATERIALS AND DESIGN. Volume: 184 (2019) - 2019 • 5581
Comparative Study of Photocarrier Dynamics in CVD-deposited CuWO4, CuO, and WO3 Thin Films for Photoelectrocatalysis
Hirst, J. and Müller, S. and Peeters, D. and Sadlo, A. and Mai, L. and Reyes, O.M. and Friedrich, D. and Mitoraj, D. and Devi, A. and Beranek, R. and Eichberger, R.
ZEITSCHRIFT FUR PHYSIKALISCHE CHEMIE. Volume: (2019) - 2019 • 5580
Atomic level bonding mechanism in steel/aluminum joints produced by cold pressure welding
Peter, N.J. and Gerlitzky, C. and Altin, A. and Wohletz, S. and Krieger, W. and Tran, T.H. and Liebscher, C.H. and Scheu, C. and Dehm, G. and Groche, P. and Erbe, A.
MATERIALIA. Volume: 7 (2019) - 2019 • 5579
Ultrastrong Medium-Entropy Single-Phase Alloys Designed via Severe Lattice Distortion
Sohn, S.S. and Kwiatkowski da Silva, A. and Ikeda, Y. and Körmann, F. and Lu, W. and Choi, W.S. and Gault, B. and Ponge, D. and Neugebauer, J. and Raabe, D.
ADVANCED MATERIALS. Volume: 31 (2019) - 2019 • 5578
Data regarding the influence of Al, Ti, and C additions to as-cast Al0.6CoCrFeNi compositionally complex alloys on microstructures and mechanical properties
Asabre, A. and Pfetzing-Micklich, J. and Stryzhyboroda, O. and Kostka, A. and Hecht, U. and Laplanche, G.
DATA IN BRIEF. Volume: 27 (2019) - 2019 • 5577
Insights from 125Te and 57Fe nuclear resonance vibrational spectroscopy: A [4Fe-4Te] cluster from two points of view
Wittkamp, F. and Mishra, N. and Wang, H. and Wille, H.-C. and Steinbrügge, R. and Kaupp, M. and Cramer, S.P. and Apfel, U.-P. and Pelmenschikov, V.
CHEMICAL SCIENCE. Volume: 10 (2019) - 2019 • 5576
Au–Sn solders applied in transient liquid phase bonding: Microstructure and mechanical behavior
Du, C. and Soeler, R. and Völker, B. and Matoy, K. and Zechner, J. and Langer, G. and Reisinger, M. and Todt, J. and Kirchlechner, C. and Dehm, G.
MATERIALIA. Volume: 8 (2019) - 2019 • 5575
How close can indents be placed without risking an erroneous pop-in statistics?
Li, J. and Dehm, G. and Kirchlechner, C.
MATERIALIA. Volume: 7 (2019) - 2019 • 5574
Geometry of the Catalytic Active Site in [FeFe]-Hydrogenase Is Determined by Hydrogen Bonding and Proton Transfer
Duan, J. and Mebs, S. and Laun, K. and Wittkamp, F. and Heberle, J. and Happe, T. and Hofmann, E. and Apfel, U.-P. and Winkler, M. and Senger, M. and Haumann, M. and Stripp, S.T.
ACS CATALYSIS. Volume: 9 (2019) - 2019 • 5573
On detecting changes in the jumps of arbitrary size of a time-continuous stochastic process
Hoffmann, M. and Dette, H.
ELECTRONIC JOURNAL OF STATISTICS. Volume: 13 (2019) - 2019 • 5572
Composition of the nanosized orthorhombic O′ phase and its direct transformation to fine α during ageing in metastable β-Ti alloys
Li, T. and Lai, M. and Kostka, A. and Salomon, S. and Zhang, S. and Somsen, C. and Dargusch, M.S. and Kent, D.
SCRIPTA MATERIALIA. Volume: 170 (2019) - 2019 • 5571
Deformation compatibility between nanotwinned and recrystallized grains enhances resistance to interface cracking in cyclic loaded stainless steel
Li, Q. and Yan, F.K. and Tao, N.R. and Ponge, D. and Raabe, D. and Lu, K.
ACTA MATERIALIA. Volume: 165 (2019) - 2019 • 5570
Tailoring the Surface Structure of Silicon Carbide Support for Copper Catalyzed Ethanol Dehydrogenation
Li, M.-Y. and Lu, W.-D. and He, L. and Schüth, F. and Lu, A.-H.
CHEMCATCHEM. Volume: 11 (2019) - 2019 • 5569
Plasma state control of reactive sputter processes
Woelfel, C. and Oberberg, M. and Awakowicz, P. and Lunze, J.
INTERNATIONAL CONFERENCE OF CONTROL, DYNAMIC SYSTEMS, AND ROBOTICS. Volume: (2019) - 2019 • 5568
Ab initio molecular dynamics simulations of the ferroelectric-paraelectric phase transition in sodium nitrite
Dürholt, J.P. and Schmid, R.
PHYSICAL REVIEW MATERIALS. Volume: 3 (2019) - 2019 • 5567
Tuning the Electric Field Response of MOFs by Rotatable Dipolar Linkers
Dürholt, J.P. and Jahromi, B.F. and Schmid, R.
ACS CENTRAL SCIENCE. Volume: 5 (2019) - 2019 • 5566
A Concept for the Estimation of Soil-Tool Abrasive Wear Using ASTM-G65 Test Data
Hoormazdi, G. and Küpferle, J. and Röttger, A. and Theisen, W. and Hackl, K.
INTERNATIONAL JOURNAL OF CIVIL ENGINEERING. Volume: 17 (2019) - 2019 • 5565
Ab Initio Derived Force Fields for Zeolitic Imidazolate Frameworks: MOF-FF for ZIFs
Dürholt, J.P. and Fraux, G. and Coudert, F.-X. and Schmid, R.
JOURNAL OF CHEMICAL THEORY AND COMPUTATION. Volume: 15 (2019) - 2019 • 5564
Influence of adjacent defects on the permeability of plasmapolymer coated plastics
Hopmann, C. and Wilski, S. and Wipperfuerth, J. and Mitschker, F. and Awakowicz, P. and Dahlmann, R.
AIP CONFERENCE PROCEEDINGS. Volume: 2139 (2019) - 2019 • 5563
Segregation-driven grain boundary spinodal decomposition as a pathway for phase nucleation in a high-entropy alloy
Li, L. and Li, Z. and Kwiatkowski da Silva, A. and Peng, Z. and Zhao, H. and Gault, B. and Raabe, D.
ACTA MATERIALIA. Volume: 178 (2019) - 2019 • 5562
Strain partitioning and strain localization in medium manganese steels measured by in situ microscopic digital image correlation
Dutta, A. and Ponge, D. and Sandlöbes, S. and Raabe, D.
MATERIALIA. Volume: 5 (2019) - 2019 • 5561
On the rejuvenation of crept Ni-Base single crystal superalloys (SX)by hot isostatic pressing (HIP)
Horst, O.M. and Ruttert, B. and Bürger, D. and Heep, L. and Wang, H. and Dlouhý, A. and Theisen, W. and Eggeler, G.
MATERIALS SCIENCE AND ENGINEERING A. Volume: 758 (2019) - 2019 • 5560
Role of elemental intermixing at the In 2 S 3 /CIGSe heterojunction deposited using reactive RF magnetron sputtering
Soni, P. and Raghuwanshi, M. and Wuerz, R. and Berghoff, B. and Knoch, J. and Raabe, D. and Cojocaru-Mirédin, O.
SOLAR ENERGY MATERIALS AND SOLAR CELLS. Volume: 195 (2019) - 2019 • 5559
Competing magnetic phases and itinerant magnetic frustration in SrCo2As2
Li, B. and Ueland, B.G. and Jayasekara, W.T. and Abernathy, D.L. and Sangeetha, N.S. and Johnston, D.C. and Ding, Q.-P. and Furukawa, Y. and Orth, P.P. and Kreyssig, A. and Goldman, A.I. and McQueeney, R.J.
PHYSICAL REVIEW B. Volume: 100 (2019) - 2019 • 5558
Sputtering as a viable route for In2S3 buffer layer deposition in high efficiency Cu(In,Ga)Se2 solar cells
Soni, P. and Raghuwanshi, M. and Wuerz, R. and Berghoff, B. and Knoch, J. and Raabe, D. and Cojocaru-Mirédin, O.
ENERGY SCIENCE AND ENGINEERING. Volume: 7 (2019) - 2019 • 5557
Antiferromagnetic stacking of ferromagnetic layers and doping-controlled phase competition in Ca1-xSrxCo2-yAs2
Li, B. and Sizyuk, Y. and Sangeetha, N.S. and Wilde, J.M. and Das, P. and Tian, W. and Johnston, D.C. and Goldman, A.I. and Kreyssig, A. and Orth, P.P. and McQueeney, R.J. and Ueland, B.G.
PHYSICAL REVIEW B. Volume: 100 (2019) - 2019 • 5556
Bio-inspired design: Bulk iron-nickel sulfide allows for efficient solvent-dependent CO 2 reduction
Piontek, S. and Junge Puring, K. and Siegmund, D. and Smialkowski, M. and Sinev, I. and Tetzlaff, D. and Roldan Cuenya, B. and Apfel, U.-P.
CHEMICAL SCIENCE. Volume: 10 (2019) - 2019 • 5555
The electrochemical dissolution of single silver nanoparticles enlightened by hyperspectral dark-field microscopy
Wonner, K. and Evers, M.V. and Tschulik, K.
ELECTROCHIMICA ACTA. Volume: 301 (2019) - 2019 • 5554
A canonical rate-independent model of geometrically linear isotropic gradient plasticity with isotropic hardening and plastic spin accounting for the Burgers vector
Ebobisse, F. and Hackl, K. and Neff, P.
CONTINUUM MECHANICS AND THERMODYNAMICS. Volume: 31 (2019) - 2019 • 5553
Antenna pattern characterization with an industrial robot assisted FMCW radar system
Piotrowsky, L. and Bernhardt, V. and Barowski, J. and Rolfes, I. and Pohl, N.
ASIA-PACIFIC MICROWAVE CONFERENCE PROCEEDINGS, APMC. Volume: 2019-December (2019) - 2019 • 5552
Strain hardening mechanisms during cold rolling of a high-Mn steel: Interplay between submicron defects and microtexture
Souza Filho, I.R. and Sandim, M.J.R. and Ponge, D. and Sandim, H.R.Z. and Raabe, D.
MATERIALS SCIENCE AND ENGINEERING A. Volume: 754 (2019) - 2019 • 5551
Density functional theory calculations of iron - vanadium carbide interfaces and the effect of hydrogen
Echeverri Restrepo, S. and Di Stefano, D. and Mrovec, M. and Paxton, A.T.
INTERNATIONAL JOURNAL OF HYDROGEN ENERGY. Volume: (2019) - 2019 • 5550
Wavelength locking of Er-doped random fiber laser
Hu, B. and Zhang, W. and Ma, R. and Guo, J. and Ludwig, Ar. and Rao, Y.
LASER PHYSICS LETTERS. Volume: 16 (2019) - 2019 • 5549
A critical comparison of neural network potentials for molecular reaction dynamics with exact permutation symmetry
Li, J. and Song, K. and Behler, J.
PHYSICAL CHEMISTRY CHEMICAL PHYSICS. Volume: 21 (2019) - 2019 • 5548
Magnetic properties of a 17.6 Mn-TRIP steel: Study of strain-induced martensite formation, austenite reversion, and athermal α′-formation
Souza Filho, I.R. and Sandim, M.J.R. and Cohen, R. and Nagamine, L.C.C.M. and Sandim, H.R.Z. and Raabe, D.
JOURNAL OF MAGNETISM AND MAGNETIC MATERIALS. Volume: 473 (2019) - 2019 • 5547
From Molecular Fragments to the Bulk: Development of a Neural Network Potential for MOF-5
Eckhoff, M. and Behler, J.
JOURNAL OF CHEMICAL THEORY AND COMPUTATION. Volume: 15 (2019) - 2019 • 5546
Martensite to austenite reversion in a high-Mn steel: Partitioning-dependent two-stage kinetics revealed by atom probe tomography, in-situ magnetic measurements and simulation
Souza Filho, I.R. and Kwiatkowski da Silva, A. and Sandim, M.J.R. and Ponge, D. and Gault, B. and Sandim, H.R.Z. and Raabe, D.
ACTA MATERIALIA. Volume: 166 (2019) - 2019 • 5545
Highly Selective Anaerobic Oxidation of Alcohols Over Fe-doped SrTiO3 Under Visible Light
Hu, Y. and Zhao, G. and Pan, Q. and Wang, H. and Shen, Z. and Peng, B. and Busser, G.W. and Wang, X. and Muhler, M.
CHEMCATCHEM. Volume: 11 (2019) - 2019 • 5544
Nitrogen-Doped Metal-Free Carbon Materials Derived from Cellulose as Electrocatalysts for the Oxygen Reduction Reaction
Wütscher, A. and Eckhard, T. and Hiltrop, D. and Lotz, K. and Schuhmann, W. and Andronescu, C. and Muhler, M.
CHEMELECTROCHEM. Volume: 6 (2019) - 2019 • 5543
Comparison of K-doped and pure cold-rolled tungsten sheets: As-rolled condition and recrystallization behaviour after isochronal annealing at different temperatures
Lied, P. and Bonnekoh, C. and Pantleon, W. and Stricker, M. and Hoffmann, A. and Reiser, J.
INTERNATIONAL JOURNAL OF REFRACTORY METALS AND HARD MATERIALS. Volume: 85 (2019) - 2019 • 5542
Partitioning of interstitial segregants during decohesion: A DFT case study of the ∑3 symmetric tilt grain boundary in ferritic steel
Huang, X. and Janisch, R.
MATERIALS. Volume: 12 (2019) - 2019 • 5541
Deformation of Borides in Nickel-based Superalloys: A study of segregation at dislocations
Lilensten, L. and Antonov, S. and Raabe, D. and Tin, S. and Gault, B. and Kontis, P.
MICROSCOPY AND MICROANALYSIS. Volume: 25 (2019) - 2019 • 5540
Oxygen vacancies and hydrogen doping in LaAlO3/SrTiO3 heterostructures: Electronic properties and impact on surface and interface reconstruction
Piyanzina, I.I. and Eyert, V. and Lysogorskiy, Yu.V. and Tayurskii, D.A. and Kopp, T.
JOURNAL OF PHYSICS CONDENSED MATTER. Volume: 31 (2019) - 2019 • 5539
On the role of nitrogen on hydrogen environment embrittlement of high-interstitial austenitic CrMnC(N) steels
Egels, G. and Fussik, R. and Weber, S. and Theisen, W.
INTERNATIONAL JOURNAL OF HYDROGEN ENERGY. Volume: 44 (2019) - 2019 • 5538
Unraveling indentation-induced slip steps in austenitic stainless steel
Xia, W. and Dehm, G. and Brinckmann, S.
MATERIALS AND DESIGN. Volume: 183 (2019) - 2019 • 5537
Effect of processing on high-velocity water vapor recession behavior of Yb-silicate environmental barrier coatings
Bakan, E. and Sohn, Y.J. and Kunz, W. and Klemm, H. and Vaßen, R.
JOURNAL OF THE EUROPEAN CERAMIC SOCIETY. Volume: 39 (2019) - 2019 • 5536
Time-resolved ATP measurements during vesicle respiration
Lin, J. and Weixler, D. and Daboss, S. and Seibold, G.M. and Andronescu, C. and Schuhmann, W. and Kranz, C.
TALANTA. Volume: 205 (2019) - 2019 • 5535
Hybrid lining segments – bearing and fracture behavior of longitudinal joints
Plückelmann, S. and Breitenbücher, R.
TUNNELS AND UNDERGROUND CITIES: ENGINEERING AND INNOVATION MEET ARCHAEOLOGY, ARCHITECTURE AND ART- PROCEEDINGS OF THE WTC 2019 ITA-AITES WORLD TUNNEL CONGRESS. Volume: (2019) - 2019 • 5534
Orbital-Dependent Electronic Friction Significantly Affects the Description of Reactive Scattering of N2 from Ru(0001)
Spiering, P. and Shakouri, K. and Behler, J. and Kroes, G.-J. and Meyer, J.
JOURNAL OF PHYSICAL CHEMISTRY LETTERS. Volume: 10 (2019) - 2019 • 5533
High-temperature materials for power generation in gas turbines
Bakan, E. and Mack, D.E. and Mauer, G. and Vaßen, R. and Lamon, J. and Padture, N.P.
ADVANCED CERAMICS FOR ENERGY CONVERSION AND STORAGE. Volume: (2019) - 2019 • 5532
Microfluidic Shear Force Assay to Determine Cell Adhesion Forces
Hümmer, J. and Koc, J. and Rosenhahn, A. and Lee-Thedieck, C.
METHODS IN MOLECULAR BIOLOGY. Volume: 2017 (2019) - 2019 • 5531
Bearable local stress of high-strength steel fiber reinforced concrete [Aufnehmbare Teilflächenspannung von hochfestem Stahlfaserbeton]
Plückelmann, S. and Breitenbücher, R. and Smarslik, M. and Mark, P.
BETON- UND STAHLBETONBAU. Volume: 114 (2019) - 2019 • 5530
Light, strong and cost effective: Martensitic steels based on the Fe – Al – C system
Springer, H. and Zhang, J.-L. and Szczepaniak, A. and Belde, M. and Gault, B. and Raabe, D.
MATERIALS SCIENCE AND ENGINEERING A. Volume: 762 (2019) - 2019 • 5529
Nanoporous carbon: Liquid-free synthesis and geometry-dependent catalytic performance
Xu, R. and Kang, L. and Knossalla, J. and Mielby, J. and Wang, Q. and Wang, B. and Feng, J. and He, G. and Qin, Y. and Xie, J. and Swertz, A.-C. and He, Q. and Kegnæs, Sø. and Brett, D.J.L. and Schüth, F. and Wang, F.R.
ACS NANO. Volume: 13 (2019) - 2019 • 5528
Investigation of a liquid air energy storage (LAES) system with different cryogenic heat storage devices
Hüttermann, L. and Span, R. and Maas, P. and Scherer, V.
ENERGY PROCEDIA. Volume: 158 (2019) - 2019 • 5527
Ultrafast spin-lasers for optical data communication
Lindemann, M. and Xu, G. and Pusch, T. and Michalzik, R. and Hofmann, M.R. and Zutic, I. and Gerhardt, N.C.
2019 CONFERENCE ON LASERS AND ELECTRO-OPTICS EUROPE AND EUROPEAN QUANTUM ELECTRONICS CONFERENCE, CLEO/EUROPE-EQEC 2019. Volume: (2019) - 2019 • 5526
Influence of misfit dislocations on nanoisland decay
Sprodowski, C. and Morgenstern, K.
PHYSICAL REVIEW B. Volume: 100 (2019) - 2019 • 5525
Sensitive and selective detection of Cu2+ ions based on fluorescent Ag nanoparticles synthesized by R-phycoerythrin from marine algae Porphyra yezoensis
Xu, Y. and Hou, Y. and Wang, Y. and Wang, Y. and Li, T. and Song, C. and Wei, N. and Wang, Q.
ECOTOXICOLOGY AND ENVIRONMENTAL SAFETY. Volume: 168 (2019) - 2019 • 5524
Elemental re-distribution inside shear bands revealed by correlative atom-probe tomography and electron microscopy in a deformed metallic glass
Balachandran, S. and Orava, J. and Köhler, M. and Breen, A.J. and Kaban, I. and Raabe, D. and Herbig, M.
SCRIPTA MATERIALIA. Volume: 168 (2019) - 2019 • 5523
Intrinsic Activity of Oxygen Evolution Catalysts Probed at Single CoFe2O4 Nanoparticles
El Arrassi, A. and Liu, Z. and Evers, M.V. and Blanc, N. and Bendt, G. and Saddeler, S. and Tetzlaff, D. and Pohl, D. and Damm, C. and Schulz, S. and Tschulik, K.
JOURNAL OF THE AMERICAN CHEMICAL SOCIETY. Volume: 141 (2019) - 2019 • 5522
Tailoring the Adsorption of ACE-Inhibiting Peptides by Nitrogen Functionalization of Porous Carbons
Huettner, C. and Hagemann, D. and Troschke, E. and Hippauf, F. and Borchardt, L. and Oswald, S. and Henle, T. and Kaskel, S.
LANGMUIR. Volume: 35 (2019) - 2019 • 5521
Ultrafast spin-lasers
Lindemann, M. and Xu, G. and Pusch, T. and Michalzik, R. and Hofmann, M.R. and Žutić, I. and Gerhardt, N.C.
NATURE. Volume: 568 (2019) - 2019 • 5520
Altering the stability of nanoislands through core-shell supports
Sprodowski, C. and Morgenstern, K.
NANOSCALE. Volume: 11 (2019) - 2019 • 5519
Fano line shapes created in metamaterials by integrating different modes of the same symmetry in composite structures
Xu, R. and Wieck, S.A.D. and Jukam, N.
INTERNATIONAL CONFERENCE ON INFRARED, MILLIMETER, AND TERAHERTZ WAVES, IRMMW-THZ. Volume: 2019-September (2019) - 2019 • 5518
A stacked planar sensor concept for minimally invasive plasma monitoring
Pohle, D. and Schulz, C. and Oberberg, M. and Friedrichs, M. and Serwa, A. and Uhlig, P. and Oberrath, J. and Awakowicz, P. and Rolfes, I.
ASIA-PACIFIC MICROWAVE CONFERENCE PROCEEDINGS, APMC. Volume: 2018-November (2019) - 2019 • 5517
Temperature-dependent accommodation of two lattices of largely different size during growth
Sprodowski, C. and Morgenstern, K.
NANOMATERIALS. Volume: 9 (2019) - 2019 • 5516
Atomic-scale investigation of hydrogen distribution in a Ti–Mo alloy
Yan, F. and Mouton, I. and Stephenson, L.T. and Breen, A.J. and Chang, Y. and Ponge, D. and Raabe, D. and Gault, B.
SCRIPTA MATERIALIA. Volume: 162 (2019) - 2019 • 5515
Effects of the Ion to Growth Flux Ratio on the Constitution and Mechanical Properties of Cr1- x-Alx-N Thin Films
Banko, L. and Ries, S. and Grochla, D. and Arghavani, M. and Salomon, S. and Pfetzing-Micklich, J. and Kostka, A. and Rogalla, D. and Schulze, J. and Awakowicz, P. and Ludwig, Al.
ACS COMBINATORIAL SCIENCE. Volume: 21 (2019) - 2019 • 5514
Millimeter Wave Short Range SAR Imaging using Cross-Polarized Radar System
Elsaadouny, M. and Barowski, J. and Rolfes, I.
2018 IEEE 9TH ANNUAL INFORMATION TECHNOLOGY, ELECTRONICS AND MOBILE COMMUNICATION CONFERENCE, IEMCON 2018. Volume: (2019) - 2019 • 5513
Minimally Invasive Supervision of Plasma-assisted Dielectric Deposition Processes
Pohle, D. and Schulz, C. and Oberberg, M. and Awakowicz, P. and Rolfes, I.
IMWS-AMP 2019 - 2019 IEEE MTT-S INTERNATIONAL MICROWAVE WORKSHOP SERIES ON ADVANCED MATERIALS AND PROCESSES FOR RF AND THZ APPLICATIONS. Volume: (2019) - 2019 • 5512
Induced Growth from a Ag Gas on Cu(111)
Sprodowski, C. and Morgenstern, K.
JOURNAL OF PHYSICAL CHEMISTRY C. Volume: 123 (2019) - 2019 • 5511
A convolutional neural network for the non-destructive testing of 3D-printed samples
Elsaadouny, M. and Barowski, J. and Rolfes, I.
INTERNATIONAL CONFERENCE ON INFRARED, MILLIMETER, AND TERAHERTZ WAVES, IRMMW-THZ. Volume: 2019-September (2019) - 2019 • 5510
Temperature dependence of the vibrational spectrum of porphycene: A qualitative failure of classical-nuclei molecular dynamics†
Litman, Y. and Behler, J. and Rossi, M.
FARADAY DISCUSSIONS. Volume: 221 (2019) - 2019 • 5509
Electrochemical oxidation of H2S on polycrystalline Ni electrodes
Yang, J. and Smulders, V. and Smits, J.J.T. and Mei, B.T. and Mul, G.
JOURNAL OF APPLIED ELECTROCHEMISTRY. Volume: 49 (2019) - 2019 • 5508
Millimeter wave SAR imaging for the non-destructive testing of 3D-printed samples
Elsaadouny, M. and Barowski, J. and Jebramcik, J. and Rolfes, I.
PROCEEDINGS OF THE 2019 21ST INTERNATIONAL CONFERENCE ON ELECTROMAGNETICS IN ADVANCED APPLICATIONS, ICEAA 2019. Volume: (2019) - 2019 • 5507
Non-Destructive Testing of 3D-printed Samples based on Machine Learning
Elsaadouny, M. and Barowski, J. and Rolfes, I.
IMWS-AMP 2019 - 2019 IEEE MTT-S INTERNATIONAL MICROWAVE WORKSHOP SERIES ON ADVANCED MATERIALS AND PROCESSES FOR RF AND THZ APPLICATIONS. Volume: (2019) - 2019 • 5506
Columnar to equiaxed transition and grain refinement of cast CrCoNi medium-entropy alloy by microalloying with titanium and carbon
Liu, X.W. and Laplanche, G. and Kostka, A. and Fries, S.G. and Pfetzing-Micklich, J. and Liu, G. and George, E.P.
JOURNAL OF ALLOYS AND COMPOUNDS. Volume: 775 (2019) - 2019 • 5505
Ionic Conductivity and Its Dependence on Structural Disorder in Halogenated Argyrodites Li6PS5X (X = Br, Cl, I)
Stamminger, A.R. and Ziebarth, B. and Mrovec, M. and Hammerschmidt, T. and Drautz, R.
CHEMISTRY OF MATERIALS. Volume: (2019) - 2019 • 5504
Humanitarian Microwave Imaging Enhancement and Classification of Shallowly Buried Objects
Elsaadouny, M. and Barowski, J. and Rolfes, I.
2019 IEEE 10TH ANNUAL INFORMATION TECHNOLOGY, ELECTRONICS AND MOBILE COMMUNICATION CONFERENCE, IEMCON 2019. Volume: (2019) - 2019 • 5503
Solvent-Controlled CO 2 Reduction by a Triphos-Iron Hydride Complex
Iffland, L. and Khedkar, A. and Petuker, A. and Lieb, M. and Wittkamp, F. and Van Gastel, M. and Roemelt, M. and Apfel, U.-P.
ORGANOMETALLICS. Volume: 38 (2019) - 2019 • 5502
Correlated mode analysis of a microwave driven ICP source
Porteanu, H.-E. and Stefanović, I. and Bibinov, N. and Klute, M. and Awakowicz, P. and Brinkmann, R.P. and Heinrich, W.
PLASMA SOURCES SCIENCE AND TECHNOLOGY. Volume: 28 (2019) - 2019 • 5501
A compact measurement setup for in-situ material characterization in the lower THz range
Barowski, J. and Jebramcik, J. and Alawneh, I. and Sheikh, F. and Kaiser, T. and Rolfes, I.
2019 2ND INTERNATIONAL WORKSHOP ON MOBILE TERAHERTZ SYSTEMS, IWMTS 2019. Volume: (2019) - 2019 • 5500
A SAR Image Enhancement Algorithm using the Discrete Wavelet Transform
Elsaadouny, M. and Barowski, J. and Rolfes, I.
2019 IEEE 10TH ANNUAL INFORMATION TECHNOLOGY, ELECTRONICS AND MOBILE COMMUNICATION CONFERENCE, IEMCON 2019. Volume: (2019) - 2019 • 5499
MD simulation of stress-assisted nanometric cutting mechanism of 3C silicon carbide
Liu, L. and Xu, Z. and Tian, D. and Hartmaier, A. and Luo, X. and Zhang, J. and Nordlund, K. and Fang, F.
INDUSTRIAL LUBRICATION AND TRIBOLOGY. Volume: 71 (2019) - 2019 • 5498
Inductively Coupled Plasma at Atmospheric Pressure, a Challenge for Miniature Devices
Porteanu, H.-E. and Stefanovic, I. and Klute, M. and Brinkmann, R.-P. and Awakowicz, P. and Heinrich, W.
IEEE INTERNATIONAL PULSED POWER CONFERENCE. Volume: 2019-June (2019) - 2019 • 5497
Deciphering Charge Transfer and Electronic Polarization Effects at Gold Nanocatalysts on Reduced Titania Support
Yoo, S.-H. and Siemer, N. and Todorova, M. and Marx, D. and Neugebauer, J.
JOURNAL OF PHYSICAL CHEMISTRY C. Volume: 123 (2019) - 2019 • 5496
Spatial Identification of Dielectric Properties using Synthetic Aperture Radar
Barowski, J. and Jebramcik, J. and Wagner, J. and Pohl, N. and Rolfes, I.
IMWS-AMP 2019 - 2019 IEEE MTT-S INTERNATIONAL MICROWAVE WORKSHOP SERIES ON ADVANCED MATERIALS AND PROCESSES FOR RF AND THZ APPLICATIONS. Volume: (2019) - 2019 • 5495
The Subsurface Objects Classification using a Convolutional Neural Network
Elsaadouny, M. and Barowski, J. and Rolfes, I.
2019 IEEE 10TH ANNUAL INFORMATION TECHNOLOGY, ELECTRONICS AND MOBILE COMMUNICATION CONFERENCE, IEMCON 2019. Volume: (2019) - 2019 • 5494
Impact of interstitial C on phase stability and stacking-fault energy of the CrMnFeCoNi high-entropy alloy
Ikeda, Y. and Tanaka, I. and Neugebauer, J. and Körmann, F.
PHYSICAL REVIEW MATERIALS. Volume: 3 (2019) - 2019 • 5493
On the interaction of precipitates and tensile twins in magnesium alloys
Liu, C. and Shanthraj, P. and Robson, J.D. and Diehl, M. and Dong, S. and Dong, J. and Ding, W. and Raabe, D.
ACTA MATERIALIA. Volume: 178 (2019) - 2019 • 5492
Comparing pore structure models of nanoporous carbons obtained from small angle X-ray scattering and gas adsorption
Prehal, C. and Grätz, S. and Krüner, B. and Thommes, M. and Borchardt, L. and Presser, V. and Paris, O.
CARBON. Volume: 152 (2019) - 2019 • 5491
Consolidation of Single-Component Grouting Mortars in the Course of Dewatering: Redistribution of Particles
Youn-Čale, B.-Y. and Breitenbücher, R.
INTERNATIONAL JOURNAL OF CIVIL ENGINEERING. Volume: 17 (2019) - 2019 • 5490
Crystal growth, microstructure, and physical properties of SrMnSb2
Liu, Y. and Ma, T. and Zhou, L. and Straszheim, W.E. and Islam, F. and Jensen, B.A. and Tian, W. and Heitmann, T. and Rosenberg, R.A. and Wilde, J.M. and Li, B. and Kreyssig, A. and Goldman, A.I. and Ueland, B.G. and McQueeney, R.J. and Vaknin, D.
PHYSICAL REVIEW B. Volume: 99 (2019) - 2019 • 5489
Expansion and deterioration of concrete due to ASR: Micromechanical modeling and analysis
Iskhakov, T. and Timothy, J.J. and Meschke, G.
CEMENT AND CONCRETE RESEARCH. Volume: 115 (2019) - 2019 • 5488
Contrast of 83% in reflection measurements on a single quantum dot
Lochner, P. and Kurzmann, A. and Schott, R. and Wieck, A.D. and Ludwig, Ar. and Lorke, A. and Geller, M.
SCIENTIFIC REPORTS. Volume: 9 (2019) - 2019 • 5487
Vertical-cavity surface-emitting laser with integrated surface grating for high birefringence splitting
Pusch, T. and Debernardi, P. and Lindemann, M. and Erb, F. and Gerhardt, N.C. and Hofmann, M.R. and Michalzik, R.
ELECTRONICS LETTERS. Volume: 55 (2019) - 2019 • 5486
Enhanced propylene oxide selectivity for gas phase direct propylene epoxidation by lattice expansion of silver atoms on nickel nanoparticles
Yu, B. and Ayvalı, T. and Raine, E. and Li, T. and Li, M.M.-J. and Zheng, J. and Wu, S. and Bagabas, A.A. and Tsang, S.C.E.
APPLIED CATALYSIS B: ENVIRONMENTAL. Volume: 243 (2019) - 2019 • 5485
In situ formed vanadium-oxide cathode coatings for selective hydrogen production
Endrődi, B. and Smulders, V. and Simic, N. and Wildlock, M. and Mul, G. and Mei, B. and Cornell, A.
APPLIED CATALYSIS B: ENVIRONMENTAL. Volume: 244 (2019) - 2019 • 5484
Excitons in InGaAs quantum dots without electron wetting layer states
Löbl, M.C. and Scholz, S. and Söllner, I. and Ritzmann, J. and Denneulin, T. and Kovács, A. and Kardynał, B.E. and Wieck, A.D. and Ludwig, Ar. and Warburton, R.J.
COMMUNICATIONS PHYSICS. Volume: 2 (2019) - 2019 • 5483
Birefringent surface gratings for ultrafast spin-VCSELs
Pusch, T. and Debernardi, P. and Lindemann, M. and Gerhardt, N.C. and Hofmann, M.R. and Michalzik, R.
2019 CONFERENCE ON LASERS AND ELECTRO-OPTICS EUROPE AND EUROPEAN QUANTUM ELECTRONICS CONFERENCE, CLEO/EUROPE-EQEC 2019. Volume: (2019) - 2019 • 5482
Photocurrent Recombination through Surface Segregation in Al-Cr-Fe-O Photocathodes
Stein, H.S. and Zhang, S. and Li, Y. and Scheu, C. and Ludwig, Al.
ZEITSCHRIFT FUR PHYSIKALISCHE CHEMIE. Volume: (2019) - 2019 • 5481
Layer-by-layer constructed hyaluronic acid/chitosan multilayers as antifouling and fouling-release coatings
Yu, W. and Koc, J. and Finlay, J.A. and Clarke, J.L. and Clare, A.S. and Rosenhahn, A.
BIOINTERPHASES. Volume: 14 (2019) - 2019 • 5480
Cobalt metalloid and polybenzoxazine derived composites for bifunctional oxygen electrocatalysis
Barwe, S. and Andronescu, C. and Engels, R. and Conzuelo, F. and Seisel, S. and Wilde, P. and Chen, Y.-T. and Masa, J. and Schuhmann, W.
ELECTROCHIMICA ACTA. Volume: 297 (2019) - 2019 • 5479
Parameterization of a Non-local Crystal Plasticity Model for Tempered Lath Martensite Using Nanoindentation and Inverse Method
Engels, J.K. and Vajragupta, N. and Hartmaier, A.
FRONTIERS IN MATERIALS. Volume: 6 (2019) - 2019 • 5478
Lanthanum tungstate membranes for H 2 extraction and CO 2 utilization: Fabrication strategies based on sequential tape casting and plasma-spray physical vapor deposition
Ivanova, M.E. and Deibert, W. and Marcano, D. and Escolástico, S. and Mauer, G. and Meulenberg, W.A. and Bram, M. and Serra, J.M. and Vaßen, R. and Guillon, O.
SEPARATION AND PURIFICATION TECHNOLOGY. Volume: (2019) - 2019 • 5477
Correlations between optical properties and Voronoi-cell area of quantum dots
Löbl, M.C. and Zhai, L. and Jahn, J.-P. and Ritzmann, J. and Huo, Y. and Wieck, A.D. and Schmidt, O.G. and Ludwig, Ar. and Rastelli, A. and Warburton, R.J.
PHYSICAL REVIEW B. Volume: 100 (2019) - 2019 • 5476
Lanthanum tungstate membranes for H2 extraction and CO2 utilization: Fabrication strategies based on sequential tape casting and plasma-spray physical vapor deposition
Ivanova, M.E. and Deibert, W. and Marcano, D. and Escolástico, S. and Mauer, G. and Meulenberg, W.A. and Bram, M. and Serra, J.M. and Vaßen, R. and Guillon, O.
SEPARATION AND PURIFICATION TECHNOLOGY. Volume: 219 (2019) - 2019 • 5475
Toward a Paradigm Shift in Electrocatalysis Using Complex Solid Solution Nanoparticles
Löffler, T. and Savan, A. and Garzón-Manjón, A. and Meischein, M. and Scheu, C. and Ludwig, Al. and Schuhmann, W.
ACS ENERGY LETTERS. Volume: 4 (2019) - 2019 • 5474
Role of surface oxide layers in the hydrogen embrittlement of austenitic stainless steels: A TOF-SIMS study
Izawa, C. and Wagner, S. and Deutges, M. and Martín, M. and Weber, S. and Pargeter, R. and Michler, T. and Uchida, H.-H. and Gemma, R. and Pundt, A.
ACTA MATERIALIA. Volume: 180 (2019) - 2019 • 5473
Single entity electrochemistry for the elucidation of lithiation kinetics of TiO2 particles in non-aqueous batteries
Löffler, T. and Clausmeyer, J. and Wilde, P. and Tschulik, K. and Schuhmann, W. and Ventosa, E.
NANO ENERGY. Volume: 57 (2019) - 2019 • 5472
Correlation between sputter deposition parameters and I-V characteristics in double-barrier memristive devices
Zahari, F. and Schlichting, F. and Strobel, J. and Dirkmann, S. and Cipo, J. and Gauter, S. and Trieschmann, J. and Marquardt, R. and Haberfehlner, G. and Kothleitner, G. and Kienle, L. and Mussenbrock, T. and Ziegler, M. and Kersten, H. and Kohlstedt, H.
JOURNAL OF VACUUM SCIENCE AND TECHNOLOGY B: NANOTECHNOLOGY AND MICROELECTRONICS. Volume: 37 (2019) - 2019 • 5471
Relationship between hydrogen embrittlement and Md30 temperature: Prediction of low-nickel austenitic stainless steel's resistance
Izawa, C. and Wagner, S. and Deutges, M. and Martín, M. and Weber, S. and Pargeter, R. and Michler, T. and Uchida, H.-H. and Gemma, R. and Pundt, A.
INTERNATIONAL JOURNAL OF HYDROGEN ENERGY. Volume: 44 (2019) - 2019 • 5470
Single entity electrochemistry for the elucidation of lithiation kinetics of TiO 2 particles in non-aqueous batteries
Löffler, T. and Clausmeyer, J. and Wilde, P. and Tschulik, K. and Schuhmann, W. and Ventosa, E.
NANO ENERGY. Volume: 57 (2019) - 2019 • 5469
Predicting grain boundary structure and energy in BCC metals by integrated atomistic and phase-field modeling
Qiu, D. and Zhao, P. and Shen, C. and Lu, W. and Zhang, D. and Mrovec, M. and Wang, Y.
ACTA MATERIALIA. Volume: 164 (2019) - 2019 • 5468
Thermo-mechanical response of FG tungsten/EUROFER multilayer under high thermal loads
Qu, D.D. and Wirtz, M. and Linke, J. and Vaßen, R. and Aktaa, J.
JOURNAL OF NUCLEAR MATERIALS. Volume: 519 (2019) - 2019 • 5467
Loss of Specific Active-Site Iron Atoms in Oxygen-Exposed [FeFe]-Hydrogenase Determined by Detailed X-ray Structure Analyses
Esselborn, J. and Kertess, L. and Apfel, U.-P. and Hofmann, E. and Happe, T.
JOURNAL OF THE AMERICAN CHEMICAL SOCIETY. Volume: 141 (2019) - 2019 • 5466
Quasi-Fermi-Level Splitting of Cu -Poor and Cu -Rich CuIn S2 Absorber Layers
Lomuscio, A. and Rödel, T. and Schwarz, T. and Gault, B. and Melchiorre, M. and Raabe, D. and Siebentritt, S.
PHYSICAL REVIEW APPLIED. Volume: 11 (2019) - 2019 • 5465
Simulation of Quasi-One-Dimensional Wigner Solid Melting in a Parabolic Confinement
Zakharov, M.Y. and Beysengulov, N.R. and Lysogorskiy, Y. and Tayurskii, D.A.
JETP LETTERS. Volume: 110 (2019) - 2019 • 5464
Additive manufacturing of CMSX-4 Ni-base superalloy by selective laser melting: Influence of processing parameters and heat treatment
Lopez-Galilea, I. and Ruttert, B. and He, J. and Hammerschmidt, T. and Drautz, R. and Gault, B. and Theisen, W.
ADDITIVE MANUFACTURING. Volume: 30 (2019) - 2019 • 5463
Structure and Dynamics of the Liquid-Water/Zinc-Oxide Interface from Machine Learning Potential Simulations
Quaranta, V. and Behler, J. and Hellström, M.
JOURNAL OF PHYSICAL CHEMISTRY C. Volume: 123 (2019) - 2019 • 5462
Role of gallium and yttrium dopants on the stability and performance of solution processed indium oxide thin-film transistors
Jaehnike, F. and Pham, D.V. and Bock, C. and Kunze, U.
JOURNAL OF MATERIALS CHEMISTRY C. Volume: 7 (2019) - 2019 • 5461
Validation of a Terminally Amino Functionalized Tetra-Alkyl Sn(IV) Precursor in Metal–Organic Chemical Vapor Deposition of SnO2 Thin Films: Study of Film Growth Characteristics, Optical, and Electrical Properties
Zanders, D. and Ciftyurek, E. and Hoppe, C. and de los Arcos, T. and Kostka, A. and Rogalla, D. and Grundmeier, G. and Schierbaum, K.D. and Devi, A.
ADVANCED MATERIALS INTERFACES. Volume: 6 (2019) - 2019 • 5460
Quadratically Regularized Optimal Transport
Lorenz, D.A. and Manns, P. and Meyer, C.
APPLIED MATHEMATICS AND OPTIMIZATION. Volume: (2019) - 2019 • 5459
PEALD of HfO2 Thin Films: Precursor Tuning and a New Near-Ambient-Pressure XPS Approach to in Situ Examination of Thin-Film Surfaces Exposed to Reactive Gases
Zanders, D. and Ciftyurek, E. and Subaşl, E. and Huster, N. and Bock, C. and Kostka, A. and Rogalla, D. and Schierbaum, K. and Devi, A.
ACS APPLIED MATERIALS AND INTERFACES. Volume: 11 (2019) - 2019 • 5458
Assessing negative thermal expansion in mesoporous metal-organic frameworks by molecular simulation
Evans, J.D. and Dürholt, J.P. and Kaskel, S. and Schmid, R.
JOURNAL OF MATERIALS CHEMISTRY A. Volume: 7 (2019) - 2019 • 5457
Material defects localization in concrete plate-like structures – Experimental and numerical study
Stojić, D. and Nestorović, T. and Marković, N. and Cvetković, R.
MECHANICS RESEARCH COMMUNICATIONS. Volume: 98 (2019) - 2019 • 5456
Strain relaxation behaviour of vortices in a multiferroic superconductor
Evans, D.M. and Schiemer, J.A. and Wolf, T. and Adelmann, P. and Böhmer, A.E. and Meingast, C. and Dutton, S.E. and Mukherjee, P. and Hsu, Y.-T. and Carpenter, M.A.
JOURNAL OF PHYSICS CONDENSED MATTER. Volume: 31 (2019) - 2019 • 5455
Tuning the Properties of Iron-Doped Porous Graphitic Carbon Synthesized by Hydrothermal Carbonization of Cellulose and Subsequent Pyrolysis
Lotz, K. and Wütscher, A. and Düdder, H. and Berger, C.M. and Russo, C. and Mukherjee, K. and Schwaab, G. and Havenith, M. and Muhler, M.
ACS OMEGA. Volume: 4 (2019) - 2019 • 5454
Strategies for improving the sustainability of structural metals
Raabe, D. and Tasan, C.C. and Olivetti, E.A.
NATURE. Volume: 575 (2019) - 2019 • 5453
Piece by Piece—Electrochemical Synthesis of Individual Nanoparticles and their Performance in ORR Electrocatalysis
Evers, M.V. and Bernal, M. and Roldan Cuenya, B. and Tschulik, K.
ANGEWANDTE CHEMIE - INTERNATIONAL EDITION. Volume: 58 (2019) - 2019 • 5452
Catalytic effect of iron phases on the oxidation of cellulose-derived synthetic char
Lotz, K. and Berger, C.M. and Muhler, M.
ENERGY PROCEDIA. Volume: 158 (2019) - 2019 • 5451
Metastability alloy design
Raabe, D. and Li, Z. and Ponge, D.
MRS BULLETIN. Volume: 44 (2019) - 2019 • 5450
Application of high powered Laser Technology to alter hard rock properties towards lower strength materials for more efficient drilling, mining, and Geothermal Energy production
Jamali, S. and Wittig, V. and Börner, J. and Bracke, R. and Ostendorf, A.
GEOMECHANICS FOR ENERGY AND THE ENVIRONMENT. Volume: (2019) - 2019 • 5449
Controlling DNA/Surface Interactions for Potential Pulse-Assisted Preparation of Multi-Probe DNA Microarrays
Jambrec, D. and Kayran, Y.U. and Schuhmann, W.
ELECTROANALYSIS. Volume: 31 (2019) - 2019 • 5448
Dislocation mechanism based size-dependent crystal plasticity modeling and simulation of gradient nano-grained copper
Lu, X. and Zhang, X. and Shi, M. and Roters, F. and Kang, G. and Raabe, D.
INTERNATIONAL JOURNAL OF PLASTICITY. Volume: 113 (2019) - 2019 • 5447
Recent advances in the preparation of zeolites for the selective catalytic reduction of NOx in diesel engines
Zhang, L. and Wu, Q. and Meng, X. and Müller, U. and Feyen, M. and Dai, D. and Maurer, S. and McGuire, R. and Moini, A. and Parvulescu, A.-N. and Zhang, W. and Shi, C. and Yokoi, T. and Pan, X. and Bao, X. and Gies, H. and Marler, B. and De Vos, D.E. and Kolb, U. and Xiao, F.-S.
REACTION CHEMISTRY AND ENGINEERING. Volume: 4 (2019) - 2019 • 5446
Precipitation Hardenable High Entropy Alloy for Tooling Applications
Stryzhyboroda, O. and Hecht, U. and Witusiewicz, V.T. and Laplanche, G. and Asabre, A. and Wilms, M.B. and Weisheit, A.
MRS ADVANCES. Volume: 4 (2019) - 2019 • 5445
Deformation-driven bidirectional transformation promotes bulk nanostructure formation in a metastable interstitial high entropy alloy
Su, J. and Wu, X. and Raabe, D. and Li, Z.
ACTA MATERIALIA. Volume: 167 (2019) - 2019 • 5444
An Asymmetric Supercapacitor–Diode (CAPode) for Unidirectional Energy Storage
Zhang, E. and Fulik, N. and Hao, G.-P. and Zhang, H.-Y. and Kaneko, K. and Borchardt, L. and Brunner, E. and Kaskel, S.
ANGEWANDTE CHEMIE - INTERNATIONAL EDITION. Volume: 58 (2019) - 2019 • 5443
Discovery of new materials using combinatorial synthesis and high-throughput characterization of thin-film materials libraries combined with computational methods
Ludwig, Al.
NPJ COMPUTATIONAL MATERIALS. Volume: 5 (2019) - 2019 • 5442
Invar effects in FeNiCo medium entropy alloys: From an Invar treasure map to alloy design
Rao, Z. and Ponge, D. and Körmann, F. and Ikeda, Y. and Schneeweiss, O. and Friák, M. and Neugebauer, J. and Raabe, D. and Li, Z.
INTERMETALLICS. Volume: 111 (2019) - 2019 • 5441
Hierarchical microstructure design to tune the mechanical behavior of an interstitial TRIP-TWIP high-entropy alloy
Su, J. and Raabe, D. and Li, Z.
ACTA MATERIALIA. Volume: 163 (2019) - 2019 • 5440
On the origin of mesopore collapse in functionalized porous carbons
Zhang, E. and Casco, M.E. and Xu, F. and Sheng, W.-B. and Oswald, S. and Giebeler, L. and Wegner, K. and Borchardt, L. and Kaskel, S.
CARBON. Volume: 149 (2019) - 2019 • 5439
Top-down fabrication and transformation properties of vanadium dioxide nanostructures
Rastjoo, S. and Wang, X. and Ludwig, Al. and Kohl, M.
JOURNAL OF APPLIED PHYSICS. Volume: 125 (2019) - 2019 • 5438
Ab initio study of the combined effects of alloying elements and h on grain boundary cohesion in ferritic steels
Subramanyam, A.P.A. and Guzmán, A.A. and Vincent, S. and Hartmaier, A. and Janisch, R.
METALS. Volume: 9 (2019) - 2019 • 5437
Ionic liquid - Electrode materials interactions studied by NMR spectroscopy, cyclic voltammetry, and impedance spectroscopy
Zhang, E. and Fulik, N. and Paasch, S. and Borchardt, L. and Kaskel, S. and Brunner, E.
ENERGY STORAGE MATERIALS. Volume: 19 (2019) - 2019 • 5436
pyiron: An integrated development environment for computational materials science
Janssen, J. and Surendralal, S. and Lysogorskiy, Y. and Todorova, M. and Hickel, T. and Drautz, R. and Neugebauer, J.
COMPUTATIONAL MATERIALS SCIENCE. Volume: 163 (2019) - 2019 • 5435
A constitutive model for the sintering of suspension plasma-sprayed thermal barrier coating with vertical cracks
Lv, B. and Mücke, R. and Zhou, D. and Fan, X. and Wang, T.J. and Guillon, O. and Vaßen, R.
JOURNAL OF THE AMERICAN CERAMIC SOCIETY. Volume: 102 (2019) - 2019 • 5434
Dislocation multiplication by cross-slip and glissile reaction in a dislocation based continuum formulation of crystal plasticity
Sudmanns, M. and Stricker, M. and Weygand, D. and Hochrainer, T. and Schulz, K.
JOURNAL OF THE MECHANICS AND PHYSICS OF SOLIDS. Volume: 132 (2019) - 2019 • 5433
Rare-earth ion exchanged Cu-SSZ-13 zeolite from organotemplate-free synthesis with enhanced hydrothermal stability in NH 3 -SCR of NO : X
Zhao, Z. and Yu, R. and Shi, C. and Gies, H. and Xiao, F.-S. and De Vos, D. and Yokoi, T. and Bao, X. and Kolb, U. and McGuire, R. and Parvulescu, A.-N. and Maurer, S. and Müller, U. and Zhang, W.
CATALYSIS SCIENCE AND TECHNOLOGY. Volume: 9 (2019) - 2019 • 5432
Plastic deformation of tungsten due to deuterium plasma exposure: Insights from micro-compression tests
Fang, X. and Rasinski, M. and Kreter, A. and Kirchlechner, C. and Linsmeier, C. and Dehm, G. and Brinckmann, S.
SCRIPTA MATERIALIA. Volume: 162 (2019) - 2019 • 5431
Transferability of interatomic potentials for molybdenum and silicon
Lysogorskiy, Y. and Hammerschmidt, T. and Janssen, J. and Neugebauer, J. and Drautz, R.
MODELLING AND SIMULATION IN MATERIALS SCIENCE AND ENGINEERING. Volume: 27 (2019) - 2019 • 5430
Cl2 Production by Photocatalytic Oxidation of HCl over TiO2
Rath, T. and Uhrich, A. and Lüken, A. and Zhao, G. and Rittermeier, A. and Muhler, M.
CHEMSUSCHEM. Volume: 12 (2019) - 2019 • 5429
Revealing fracture mechanisms of medium manganese steels with and without delta-ferrite
Sun, B. and Palanisamy, D. and Ponge, D. and Gault, B. and Fazeli, F. and Scott, C. and Yue, S. and Raabe, D.
ACTA MATERIALIA. Volume: 164 (2019) - 2019 • 5428
Photocatalytic Oxidation of α-C−H Bonds in Unsaturated Hydrocarbons through a Radical Pathway Induced by a Molecular Cocatalyst
Zhao, G. and Hu, B. and Busser, G.W. and Peng, B. and Muhler, M.
CHEMSUSCHEM. Volume: 12 (2019) - 2019 • 5427
Heterogeneous Interactions between Gas-Phase Pyruvic Acid and Hydroxylated Silica Surfaces: A Combined Experimental and Theoretical Study
Fang, Y. and Lesnicki, D. and Wall, K.J. and Gaigeot, M.-P. and Sulpizi, M. and Vaida, V. and Grassian, V.H.
JOURNAL OF PHYSICAL CHEMISTRY A. Volume: 123 (2019) - 2019 • 5426
Anaerobic Alcohol Conversion to Carbonyl Compounds over Nanoscaled Rh-Doped SrTiO 3 under Visible Light
Zhao, G. and Busser, G.W. and Froese, C. and Hu, B. and Bonke, S.A. and Schnegg, A. and Ai, Y. and Wei, D. and Wang, X. and Peng, B. and Muhler, M.
JOURNAL OF PHYSICAL CHEMISTRY LETTERS. Volume: 10 (2019) - 2019 • 5425
Macroscopic to nanoscopic in situ investigation on yielding mechanisms in ultrafine grained medium Mn steels: Role of the austenite-ferrite interface
Sun, B. and Ma, Y. and Vanderesse, N. and Varanasi, R.S. and Song, W. and Bocher, P. and Ponge, D. and Raabe, D.
ACTA MATERIALIA. Volume: 178 (2019) - 2019 • 5424
Development of Multilayer Sinter Cladding of Cold Work Tool Steel on Hadfield Steel Plates for Wear-Resistant Applications
Farayibi, P.K. and Blüm, M. and Theisen, W. and Weber, S.
JOURNAL OF MATERIALS ENGINEERING AND PERFORMANCE. Volume: 28 (2019) - 2019 • 5423
Thin plasma polymerised coatings for corrosion protection against strong alkaline solutions
Jaritz, M. and Hopmann, C. and Wilski, S. and Kleines, L. and Rudolph, M. and Awakowicz, P. and Dahlmann, R.
SURFACE AND COATINGS TECHNOLOGY. Volume: 374 (2019) - 2019 • 5422
A photosystem i monolayer with anisotropic electron flow enables Z-scheme like photosynthetic water splitting
Zhao, F. and Wang, P. and Ruff, A. and Hartmann, V. and Zacarias, S. and Pereira, I.A.C. and Nowaczyk, M.M. and Rögner, M. and Conzuelo, F. and Schuhmann, W.
ENERGY AND ENVIRONMENTAL SCIENCE. Volume: 12 (2019) - 2019 • 5421
Modelocked diode lasers for THz asynchronous optical sampling
Surkamp, N. and Döpke, B. and Brenner, C. and Orend, K. and Baer, C. and Musch, T. and Prziwarka, T. and Klehr, A. and Knigge, A. and Hofmann, M.R.
PROCEEDINGS OF SPIE - THE INTERNATIONAL SOCIETY FOR OPTICAL ENGINEERING. Volume: 10917 (2019) - 2019 • 5420
A light-driven Nernstian biosupercapacitor
Zhao, F. and Bobrowski, T. and Ruff, A. and Hartmann, V. and Nowaczyk, M.M. and Rögner, M. and Conzuelo, F. and Schuhmann, W.
ELECTROCHIMICA ACTA. Volume: 306 (2019) - 2019 • 5419
Extended Operational Lifetime of a Photosystem-Based Bioelectrode
Zhao, F. and Ruff, A. and Rögner, M. and Schuhmann, W. and Conzuelo, F.
JOURNAL OF THE AMERICAN CHEMICAL SOCIETY. Volume: 141 (2019) - 2019 • 5418
Characterization of layered dielectric materials using ultra-wideband FMCW-radar measurements
Jebramcik, J. and Barowski, J. and Rolfes, I.
ASIA-PACIFIC MICROWAVE CONFERENCE PROCEEDINGS, APMC. Volume: 2018-November (2019) - 2019 • 5417
Influence of phase decomposition on mechanical behavior of an equiatomic CoCuFeMnNi high entropy alloy
MacDonald, B.E. and Fu, Z. and Wang, X. and Li, Z. and Chen, W. and Zhou, Y. and Raabe, D. and Schoenung, J. and Hahn, H. and Lavernia, E.J.
ACTA MATERIALIA. Volume: 181 (2019) - 2019 • 5416
A Novel Calibration Technique for FMCW Radar Systems Enabling Material Characterization in Variable Distances
Jebramcik, J. and Rolfes, I. and Barowski, J.
IMWS-AMP 2019 - 2019 IEEE MTT-S INTERNATIONAL MICROWAVE WORKSHOP SERIES ON ADVANCED MATERIALS AND PROCESSES FOR RF AND THZ APPLICATIONS. Volume: (2019) - 2019 • 5415
Perspective of Surfactant-Free Colloidal Nanoparticles in Heterogeneous Catalysis
Reichenberger, S. and Marzun, G. and Muhler, M. and Barcikowski, S.
CHEMCATCHEM. Volume: 11 (2019) - 2019 • 5414
On-chip nano-electro-mechanical switching of deterministic single photons
Zhou, X. and Papon, C. and Thyrrestrup, H. and Liu, Z. and Stobbe, S. and Schott, R. and Wieck, A.D. and Ludwig, Ar. and Lodahl, P. and Midolo, L.
2019 CONFERENCE ON LASERS AND ELECTRO-OPTICS, CLEO 2019 - PROCEEDINGS. Volume: (2019) - 2019 • 5413
First-principles characterization of reversible martensitic transformations
Ferrari, A. and Sangiovanni, D.G. and Rogal, J. and Drautz, R.
PHYSICAL REVIEW B. Volume: 99 (2019) - 2019 • 5412
Radar Based Material Characterization at 145 GHz Utilizing an Ellipsoidal Reflector
Jebramcik, J. and Barowski, J. and Wagner, J. and Rolfes, I.
2019 49TH EUROPEAN MICROWAVE CONFERENCE, EUMC 2019. Volume: (2019) - 2019 • 5411
Evolution of porosity, crack density, and CMAS penetration in thermal barrier coatings subjected to burner rig testing
Mack, D.E. and Laquai, R. and Müller, B. and Helle, O. and Sebold, D. and Vaßen, R. and Bruno, G.
JOURNAL OF THE AMERICAN CERAMIC SOCIETY. Volume: 102 (2019) - 2019 • 5410
Modeling Macroscopic Material Behavior With Machine Learning Algorithms Trained by Micromechanical Simulations
Reimann, D. and Nidadavolu, K. and ul Hassan, H. and Vajragupta, N. and Glasmachers, T. and Junker, P. and Hartmaier, A.
FRONTIERS IN MATERIALS. Volume: 6 (2019) - 2019 • 5409
Architecture designs for extending thermal cycling lifetime of suspension plasma sprayed thermal barrier coatings
Zhou, D. and Mack, D.E. and Gerald, P. and Guillon, O. and Vaßen, R.
CERAMICS INTERNATIONAL. Volume: 45 (2019) - 2019 • 5408
Discovery of ω -free high-temperature Ti-Ta- X shape memory alloys from first-principles calculations
Ferrari, A. and Paulsen, A. and Langenkämper, D. and Piorunek, D. and Somsen, C. and Frenzel, J. and Rogal, J. and Eggeler, G. and Drautz, R.
PHYSICAL REVIEW MATERIALS. Volume: 3 (2019) - 2019 • 5407
Crowd-sourcing materials-science challenges with the NOMAD 2018 Kaggle competition
Sutton, C. and Ghiringhelli, L.M. and Yamamoto, T. and Lysogorskiy, Y. and Blumenthal, L. and Hammerschmidt, T. and Golebiowski, J.R. and Liu, X. and Ziletti, A. and Scheffler, M.
NPJ COMPUTATIONAL MATERIALS. Volume: 5 (2019) - 2019 • 5406
Sintering behavior of columnar thermal barrier coatings deposited by axial suspension plasma spraying (SPS)
Zhou, D. and Malzbender, J. and Sohn, Y.J. and Guillon, O. and Vaßen, R.
JOURNAL OF THE EUROPEAN CERAMIC SOCIETY. Volume: 39 (2019) - 2019 • 5405
Phase transitions in titanium with an analytic bond-order potential
Ferrari, A. and Schröder, M. and Lysogorskiy, Y. and Rogal, J. and Mrovec, M. and Drautz, R.
MODELLING AND SIMULATION IN MATERIALS SCIENCE AND ENGINEERING. Volume: 27 (2019) - 2019 • 5404
Solute trapping in non-equilibrium solidification: A comparative model study
Reuther, K. and Hubig, S. and Steinbach, I. and Rettenmayr, M.
MATERIALIA. Volume: 6 (2019) - 2019 • 5403
Fabrication of heterostructured p-CuO/n-SnO 2 core-shell nanowires for enhanced sensitive and selective formaldehyde detection
Zhu, L.-Y. and Yuan, K. and Yang, J.-G. and Ma, H.-P. and Wang, T. and Ji, X.-M. and Feng, J.-J. and Devi, A. and Lu, H.-L.
SENSORS AND ACTUATORS, B: CHEMICAL. Volume: (2019) - 2019 • 5402
Reconciling Experimental and Theoretical Data in the Structural Analysis of Ti–Ta Shape-Memory Alloys
Ferrari, A. and Kadletz, P.M. and Chakraborty, T. and Liao, K. and Langenkämper, D. and Motemani, Y. and Paulsen, A. and Lysogorskiy, Y. and Frenzel, J. and Rogal, J. and Ludwig, Al. and Somsen, C. and Drautz, R. and Schmahl, W.W.
SHAPE MEMORY AND SUPERELASTICITY. Volume: 5 (2019) - 2019 • 5401
Plasmon Enhanced Two-Photon Probing with Gold and Silver Nanovoid Structures
Madzharova, F. and Öhl, D. and Junqueira, J. and Schuhmann, W. and Kneipp, J.
ADVANCED OPTICAL MATERIALS. Volume: 7 (2019) - 2019 • 5400
Fabrication of heterostructured p-CuO/n-SnO2 core-shell nanowires for enhanced sensitive and selective formaldehyde detection
Zhu, L.-Y. and Yuan, K. and Yang, J.-G. and Ma, H.-P. and Wang, T. and Ji, X.-M. and Feng, J.-J. and Devi, A. and Lu, H.-L.
SENSORS AND ACTUATORS, B: CHEMICAL. Volume: 290 (2019) - 2019 • 5399
Optimization with constraints considering polymorphic uncertainties
Mäck, M. and Caylak, I. and Edler, P. and Freitag, S. and Hanss, M. and Mahnken, R. and Meschke, G. and Penner, E.
GAMM MITTEILUNGEN. Volume: 42 (2019) - 2019 • 5398
Emergence and impact of Al2TiO5 in Al2O3-TiO2 APS coatings
Richter, A. and Berger, L.-M. and Conze, S. and Sohn, Y.J. and Vaßen, R.
IOP CONFERENCE SERIES: MATERIALS SCIENCE AND ENGINEERING. Volume: 480 (2019) - 2019 • 5397
Ballistic rectification based on inhomogeneous magnetic stray fields
Szelong, M. and Ludwig, Ar. and Wieck, A.D. and Kunze, U.
JOURNAL OF APPLIED PHYSICS. Volume: 125 (2019) - 2019 • 5396
Influence of spore size distribution, gas mixture, and process time on the removal rate of B. subtilis spores in low-pressure plasmas
Fiebrandt, M. and Roggendorf, J. and Moeller, R. and Awakowicz, P.
JOURNAL OF PHYSICS D: APPLIED PHYSICS. Volume: 52 (2019) - 2019 • 5395
Tailored microstructures of gadolinium zirconate/YSZ multi-layered thermal barrier coatings produced by suspension plasma spray: Durability and erosion testing
Mahade, S. and Zhou, D. and Curry, N. and Markocsan, N. and Nylén, P. and Vaßen, R.
JOURNAL OF MATERIALS PROCESSING TECHNOLOGY. Volume: 264 (2019) - 2019 • 5394
Impact of Al2O3-40 wt.% TiO2 feedstock powder characteristics on the sprayability, microstructure and mechanical properties of plasma sprayed coatings
Richter, A. and Berger, L.-M. and Sohn, Y.J. and Conze, S. and Sempf, K. and Vaßen, R.
JOURNAL OF THE EUROPEAN CERAMIC SOCIETY. Volume: 39 (2019) - 2019 • 5393
Sound-driven single-electron transfer in a circuit of coupled quantum rails
Takada, S. and Edlbauer, H. and Lepage, H.V. and Wang, J. and Mortemousque, P.-A. and Georgiou, G. and Barnes, C.H.W. and Ford, C.J.B. and Yuan, M. and Santos, P.V. and Waintal, X. and Ludwig, Ar. and Wieck, A.D. and Urdampilleta, M. and Meunier, T. and Bäuerle, C.
NATURE COMMUNICATIONS. Volume: 10 (2019) - 2019 • 5392
Multi-scale characterization of austenite reversion and martensite recovery in a cold-rolled medium-Mn steel
Benzing, J.T. and Kwiatkowski da Silva, A. and Morsdorf, L. and Bentley, J. and Ponge, D. and Dutta, A. and Han, J. and McBride, J.R. and Van Leer, B. and Gault, B. and Raabe, D. and Wittig, J.E.
ACTA MATERIALIA. Volume: 166 (2019) - 2019 • 5391
Low-Temperature Plasma-Enhanced Atomic Layer Deposition of Tin(IV) Oxide from a Functionalized Alkyl Precursor: Fabrication and Evaluation of SnO2-Based Thin-Film Transistor Devices
Mai, L. and Zanders, D. and Subaşl, E. and Ciftyurek, E. and Hoppe, C. and Rogalla, D. and Gilbert, W. and Arcos, T.D.L. and Schierbaum, K. and Grundmeier, G. and Bock, C. and Devi, A.
ACS APPLIED MATERIALS AND INTERFACES. Volume: (2019) - 2019 • 5390
Carbon and strain partitioning in a quenched and partitioned steel containing ferrite
Tan, X. and Ponge, D. and Lu, W. and Xu, Y. and Yang, X. and Rao, X. and Wu, D. and Raabe, D.
ACTA MATERIALIA. Volume: 165 (2019) - 2019 • 5389
Experimental and numerical study of mechanical properties of multi-phase medium-Mn TWIP-TRIP steel: Influences of strain rate and phase constituents
Benzing, J.T. and Liu, Y. and Zhang, X. and Luecke, W.E. and Ponge, D. and Dutta, A. and Oskay, C. and Raabe, D. and Wittig, J.E.
ACTA MATERIALIA. Volume: 177 (2019) - 2019 • 5388
Potential Precursor Alternatives to the Pyrophoric Trimethylaluminium for the Atomic Layer Deposition of Aluminium Oxide
Mai, L. and Boysen, N. and Zanders, D. and de los Arcos, T. and Mitschker, F. and Mallick, B. and Grundmeier, G. and Awakowicz, P. and Devi, A.
CHEMISTRY - A EUROPEAN JOURNAL. Volume: 25 (2019) - 2019 • 5387
A Z-Scheme-Inspired Photobioelectrochemical H2O/O2 Cell with a 1 V Open-Circuit Voltage Combining Photosystem II and PbS Quantum Dots
Riedel, M. and Wersig, J. and Ruff, A. and Schuhmann, W. and Zouni, A. and Lisdat, F.
ANGEWANDTE CHEMIE - INTERNATIONAL EDITION. Volume: 58 (2019) - 2019 • 5386
Glancing-angle deposition of nanostructures on an implant material surface
Ziegler, N. and Sengstock, C. and Mai, V. and Schildhauer, T.A. and Köller, M. and Ludwig, Al.
NANOMATERIALS. Volume: 9 (2019) - 2019 • 5385
Real-time multitarget tracking for sensor-based sorting: A new implementation of the auction algorithm for graphics processing units
Maier, G. and Pfaff, F. and Wagner, M. and Pieper, C. and Gruna, R. and Noack, B. and Kruggel-Emden, H. and Längle, T. and Hanebeck, U.D. and Wirtz, S. and Scherer, V. and Beyerer, J.
JOURNAL OF REAL-TIME IMAGE PROCESSING. Volume: 16 (2019) - 2019 • 5384
A Z-Scheme-Inspired Photobioelectrochemical H 2 O/O 2 Cell with a 1 V Open-Circuit Voltage Combining Photosystem II and PbS Quantum Dots
Riedel, M. and Wersig, J. and Ruff, A. and Schuhmann, W. and Zouni, A. and Lisdat, F.
ANGEWANDTE CHEMIE - INTERNATIONAL EDITION. Volume: 58 (2019) - 2019 • 5383
The BBQ-Guideline of DAfStb - Best Practice for the quality chain in concrete construction [Die BBQ-Richtlinie des DAfStb: Best Practice für die Qualitätskette im Betonbau]
Fingerloos, F. and Meyer, L. and Breitenbücher, R.
BETON- UND STAHLBETONBAU. Volume: 114 (2019) - 2019 • 5382
Scanning Electrochemical Cell Microscopy Investigation of Single ZIF-Derived Nanocomposite Particles as Electrocatalysts for Oxygen Evolution in Alkaline Media
Tarnev, T. and Aiyappa, H.B. and Botz, A. and Erichsen, T. and Ernst, A. and Andronescu, C. and Schuhmann, W.
ANGEWANDTE CHEMIE - INTERNATIONAL EDITION. Volume: 58 (2019) - 2019 • 5381
Encapsulation of sub-micrometer sized zeolites by porous silica – towards a rational design strategy for functional yolk-shells
Joshi, H. and Schmidt, W. and Schüth, F.
MICROPOROUS AND MESOPOROUS MATERIALS. Volume: (2019) - 2019 • 5380
Ion energy control via the electrical asymmetry effect to tune coating properties in reactive radio frequency sputtering
Ries, S. and Banko, L. and Hans, M. and Primetzhofer, D. and Schneider, J.M. and Ludwig, Al. and Awakowicz, P. and Schulze, J.
PLASMA SOURCES SCIENCE AND TECHNOLOGY. Volume: 28 (2019) - 2019 • 5379
Non-monotonic effect of additive particle size on the glass transition in polymers
Zirdehi, E.M. and Varnik, F.
JOURNAL OF CHEMICAL PHYSICS. Volume: 150 (2019) - 2019 • 5378
Anomalously Low Barrier for Water Dimer Diffusion on Cu(111)
Bertram, C. and Fang, W. and Pedevilla, P. and Michaelides, A. and Morgenstern, K.
NANO LETTERS. Volume: 19 (2019) - 2019 • 5377
Amperometric Detection of the Urinary Disease Biomarker p-HPA by Allosteric Modulation of a Redox Polymer-Embedded Bacterial Reductase
Teanphonkrang, S. and Ernst, A. and Janke, S. and Chaiyen, P. and Sucharitakul, J. and Suginta, W. and Khunkaewla, P. and Schuhmann, W. and Schulte, A. and Ruff, A.
ACS SENSORS. Volume: 4 (2019) - 2019 • 5376
Optimized parallel simulations of analytic bond-order potentials on hybrid shared/distributed memory with MPI and OpenMP
Teijeiro, C. and Hammerschmidt, T. and Drautz, R. and Sutmann, G.
INTERNATIONAL JOURNAL OF HIGH PERFORMANCE COMPUTING APPLICATIONS. Volume: 33 (2019) - 2019 • 5375
The Key Role of Water Activity for the Operating Behavior and Dynamics of Oxygen Depolarized Cathodes
Röhe, M. and Botz, A. and Franzen, D. and Kubannek, F. and Ellendorff, B. and Öhl, D. and Schuhmann, W. and Turek, T. and Krewer, U.
CHEMELECTROCHEM. Volume: 6 (2019) - 2019 • 5374
Simultaneous Anodic Adsorptive Stripping Voltammetric Determination of Luteolin and 3-Hydroxyflavone in Biological Fluids Using Renewable Pencil Graphite Electrodes
Temerk, Y. and Ibrahim, H. and Schuhmann, W.
ELECTROANALYSIS. Volume: 31 (2019) - 2019 • 5373
Digital holographic microscopy for sub-µm scale high aspect ratio structures in transparent materials
Besaga, V.R. and Saetchnikov, A.V. and Gerhardt, N.C. and Ostendorf, A. and Hofmann, M.R.
OPTICS AND LASERS IN ENGINEERING. Volume: 121 (2019) - 2019 • 5372
Knight Shift and Leading Superconducting Instability from Spin Fluctuations in Sr2RuO4
Rømer, A.T. and Scherer, D.D. and Eremin, I.M. and Hirschfeld, P.J. and Andersen, B.M.
PHYSICAL REVIEW LETTERS. Volume: 123 (2019) - 2019 • 5371
Monitoring of photochemically induced changes in phase-modulating samples with digital holographic microscopy
Besaga, V.R. and Saetchnikov, A.V. and Gerhardt, N.C. and Ostendorf, A. and Hofmann, M.R.
APPLIED OPTICS. Volume: 58 (2019) - 2019 • 5370
A fast and robust numerical treatment of a gradient-enhanced model for brittle damage
Junker, P. and Schwarz, S. and Jantos, D.R. and Hackl, K.
INTERNATIONAL JOURNAL FOR MULTISCALE COMPUTATIONAL ENGINEERING. Volume: 17 (2019) - 2019 • 5369
Time-Dependent Evolution of Microstructure and Mechanical Properties of Mortar
Röttger, A. and Youn-Čale, B.-Y. and Küpferle, J. and Lentz, J. and Breitenbücher, R. and Theisen, W.
INTERNATIONAL JOURNAL OF CIVIL ENGINEERING. Volume: 17 (2019) - 2019 • 5368
A machine learning approach for automated fine-tuning of semiconductor spin qubits
Teske, J.D. and Humpohl, S.S. and Otten, R. and Bethke, P. and Cerfontaine, P. and Dedden, J. and Ludwig, Ar. and Wieck, A.D. and Bluhm, H.
APPLIED PHYSICS LETTERS. Volume: 114 (2019) - 2019 • 5367
Tuning Light-Driven Water Splitting Efficiency of Mo-Doped BiVO4: Optimised Preparation and Impact of Oxygen Evolution Electrocatalysts
Junqueira, J.R.C. and Bobrowski, T. and Krysiak, O.A. and Gutkowski, R. and Schuhmann, W.
CHEMCATCHEM. Volume: 11 (2019) - 2019 • 5366
Synthesis and mechanical testing of grain boundaries at the micro and sub-micro scale
Malyar, N.V. and Springer, H. and Wichert, J. and Dehm, G. and Kirchlechner, C.
MATERIALPRUEFUNG/MATERIALS TESTING. Volume: 61 (2019) - 2019 • 5365
Neural-Network-Based Path Collective Variables for Enhanced Sampling of Phase Transformations
Rogal, J. and Schneider, E. and Tuckerman, M.E.
PHYSICAL REVIEW LETTERS. Volume: 123 (2019) - 2019 • 5364
FexNi9-xS8 (x = 3-6) as potential photocatalysts for solar-driven hydrogen production?
Tetzlaff, D. and Simon, C. and Achilleos, D.S. and Smialkowski, M. and Junge Puring, K. and Bloesser, A. and Piontek, S. and Kasap, H. and Siegmund, D. and Reisner, E. and Marschall, R. and Apfel, U.-P.
FARADAY DISCUSSIONS. Volume: 215 (2019) - 2019 • 5363
Biomimetic structural coloration with tunable degree of angle-independence generated by two-photon polymerization
Zyla, G. and Kovalev, A. and Heisterkamp, S. and Esen, C. and Gurevich, E.L. and Gorb, S. and Ostendorf, A.
OPTICAL MATERIALS EXPRESS. Volume: 9 (2019) - 2019 • 5362
Near real-time digital holographic imaging on conventional central processing unit
Besaga, V.R. and Saetchnikov, A.V. and Gerhardt, N.C. and Ostendorf, A. and Hofmann, M.R.
PROCEEDINGS OF SPIE - THE INTERNATIONAL SOCIETY FOR OPTICAL ENGINEERING. Volume: 11056 (2019) - 2019 • 5361
Tailoring quasi-two-dimensional high conductivity and superconductivity areas at the interfaces of ferroelectric/dielectric heterostructures
Mamin, R.F. and Pavlov, D.P. and Piyanzina, I.I. and Lysogorskiy, Y.V. and Mukhortov, V.M. and Adachi, T. and Kawamata, T. and Koike, Y. and Balbashev, A.M. and Tayurskii, D.A. and Kabanov, V.V.
IOP CONFERENCE SERIES: MATERIALS SCIENCE AND ENGINEERING. Volume: 699 (2019) - 2019 • 5360
Critical evaluation of the material characteristics and environmental potential of laser beam melting processes for the additive manufacturing of metallic components
Teubler, J. and Weber, S. and Suski, P. and Peschke, I. and Liedtke, C.
JOURNAL OF CLEANER PRODUCTION. Volume: 237 (2019) - 2019 • 5359
Lead diffusion in CaTiO3: A combined study using Rutherford backscattering and TOF-SIMS for depth profiling to reveal the role of lattice strain in diffusion processes
Beyer, C. and Dohmen, R. and Rogalla, D. and Becker, H.-W. and Marquardt, K. and Vollmer, C. and Hagemann, U. and Hartmann, N. and Chakraborty, S.
AMERICAN MINERALOGIST. Volume: 104 (2019) - 2019 • 5358
Discovery of Elusive K4O6, a Compound Stabilized by Configurational Entropy of Polarons
Freysoldt, C. and Merz, P. and Schmidt, M. and Mohitkar, S. and Felser, C. and Neugebauer, J. and Jansen, M.
ANGEWANDTE CHEMIE - INTERNATIONAL EDITION. Volume: 58 (2019) - 2019 • 5357
Elasticity of phases in Fe-Al-Ti superalloys: Impact of atomic order and anti-phase boundaries
Friák, M. and Buršíková, V. and Pizúrová, N. and Pavlů, J. and Jirásková, Y. and Homola, V. and Miháliková, I. and Slávik, A. and Holec, D. and Všianská, M. and Koutná, N. and Fikar, J. and Janičkovič, D. and Šob, M. and Neugebauer, J.
CRYSTALS. Volume: 9 (2019) - 2019 • 5356
PS-PVD Processing of Single-Phase Lanthanum Tungstate Layers for Hydrogen-Related Applications
Marcano, D. and Ivanova, M.E. and Mauer, G. and Sohn, Y.J. and Schwedt, A. and Bram, M. and Menzler, N.H. and Vaßen, R.
JOURNAL OF THERMAL SPRAY TECHNOLOGY. Volume: 28 (2019) - 2019 • 5355
An insight into using DFT data for Calphad modeling of solid phases in the third generation of Calphad databases, a case study for Al
Bigdeli, S. and Zhu, L.-F. and Glensk, A. and Grabowski, B. and Lindahl, B. and Hickel, T. and Selleby, M.
CALPHAD: COMPUTER COUPLING OF PHASE DIAGRAMS AND THERMOCHEMISTRY. Volume: 65 (2019) - 2019 • 5354
Microscopic Determination of Carrier Density and Mobility in Working Organic Electrochemical Transistors
Mariani, F. and Conzuelo, F. and Cramer, T. and Gualandi, I. and Possanzini, L. and Tessarolo, M. and Fraboni, B. and Schuhmann, W. and Scavetta, E.
SMALL. Volume: 15 (2019) - 2019 • 5353
DAMASK – The Düsseldorf Advanced Material Simulation Kit for modeling multi-physics crystal plasticity, thermal, and damage phenomena from the single crystal up to the component scale
Roters, F. and Diehl, M. and Shanthraj, P. and Eisenlohr, P. and Reuber, C. and Wong, S.L. and Maiti, T. and Ebrahimi, A. and Hochrainer, T. and Fabritius, H.-O. and Nikolov, S. and Friák, M. and Fujita, N. and Grilli, N. and Janssens, K.G.F. and Jia, N. and Kok, P.J.J. and Ma, D. and Meier, F. and Werner, E. and Stricker, M. and Weygand, D. and Raabe, D.
COMPUTATIONAL MATERIALS SCIENCE. Volume: 158 (2019) - 2019 • 5352
Methane to Chloromethane by Mechanochemical Activation: A Selective Radical Pathway
Bilke, M. and Losch, P. and Vozniuk, O. and Bodach, A. and Schüth, F.
JOURNAL OF THE AMERICAN CHEMICAL SOCIETY. Volume: 141 (2019) - 2019 • 5351
Repair of Ni-based single-crystal superalloys using vacuum plasma spray
Kalfhaus, T. and Schneider, M. and Ruttert, B. and Sebold, D. and Hammerschmidt, T. and Frenzel, J. and Drautz, R. and Theisen, W. and Eggeler, G. and Guillon, O. and Vassen, R.
MATERIALS AND DESIGN. Volume: 168 (2019) - 2019 • 5350
Ni-base superalloy single crystal (SX) mosaicity characterized by the Rotation Vector Base Line Electron Back Scatter Diffraction (RVB-EBSD) method
Thome, P. and Medghalchi, S. and Frenzel, J. and Schreuer, J. and Eggeler, G.
ULTRAMICROSCOPY. Volume: 206 (2019) - 2019 • 5349
Soil-building interaction in mechanized tunneling: A comparison of modeling approaches
Marwan, A. and Alsahly, A. and Obel, M. and Mark, P. and Meschke, G.
TUNNELS AND UNDERGROUND CITIES: ENGINEERING AND INNOVATION MEET ARCHAEOLOGY, ARCHITECTURE AND ART- PROCEEDINGS OF THE WTC 2019 ITA-AITES WORLD TUNNEL CONGRESS. Volume: (2019) - 2019 • 5348
Angular momentum transfer from photon polarization to an electron spin in a gate-defined quantum dot
Fujita, T. and Morimoto, K. and Kiyama, H. and Allison, G. and Larsson, M. and Ludwig, Ar. and Valentin, S.R. and Wieck, A.D. and Oiwa, A. and Tarucha, S.
NATURE COMMUNICATIONS. Volume: 10 (2019) - 2019 • 5347
On the Theory of Electrolytic Dissociation, the Greenhouse Effect, and Activation Energy in (Electro)Catalysis: A Tribute to Svante Augustus Arrhenius
Masa, J. and Barwe, S. and Andronescu, C. and Schuhmann, W.
CHEMISTRY - A EUROPEAN JOURNAL. Volume: 25 (2019) - 2019 • 5346
Polymer-Bound DuBois-Type Molecular H 2 Oxidation Ni Catalysts Are Protected by Redox Polymer Matrices
Ruff, A. and Janke, S. and Szczesny, J. and Alsaoub, S. and Ruff, I. and Lubitz, W. and Schuhmann, W.
ACS APPLIED ENERGY MATERIALS. Volume: 2 (2019) - 2019 • 5345
The through-process texture analysis of plate rolling by coupling finite element and fast Fourier transform crystal plasticity analysis
Fujita, N. and Igi, S. and Diehl, M. and Roters, F. and Raabe, D.
MODELLING AND SIMULATION IN MATERIALS SCIENCE AND ENGINEERING. Volume: 27 (2019) - 2019 • 5344
Role of Boron and Phosphorus in Enhanced Electrocatalytic Oxygen Evolution by Nickel Borides and Nickel Phosphides
Masa, J. and Andronescu, C. and Antoni, H. and Sinev, I. and Seisel, S. and Elumeeva, K. and Barwe, S. and Marti-Sanchez, S. and Arbiol, J. and Roldan Cuenya, B. and Muhler, M. and Schuhmann, W.
CHEMELECTROCHEM. Volume: 6 (2019) - 2019 • 5343
Polymer-Bound DuBois-Type Molecular H2 Oxidation Ni Catalysts Are Protected by Redox Polymer Matrices
Ruff, A. and Janke, S. and Szczesny, J. and Alsaoub, S. and Ruff, I. and Lubitz, W. and Schuhmann, W.
ACS APPLIED ENERGY MATERIALS. Volume: 2 (2019) - 2019 • 5342
Temperature and load-ratio dependent fatigue-crack growth in the CrMnFeCoNi high-entropy alloy
Thurston, K.V.S. and Gludovatz, B. and Yu, Q. and Laplanche, G. and George, E.P. and Ritchie, R.O.
JOURNAL OF ALLOYS AND COMPOUNDS. Volume: 794 (2019) - 2019 • 5341
Irradiation Effects on Induced Electron Conductivity in an un-doped GaAs/AlGaAs Quantum Well Hall Bar
Fujita, T. and Hayashi, R. and Kohda, M. and Ritzmann, J. and Ludwig, Ar. and Nitta, J. and Wieck, A.D. and Oiwa, A.
2019 COMPOUND SEMICONDUCTOR WEEK, CSW 2019 - PROCEEDINGS. Volume: (2019) - 2019 • 5340
The Role of Non-Metallic and Metalloid Elements on the Electrocatalytic Activity of Cobalt and Nickel Catalysts for the Oxygen Evolution Reaction
Masa, J. and Schuhmann, W.
CHEMCATCHEM. Volume: 11 (2019) - 2019 • 5339
On the onset of deformation twinning in the CrFeMnCoNi high-entropy alloy using a novel tensile specimen geometry
Thurston, K.V.S. and Hohenwarter, A. and Laplanche, G. and George, E.P. and Gludovatz, B. and Ritchie, R.O.
INTERMETALLICS. Volume: 110 (2019) - 2019 • 5338
Ni-Metalloid (B, Si, P, As, and Te) Alloys as Water Oxidation Electrocatalysts
Masa, J. and Piontek, S. and Wilde, P. and Antoni, H. and Eckhard, T. and Chen, Y.-T. and Muhler, M. and Apfel, U.-P. and Schuhmann, W.
ADVANCED ENERGY MATERIALS. Volume: 9 (2019) - 2019 • 5337
Influence of Microstructural Features on the Strain Hardening Behavior of Additively Manufactured Metallic Components
Biswas, A. and Prasad, M.R.G. and Vajragupta, N. and ul Hassan, H. and Brenne, F. and Niendorf, T. and Hartmaier, A.
ADVANCED ENGINEERING MATERIALS. Volume: 21 (2019) - 2019 • 5336
Priming effects in the crystallization of the phase change compound GeTe from atomistic simulations
Gabardi, S. and Sosso, G.G. and Behler, J. and Bernasconi, M.
FARADAY DISCUSSIONS. Volume: 213 (2019) - 2019 • 5335
Pump-probe microscopy of femtosecond laser ablation in air and liquids
Kanitz, A. and Förster, D.J. and Hoppius, J.S. and Weber, R. and Ostendorf, A. and Gurevich, E.L.
APPLIED SURFACE SCIENCE. Volume: 475 (2019) - 2019 • 5334
A neutron diffraction demonstration of long-range magnetic order in the quasicrystal approximant DyCd6
Ryan, D.H. and Cadogan, J.M. and Kong, T. and Canfield, P.C. and Goldman, A.I. and Kreyssig, A.
AIP ADVANCES. Volume: 9 (2019) - 2019 • 5333
The kinetics of glycerol hydrodeoxygenation to 1,2-propanediol over Cu/ZrO 2 in the aqueous phase
Gabrysch, T. and Muhler, M. and Peng, B.
APPLIED CATALYSIS A: GENERAL. Volume: (2019) - 2019 • 5332
Review on experimental and theoretical investigations of the early stage, femtoseconds to microseconds processes during laser ablation in liquid-phase for the synthesis of colloidal nanoparticles
Kanitz, A. and Kalus, M.-R. and Gurevich, E.L. and Ostendorf, A. and Barcikowski, S. and Amans, D.
PLASMA SOURCES SCIENCE AND TECHNOLOGY. Volume: 28 (2019) - 2019 • 5331
The kinetics of glycerol hydrodeoxygenation to 1,2-propanediol over Cu/ZrO2 in the aqueous phase
Gabrysch, T. and Muhler, M. and Peng, B.
APPLIED CATALYSIS A: GENERAL. Volume: 576 (2019) - 2019 • 5330
Active learning for accurate settlement prediction using numerical simulations in mechanized tunneling
Saadallah, A. and Egorov, A. and Cao, B.-T. and Freitag, S. and Morik, K. and Meschke, G.
PROCEDIA CIRP. Volume: 81 (2019) - 2019 • 5329
Concentration-dependent atomic mobilities in FCC CoCrFeMnNi high-entropy alloys
Gaertner, D. and Abrahams, K. and Kottke, J. and Esin, V.A. and Steinbach, I. and Wilde, G. and Divinski, S.V.
ACTA MATERIALIA. Volume: 166 (2019) - 2019 • 5328
New flat-punch indentation creep testing approach for characterizing the local creep properties at high temperatures
Matschkal-Amberger, D. and Kolb, M. and Neumeier, S. and Gao, S. and Hartmaier, A. and Durst, K. and Göken, M.
MATERIALS AND DESIGN. Volume: 183 (2019) - 2019 • 5327
Special Collection on Bioelectrochemistry
Matsue, T. and Pingarrón, J. and Schuhmann, W.
CHEMELECTROCHEM. Volume: 6 (2019) - 2019 • 5326
Cr 2 AlC MAX phase foams by replica method
Karimi, S. and Go, T. and Vaßen, R. and Gonzalez-Julian, J.
MATERIALS LETTERS. Volume: 240 (2019) - 2019 • 5325
On the effects of microstructure on the mechanical properties of open-pore Al–11Zn foams
Matz, A.M. and Matz, B.S. and Parsa, A.B. and Jost, N. and Eggeler, G.
MATERIALS SCIENCE AND ENGINEERING A. Volume: 759 (2019) - 2019 • 5324
Degradation of iridium oxides via oxygen evolution from the lattice: Correlating atomic scale structure with reaction mechanisms
Kasian, O. and Geiger, S. and Li, T. and Grote, J.-P. and Schweinar, K. and Zhang, S. and Scheu, C. and Raabe, D. and Cherevko, S. and Gault, B. and Mayrhofer, K.J.J.
ENERGY AND ENVIRONMENTAL SCIENCE. Volume: 12 (2019) - 2019 • 5323
Reusable dispersed resonators-based biochemical sensor for parallel probing
Saetchnikov, A.V. and Tcherniavskaia, E.A. and Skakun, V.V. and Saetchnikov, V.A. and Ostendorf, A.
IEEE SENSORS JOURNAL. Volume: 19 (2019) - 2019 • 5322
Testing for independence of large dimensional vectors
Bodnar, T. and Dette, H. and Parolya, N.
ANNALS OF STATISTICS. Volume: 47 (2019) - 2019 • 5321
Femtosecond laser patterning of graphene electrodes for thin-film transistors
Kasischke, M. and Subaşı, E. and Bock, C. and Pham, D.-V. and Gurevich, E.L. and Kunze, U. and Ostendorf, A.
APPLIED SURFACE SCIENCE. Volume: 478 (2019) - 2019 • 5320
Structural reproducibility enhancement of optical resonance arrays produced by two-photon polymerization
Saetchnikov, A. and Saetchnikov, V. and Tcherniavskaia, E. and Ostendorf, A.
2019 CONFERENCE ON LASERS AND ELECTRO-OPTICS EUROPE AND EUROPEAN QUANTUM ELECTRONICS CONFERENCE, CLEO/EUROPE-EQEC 2019. Volume: (2019) - 2019 • 5319
On the numerical modeling of nucleation and growth of microstructurally short cracks in polycrystals under cyclic loading
Boeff, M. and Hassan, H.U. and Hartmaier, A.
JOURNAL OF MATERIALS RESEARCH. Volume: (2019) - 2019 • 5318
Influence of excess volumes induced by Re and W on dislocation motion and creep in ni-base single crystal superalloys: A 3D discrete dislocation dynamics study
Gao, S. and Yang, Z. and Grabowski, M. and Rogal, J. and Drautz, R. and Hartmaier, A.
METALS. Volume: 9 (2019) - 2019 • 5317
Imaging individual solute atoms at crystalline imperfections in metals
Katnagallu, S. and Stephenson, L.T. and Mouton, I. and Freysoldt, C. and Subramanyam, A.P.A. and Jenke, J. and Ladines, A.N. and Neumeier, S. and Hammerschmidt, T. and Drautz, R. and Neugebauer, J. and Vurpillot, F. and Raabe, D. and Gault, B.
NEW JOURNAL OF PHYSICS. Volume: 21 (2019) - 2019 • 5316
Biochemical kinetic parameters evaluation by resonator-based multiplexed sensor
Saetchnikov, A. and Tcherniavskaia, E. and Saetchnikov, V. and Ostendorf, A.
2019 CONFERENCE ON LASERS AND ELECTRO-OPTICS EUROPE AND EUROPEAN QUANTUM ELECTRONICS CONFERENCE, CLEO/EUROPE-EQEC 2019. Volume: (2019) - 2019 • 5315
Strengthening of a CrMnFeCoNi high-entropy alloy by carbide precipitation
Gao, N. and Lu, D.H. and Zhao, Y.Y. and Liu, X.W. and Liu, G.H. and Wu, Y. and Liu, G. and Fan, Z.T. and Lu, Z.P. and George, E.P.
JOURNAL OF ALLOYS AND COMPOUNDS. Volume: (2019) - 2019 • 5314
Modelling of direct laser writing in multilayer absorbing medium
Saetchnikov, A. and Tcherniavskaia, E. and Saetchnikov, V. and Ostendorf, A.
PROCEEDINGS OF SPIE - THE INTERNATIONAL SOCIETY FOR OPTICAL ENGINEERING. Volume: 11057 (2019) - 2019 • 5313
Distinct pressure evolution of coupled nematic and magnetic orders in FeSe
Böhmer, A.E. and Kothapalli, K. and Jayasekara, W.T. and Wilde, J.M. and Li, B. and Sapkota, A. and Ueland, B.G. and Das, P. and Xiao, Y. and Bi, W. and Zhao, J. and Alp, E.E. and Bud'Ko, S.L. and Canfield, P.C. and Goldman, A.I. and Kreyssig, A.
PHYSICAL REVIEW B. Volume: 100 (2019) - 2019 • 5312
Quasiparticle interference and symmetry of superconducting order parameter in strongly electron-doped iron-based superconductors
Böker, J. and Volkov, P.A. and Hirschfeld, P.J. and Eremin, I.
NEW JOURNAL OF PHYSICS. Volume: 21 (2019) - 2019 • 5311
Fe/Co/Ni mixed oxide nanoparticles supported on oxidized multi-walled carbon nanotubes as electrocatalysts for the oxygen reduction and the oxygen evolution reactions in alkaline media
Kazakova, M.A. and Morales, D.M. and Andronescu, C. and Elumeeva, K. and Selyutin, A.G. and Ishchenko, A.V. and Golubtsov, G.V. and Dieckhöfer, S. and Schuhmann, W. and Masa, J.
CATALYSIS TODAY. Volume: (2019) - 2019 • 5310
Differential Protonation at the Catalytic Six-Iron Cofactor of [FeFe]-Hydrogenases Revealed by 57 Fe Nuclear Resonance X-ray Scattering and Quantum Mechanics/Molecular Mechanics Analyses
Mebs, S. and Duan, J. and Wittkamp, F. and Stripp, S.T. and Happe, T. and Apfel, U.-P. and Winkler, M. and Haumann, M.
INORGANIC CHEMISTRY. Volume: (2019) - 2019 • 5309
Modelling cyclic behaviour of martensitic steel with J2 plasticity and crystal plasticity
Sajjad, H.M. and Hanke, S. and Güler, S. and ul Hassan, H. and Fischer, A. and Hartmaier, A.
MATERIALS. Volume: 12 (2019) - 2019 • 5308
New Insights into the Catalytic Activity of Cobalt Orthophosphate Co3(PO4)2 from Charge Density Analysis
Keil, H. and Hellström, M. and Stückl, C. and Herbst-Irmer, R. and Behler, J. and Stalke, D.
CHEMISTRY - A EUROPEAN JOURNAL. Volume: 25 (2019) - 2019 • 5307
Optimizing the synthesis of Co/Co–Fe nanoparticles/N-doped carbon composite materials as bifunctional oxygen electrocatalysts
Medina, D. and Barwe, S. and Masa, J. and Seisel, S. and Schuhmann, W. and Andronescu, C.
ELECTROCHIMICA ACTA. Volume: 318 (2019) - 2019 • 5306
Spin Detection in GaAs/AlGaAs Quantum Wells by Inverse Spin-Hall Effect
Sakai, Y. and Chatani, T. and Nakagawa, T. and Ritzmann, J. and Ludwig, Ar. and Wieck, A.D. and Oiwa, A.
2019 COMPOUND SEMICONDUCTOR WEEK, CSW 2019 - PROCEEDINGS. Volume: (2019) - 2019 • 5305
Solution of high order compact discretized 3D elliptic partial differential equations by an accelerated multigrid method
Medina, A.C. and Schmid, R.
JOURNAL OF COMPUTATIONAL AND APPLIED MATHEMATICS. Volume: 350 (2019) - 2019 • 5304
Synthesis, microstructure, and hardness of rapidly solidified Cu-Cr alloys
Garzón-Manjón, A. and Christiansen, L. and Kirchlechner, I. and Breitbach, B. and Liebscher, C.H. and Springer, H. and Dehm, G.
JOURNAL OF ALLOYS AND COMPOUNDS. Volume: 794 (2019) - 2019 • 5303
A numerical analysis of a microwave induced coaxial surface wave discharge fed with a mixture of oxygen and hexamethyldisiloxane for the purpose of deposition
Kemaneci, E. and Mitschker, F. and Benedikt, J. and Eremin, D. and Awakowicz, P. and Brinkmann, R.P.
PLASMA SOURCES SCIENCE AND TECHNOLOGY. Volume: 28 (2019) - 2019 • 5302
High Order Compact Multigrid Solver for Implicit Solvation Models
Medina, A.C. and Schmid, R.
JOURNAL OF CHEMICAL THEORY AND COMPUTATION. Volume: (2019) - 2019 • 5301
Ag-Functionalized CuWO4/WO3 nanocomposites for solar water splitting
Salimi, R. and Sabbagh Alvani, A.A. and Mei, B.T. and Naseri, N. and Du, S.F. and Mul, G.
NEW JOURNAL OF CHEMISTRY. Volume: 43 (2019) - 2019 • 5300
Bulk Superconductivity and Role of Fluctuations in the Iron-Based Superconductor FeSe at High Pressures
Gati, E. and Böhmer, A.E. and Bud'ko, S.L. and Canfield, P.C.
PHYSICAL REVIEW LETTERS. Volume: 123 (2019) - 2019 • 5299
Influences of Si Substitution on Existence, Structural and Magnetic Properties of the CoMnGe Phase Investigated in a Co-Mn-Ge-Si Thin-Film Materials Library
Salomon, S. and Wöhrle, F. and Hübner, P. and Decker, P. and Ludwig, Al.
ACS COMBINATORIAL SCIENCE. Volume: 21 (2019) - 2019 • 5298
The brittle-to-ductile transition in cold rolled tungsten plates: Impact of crystallographic texture, grain size and dislocation density on the transition temperature
Bonnekoh, C. and Jäntsch, U. and Hoffmann, J. and Leiste, H. and Hartmaier, A. and Weygand, D. and Hoffmann, A. and Reiser, J.
INTERNATIONAL JOURNAL OF REFRACTORY METALS AND HARD MATERIALS. Volume: 78 (2019) - 2019 • 5297
Surface roughening of Al2O3/Al2O3-ceramic matrix composites by nanosecond laser ablation prior to thermal spraying
Gatzen, C. and Mack, D.E. and Guillon, O. and Vaßen, R.
JOURNAL OF LASER APPLICATIONS. Volume: 31 (2019) - 2019 • 5296
Effect of heat treatment on the high temperature fatigue life of single crystalline nickel base superalloy additively manufactured by means of selective electron beam melting
Meid, C. and Dennstedt, A. and Ramsperger, M. and Pistor, J. and Ruttert, B. and Lopez-Galilea, I. and Theisen, W. and Körner, C. and Bartsch, M.
SCRIPTA MATERIALIA. Volume: 168 (2019) - 2019 • 5295
Water vapor corrosion test using supersonic gas velocities
Gatzen, C. and Mack, D.E. and Guillon, O. and Vaßen, R.
JOURNAL OF THE AMERICAN CERAMIC SOCIETY. Volume: 102 (2019) - 2019 • 5294
Molecular Dynamics Simulations of the “Breathing” Phase Transformation of MOF Nanocrystallites
Keupp, J. and Schmid, R.
ADVANCED THEORY AND SIMULATIONS. Volume: 2 (2019) - 2019 • 5293
Miniaturization of low cycle fatigue-testing of single crystal superalloys at high temperature for uncoated and coated specimens [Miniaturisierung der Versuchstechnik für Niedrig-Lastwechsel-Ermüdung bei Hochtemperatur an Proben aus einkristallinen Superlegierungen mit und ohne Schutzschichten]
Meid, C. and Waedt, U. and Subramaniam, A. and Wischek, J. and Bartsch, M. and Terberger, P. and Vaßen, R.
MATERIALWISSENSCHAFT UND WERKSTOFFTECHNIK. Volume: 50 (2019) - 2018 • 5292
Elemental partitioning and site-occupancy in γ/γ′ forming Co-Ti-Mo and Co-Ti-Cr alloys
Im, H.J. and Makineni, S.K. and Gault, B. and Stein, F. and Raabe, D. and Choi, P.-P.
SCRIPTA MATERIALIA. Volume: 154 (2018) - 2018 • 5291
Automatic selection of atomic fingerprints and reference configurations for machine-learning potentials
Imbalzano, G. and Anelli, A. and Giofré, D. and Klees, S. and Behler, J. and Ceriotti, M.
JOURNAL OF CHEMICAL PHYSICS. Volume: 148 (2018) - 2018 • 5290
Aftershocks following crash of currency exchange rate: The case of RUB/USD in 2014
Usmanova, V. and Lysogorskiy, Y.V. and Abe, S.
EPL. Volume: 121 (2018) - 2018 • 5289
Computational Structure Prediction of (4,4)-Connected Copper Paddle-wheel-based MOFs: Influence of Ligand Functionalization on the Topological Preference
Impeng, S. and Cedeno, R. and Dürholt, J.P. and Schmid, R. and Bureekaew, S.
CRYSTAL GROWTH AND DESIGN. Volume: 18 (2018) - 2018 • 5288
Method for conducting in situ high temperature DIC with simultaneous synchrotron measurements under thermomechanical load
Rossmann, L. and Sarley, B. and Hernandez, J. and Kenesei, P. and Almer, J. and Wischek, J. and Bartsch, M. and Kösterk, A. and Maurel, V. and Raghavan, S.
AIAA/ASCE/AHS/ASC STRUCTURES, STRUCTURAL DYNAMICS, AND MATERIALS CONFERENCE, 2018. Volume: (2018) - 2018 • 5287
Dynamical heterogeneities of rotational motion in room temperature ionic liquids evidenced by molecular dynamics simulations
Usui, K. and Hunger, J. and Bonn, M. and Sulpizi, M.
JOURNAL OF CHEMICAL PHYSICS. Volume: 148 (2018) - 2018 • 5286
Wet-Chemical Synthesis of Pd-Au Core-Shell Nanoparticles (8 nm): From Nanostructure to Biological Properties
Rostek, A. and Breisch, M. and Loza, K. and Garcia, P.R.A.F. and Oliveira, C.L.P. and Prymak, O. and Heggen, M. and Köller, M. and Sengstock, C. and Epple, M.
CHEMISTRYSELECT. Volume: 3 (2018) - 2018 • 5285
Bifunctional Oxygen Reduction/Oxygen Evolution Activity of Mixed Fe/Co Oxide Nanoparticles with Variable Fe/Co Ratios Supported on Multiwalled Carbon Nanotubes
Elumeeva, K. and Kazakova, M.A. and Morales, D.M. and Medina, D. and Selyutin, A. and Golubtsov, G. and Ivanov, Y. and Kuznetzov, V. and Chuvilin, A. and Antoni, H. and Muhler, M. and Schuhmann, W. and Masa, J.
CHEMSUSCHEM. Volume: 11 (2018) - 2018 • 5284
Comparative biological effects of spherical noble metal nanoparticles (Rh, Pd, Ag, Pt, Au) with 4-8 nm diameter
Rostek, A. and Breisch, M. and Pappert, K. and Loza, K. and Heggen, M. and Köller, M. and Sengstock, C. and Epple, M.
BEILSTEIN JOURNAL OF NANOTECHNOLOGY. Volume: 9 (2018) - 2018 • 5283
Illumination-induced nonequilibrium charge states in self-assembled quantum dots
Valentin, S.R. and Schwinger, J. and Eickelmann, P. and Labud, P.A. and Wieck, A.D. and Sothmann, B. and Ludwig, Ar.
PHYSICAL REVIEW B. Volume: 97 (2018) - 2018 • 5282
Microscopic Insight into Electron-Induced Dissociation of Aromatic Molecules on Ice
Auburger, P. and Kemeny, I. and Bertram, C. and Ligges, M. and Bockstedte, M. and Bovensiepen, U. and Morgenstern, K.
PHYSICAL REVIEW LETTERS. Volume: 121 (2018) - 2018 • 5281
Four single-spin Rabi oscillations in a quadruple quantum dot
Ito, T. and Otsuka, T. and Nakajima, T. and Delbecq, M.R. and Amaha, S. and Yoneda, J. and Takeda, K. and Noiri, A. and Allison, G. and Ludwig, Ar. and Wieck, A.D. and Tarucha, S.
APPLIED PHYSICS LETTERS. Volume: 113 (2018) - 2018 • 5280
Unveiling the bosonic nature of an ultrashort few-electron pulse
Roussely, G. and Arrighi, E. and Georgiou, G. and Takada, S. and Schalk, M. and Urdampilleta, M. and Ludwig, Ar. and Wieck, A.D. and Armagnat, P. and Kloss, T. and Waintal, X. and Meunier, T. and Bäuerle, C.
NATURE COMMUNICATIONS. Volume: 9 (2018) - 2018 • 5279
Fourier Analysis of Serial Dependence Measures
Van Hecke, R. and Volgushev, S. and Dette, H.
JOURNAL OF TIME SERIES ANALYSIS. Volume: 39 (2018) - 2018 • 5278
A fully protected hydrogenase/polymer-based bioanode for high-performance hydrogen/glucose biofuel cells
Ruff, A. and Szczesny, J. and Marković, N. and Conzuelo, F. and Zacarias, S. and Pereira, I.A.C. and Lubitz, W. and Schuhmann, W.
NATURE COMMUNICATIONS. Volume: 9 (2018) - 2018 • 5277
Vacuum plasma spraying of functionally graded tungsten/EUROFER97 coatings for fusion applications
Vaßen, R. and Rauwald, K.-H. and Guillon, O. and Aktaa, J. and Weber, T. and Back, H.C. and Qu, D. and Gibmeier, J.
FUSION ENGINEERING AND DESIGN. Volume: 133 (2018) - 2018 • 5276
Development of W-coating with functionally graded W/EUROFER-layers for protection of First-Wall materials
Emmerich, T. and Qu, D. and Vaßen, R. and Aktaa, J.
FUSION ENGINEERING AND DESIGN. Volume: 128 (2018) - 2018 • 5275
Water assisted atomic layer deposition of yttrium oxide using tris(N,N0-diisopropyl-2-dimethylamido-guanidinato) yttrium(III): Process development, film characterization and functional properties†
Mai, L. and Boysen, N. and Subaşı, E. and De Los Arcos, T. and Rogalla, D. and Grundmeier, G. and Bock, C. and Lu, H.-L. and Devi, A.
RSC ADVANCES. Volume: 8 (2018) - 2018 • 5274
Effect of porosity and eutectics on the high-temperature low-cycle fatigue performance of a nickel-base single-crystal superalloy
Ruttert, B. and Meid, C. and Mujica Roncery, L. and Lopez-Galilea, I. and Bartsch, M. and Theisen, W.
SCRIPTA MATERIALIA. Volume: 155 (2018) - 2018 • 5273
Motion-based material characterization in sensor-based sorting
Maier, G. and Pfaff, F. and Becker, F. and Pieper, C. and Gruna, R. and Noack, B. and Kruggel-Emden, H. and Längle, T. and Hanebeck, U.D. and Wirtz, S. and Scherer, V. and Beyerer, J.
TECHNISCHES MESSEN. Volume: 85 (2018) - 2018 • 5272
Rejuvenation of Single-Crystal Ni-Base Superalloy Turbine Blades: Unlimited Service Life?
Ruttert, B. and Horst, O. and Lopez-galilea, I. and Langenkämper, D. and Kostka, A. and Somsen, C. and Goerler, J.V. and Ali, M.A. and Shchyglo, O. and Steinbach, I. and Eggeler, G. and Theisen, W.
METALLURGICAL AND MATERIALS TRANSACTIONS A: PHYSICAL METALLURGY AND MATERIALS SCIENCE. Volume: (2018) - 2018 • 5271
Towards sustainable chlorate production: The effect of permanganate addition on current efficiency
Endrődi, B. and Sandin, S. and Smulders, V. and Simic, N. and Wildlock, M. and Mul, G. and Mei, B.T. and Cornell, A.
JOURNAL OF CLEANER PRODUCTION. Volume: 182 (2018) - 2018 • 5270
Robotic microplate voltammetry for real-time hydrogel drug release testing
Jaikaew, W. and Ruff, A. and Khunkaewla, P. and Erichsen, T. and Schuhmann, W. and Schulte, A.
ANALYTICA CHIMICA ACTA. Volume: 1041 (2018) - 2018 • 5269
On the diffusive phase transformation mechanism assisted by extended dislocations during creep of a single crystal CoNi-based superalloy
Makineni, S.K. and Kumar, A. and Lenz, M. and Kontis, P. and Meiners, T. and Zenk, C. and Zaefferer, S. and Eggeler, G. and Neumeier, S. and Spiecker, E. and Raabe, D. and Gault, B.
ACTA MATERIALIA. Volume: 155 (2018) - 2018 • 5268
Amphiphilic Alginates for Marine Antifouling Applications
Jakobi, V. and Schwarze, J. and Finlay, J.A. and Nolte, K.A. and Spöllmann, S. and Becker, H.-W. and Clare, A.S. and Rosenhahn, A.
BIOMACROMOLECULES. Volume: 19 (2018) - 2018 • 5267
Elemental segregation to antiphase boundaries in a crept CoNi-based single crystal superalloy
Makineni, S.K. and Lenz, M. and Neumeier, S. and Spiecker, E. and Raabe, D. and Gault, B.
SCRIPTA MATERIALIA. Volume: 157 (2018) - 2018 • 5266
Tailored β-Ketoiminato Complexes of Iron: Synthesis, Characterization, and Evaluation towards Solution-Based Deposition of Iron Oxide Thin Films
Sadlo, A. and Beer, S.M.J. and Rahman, S. and Grafen, M. and Rogalla, D. and Winter, M. and Ostendorf, A. and Devi, A.
EUROPEAN JOURNAL OF INORGANIC CHEMISTRY. Volume: 2018 (2018) - 2018 • 5265
A Newly Developed mm-Wave Sensor for Detecting Plaques of Arterial Vessels
Vogt, S. and Detert, M. and Wagner, D. and Wessel, J. and Ramzan, R. and Nimphius, W. and Ramaswamy, A. and Guha, S. and Wenger, C. and Jamal, F.I. and Eissa, M.H. and Schumann, U. and Schmidt, B. and Rose, G. and Dahl, C. and Rolfes, I. and Notzon, G. and Baer, C. and Musch, T.
THORACIC AND CARDIOVASCULAR SURGEON. Volume: 66 (2018) - 2018 • 5264
Potential-pulse assisted thiol chemisorption minimizes non-specific adsorptions in DNA assays
Jambrec, D. and Conzuelo, F. and Zhao, B. and Schuhmann, W.
ELECTROCHIMICA ACTA. Volume: 276 (2018) - 2018 • 5263
Synthesis and stabilization of a new phase regime in a Mo-Si-B based alloy by laser-based additive manufacturing
Makineni, S.K. and Kini, A.R. and Jägle, E.A. and Springer, H. and Raabe, D. and Gault, B.
ACTA MATERIALIA. Volume: 151 (2018) - 2018 • 5262
Designing Stability into Thermally Reactive Plumbylenes
Bačić, G. and Zanders, D. and Mallick, B. and Devi, A. and Barry, S.T.
INORGANIC CHEMISTRY. Volume: 57 (2018) - 2018 • 5261
Correlative Microscopy—Novel Methods and Their Applications to Explore 3D Chemistry and Structure of Nanoscale Lattice Defects: A Case Study in Superalloys
Makineni, S.K. and Lenz, M. and Kontis, P. and Li, Z. and Kumar, A. and Felfer, P.J. and Neumeier, S. and Herbig, M. and Spiecker, E. and Raabe, D. and Gault, B.
JOM. Volume: (2018) - 2018 • 5260
Mapping of the detecting units of the resonator-based multiplexed sensor
Saetchnikov, A. and Tcherniavskaia, E. and Saetchnikov, V. and Ostendorf, A.
PROCEEDINGS OF SPIE - THE INTERNATIONAL SOCIETY FOR OPTICAL ENGINEERING. Volume: 10678 (2018) - 2018 • 5259
Thermophysical and Mechanical Properties of Advanced Single Crystalline Co-base Superalloys
Volz, N. and Zenk, C.H. and Cherukuri, R. and Kalfhaus, T. and Weiser, M. and Makineni, S.K. and Betzing, C. and Lenz, M. and Gault, B. and Fries, S.G. and Schreuer, J. and Vaßen, R. and Virtanen, S. and Raabe, D. and Spiecker, E. and Neumeier, S. and Göken, M.
METALLURGICAL AND MATERIALS TRANSACTIONS A: PHYSICAL METALLURGY AND MATERIALS SCIENCE. Volume: 49 (2018) - 2018 • 5258
Effect of a thin reflective film between substrate and photoresin on two-photon polymerization
Saetchnikov, A. and Saetchnikov, V. and Tcherniavskaia, E. and Ostendorf, A.
ADDITIVE MANUFACTURING. Volume: 24 (2018) - 2018 • 5257
Humanitarian Microwave Detection of Improvised Explosive Devices in Colombia
Baer, C. and Schulz, C. and Just, T. and Gutierrez, S. and Orend, K. and Barowski, J. and Martinez, D. and Hattenhorst, B. and Jebramcik, J. and Pantoja, J. and Musch, T. and Rolfes, I. and Sachs, J. and Vega, F.
PROCEEDINGS OF THE 2018 20TH INTERNATIONAL CONFERENCE ON ELECTROMAGNETICS IN ADVANCED APPLICATIONS, ICEAA 2018. Volume: (2018) - 2018 • 5256
Oxidation and stability of multi-walled carbon nanotubes in hydrogen peroxide solution
Safo, I.A. and Liu, F. and Xie, K. and Xia, W.
MATERIALS CHEMISTRY AND PHYSICS. Volume: 214 (2018) - 2018 • 5255
Local laser-strengthening: Customizing the forming behavior of car body steel sheets
Wagner, M. and Jahn, A. and Beyer, E. and Balzani, D.
AIP CONFERENCE PROCEEDINGS. Volume: 1960 (2018) - 2018 • 5254
A Simple Test for White Noise in Functional Time Series
Bagchi, P. and Characiejus, V. and Dette, H.
JOURNAL OF TIME SERIES ANALYSIS. Volume: 39 (2018) - 2018 • 5253
Oxygen Evolution Catalysis with Mössbauerite—A Trivalent Iron-Only Layered Double Hydroxide
Ertl, M. and Andronescu, C. and Moir, J. and Zobel, M. and Wagner, F.E. and Barwe, S. and Ozin, G. and Schuhmann, W. and Breu, J.
CHEMISTRY - A EUROPEAN JOURNAL. Volume: 24 (2018) - 2018 • 5252
Dislocation slip transmission through a coherent Σ3{111} copper twin boundary: Strain rate sensitivity, activation volume and strength distribution function
Malyar, N.V. and Grabowski, B. and Dehm, G. and Kirchlechner, C.
ACTA MATERIALIA. Volume: 161 (2018) - 2018 • 5251
Hardware-accelerated embedded SAR processor for realtime FMCW radar applications
Wagner, J. and Barowski, J. and Kalb, T. and Rolfes, I. and Göhringer, D.
GEMIC 2018 - 2018 GERMAN MICROWAVE CONFERENCE. Volume: 2018-January (2018) - 2018 • 5250
Scanning Bipolar Electrochemical Microscopy
Eßmann, V. and Santana Santos, C. and Tarnev, T. and Bertotti, M. and Schuhmann, W.
ANALYTICAL CHEMISTRY. Volume: 90 (2018) - 2018 • 5249
Optimizing the Synthesis of Zinc-rich Gallium Zinc Oxynitrides by Combining Co-Precipitation and Moisture-Assisted Nitridation
Jansen, H. and Menze, J. and Muhler, M.
ZEITSCHRIFT FUR ANORGANISCHE UND ALLGEMEINE CHEMIE. Volume: 644 (2018) - 2018 • 5248
Comparison of thermodynamic topology optimization with SIMP
Jantos, D.R. and Riedel, C. and Hackl, K. and Junker, P.
CONTINUUM MECHANICS AND THERMODYNAMICS. Volume: (2018) - 2018 • 5247
Photocatalytic activity of ZnV 2 O 6 /reduced graphene oxide nanocomposite: From theory to experiment
Sameie, H. and Sabbagh Alvani, A.A. and Naseri, N. and Rosei, F. and Mul, G. and Mei, B.T.
JOURNAL OF THE ELECTROCHEMICAL SOCIETY. Volume: 165 (2018) - 2018 • 5246
Cold gas spraying of Ti-48Al-2Cr-2Nb intermetallic for jet engine applications
Bakan, E. and Mauer, G. and Sohn, Y.J. and Schwedt, A. and Rackel, M.W. and Riedlberger, F. and Pyczak, F. and Peters, J.O. and Mecklenburg, M. and Gartner, T.M. and Vaßen, R.
SURFACE AND COATINGS TECHNOLOGY. Volume: (2018) - 2018 • 5245
An accurate and fast regularization approach to thermodynamic topology optimization
Jantos, D.R. and Hackl, K. and Junker, P.
INTERNATIONAL JOURNAL FOR NUMERICAL METHODS IN ENGINEERING. Volume: (2018) - 2018 • 5244
Temperature-dependent Shape Changes of Ice Nanoclusters on Ag(100)
Bakradze, G. and Morgenstern, K.
CHEMPHYSCHEM. Volume: 19 (2018) - 2018 • 5243
Decay and revival of electron spin polarization in an ensemble of (In,Ga)As quantum dots
Evers, E. and Belykh, V.V. and Kopteva, N.E. and Yugova, I.A. and Greilich, A. and Yakovlev, D.R. and Reuter, D. and Wieck, A.D. and Bayer, M.
PHYSICAL REVIEW B. Volume: 98 (2018) - 2018 • 5242
Spin-photon interface and spin-controlled photon switching in a nanobeam waveguide
Javadi, A. and Ding, D. and Appel, M.H. and Mahmoodian, S. and Löbl, M.C. and Söllner, I. and Schott, R. and Papon, C. and Pregnolato, T. and Stobbe, Sø. and Midolo, L. and Schröder, T. and Wieck, A.D. and Ludwig, Ar. and Warburton, R.J. and Lodahl, P.
NATURE NANOTECHNOLOGY. Volume: 13 (2018) - 2018 • 5241
Spannung im Dunkelfeld
Evers, M.V. and Wonner, K. and Tschulik, K.
NACHRICHTEN AUS DER CHEMIE. Volume: 66 (2018) - 2018 • 5240
Grain boundaries in bcc-Fe: A density-functional theory and tight-binding study
Wang, J. and Madsen, G.K.H. and Drautz, R.
MODELLING AND SIMULATION IN MATERIALS SCIENCE AND ENGINEERING. Volume: 26 (2018) - 2018 • 5239
Ultrathin 2D Cobalt Zeolite-Imidazole Framework Nanosheets for Electrocatalytic Oxygen Evolution
Jayaramulu, K. and Masa, J. and Morales, D.M. and Tomanec, O. and Ranc, V. and Petr, M. and Wilde, P. and Chen, Y.-T. and Zboril, R. and Schuhmann, W. and Fischer, R.A.
ADVANCED SCIENCE. Volume: 5 (2018) - 2018 • 5238
The Impact of Hysteresis on the Electrocaloric Effect at First-Order Phase Transitions
Marathe, M. and Ederer, C. and Grünebohm, A.
PHYSICA STATUS SOLIDI (B) BASIC RESEARCH. Volume: 255 (2018) - 2018 • 5237
Transient DEM-CFD simulation of solid and fluid flow in a three dimensional blast furnace model
Bambauer, F. and Wirtz, S. and Scherer, V. and Bartusch, H.
POWDER TECHNOLOGY. Volume: 334 (2018) - 2018 • 5236
A simulation concept based on the FDFD method for ground penetrating radar used in humanitarian demining
Jebramcik, J. and Barowski, J. and Pohle, D. and Baer, C. and Rolfes, I.
EUROPEAN MICROWAVE WEEK 2017: "A PRIME YEAR FOR A PRIME EVENT", EUMW 2017 - CONFERENCE PROCEEDINGS; 14TH EUROPEAN MICROWAVE CONFERENCE, EURAD 2017. Volume: 2018-January (2018) - 2018 • 5235
Monte Carlo simulation of column growth in plasma spray physical vapor deposition process
Wang, P. and He, W. and Mauer, G. and Mücke, R. and Vaßen, R.
SURFACE AND COATINGS TECHNOLOGY. Volume: 335 (2018) - 2018 • 5234
Electronic structure based descriptor for characterizing local atomic environments
Jenke, J. and Subramanyam, A.P.A. and Densow, M. and Hammerschmidt, T. and Pettifor, D.G. and Drautz, R.
PHYSICAL REVIEW B. Volume: 98 (2018) - 2018 • 5233
Numerical analysis of wet plastic particle separation using a coupled DEM-SPH method
Markauskas, D. and Kruggel-Emden, H. and Scherer, V.
POWDER TECHNOLOGY. Volume: 325 (2018) - 2018 • 5232
In-situ SEM observation of phase transformation and twinning mechanisms in an interstitial high-entropy alloy
Wang, M. and Li, Z. and Raabe, D.
ACTA MATERIALIA. Volume: 147 (2018) - 2018 • 5231
Development of Single-Crystal Ni-Base Superalloys Based on Multi-criteria Numerical Optimization and Efficient Use of Refractory Elements
Markl, M. and Müller, A. and Ritter, N. and Hofmeister, M. and Naujoks, D. and Schaar, H. and Abrahams, K. and Frenzel, J. and Subramanyam, A.P.A. and Ludwig, Al. and Pfetzing-Micklich, J. and Hammerschmidt, T. and Drautz, R. and Steinbach, I. and Rettig, R. and Singer, R.F. and Körner, C.
METALLURGICAL AND MATERIALS TRANSACTIONS A: PHYSICAL METALLURGY AND MATERIALS SCIENCE. Volume: 49 (2018) - 2018 • 5230
Understanding precipitate evolution during friction stir welding of Al-Zn-Mg-Cu alloy through in-situ measurement coupled with simulation
dos Santos, J.F. and Staron, P. and Fischer, T. and Robson, J.D. and Kostka, A. and Colegrove, P. and Wang, H. and Hilgert, J. and Bergmann, L. and Hütsch, L.L. and Huber, N. and Schreyer, A.
ACTA MATERIALIA. Volume: 148 (2018) - 2018 • 5229
Cu supported on thin carbon layer-coated porous SiO2 for efficient ethanol dehydrogenation
Wang, Q.-N. and Shi, L. and Li, W. and Li, W.-C. and Si, R. and Schüth, F. and Lu, A.-H.
CATALYSIS SCIENCE AND TECHNOLOGY. Volume: 8 (2018) - 2018 • 5228
An Air-breathing Carbon Cloth-based Screen-printed Electrode for Applications in Enzymatic Biofuel Cells
Marković, N. and Conzuelo, F. and Szczesny, J. and González García, M.B. and Hernández Santos, D. and Ruff, A. and Schuhmann, W.
ELECTROANALYSIS. Volume: (2018) - 2018 • 5227
Live cyanobacteria produce photocurrent and hydrogen using both the respiratory and photosynthetic systems
Saper, G. and Kallmann, D. and Conzuelo, F. and Zhao, F. and Tóth, T.N. and Liveanu, V. and Meir, S. and Szymanski, J. and Aharoni, A. and Schuhmann, W. and Rothschild, A. and Schuster, G. and Adir, N.
NATURE COMMUNICATIONS. Volume: 9 (2018) - 2018 • 5226
Carbide types in an advanced microalloyed bainitic/ferritic Cr–Mo Steel – TEM observations and thermodynamic calculations [Karbide in einem mikrolegierten bainitisch-ferritischen Cr–Mo-Stahl – TEM Charakterisierung und thermodynamische Berechnungen]
Wang, H. and Somsen, C. and Eggeler, G. and Detemple, E.
MATERIALWISSENSCHAFT UND WERKSTOFFTECHNIK. Volume: 49 (2018) - 2018 • 5225
Pressure effects on the electronic properties of the undoped superconductor ThFeAsN
Barbero, N. and Holenstein, S. and Shang, T. and Shermadini, Z. and Lochner, F. and Eremin, I. and Wang, C. and Cao, G.-H. and Khasanov, R. and Ott, H.-R. and Mesot, J. and Shiroka, T.
PHYSICAL REVIEW B. Volume: 97 (2018) - 2018 • 5224
Comment on ‘Formation of fast-spreading lower oceanic crust as revealed by a new Mg–REE coupled geospeedometer’ by Sun and Lissenberg
Faak, K. and Chakraborty, S. and Coogan, L.A. and Dohmen, R.
EARTH AND PLANETARY SCIENCE LETTERS. Volume: 502 (2018) - 2018 • 5223
Doping evolution of spin fluctuations and their peculiar suppression at low temperatures in Ca(Fe1-xCox)2As2
Sapkota, A. and Das, P. and Böhmer, A.E. and Ueland, B.G. and Abernathy, D.L. and Bud'Ko, S.L. and Canfield, P.C. and Kreyssig, A. and Goldman, A.I. and McQueeney, R.J.
PHYSICAL REVIEW B. Volume: 97 (2018) - 2018 • 5222
Development of high modulus steels based on the Fe – Cr – B system
Baron, C. and Springer, H. and Raabe, D.
MATERIALS SCIENCE AND ENGINEERING A. Volume: 724 (2018) - 2018 • 5221
Precipitation hardening effects on extension twinning in magnesium alloys
Fan, H. and Zhu, Y. and El-Awady, J.A. and Raabe, D.
INTERNATIONAL JOURNAL OF PLASTICITY. Volume: 106 (2018) - 2018 • 5220
Construction of statistically similar representative volume elements for discontinuous fiber composites
Sasagawa, T. and Tanaka, M. and Omote, R. and Balzani, D.
COMPOSITE STRUCTURES. Volume: 203 (2018) - 2018 • 5219
Influences of W Content on the Phase Transformation Properties and the Associated Stress Change in Thin Film Substrate Combinations Studied by Fabrication and Characterization of Thin Film V1- xWxO2 Materials Libraries
Wang, X. and Rogalla, D. and Ludwig, Al.
ACS COMBINATORIAL SCIENCE. Volume: 20 (2018) - 2018 • 5218
Millimeter-wave characterization of dielectric materials using calibrated FMCW transceivers
Barowski, J. and Zimmermanns, M. and Rolfes, I.
IEEE TRANSACTIONS ON MICROWAVE THEORY AND TECHNIQUES. Volume: 66 (2018) - 2018 • 5217
Hydrogen embrittlement of tungsten induced by deuterium plasma: Insights from nanoindentation tests
Fang, X. and Kreter, A. and Rasinski, M. and Kirchlechner, C. and Brinckmann, S. and Linsmeier, C. and Dehm, G.
JOURNAL OF MATERIALS RESEARCH. Volume: 33 (2018) - 2018 • 5216
Time-resolved impact electrochemistry - A new method to determine diffusion coefficients of ions in solution
Saw, E.N. and Blanc, N. and Kanokkanchana, K. and Tschulik, K.
ELECTROCHIMICA ACTA. Volume: 282 (2018) - 2018 • 5215
Investigation of multiple laser shock peening on the mechanical property and corrosion resistance of shipbuilding 5083Al alloy under a simulated seawater environment
Wang, H. and Huang, Y. and Zhang, W. and Ostendorf, A.
APPLIED OPTICS. Volume: 57 (2018) - 2018 • 5214
Millimeter wave material characterization using FMCW-transceivers
Barowski, J. and Rolfes, I.
2017 IEEE MTT-S INTERNATIONAL MICROWAVE WORKSHOP SERIES ON ADVANCED MATERIALS AND PROCESSES FOR RF AND THZ APPLICATIONS, IMWS-AMP 2017. Volume: 2018-January (2018) - 2018 • 5213
On the role of the collinear dislocation interaction in deformation patterning and laminate formation in single crystal plasticity
Wang, D. and Diehl, M. and Roters, F. and Raabe, D.
MECHANICS OF MATERIALS. Volume: 125 (2018) - 2018 • 5212
Manganese(II) Molecular Sources for Plasma-Assisted CVD of Mn Oxides and Fluorides: From Precursors to Growth Process
Barreca, D. and Carraro, G. and Fois, E. and Gasparotto, A. and Gri, F. and Seraglia, R. and Wilken, M. and Venzo, A. and Devi, A. and Tabacchi, G. and Maccato, C.
JOURNAL OF PHYSICAL CHEMISTRY C. Volume: 122 (2018) - 2018 • 5211
Analysis of hydrogen diffusion and trapping in ultra-high strength steel grades
Schaffner, T. and Hartmaier, A. and Kokotin, V. and Pohl, M.
JOURNAL OF ALLOYS AND COMPOUNDS. Volume: 746 (2018) - 2018 • 5210
Scalable One-Pot Synthesis of Yolk-Shell Carbon Nanospheres with Yolk-Supported Pd Nanoparticles for Size-Selective Catalysis
Wang, G.-H. and Chen, K. and Engelhardt, J. and Tüysüz, H. and Bongard, H.-J. and Schmidt, W. and Schüth, F.
CHEMISTRY OF MATERIALS. Volume: 30 (2018) - 2018 • 5209
Overcoming cathode poisoning from electrolyte impurities in alkaline electrolysis by means of self-healing electrocatalyst films
Barwe, S. and Mei, B. and Masa, J. and Schuhmann, W. and Ventosa, E.
NANO ENERGY. Volume: 53 (2018) - 2018 • 5208
Direct visualization of phase separation between superconducting and nematic domains in Co-doped CaFe2As2 close to a first-order phase transition
Fente, A. and Correa-Orellana, A. and Böhmer, A.E. and Kreyssig, A. and Ran, S. and Bud'Ko, S.L. and Canfield, P.C. and Mompean, F.J. and García-Hernández, M. and Munuera, C. and Guillamón, I. and Suderow, H.
PHYSICAL REVIEW B. Volume: 97 (2018) - 2018 • 5207
Ex situ and in situ TEM investigations of carbide precipitation in a 10Cr martensitic steel
Wang, H.
JOURNAL OF MATERIALS SCIENCE. Volume: 53 (2018) - 2018 • 5206
Electrocatalytic Oxidation of 5-(Hydroxymethyl)furfural Using High-Surface-Area Nickel Boride
Barwe, S. and Weidner, J. and Cychy, S. and Morales, D.M. and Dieckhöfer, S. and Hiltrop, D. and Masa, J. and Muhler, M. and Schuhmann, W.
ANGEWANDTE CHEMIE - INTERNATIONAL EDITION. Volume: 57 (2018) - 2018 • 5205
Unusual composition dependence of transformation temperatures in Ti-Ta-X shape memory alloys
Ferrari, A. and Paulsen, A. and Frenzel, J. and Rogal, J. and Eggeler, G. and Drautz, R.
PHYSICAL REVIEW MATERIALS. Volume: 2 (2018) - 2018 • 5204
Indication of subdominant D-wave interaction in superconducting CaKFe4As4
Jost, D. and Scholz, J.-R. and Zweck, U. and Meier, W.R. and Böhmer, A.E. and Canfield, P.C. and Lazarević, N. and Hackl, R.
PHYSICAL REVIEW B. Volume: 98 (2018) - 2018 • 5203
Temporal temperature evolution in laser micro-spot welding of copper considering temperature-dependent material parameters
Mattern, M. and Weigel, T. and Ostendorf, A.
MATERIALS RESEARCH EXPRESS. Volume: 5 (2018) - 2018 • 5202
Atomic Layer Deposition of Molybdenum and Tungsten Oxide Thin Films Using Heteroleptic Imido-Amidinato Precursors: Process Development, Film Characterization, and Gas Sensing Properties
Mattinen, M. and Wree, J.-L. and Stegmann, N. and Ciftyurek, E. and Achhab, M.E. and King, P.J. and Mizohata, K. and Räisänen, J. and Schierbaum, K.D. and Devi, A. and Ritala, M. and Leskelä, M.
CHEMISTRY OF MATERIALS. Volume: 30 (2018) - 2018 • 5201
Coupled effect of crystallographic orientation and indenter geometry on nanoindentation of single crystalline copper
Wang, Z. and Zhang, J. and Hassan, H.U. and Zhang, J. and Yan, Y. and Hartmaier, A. and Sun, T.
INTERNATIONAL JOURNAL OF MECHANICAL SCIENCES. Volume: 148 (2018) - 2018 • 5200
Plasmatechnik 4.0: Stand der Technik, Entwicklungen und Erwartungen
Ferse, K. and Awakowicz, P. and Beck, U. and Brand, C. and Engelstädter, J.P. and Fiedler, W. and Foest, R. and Kersten, H. and Lemmer, O. and Schäfer, H.-J. and Schwock, A.
VAKUUM IN FORSCHUNG UND PRAXIS. Volume: 30 (2018) - 2018 • 5199
On the accumulation of irreversible plastic strain during compression loading of open-pore metallic foams
Matz, A.M. and Matz, B.S. and Jost, N. and Eggeler, G.
MATERIALS SCIENCE AND ENGINEERING A. Volume: 728 (2018) - 2018 • 5198
Multi-phase-field method for surface tension induced elasticity
Schiedung, R. and Steinbach, I. and Varnik, F.
PHYSICAL REVIEW B. Volume: 97 (2018) - 2018 • 5197
Conditions for nucleation and growth in the substrate boundary layer at plasma spray-physical vapor deposition (PS-PVD)
Mauer, G. and Vaßen, R.
SURFACE AND COATINGS TECHNOLOGY. Volume: (2018) - 2018 • 5196
Interface dominated cooperative nanoprecipitation in interstitial alloys
Wang, H. and Zhang, X. and Yan, D. and Somsen, C. and Eggeler, G.
NATURE COMMUNICATIONS. Volume: 9 (2018) - 2018 • 5195
Universal Ratio of Coulomb Interaction to Geometric Quantization in (In, Ga)As/GaAs Quantum Dots
Bayer, M. and Ludwig, Ar. and Wieck, A.
PHYSICS OF THE SOLID STATE. Volume: 60 (2018) - 2018 • 5194
Inactivation of B. subtilis spores by low pressure plasma - Influence of optical filters and photon/particle fluxes on the inactivation efficiency
Fiebrandt, M. and Hillebrand, B. and Lackmann, J.-W. and Raguse, M. and Moeller, R. and Awakowicz, P. and Stapelmann, K.
JOURNAL OF PHYSICS D: APPLIED PHYSICS. Volume: 51 (2018) - 2018 • 5193
Rational Development of Cobalt β-Ketoiminate Complexes: Alternative Precursors for Vapor-Phase Deposition of Spinel Cobalt Oxide Photoelectrodes
Junge Puring, K. and Zywitzki, D. and Taffa, D.H. and Rogalla, D. and Winter, M. and Wark, M. and Devi, A.
INORGANIC CHEMISTRY. Volume: 57 (2018) - 2018 • 5192
A comparison of the torrefaction behavior of wood, miscanthus and palm kernel shells: Measurements on single particles with geometries of technical relevance
Becker, A. and Scherer, V.
FUEL. Volume: 224 (2018) - 2018 • 5191
Crystallographic Structure Analysis of a Ti-Ta Thin Film Materials Library Fabricated by Combinatorial Magnetron Sputtering
Kadletz, P.M. and Motemani, Y. and Iannotta, J. and Salomon, S. and Khare, C. and Grossmann, L. and Maier, H.J. and Ludwig, Al. and Schmahl, W.W.
ACS COMBINATORIAL SCIENCE. Volume: 20 (2018) - 2018 • 5190
Fouling-Release Properties of Dendritic Polyglycerols against Marine Diatoms
Wanka, R. and Finlay, J.A. and Nolte, K.A. and Koc, J. and Jakobi, V. and Anderson, C. and Clare, A.S. and Gardner, H. and Hunsucker, K.Z. and Swain, G.W. and Rosenhahn, A.
ACS APPLIED MATERIALS AND INTERFACES. Volume: 10 (2018) - 2018 • 5189
Ab initio simulation of hydrogen-induced decohesion in cementite-containing microstructures
McEniry, E.J. and Hickel, T. and Neugebauer, J.
ACTA MATERIALIA. Volume: 150 (2018) - 2018 • 5188
Far-field nanoscopy on a semiconductor quantum dot via a rapid-adiabatic-passage-based switch
Kaldewey, T. and Kuhlmann, A.V. and Valentin, S.R. and Ludwig, Ar. and Wieck, A.D. and Warburton, R.J.
NATURE PHOTONICS. Volume: 12 (2018) - 2018 • 5187
Hydrogen and oxygen trapping at the H-cluster of [FeFe]-hydrogenase revealed by site-selective spectroscopy and QM/MM calculations
Mebs, S. and Kositzki, R. and Duan, J. and Kertess, L. and Senger, M. and Wittkamp, F. and Apfel, U.-P. and Happe, T. and Stripp, S.T. and Winkler, M. and Haumann, M.
BIOCHIMICA ET BIOPHYSICA ACTA - BIOENERGETICS. Volume: 1859 (2018) - 2018 • 5186
Multiscale Characterization of Microstructure in Near-Surface Regions of a 16MnCr5 Gear Wheel After Cyclic Loading
Medghalchi, S. and Jamebozorgi, V. and Bala Krishnan, A. and Vincent, S. and Salomon, S. and Basir Parsa, A. and Pfetzing, J. and Kostka, A. and Li, Y. and Eggeler, G. and Li, T.
JOM. Volume: (2018) - 2018 • 5185
Consistent simulation of capacitive radio-frequency discharges and external matching networks
Schmidt, F. and Mussenbrock, T. and Trieschmann, J.
PLASMA SOURCES SCIENCE AND TECHNOLOGY. Volume: 27 (2018) - 2018 • 5184
Coupling Phenomena in Magnetocaloric Materials
Waske, A. and Dutta, B. and Teichert, N. and Weise, B. and Shayanfar, N. and Becker, A. and Hütten, A. and Hickel, T.
ENERGY TECHNOLOGY. Volume: 6 (2018) - 2018 • 5183
Fabrication of zinc-dicarboxylate- and zinc-pyrazolate-carboxylate-framework thin films through vapour-solid deposition
Medishetty, R. and Zhang, Z. and Sadlo, A. and Cwik, S. and Peeters, D. and Henke, S. and Mangayarkarasi, N. and Devi, A.
DALTON TRANSACTIONS. Volume: 47 (2018) - 2018 • 5182
Multi frequency matching for voltage waveform tailoring
Schmidt, F. and Schulze, J. and Johnson, E. and Booth, J.-P. and Keil, D. and French, D.M. and Trieschmann, J. and Mussenbrock, T.
PLASMA SOURCES SCIENCE AND TECHNOLOGY. Volume: 27 (2018) - 2018 • 5181
A focused information criterion for quantile regression: Evidence for the rebound effect
Behl, P. and Dette, H. and Frondel, M. and Vance, C.
QUARTERLY REVIEW OF ECONOMICS AND FINANCE. Volume: (2018) - 2018 • 5180
High-performance elastocaloric materials for the engineering of bulk- and micro-cooling devices
Frenzel, J. and Eggeler, G. and Quandt, E. and Seelecke, S. and Kohl, M.
MRS BULLETIN. Volume: 43 (2018) - 2018 • 5179
Ultrahigh-temperature tensile creep of TiC-reinforced Mo-Si-B-based alloy
Kamata, S.Y. and Kanekon, D. and Lu, Y. and Sekido, N. and Maruyama, K. and Eggeler, G. and Yoshimi, K.
SCIENTIFIC REPORTS. Volume: 8 (2018) - 2018 • 5178
Driving Surface Redox Reactions in Heterogeneous Photocatalysis: The Active State of Illuminated Semiconductor-Supported Nanoparticles during Overall Water-Splitting
Mei, B. and Han, K. and Mul, G.
ACS CATALYSIS. Volume: 8 (2018) - 2018 • 5177
First-principles calculations for charged defects at surfaces, interfaces, and two-dimensional materials in the presence of electric fields
Freysoldt, C. and Neugebauer, J.
PHYSICAL REVIEW B. Volume: 97 (2018) - 2018 • 5176
Hedgehog spin-vortex crystal stabilized in a hole-doped iron-based superconductor
Meier, W.R. and Ding, Q.-P. and Kreyssig, A. and Bud'Ko, S.L. and Sapkota, A. and Kothapalli, K. and Borisov, V. and Valentí, R. and Batista, C.D. and Orth, P.P. and Fernandes, R.M. and Goldman, A.I. and Furukawa, Y. and Böhmer, A.E. and Canfield, P.C.
NPJ QUANTUM MATERIALS. Volume: 3 (2018) - 2018 • 5175
An Alternative Approach for the Estimation of Biochar Yields
Weber, K. and Heuer, S. and Quicker, P. and Li, T. and Løvås, T. and Scherer, V.
ENERGY AND FUELS. Volume: 32 (2018) - 2018 • 5174
Origin of the low magnetic moment in Fe2AlTi: An Ab initio study
Friák, M. and Slávik, A. and Miháliková, I. and Holec, D. and Všianská, M. and Šob, M. and Palm, M. and Neugebauer, J.
MATERIALS. Volume: 11 (2018) - 2018 • 5173
Sulfur – induced embrittlement in high-purity, polycrystalline copper
Meiners, T. and Peng, Z. and Gault, B. and Liebscher, C.H. and Dehm, G.
ACTA MATERIALIA. Volume: 156 (2018) - 2018 • 5172
Nano Impact Electrochemistry: Effects of Electronic Filtering on Peak Height, Duration and Area
Kanokkanchana, K. and Saw, E.N. and Tschulik, K.
CHEMELECTROCHEM. Volume: 5 (2018) - 2018 • 5171
Machine-learning-based atom probe crystallographic analysis
Wei, Y. and Gault, B. and Varanasi, R.S. and Raabe, D. and Herbig, M. and Breen, A.J.
ULTRAMICROSCOPY. Volume: 194 (2018) - 2018 • 5170
Nano-Impact Electrochemistry: Effects of Electronic Filtering on Peak Height, Duration, and Area
Kanokkanchana, K. and Saw, E.N. and Tschulik, K.
CHEMELECTROCHEM. Volume: 5 (2018) - 2018 • 5169
Using Instability of a Non-stoichiometric Mixed Oxide Oxygen Evolution Catalyst As a Tool to Improve Its Electrocatalytic Performance
Kasian, O. and Geiger, S. and Schalenbach, M. and Mingers, A.M. and Savan, A. and Ludwig, Al. and Cherevko, S. and Mayrhofer, K.J.J.
ELECTROCATALYSIS. Volume: 9 (2018) - 2018 • 5168
Simultaneous nanopatterning and reduction of graphene oxide by femtosecond laser pulses
Kasischke, M. and Maragkaki, S. and Volz, S. and Ostendorf, A. and Gurevich, E.L.
APPLIED SURFACE SCIENCE. Volume: 445 (2018) - 2018 • 5167
27. Bioorganik-Nachwuchssymposium in Bochum
Merten, C. and Apfel, U.-P.
NACHRICHTEN AUS DER CHEMIE. Volume: 66 (2018) - 2018 • 5166
Cobalt-metalloid alloys for electrochemical oxidation of 5-hydroxymethylfurfural as an alternative anode reaction in lieu of oxygen evolution during water splitting
Weidner, J. and Barwe, S. and Sliozberg, K. and Piontek, S. and Masa, J. and Apfel, U.-P. and Schuhmann, W.
BEILSTEIN JOURNAL OF ORGANIC CHEMISTRY. Volume: 14 (2018) - 2018 • 5165
Impact of local electrostatic field rearrangement on field ionization
Katnagallu, S. and Dagan, M. and Parviainen, S. and Nematollahi, A. and Grabowski, B. and Bagot, P.A.J. and Rolland, N. and Neugebauer, J. and Raabe, D. and Vurpillot, F. and Moody, M.P. and Gault, B.
JOURNAL OF PHYSICS D: APPLIED PHYSICS. Volume: 51 (2018) - 2018 • 5164
Deep etching of Zerodur glass ceramics in a fluorine-based plasma
Weigel, C. and Schulze, M. and Gargouri, H. and Hoffmann, M.
MICROELECTRONIC ENGINEERING. Volume: 185-186 (2018) - 2018 • 5163
Advanced data mining in field ion microscopy
Katnagallu, S. and Gault, B. and Grabowski, B. and Neugebauer, J. and Raabe, D. and Nematollahi, A.
MATERIALS CHARACTERIZATION. Volume: (2018) - 2018 • 5162
A microoptical sidestream cuvette based on fast passive gas exchange for capnography
Weigel, C. and Grewe, A. and Sinzinger, S. and Hoffmann, M.
SENSORS AND ACTUATORS, A: PHYSICAL. Volume: 276 (2018) - 2018 • 5161
Molecular dynamics simulation of silicon ion implantation into diamond and subsequent annealing
Fu, X. and Xu, Z. and He, Z. and Hartmaier, A. and Fang, F.
NUCLEAR INSTRUMENTS AND METHODS IN PHYSICS RESEARCH, SECTION B: BEAM INTERACTIONS WITH MATERIALS AND ATOMS. Volume: (2018) - 2018 • 5160
Monitoring Potential-Induced DNA Dehybridization Kinetics for Single Nucleotide Polymorphism Detection by using In Situ Surface Enhanced Raman Scattering
Kayran, Y.U. and Cinar, N. and Jambrec, D. and Schuhmann, W.
CHEMELECTROCHEM. Volume: (2018) - 2018 • 5159
Rapid Assessment of Sputtered Nanoparticle Ionic Liquid Combinations
Meyer, H. and Meischein, M. and Ludwig, Al.
ACS COMBINATORIAL SCIENCE. Volume: 20 (2018) - 2018 • 5158
Deep etched and released microstructures in Zerodur in a fluorine-based plasma
Weigel, C. and Sinzinger, S. and Hoffmann, M.
MICROELECTRONIC ENGINEERING. Volume: 198 (2018) - 2018 • 5157
Electrocatalytic Nanoparticles That Mimic the Three-Dimensional Geometric Architecture of Enzymes: Nanozymes
Benedetti, T.M. and Andronescu, C. and Cheong, S. and Wilde, P. and Wordsworth, J. and Kientz, M. and Tilley, R.D. and Schuhmann, W. and Gooding, J.J.
JOURNAL OF THE AMERICAN CHEMICAL SOCIETY. Volume: 140 (2018) - 2018 • 5156
Nanostructured DNA Microarrays for Dual SERS and Electrochemical Read-Out
Kayran, Y.U. and Jambrec, D. and Schuhmann, W.
ELECTROANALYSIS. Volume: (2018) - 2018 • 5155
Local Surface Structure and Composition Control the Hydrogen Evolution Reaction on Iron Nickel Sulfides
Bentley, C.L. and Andronescu, C. and Smialkowski, M. and Kang, M. and Tarnev, T. and Marler, B. and Unwin, P.R. and Apfel, U.-P. and Schuhmann, W.
ANGEWANDTE CHEMIE - INTERNATIONAL EDITION. Volume: 57 (2018) - 2018 • 5154
Experimental-numerical study on strain and stress partitioning in bainitic steels with martensite-austenite constituents
Fujita, N. and Ishikawa, N. and Roters, F. and Tasan, C.C. and Raabe, D.
INTERNATIONAL JOURNAL OF PLASTICITY. Volume: (2018) - 2018 • 5153
Robust nonlinear control of atomic force microscope via immersion and invariance
Keighobadi, J. and Hosseini-Pishrobat, M. and Faraji, J. and Oveisi, A. and Nestorović, T.
INTERNATIONAL JOURNAL OF ROBUST AND NONLINEAR CONTROL. Volume: (2018) - 2018 • 5152
Laplace deep level transient spectroscopy on self-assembled quantum dots
Schnorr, L. and Heinzel, T. and Scholz, S. and Ludwig, Ar. and Wieck, A.D.
JOURNAL OF APPLIED PHYSICS. Volume: 124 (2018) - 2018 • 5151
Effects of strain rate on mechanical properties and deformation behavior of an austenitic Fe-25Mn-3Al-3Si TWIP-TRIP steel
Benzing, J.T. and Poling, W.A. and Pierce, D.T. and Bentley, J. and Findley, K.O. and Raabe, D. and Wittig, J.E.
MATERIALS SCIENCE AND ENGINEERING A. Volume: 711 (2018) - 2018 • 5150
Electrolyte mobility in supercapacitor electrodes – Solid state NMR studies on hierarchical and narrow pore sized carbons
Fulik, N. and Hippauf, F. and Leistenschneider, D. and Paasch, S. and Kaskel, S. and Brunner, E. and Borchardt, L.
ENERGY STORAGE MATERIALS. Volume: 12 (2018) - 2018 • 5149
Exploring the long-term hydrolytic behavior of zwitterionic polymethacrylates and polymethacrylamides
Schönemann, E. and Laschewsky, A. and Rosenhahn, A.
POLYMERS. Volume: 10 (2018) - 2018 • 5148
On the Electropolishing Mechanism of Nickel Titanium in Methanolic Sulfuric acid − An Electrochemical Impedance Study
Fushimi, K. and Neelakantan, L. and Eggeler, G. and Hassel, A.W.
PHYSICA STATUS SOLIDI (A) APPLICATIONS AND MATERIALS SCIENCE. Volume: 215 (2018) - 2018 • 5147
Understanding the Effect of Au in Au-Pd Bimetallic Nanocrystals on the Electrocatalysis of the Methanol Oxidation Reaction
Kelly, C.H.W. and Benedetti, T.M. and Alinezhad, A. and Schuhmann, W. and Gooding, J.J. and Tilley, R.D.
JOURNAL OF PHYSICAL CHEMISTRY C. Volume: 122 (2018) - 2018 • 5146
Investigation of austenitic FeCrNi steels with regard to stacking-fault energy and thermal austenite stability
Fussik, R. and Walter, M. and Theisen, W. and Weber, S.
MATERIALIA. Volume: 3 (2018) - 2018 • 5145
Encapsulation Efficiency and Release Behavior of Fat Microcapsules with Lipophilic Emulsifiers [Verkapselungseffizienz und Freisetzungsverhalten fettbasierter Mikrokapseln mit lipophilen Emulgatoren]
Scholz, R. and Davico, L. and Jakobi, V. and Kareth, S. and Rosenhahn, A. and Weidner, E.
CHEMIE-INGENIEUR-TECHNIK. Volume: 90 (2018) - 2018 • 5144
Derivation of property distribution images from microstructural analyses of X2CrNi18-9 with regard to hydrogen embrittlement [Ableitung von Eigenschaftsverteilungsbildern aus mikrostrukturellen Analysen des X2CrNi18-9 im Zusammenhang mit der Wasserstoffversprödung]
Fussik, R. and Weber, S.
PRAKTISCHE METALLOGRAPHIE/PRACTICAL METALLOGRAPHY. Volume: 55 (2018) - 2018 • 5143
Self-Organized Growth of Quantum Dots and Quantum Wires by Combination of Focused Ion Beams and Molecular Beam Epitaxy
Scholz, S. and Schott, R. and Schmidt, M. and Mehta, M. and Ludwig, Ar. and Wieck, A.D.
PHYSICA STATUS SOLIDI (B) BASIC RESEARCH. Volume: (2018) - 2018 • 5142
Role of disorder when upscaling magnetocaloric Ni-Co-Mn-Al Heusler alloys from thin films to ribbons
Weise, B. and Dutta, B. and Teichert, N. and Hütten, A. and Hickel, T. and Waske, A.
SCIENTIFIC REPORTS. Volume: 8 (2018) - 2018 • 5141
Investigation of the local austenite stability related to hydrogen environment embrittlement of austenitic stainless steels
Fussik, R. and Egels, G. and Theisen, W. and Weber, S.
MATERIALS SCIENCE FORUM. Volume: 941 MSF (2018) - 2018 • 5140
Optimal designs for non-competitive enzyme inhibition kinetic models
Schorning, K. and Dette, H. and Kettelhake, K. and Möller, T.
STATISTICS. Volume: 52 (2018) - 2018 • 5139
Influence of Feedstock Powder Modification by Heat Treatments on the Properties of APS-Sprayed Al2O3-40% TiO2 Coatings
Berger, L.-M. and Sempf, K. and Sohn, Y.J. and Vaßen, R.
JOURNAL OF THERMAL SPRAY TECHNOLOGY. Volume: 27 (2018) - 2018 • 5138
The Role of Metallic Copper in the Selective Hydrodeoxygenation of Glycerol to 1,2-Propanediol over Cu/ZrO2
Gabrysch, T. and Peng, B. and Bunea, S. and Dyker, G. and Muhler, M.
CHEMCATCHEM. Volume: 10 (2018) - 2018 • 5137
Influence of short-term heat treatment on the mechanical properties of Al–Mg–Si profiles
Kernebeck, S. and Weber, S.
METALS. Volume: 8 (2018) - 2018 • 5136
High-dimensional neural network potentials for solvation: The case of protonated water clusters in helium
Schran, C. and Uhl, F. and Behler, J. and Marx, D.
JOURNAL OF CHEMICAL PHYSICS. Volume: 148 (2018) - 2018 • 5135
TopoFF: MOF structure prediction using specifically optimized blueprints
Keupp, J. and Schmid, R.
FARADAY DISCUSSIONS. Volume: 211 (2018) - 2018 • 5134
Comparative study on the deposition of silicon oxide permeation barrier coatings for polymers using hexamethyldisilazane (HMDSN) and hexamethyldisiloxane (HMDSO)
Mitschker, F. and Schücke, L. and Hoppe, C. and Jaritz, M. and Dahlmann, R. and De Los Arcos, T. and Hopmann, C. and Grundmeier, G. and Awakowicz, P.
JOURNAL OF PHYSICS D: APPLIED PHYSICS. Volume: 51 (2018) - 2018 • 5133
Observation of the generation of multiple electron beams during a single sheath expansion phase in capacitive RF plasmas
Berger, B. and You, K. and Lee, H.-C. and Mussenbrock, T. and Awakowicz, P. and Schulze, J.
PLASMA SOURCES SCIENCE AND TECHNOLOGY. Volume: 27 (2018) - 2018 • 5132
Influence of average ion energy and atomic oxygen flux per Si atom on the formation of silicon oxide permeation barrier coatings on PET
Mitschker, F. and Wißing, J. and Hoppe, C. and De Los Arcos, T. and Grundmeier, G. and Awakowicz, P.
JOURNAL OF PHYSICS D: APPLIED PHYSICS. Volume: 51 (2018) - 2018 • 5131
Spin-photon interface controlled optical switching in a nanobeam waveguide
Schröder, T. and Javadi, A. and Ding, D. and Appel, M.H. and Mahmoodian, S. and Löbl, M.C. and Söllner, I. and Schott, R. and Papon, C. and Pregnolato, T. and Stobbe, S. and Midolo, L. and Wieck, A.D. and Ludwig, Ar. and Warburton, R.J. and Lodahl, P.
OPTICS INFOBASE CONFERENCE PAPERS. Volume: Part F92-CLEO_AT 2018 (2018) - 2018 • 5130
Modeling of microstructures in a cosserat continuum using relaxed energies
Khan, M.S. and Hackl, K.
SPRINGER INDAM SERIES. Volume: 27 (2018) - 2018 • 5129
Persistent correlation between superconductivity and antiferromagnetic fluctuations near a nematic quantum critical point in FeSe1-x Sx
Wiecki, P. and Rana, K. and Böhmer, A.E. and Lee, Y. and Bud'Ko, S.L. and Canfield, P.C. and Furukawa, Y.
PHYSICAL REVIEW B. Volume: 98 (2018) - 2018 • 5128
Metal-free trifluoromethylthiolation of arenediazonium salts with Me4NSCF3
Bertoli, G. and Exner, B. and Evers, M.V. and Tschulik, K. and Gooßen, L.J.
JOURNAL OF FLUORINE CHEMISTRY. Volume: 210 (2018) - 2018 • 5127
Regulatory assessment of drug dissolution profiles comparability via maximum deviation
Moellenhoff, K. and Dette, H. and Kotzagiorgis, E. and Volgushev, S. and Collignon, O.
STATISTICS IN MEDICINE. Volume: 37 (2018) - 2018 • 5126
Parallelization comparison and optimization of a scale-bridging framework to model Cottrell atmospheres
Ganesan, H. and Teijeiro, C. and Sutmann, G.
COMPUTATIONAL MATERIALS SCIENCE. Volume: 155 (2018) - 2018 • 5125
In-plane magnetic penetration depth of superconducting CaKFe4As4
Khasanov, R. and Meier, W.R. and Wu, Y. and Mou, D. and Bud'Ko, S.L. and Eremin, I. and Luetkens, H. and Kaminski, A. and Canfield, P.C. and Amato, A.
PHYSICAL REVIEW B. Volume: 97 (2018) - 2018 • 5124
From Enzymes to Functional Materials—Towards Activation of Small Molecules
Möller, F. and Piontek, S. and Miller, R.G. and Apfel, U.-P.
CHEMISTRY - A EUROPEAN JOURNAL. Volume: 24 (2018) - 2018 • 5123
Common Pitfalls of Catalysis Manuscripts Submitted to Chemistry of Materials
Schüth, F. and Ward, M.D. and Buriak, J.M.
CHEMISTRY OF MATERIALS. Volume: 30 (2018) - 2018 • 5122
MC/MD coupling for scale bridging simulations of solute segregation in solids: An application study
Ganesan, H. and Begau, C. and Sutmann, G.
COMMUNICATIONS IN COMPUTER AND INFORMATION SCIENCE. Volume: 889 (2018) - 2018 • 5121
A direct-view customer-oriented digital holographic camera
Besaga, V.R. and Gerhardt, N.C. and Maksimyak, P.P. and Hofmann, M.R.
PROCEEDINGS OF SPIE - THE INTERNATIONAL SOCIETY FOR OPTICAL ENGINEERING. Volume: 10612 (2018) - 2018 • 5120
Variational approach to interface element modeling of brittle fracture propagation
Khisamitov, I. and Meschke, G.
COMPUTER METHODS IN APPLIED MECHANICS AND ENGINEERING. Volume: 328 (2018) - 2018 • 5119
{110} planar faults in strained bcc metals: Origins and implications of a commonly observed artifact of classical potentials
Möller, J.J. and Mrovec, M. and Bleskov, I. and Neugebauer, J. and Hammerschmidt, T. and Drautz, R. and Elsässer, C. and Hickel, T. and Bitzek, E.
PHYSICAL REVIEW MATERIALS. Volume: 2 (2018) - 2018 • 5118
Tailoring columnar microstructure of axial suspension plasma sprayed TBCs for superior thermal shock performance
Ganvir, A. and Joshi, S. and Markocsan, N. and Vassen, R.
MATERIALS AND DESIGN. Volume: 144 (2018) - 2018 • 5117
Fracture ab initio: A force-based scaling law for atomistically informed continuum models
Möller, J.J. and Bitzek, E. and Janisch, R. and Ul Hassan, H. and Hartmaier, A.
JOURNAL OF MATERIALS RESEARCH. Volume: 33 (2018) - 2018 • 5116
A compact and broadband feeding structure for in-situ plasma probe measurements
Schulz, C. and Rolfes, I.
ASIA-PACIFIC MICROWAVE CONFERENCE PROCEEDINGS, APMC. Volume: (2018) - 2018 • 5115
Numerically efficient microstructure-based calculation of internal stresses in superalloys
Gao, S. and Gogilan, U. and Ma, A. and Hartmaier, A.
MODELLING AND SIMULATION IN MATERIALS SCIENCE AND ENGINEERING. Volume: 26 (2018) - 2018 • 5114
Vibrational properties and magnetic specific heat of the covalent chain antiferromagnet RbFeS e2
Kiiamov, A.G. and Lysogorskiy, Y.V. and Vagizov, F.G. and Tagirov, L.R. and Tayurskii, D.A. and Seidov, Z. and Krug Von Nidda, H.-A. and Tsurkan, V. and Croitori, D. and Günther, A. and Mayr, F. and Loidl, A.
PHYSICAL REVIEW B. Volume: 98 (2018) - 2018 • 5113
Disparity between current and voltage driven capacitively coupled radio frequency discharges
Wilczek, S. and Trieschmann, J. and Schulze, J. and Donkó, Z. and Brinkmann, R.P. and Mussenbrock, T.
PLASMA SOURCES SCIENCE AND TECHNOLOGY. Volume: 27 (2018) - 2018 • 5112
A phenomenological creep model for nickel-base single crystal superalloys at intermediate temperatures
Gao, S. and Wollgramm, P. and Eggeler, G. and Ma, A. and Schreuer, J. and Hartmaier, A.
MODELLING AND SIMULATION IN MATERIALS SCIENCE AND ENGINEERING. Volume: 26 (2018) - 2018 • 5111
Vibrational properties and lattice specific heat of RbFeS2
Kiiamov, A. and Lysogorskiy, Y. and Seidov, Z. and Von Nidda, H.-A.K. and Tsurkan, V. and Tayurskii, D. and Tagirov, L.
AIP CONFERENCE PROCEEDINGS. Volume: 2041 (2018) - 2018 • 5110
High resolution, binder-free investigation of the intrinsic activity of immobilized NiFe LDH nanoparticles on etched carbon nanoelectrodes
Wilde, P. and Barwe, S. and Andronescu, C. and Schuhmann, W. and Ventosa, E.
NANO RESEARCH. Volume: 11 (2018) - 2018 • 5109
Compositional evolution of long-period stacking ordered structures in magnesium studied by atom probe tomography
Kim, J.-K. and Guo, W. and Choi, P.-P. and Raabe, D.
SCRIPTA MATERIALIA. Volume: 156 (2018) - 2018 • 5108
Crystallographic examination of the interaction between texture evolution, mechanically induced martensitic transformation and twinning in nanostructured bainite
Morales-Rivas, L. and Archie, F. and Zaefferer, S. and Benito-Alfonso, M. and Tsai, S.-P. and Yang, J.-R. and Raabe, D. and Garcia-Mateo, C. and Caballero, F.G.
JOURNAL OF ALLOYS AND COMPOUNDS. Volume: 752 (2018) - 2018 • 5107
Interlayer charge transfer in n-modulation doped Al1-xGaxAs-GaAs single heterostructures
Schuster, J. and Kim, T.Y. and Batke, E. and Reuter, D. and Wieck, A.D.
SEMICONDUCTOR SCIENCE AND TECHNOLOGY. Volume: 33 (2018) - 2018 • 5106
Towards Reproducible Fabrication of Nanometre-Sized Carbon Electrodes: Optimisation of Automated Nanoelectrode Fabrication by Means of Transmission Electron Microscopy
Wilde, P. and Quast, T. and Aiyappa, H.B. and Chen, Y.-T. and Botz, A. and Tarnev, T. and Marquitan, M. and Feldhege, S. and Lindner, A. and Andronescu, C. and Schuhmann, W.
CHEMELECTROCHEM. Volume: 5 (2018) - 2018 • 5105
Exceeding 6500 cycles for LiFePO4/Li metal batteries through understanding pulsed charging protocols
García, G. and Dieckhöfer, S. and Schuhmann, W. and Ventosa, E.
JOURNAL OF MATERIALS CHEMISTRY A. Volume: 6 (2018) - 2018 • 5104
Correlative transmission Kikuchi diffraction and atom probe tomography study of Cu(In,Ga)Se2 grain boundaries
Schwarz, T. and Stechmann, G. and Gault, B. and Cojocaru-Mirédin, O. and Wuerz, R. and Raabe, D.
PROGRESS IN PHOTOVOLTAICS: RESEARCH AND APPLICATIONS. Volume: 26 (2018) - 2018 • 5103
On Wigner–Ville Spectra and the Uniqueness of Time-Varying Copula-Based Spectral Densities
Birr, S. and Dette, H. and Hallin, M. and Kley, T. and Volgushev, S.
JOURNAL OF TIME SERIES ANALYSIS. Volume: 39 (2018) - 2018 • 5102
Controlling the amorphous and crystalline state of multinary alloy nanoparticles in an ionic liquid
Garzón-Manjón, A. and Meyer, H. and Grochla, D. and Löffler, T. and Schuhmann, W. and Ludwig, Al. and Scheu, C.
NANOMATERIALS. Volume: 8 (2018) - 2018 • 5101
Experimental and computational investigations of electron dynamics in micro atmospheric pressure radio-frequency plasma jets operated in He/N 2 mixtures
Bischoff, L. and Hübner, G. and Korolov, I. and Donkó, Z. and Hartmann, P. and Gans, T. and Held, J. and Schulz-Von Der Gathen, V. and Liu, Y. and Mussenbrock, T. and Schulze, J.
PLASMA SOURCES SCIENCE AND TECHNOLOGY. Volume: 27 (2018) - 2018 • 5100
Analysis of the micro-processes occurring on different wear protection layers under abrasive and impact wear using laser scanning microscopy [Analyse der Mikroprozesse beim Abrasiv-und Prallverschleiß an unterschiedlichen Verschleißschutzschichten mittels Laser-Scanning-Mikroskopie]
Schwarz, P. and Deuerler, F. and Weber, S. and Peterseim, J.
PRAKTISCHE METALLOGRAPHIE/PRACTICAL METALLOGRAPHY. Volume: 55 (2018) - 2018 • 5099
Influence of spokes on the ionized metal flux fraction in chromium high power impulse magnetron sputtering
Biskup, B. and Maszl, C. and Breilmann, W. and Held, J. and Böke, M. and Benedikt, J. and Von Keudell, A.
JOURNAL OF PHYSICS D: APPLIED PHYSICS. Volume: 51 (2018) - 2018 • 5098
The effect of plasticity on damage evolution using a relaxation-based material model
Schwarz, S. and Hackl, K. and Junker, P.
JOURNAL OF THE MECHANICAL BEHAVIOR OF MATERIALS. Volume: 27 (2018) - 2018 • 5097
Insight into induced charges at metal surfaces and biointerfaces using a polarizable Lennard-Jones potential
Geada, I.L. and Ramezani-Dakhel, H. and Jamil, T. and Sulpizi, M. and Heinz, H.
NATURE COMMUNICATIONS. Volume: 9 (2018) - 2018 • 5096
Unravelling the GLY-PRO-GLU tripeptide induced reconstruction of the Au(110) surface at the molecular scale
Geada, I.L. and Petit, I. and Sulpizi, M. and Tielens, F.
SURFACE SCIENCE. Volume: 677 (2018) - 2018 • 5095
Improved homogeneity of plasma and coating properties using a lance matrix gas distribution in MW-PECVD
Kirchheim, D. and Wilski, S. and Jaritz, M. and Mitschker, F. and Oberberg, M. and Trieschmann, J. and Banko, L. and Brochhagen, M. and Schreckenberg, R. and Hopmann, C. and Böke, M. and Benedikt, J. and de los Arcos, T. and Grundmeier, G. and Grochla, D. and Ludwig, Al. and Mussenbrock, T. and Brinkmann, R.P. and Awakowicz, P. and Dahlmann, R.
JOURNAL OF COATINGS TECHNOLOGY AND RESEARCH. Volume: (2018) - 2018 • 5094
Experimental and Numerical Investigations on Interdiffusion Profiles in Compounds Produced by Sinter-Cladding
Blüm, M. and Theisen, W. and Weber, S.
METALLURGICAL AND MATERIALS TRANSACTIONS A: PHYSICAL METALLURGY AND MATERIALS SCIENCE. Volume: 49 (2018) - 2018 • 5093
NiTi-Based Elastocaloric Cooling on the Macroscale: From Basic Concepts to Realization
Kirsch, S.-M. and Welsch, F. and Michaelis, N. and Schmidt, M. and Wieczorek, A. and Frenzel, J. and Eggeler, G. and Schütze, A. and Seelecke, S.
ENERGY TECHNOLOGY. Volume: 6 (2018) - 2018 • 5092
Computationally Efficient Phase-field Simulation Studies Using RVE Sampling and Statistical Analysis
Schwarze, C. and Darvishi Kamachali, R. and Kühbach, M. and Mießen, C. and Tegeler, M. and Barrales-Mora, L. and Steinbach, I. and Gottstein, G.
COMPUTATIONAL MATERIALS SCIENCE. Volume: 147 (2018) - 2018 • 5091
A combinatorial approach to enhance barrier properties of thin films on polymers: Seeding and capping of PECVD thin films by PEALD
Gebhard, M. and Mitschker, F. and Hoppe, C. and Aghaee, M. and Rogalla, D. and Creatore, M. and Grundmeier, G. and Awakowicz, P. and Devi, A.
PLASMA PROCESSES AND POLYMERS. Volume: 15 (2018) - 2018 • 5090
Hydrogenases: Recent developments and future perspectives
Wittkamp, F. and Senger, M. and Stripp, S.T. and Apfel, U.-P.
CHEMICAL COMMUNICATIONS. Volume: 54 (2018) - 2018 • 5089
Rechargeable, flexible and mediator-free biosupercapacitor based on transparent ITO nanoparticle modified electrodes acting in µM glucose containing buffers
Bobrowski, T. and González Arribas, E. and Ludwig, R. and Toscano, M.D. and Shleev, S. and Schuhmann, W.
BIOSENSORS AND BIOELECTRONICS. Volume: 101 (2018) - 2018 • 5088
PEALD of SiO2 and Al2O3 Thin Films on Polypropylene: Investigations of the Film Growth at the Interface, Stress, and Gas Barrier Properties of Dyads
Gebhard, M. and Mai, L. and Banko, L. and Mitschker, F. and Hoppe, C. and Jaritz, M. and Kirchheim, D. and Zekorn, C. and De Los Arcos, T. and Grochla, D. and Dahlmann, R. and Grundmeier, G. and Awakowicz, P. and Ludwig, Al. and Devi, A.
ACS APPLIED MATERIALS AND INTERFACES. Volume: 10 (2018) - 2018 • 5087
Short-time dynamics in s+is -wave superconductor with incipient bands
Müller, M.A. and Shen, P. and Dzero, M. and Eremin, I.
PHYSICAL REVIEW B. Volume: 98 (2018) - 2018 • 5086
Long-term implantable glucose biosensors
Bobrowski, T. and Schuhmann, W.
CURRENT OPINION IN ELECTROCHEMISTRY. Volume: 10 (2018) - 2018 • 5085
The stability number as a metric for electrocatalyst stability benchmarking
Geiger, S. and Kasian, O. and Ledendecker, M. and Pizzutilo, E. and Mingers, A.M. and Fu, W.T. and Diaz-Morales, O. and Li, Z. and Oellers, T. and Fruchter, L. and Ludwig, Al. and Mayrhofer, K.J.J. and Koper, M.T.M. and Cherevko, S.
NATURE CATALYSIS. Volume: 1 (2018) - 2018 • 5084
Neural network based linearization and control of sputter processes
Woelfel, C. and Kockmann, S. and Awakowicz, P. and Lunze, J.
2017 ASIAN CONTROL CONFERENCE, ASCC 2017. Volume: 2018-January (2018) - 2018 • 5083
Model approximation and stabilization of reactive sputter processes
Woelfel, C. and Bockhorn, D. and Awakowicz, P. and Lunze, J.
JOURNAL OF PROCESS CONTROL. Volume: (2018) - 2018 • 5082
Integration of external electric fields in molecular dynamics simulation models for resistive switching devices
Gergs, T. and Dirkmann, S. and Mussenbrock, T.
JOURNAL OF APPLIED PHYSICS. Volume: 123 (2018) - 2018 • 5081
The influence of operating parameters on the temperature distribution in flighted rotary drums
Seidenbecher, J. and Herz, F. and Specht, E. and Wirtz, S. and Berndt, A. and Scherer, V.
PROCEEDINGS OF THE THERMAL AND FLUIDS ENGINEERING SUMMER CONFERENCE. Volume: 2018-March (2018) - 2018 • 5080
Nematicity, magnetism and superconductivity in FeSe
Böhmer, A.E. and Kreisel, A.
JOURNAL OF PHYSICS CONDENSED MATTER. Volume: 30 (2018) - 2018 • 5079
Continuous Wave Multimode Amplitude THz Spectroscopy
Gerling, A. and Dulme, S. and Schrinski, N. and Stöhr, A. and Hofmann, M.R. and Brenner, C.
INTERNATIONAL CONFERENCE ON INFRARED, MILLIMETER, AND TERAHERTZ WAVES, IRMMW-THZ. Volume: 2018-September (2018) - 2018 • 5078
Numerical and Experimental Study of the Spatial Stress Distribution on the Cornea Surface During a Non-Contact Tonometry Examination
Muench, S. and Roellig, M. and Spoerl, E. and Balzani, D.
EXPERIMENTAL MECHANICS. Volume: (2018) - 2018 • 5077
The convective heat transfer coefficient in flighted rotary drums
Seidenbecher, J. and Meitzner, C. and Herz, F. and Wirtz, S. and Berndt, A. and Scherer, V.
INTERNATIONAL HEAT TRANSFER CONFERENCE. Volume: 2018-August (2018) - 2018 • 5076
High Speed Single Point THz Phase Measurement Based on Dual Channel Lock-in Technique
Gerling, A. and Hofmann, M.R. and Brenner, C.
2018 1ST INTERNATIONAL WORKSHOP ON MOBILE TERAHERTZ SYSTEMS, IWMTS 2018. Volume: (2018) - 2018 • 5075
Subsurface characterization of high-strength high-interstitial austenitic steels after impact wear
Mujica Roncery, L. and Agudo Jácome, L. and Aghajani, A. and Theisen, W. and Weber, S.
WEAR. Volume: 402-403 (2018) - 2018 • 5074
Protonation/reduction dynamics at the [4Fe-4S] cluster of the hydrogen-forming cofactor in [FeFe]-hydrogenases
Senger, M. and Mebs, S. and Duan, J. and Shulenina, O. and Laun, K. and Kertess, L. and Wittkamp, F. and Apfel, U.-P. and Happe, T. and Winkler, M. and Haumann, M. and Stripp, S.T.
PHYSICAL CHEMISTRY CHEMICAL PHYSICS. Volume: 20 (2018) - 2018 • 5073
Pyrolysis and Thermal Annealing of Coal and Biomass in CO2-Rich Atmospheres
Senneca, O. and Apicella, B. and Russo, C. and Cerciello, F. and Salatino, P. and Heuer, S. and Wütscher, A. and Schiemann, M. and Muhler, M. and Scherer, V.
ENERGY AND FUELS. Volume: 32 (2018) - 2018 • 5072
Investigation of heat transfer in a copper-infiltrated tool steel based on measurement, microtomography, and numerical simulation
Klein, S. and Weber, S. and Theisen, W.
MATERIALS AND DESIGN. Volume: 156 (2018) - 2018 • 5071
Effects of CO2 enriched atmosphere on chars from walnut shells pyrolysis in a drop tube reactor
Senneca, O. and Cerciello, F. and Cortese, L. and Heuer, S. and Schiemann, M. and Scherer, V.
FUEL. Volume: 229 (2018) - 2018 • 5070
Simultaneous Opto- and Spectro-Electrochemistry: Reactions of Individual Nanoparticles Uncovered by Dark-Field Microscopy
Wonner, K. and Evers, M.V. and Tschulik, K.
JOURNAL OF THE AMERICAN CHEMICAL SOCIETY. Volume: 140 (2018) - 2018 • 5069
Revising the Concept of Pore Hierarchy for Ionic Transport in Carbon Materials for Supercapacitors
Borchardt, L. and Leistenschneider, D. and Haase, J. and Dvoyashkin, M.
ADVANCED ENERGY MATERIALS. Volume: 8 (2018) - 2018 • 5068
The influence of water and solvent uptake on functional properties of shape-memory polymers
Ghobadi, E. and Marquardt, A. and Zirdehi, E.M. and Neuking, K. and Varnik, F. and Eggeler, G. and Steeb, H.
INTERNATIONAL JOURNAL OF POLYMER SCIENCE. Volume: 2018 (2018) - 2018 • 5067
Boron doped ultrastrong and ductile high-entropy alloys
Seol, J.B. and Bae, J.W. and Li, Z. and Chan Han, J. and Kim, J.G. and Raabe, D. and Kim, H.S.
ACTA MATERIALIA. Volume: 151 (2018) - 2018 • 5066
Methane Hydrate in Confined Spaces: An Alternative Storage System
Borchardt, L. and Casco, M.E. and Silvestre-Albero, J.
CHEMPHYSCHEM. Volume: 19 (2018) - 2018 • 5065
Systematic Investigation on the Influence of Spray Parameters on the Mechanical Properties of Atmospheric Plasma-Sprayed YSZ Coatings
Mutter, M. and Mauer, G. and Mücke, R. and Guillon, O. and Vaßen, R.
JOURNAL OF THERMAL SPRAY TECHNOLOGY. Volume: (2018) - 2018 • 5064
On the Ni-Ion release rate from surfaces of binary NiTi shape memory alloys
Ševčíková, J. and Bártková, D. and Goldbergová, M. and Kuběnová, M. and Čermák, J. and Frenzel, J. and Weiser, A. and Dlouhý, A.
APPLIED SURFACE SCIENCE. Volume: 427 (2018) - 2018 • 5063
Local Activities of Hydroxide and Water Determine the Operation of Silver-Based Oxygen Depolarized Cathodes
Botz, A. and Clausmeyer, J. and Öhl, D. and Tarnev, T. and Franzen, D. and Turek, T. and Schuhmann, W.
ANGEWANDTE CHEMIE - INTERNATIONAL EDITION. Volume: 57 (2018) - 2018 • 5062
Analysis of Energy Dissipation Channels in a Benchmark System of Activated Dissociation: N2 on Ru(0001)
Shakouri, K. and Behler, J. and Meyer, J. and Kroes, G.-J.
JOURNAL OF PHYSICAL CHEMISTRY C. Volume: 122 (2018) - 2018 • 5061
On the nucleation of planar faults during low temperature and high stress creep of single crystal Ni-base superalloys
Wu, X. and Dlouhy, A. and Eggeler, Y.M. and Spiecker, E. and Kostka, A. and Somsen, C. and Eggeler, G.
ACTA MATERIALIA. Volume: 144 (2018) - 2018 • 5060
Tuning Methods for Semiconductor Spin Qubits
Botzem, T. and Shulman, M.D. and Foletti, S. and Harvey, S.P. and Dial, O.E. and Bethke, P. and Cerfontaine, P. and McNeil, R.P.G. and Mahalu, D. and Umansky, V. and Ludwig, Ar. and Wieck, A. and Schuh, D. and Bougeard, D. and Yacoby, A. and Bluhm, H.
PHYSICAL REVIEW APPLIED. Volume: 10 (2018) - 2018 • 5059
Laser metal deposition of lattice structures by columnar built-up
Sharma, M. and Dobbelstein, H. and Thiele, M. and Ostendorf, A.
PROCEDIA CIRP. Volume: 74 (2018) - 2018 • 5058
On the segregation of Re at dislocations in the γ’ phase of Ni-based single crystal superalloys
Wu, X. and Makineni, S.K. and Kontis, P. and Dehm, G. and Raabe, D. and Gault, B. and Eggeler, G.
MATERIALIA. Volume: 4 (2018) - 2018 • 5057
Modifying the nanostructure and the mechanical properties of Mo2BC hard coatings: Influence of substrate temperature during magnetron sputtering
Gleich, S. and Soler, R. and Fager, H. and Bolvardi, H. and Achenbach, J.-O. and Hans, M. and Primetzhofer, D. and Schneider, J.M. and Dehm, G. and Scheu, C.
MATERIALS AND DESIGN. Volume: 142 (2018) - 2018 • 5056
A Charge-Tunable Quantum Dot Deep in the Strong Coupling Regime of Cavity QED
Najer, D. and Sollner, I. and Loebl, M.C. and Riedel, D. and Petrak, B. and Starosielec, S. and Dolique, V. and Valentin, S.R. and Schott, R. and Wieck, A.D. and Ludwig, Ar. and Warburton, R.J.
IEEE PHOTONICS SOCIETY SUMMER TOPICALS MEETING SERIES, SUM 2018. Volume: (2018) - 2018 • 5055
An N-Heterocyclic Carbene Based Silver Precursor for Plasma-Enhanced Spatial Atomic Layer Deposition of Silver Thin Films at Atmospheric Pressure
Boysen, N. and Hasselmann, T. and Karle, S. and Rogalla, D. and Theirich, D. and Winter, M. and Riedl, T. and Devi, A.
ANGEWANDTE CHEMIE - INTERNATIONAL EDITION. Volume: 57 (2018) - 2018 • 5054
Thermal stability of nanocomposite Mo2BC hard coatings deposited by magnetron sputtering
Gleich, S. and Breitbach, B. and Peter, N.J. and Soler, R. and Bolvardi, H. and Schneider, J.M. and Dehm, G. and Scheu, C.
SURFACE AND COATINGS TECHNOLOGY. Volume: 349 (2018) - 2018 • 5053
Impact of asymmetric martensite and austenite nucleation and growth behavior on the phase stability and hysteresis of freestanding shape-memory nanoparticles
Ko, W.-S. and Grabowski, B. and Neugebauer, J.
PHYSICAL REVIEW MATERIALS. Volume: 2 (2018) - 2018 • 5052
Coherent transfer of electron spin correlations assisted by dephasing noise
Nakajima, T. and Delbecq, M.R. and Otsuka, T. and Amaha, S. and Yoneda, J. and Noiri, A. and Takeda, K. and Allison, G. and Ludwig, Ar. and Wieck, A.D. and Hu, X. and Nori, F. and Tarucha, S.
NATURE COMMUNICATIONS. Volume: 9 (2018) - 2018 • 5051
Monitoring Cobalt-Oxide Single Particle Electrochemistry with Subdiffraction Accuracy
Brasiliense, V. and Clausmeyer, J. and Berto, P. and Tessier, G. and Combellas, C. and Schuhmann, W. and Kanoufi, F.
ANALYTICAL CHEMISTRY. Volume: 90 (2018) - 2018 • 5050
Three-Dimensional Branched and Faceted Gold–Ruthenium Nanoparticles: Using Nanostructure to Improve Stability in Oxygen Evolution Electrocatalysis
Gloag, L. and Benedetti, T.M. and Cheong, S. and Li, Y. and Chan, X.-H. and Lacroix, L.-M. and Chang, S.L.Y. and Arenal, R. and Florea, I. and Barron, H. and Barnard, A.S. and Henning, A.M. and Zhao, C. and Schuhmann, W. and Gooding, J.J. and Tilley, R.D.
ANGEWANDTE CHEMIE - INTERNATIONAL EDITION. Volume: 57 (2018) - 2018 • 5049
Investigation of albumin-derived perfluorocarbon-based capsules by holographic optical trapping
Köhler, J. and Ruschke, J. and Ferenz, K.B. and Esen, C. and Kirsch, M. and Ostendorf, A.
BIOMEDICAL OPTICS EXPRESS. Volume: 9 (2018) - 2018 • 5048
Combinatorial Study on Phase Formation and Oxidation in the Thin Film Superalloy Subsystems Co-Al-Cr and Co-Al-Cr-W
Naujoks, D. and Weiser, M. and Salomon, S. and Stein, H. and Virtanen, S. and Ludwig, Al.
ACS COMBINATORIAL SCIENCE. Volume: 20 (2018) - 2018 • 5047
High-field superconductivity on iron chalcogenide FeSe
Shi, A. and Kitagawa, S. and Ishida, K. and Böhmer, A.E. and Meingast, C. and Wolf, T.
JOURNAL OF THE PHYSICAL SOCIETY OF JAPAN. Volume: 87 (2018) - 2018 • 5046
Single Nanoparticle Growth from Nanoparticle Tracking Analysis: From Monte Carlo Simulations to Nanoparticle Electrogeneration
Brasiliense, V. and Noël, J.-M. and Wonner, K. and Tschulik, K. and Combellas, C. and Kanoufi, F.
CHEMELECTROCHEM. Volume: 5 (2018) - 2018 • 5045
Digital holography for the investigation of buried structures with a common-path reflection microscope
Göaring, L. and Finkeldey, M. and Hofmann, M.R. and Gerhardt, N.C.
PROCEEDINGS OF SPIE - THE INTERNATIONAL SOCIETY FOR OPTICAL ENGINEERING. Volume: 10558 (2018) - 2018 • 5044
Bacterial cell division is involved in the damage of gram-negative bacteria on a nano-pillar titanium surface
Köller, M. and Ziegler, N. and Sengstock, C. and Schildhauer, T.A. and Ludwig, Al.
BIOMEDICAL PHYSICS AND ENGINEERING EXPRESS. Volume: 4 (2018) - 2018 • 5043
Pseudogap behavior of the nuclear spin-lattice relaxation rate in FeSe probed by 77Se-NMR
Shi, A. and Arai, T. and Kitagawa, S. and Yamanaka, T. and Ishida, K. and Böhmer, A.E. and Meingast, C. and Wolf, T. and Hirata, M. and Sasaki, T.
JOURNAL OF THE PHYSICAL SOCIETY OF JAPAN. Volume: 87 (2018) - 2018 • 5042
Electrochemical stability of hexagonal tungsten carbide in the potential window of fuel cells and water electrolyzers investigated in a half-cell configuration
Göhl, D. and Mingers, A.M. and Geiger, S. and Schalenbach, M. and Cherevko, S. and Knossalla, J. and Jalalpoor, D. and Schüth, F. and Mayrhofer, K.J.J. and Ledendecker, M.
ELECTROCHIMICA ACTA. Volume: 270 (2018) - 2018 • 5041
High surface area black TiO2 templated from ordered mesoporous carbon for solar driven hydrogen evolution
Xiong, Y. and Gu, D. and Deng, X. and Tüysüz, H. and van Gastel, M. and Schüth, F. and Marlow, F.
MICROPOROUS AND MESOPOROUS MATERIALS. Volume: 268 (2018) - 2018 • 5040
Optical metrology for the investigation of buried technical structures
Göring, L. and Finkeldey, M. and Schellenberg, F. and Brenner, C. and Hofmann, M.R. and Gerhardt, N.C.
TECHNISCHES MESSEN. Volume: 85 (2018) - 2018 • 5039
Microstructure and Mechanical Properties of CMSX-4 Single Crystals Prepared by Additive Manufacturing
Körner, C. and Ramsperger, M. and Meid, C. and Bürger, D. and Wollgramm, P. and Bartsch, M. and Eggeler, G.
METALLURGICAL AND MATERIALS TRANSACTIONS A: PHYSICAL METALLURGY AND MATERIALS SCIENCE. Volume: (2018) - 2018 • 5038
Atomic scale analysis of grain boundary deuteride growth front in Zircaloy-4
Breen, A.J. and Mouton, I. and Lu, W. and Wang, S. and Szczepaniak, A. and Kontis, P. and Stephenson, L.T. and Chang, Y. and da Silva, A.K. and Liebscher, C.H. and Raabe, D. and Britton, T.B. and Herbig, M. and Gault, B.
SCRIPTA MATERIALIA. Volume: 156 (2018) - 2018 • 5037
Temperature dependence of the Gibbs energy of vacancy formation of fcc Ni
Gong, Y. and Grabowski, B. and Glensk, A. and Körmann, F. and Neugebauer, J. and Reed, R.C.
PHYSICAL REVIEW B. Volume: 97 (2018) - 2018 • 5036
On the grain boundary strengthening effect of boron in γ/γ′ Cobalt-base superalloys
Kolb, M. and Freund, L.P. and Fischer, F. and Povstugar, I. and Makineni, S.K. and Gault, B. and Raabe, D. and Müller, J. and Spiecker, E. and Neumeier, S. and Göken, M.
ACTA MATERIALIA. Volume: 145 (2018) - 2018 • 5035
Evolution of dislocation patterns inside the plastic zone introduced by fatigue in an aged aluminium alloy AA2024-T3
Breitbarth, E. and Zaefferer, S. and Archie, F. and Besel, M. and Raabe, D. and Requena, G.
MATERIALS SCIENCE AND ENGINEERING A. Volume: 718 (2018) - 2018 • 5034
Fabrication of perovskite-based porous nanotubes as efficient bifunctional catalyst and application in hybrid lithium-oxygen batteries
Gong, H. and Wang, T. and Guo, H. and Fan, X. and Liu, X. and Song, L. and Xia, W. and Gao, B. and Huang, X. and He, J.
JOURNAL OF MATERIALS CHEMISTRY A. Volume: 6 (2018) - 2018 • 5033
Superresolution Microscopy of Single Rare-Earth Emitters in YAG and H3 Centers in Diamond
Kolesov, R. and Lasse, S. and Rothfuchs, C. and Wieck, A.D. and Xia, K. and Kornher, T. and Wrachtrup, J.
PHYSICAL REVIEW LETTERS. Volume: 120 (2018) - 2018 • 5032
Treatment of Fresh Concrete Surfaces – An Approach to the Evaluation of Appropriate Fresh Concretes
Breitenbücher, R. and Kilinc, H. and Youn-Čale, B.-Y.
BETON- UND STAHLBETONBAU. Volume: 113 (2018) - 2018 • 5031
Thermal cycling testing of TBCs on Cr2AlC MAX phase substrates
Gonzalez-Julian, J. and Go, T. and Mack, D.E. and Vaßen, R.
SURFACE AND COATINGS TECHNOLOGY. Volume: 340 (2018) - 2018 • 5030
On the nature of spillover hydrogen species on platinum/nitrogen-doped mesoporous carbon composites: A temperature-programmed nitrobenzene desorption study
Yang, F. and Hu, B. and Xia, W. and Peng, B. and Shen, J. and Muhler, M.
JOURNAL OF CATALYSIS. Volume: 365 (2018) - 2018 • 5029
Einfluss ermüdungsinduzierter Mikrorisse in Betonbauteilen auf den Eintrag flüssiger Medien
Breitenbücher, R. and Przondziono, R.
BAUTECHNIK. Volume: 95 (2018) - 2018 • 5028
High-temperature oxidation and compressive strength of Cr2AlC MAX phase foams with controlled porosity
Gonzalez-Julian, J. and Onrubia, S. and Bram, M. and Broeckmann, C. and Vassen, R. and Guillon, O.
JOURNAL OF THE AMERICAN CERAMIC SOCIETY. Volume: 101 (2018) - 2018 • 5027
Bond behaviour of thin concrete overlays for maintenance of concrete pavements
Breitenbücher, R. and Schulte-Schrepping, C. and Kunz, S.
MATEC WEB OF CONFERENCES. Volume: 199 (2018) - 2018 • 5026
Environmental resistance of Cr2AlC MAX phase under thermal gradient loading using a burner rig
Gonzalez-Julian, J. and Go, T. and Mack, D.E. and Vaßen, R.
JOURNAL OF THE AMERICAN CERAMIC SOCIETY. Volume: (2018) - 2018 • 5025
Reconstructing disturbance zones ahead of the tunnel face by elastic waveform inversion supported by a parametric level-set representation
Nguyen, L.T. and Nestorović, T.
SOIL DYNAMICS AND EARTHQUAKE ENGINEERING. Volume: 115 (2018) - 2018 • 5024
Optimizing the Acid Resistance of Concrete with Granulated Blast-Furnace Slag
Breitenbücher, R. and Bäcker, J. and Kunz, S. and Ehrenberg, A. and Gerten, C.
MATEC WEB OF CONFERENCES. Volume: 199 (2018) - 2018 • 5023
Comparison of permutationally invariant polynomials, neural networks, and Gaussian approximation potentials in representing water interactions through many-body expansions
Nguyen, T.T. and Székely, E. and Imbalzano, G. and Behler, J. and Csányi, G. and Ceriotti, M. and Götz, A.W. and Paesani, F.
JOURNAL OF CHEMICAL PHYSICS. Volume: 148 (2018) - 2018 • 5022
Spectral Evidence for Emergent Order in Ba1-xNaxFe2As2
Yi, M. and Frano, A. and Lu, D.H. and He, Y. and Wang, M. and Frandsen, B.A. and Kemper, A.F. and Yu, R. and Si, Q. and Wang, L. and He, M. and Hardy, F. and Schweiss, P. and Adelmann, P. and Wolf, T. and Hashimoto, M. and Mo, S.-K. and Hussain, Z. and Le Tacon, M. and Böhmer, A.E. and Lee, D.-H. and Shen, Z.-X. and Meingast, C. and Birgeneau, R.J.
PHYSICAL REVIEW LETTERS. Volume: 121 (2018) - 2018 • 5021
Impact of fatigue-induced microcracks in concrete on the fluid ingress [Einfluss ermüdungsinduzierter Mikrorisse in Betonbauteilen auf den Eintrag flüssiger Medien]
Breitenbücher, R. and Przondziono, R.
BAUTECHNIK. Volume: 95 (2018) - 2018 • 5020
Image inverting, topography and feature size manipulation using organic/inorganic bi-layer lift-off for nanoimprint template
Si, S. and Hoffmann, M.
MICROELECTRONIC ENGINEERING. Volume: 197 (2018) - 2018 • 5019
Selective Solvent-Induced Stabilization of Polar Oxide Surfaces in an Electrochemical Environment
Yoo, S.-H. and Todorova, M. and Neugebauer, J.
PHYSICAL REVIEW LETTERS. Volume: 120 (2018) - 2018 • 5018
Near Infrared Diode Laser THz Systems
Brenner, C. and Hu, Y. and Gwaro, J. and Surkamp, N. and Döpke, B. and Hofmann, M.R. and Kani, B. and Stöhr, A. and Sumpf, B. and Klehr, A. and Fricke, J.
ADVANCES IN RADIO SCIENCE. Volume: 16 (2018) - 2018 • 5017
Microstructural degradation of polycrystalline superalloys from oxidized carbides and implications on crack initiation
Kontis, P. and Collins, D.M. and Wilkinson, A.J. and Reed, R.C. and Raabe, D. and Gault, B.
SCRIPTA MATERIALIA. Volume: 147 (2018) - 2018 • 5016
Unexpected cyclic stress-strain response of dual-phase high-entropy alloys induced by partial reversibility of deformation
Niendorf, T. and Wegener, T. and Li, Z. and Raabe, D.
SCRIPTA MATERIALIA. Volume: 143 (2018) - 2018 • 5015
Assessing the similarity of dose response and target doses in two non-overlapping subgroups
Bretz, F. and Möllenhoff, K. and Dette, H. and Liu, W. and Trampisch, M.
STATISTICS IN MEDICINE. Volume: 37 (2018) - 2018 • 5014
Emissivity Comparison between Chars and Demineralized Coal Chars under Oxycombustion Conditions
Graeser, P. and Wütscher, A. and Cerciello, F. and Muhler, M. and Schiemann, M.
CHEMICAL ENGINEERING AND TECHNOLOGY. Volume: 41 (2018) - 2018 • 5013
The effect of chromium and cobalt segregation at dislocations on nickel-based superalloys
Kontis, P. and Li, Z. and Collins, D.M. and Cormier, J. and Raabe, D. and Gault, B.
SCRIPTA MATERIALIA. Volume: 145 (2018) - 2018 • 5012
Synthesis of rare-earth metal and rare-earth metal-fluoride nanoparticles in ionic liquids and propylene carbonate
Siebels, M. and Mai, L. and Schmolke, L. and Schütte, K. and Barthel, J. and Yue, J. and Thomas, J. and Smarsly, B.M. and Devi, A. and Fischer, R.A. and Janiak, C.
BEILSTEIN JOURNAL OF NANOTECHNOLOGY. Volume: 9 (2018) - 2018 • 5011
Ag-Segregation to Dislocations in PbTe-Based Thermoelectric Materials
Yu, Y. and Zhang, S. and Mio, A.M. and Gault, B. and Sheskin, A. and Scheu, C. and Raabe, D. and Zu, F. and Wuttig, M. and Amouyal, Y. and Cojocaru-Mirédin, O.
ACS APPLIED MATERIALS AND INTERFACES. Volume: 10 (2018) - 2018 • 5010
Solvent-free synthesis of a porous thiophene polymer by mechanochemical oxidative polymerization
Grätz, S. and Oltermann, M. and Troschke, E. and Paasch, S. and Krause, S. and Brunner, E. and Borchardt, L.
JOURNAL OF MATERIALS CHEMISTRY A. Volume: 6 (2018) - 2018 • 5009
Nano-laminated thin film metallic glass design for outstanding mechanical properties
Kontis, P. and Köhler, M. and Evertz, S. and Chen, Y.-T. and Schnabel, V. and Soler, R. and Bednarick, J. and Kirchlechner, C. and Dehm, G. and Raabe, D. and Schneider, J.M. and Gault, B.
SCRIPTA MATERIALIA. Volume: 155 (2018) - 2018 • 5008
Atomic-Scale Explanation of O2 Activation at the Au-TiO2 Interface
Siemer, N. and Lüken, A. and Zalibera, M. and Frenzel, J. and Muñoz-Santiburcio, D. and Savitsky, A. and Lubitz, W. and Muhler, M. and Marx, D. and Strunk, J.
JOURNAL OF THE AMERICAN CHEMICAL SOCIETY. Volume: 140 (2018) - 2018 • 5007
The mechanochemical Scholl reaction-a solvent-free and versatile graphitization tool
Grätz, S. and Beyer, D. and Tkachova, V. and Hellmann, S. and Berger, R. and Feng, X. and Borchardt, L.
CHEMICAL COMMUNICATIONS. Volume: 54 (2018) - 2018 • 5006
The Role of Oxidized Carbides on Thermal-Mechanical Performance of Polycrystalline Superalloys
Kontis, P. and Li, Z. and Segersäll, M. and Moverare, J.J. and Reed, R.C. and Raabe, D. and Gault, B.
METALLURGICAL AND MATERIALS TRANSACTIONS A: PHYSICAL METALLURGY AND MATERIALS SCIENCE. Volume: 49 (2018) - 2018 • 5005
Fluctuation-induced Néel and Bloch skyrmions at topological insulator surfaces
Nogueira, F.S. and Eremin, I. and Katmis, F. and Moodera, J.S. and Van Den Brink, J. and Kravchuk, V.P.
PHYSICAL REVIEW B. Volume: 98 (2018) - 2018 • 5004
Shape-Dependent Dissolution and Cellular Uptake of Silver Nanoparticles
Graf, C. and Nordmeyer, D. and Sengstock, C. and Ahlberg, S. and Diendorf, J. and Raabe, J. and Epple, M. and Köller, M. and Lademann, J. and Vogt, A. and Rancan, F. and Rühl, E.
LANGMUIR. Volume: 34 (2018) - 2018 • 5003
A fast quantum interface between different spin qubit encodings
Noiri, A. and Nakajima, T. and Yoneda, J. and Delbecq, M.R. and Stano, P. and Otsuka, T. and Takeda, K. and Amaha, S. and Allison, G. and Kawasaki, K. and Kojima, Y. and Ludwig, Ar. and Wieck, A.D. and Loss, D. and Tarucha, S.
NATURE COMMUNICATIONS. Volume: 9 (2018) - 2018 • 5002
A combined growth and remodeling framework for the approximation of residual stresses in arterial walls
Zahn, A. and Balzani, D.
ZAMM ZEITSCHRIFT FUR ANGEWANDTE MATHEMATIK UND MECHANIK. Volume: 98 (2018) - 2018 • 5001
Bioelectrocatalytic and electrochemical cascade for phosphate sensing with up to 6 electrons per analyte molecule
Kopiec, G. and Starzec, K. and Kochana, J. and Kinnunen-Skidmore, T.P. and Schuhmann, W. and Campbell, W.H. and Ruff, A. and Plumeré, N.
BIOSENSORS AND BIOELECTRONICS. Volume: 117 (2018) - 2018 • 5000
Parallelized microfluidic diatom accumulation assay to test fouling-release coatings
Nolte, K.A. and Schwarze, J. and Beyer, C.D. and Özcan, O. and Rosenhahn, A.
BIOINTERPHASES. Volume: 13 (2018) - 2018 • 4999
Time-resolved measurement of elemental carbon in urban environment: Comparison of Raman backscattering and aethalometer results
Grafen, M. and Schweiger, G. and Esen, C. and Ostendorf, A.
JOURNAL OF AEROSOL SCIENCE. Volume: 117 (2018) - 2018 • 4998
Modeling of phase equilibria in Ni-H: Bridging the atomistic with the continuum scale
Korbmacher, D. and Von Pezold, J. and Brinckmann, S. and Neugebauer, J. and Hüter, C. and Spatschek, R.
METALS. Volume: 8 (2018) - 2018 • 4997
Dynamic field testing of coating chemistry candidates by a rotating disk system
Nolte, K.A. and Koc, J. and Barros, J.M. and Hunsucker, K. and Schultz, M.P. and Swain, G.W. and Rosenhahn, A.
BIOFOULING. Volume: 34 (2018) - 2018 • 4996
Evaluation and benchmarking of an EC-QCL-based mid-infrared spectrometer for monitoring metabolic blood parameters in critical care units
Grafen, M. and Delbeck, S. and Busch, H. and Heise, H.M. and Ostendorf, A.
PROGRESS IN BIOMEDICAL OPTICS AND IMAGING - PROCEEDINGS OF SPIE. Volume: 10501 (2018) - 2018 • 4995
Proof of Equivalent Catalytic Functionality upon Photon-Induced and Thermal Activation of Supported Isolated Vanadia Species in Methanol Oxidation
Kortewille, B. and Wachs, I.E. and Cibura, N. and Pfingsten, O. and Bacher, G. and Muhler, M. and Strunk, J.
CHEMCATCHEM. Volume: 10 (2018) - 2018 • 4994
Influence of coating thickness on residual stress and adhesion-strength of cold-sprayed Inconel 718 coatings
Singh, R. and Schruefer, S. and Wilson, S. and Gibmeier, J. and Vassen, R.
SURFACE AND COATINGS TECHNOLOGY. Volume: 350 (2018) - 2018 • 4993
Experimental investigations of the magnetic asymmetry effect in capacitively coupled radio frequency plasmas
Oberberg, M. and Kallahn, J. and Awakowicz, P. and Schulze, J.
PLASMA SOURCES SCIENCE AND TECHNOLOGY. Volume: 27 (2018) - 2018 • 4992
Decoupling of ion- and photon-activation mechanisms in polymer surfaces exposed to low-temperature plasmas
Budde, M. and Corbella, C. and Große-Kreul, S. and de los Arcos, T. and Grundmeier, G. and von Keudell, A.
PLASMA PROCESSES AND POLYMERS. Volume: (2018) - 2018 • 4991
Density anomaly of water at negative pressures from first principles
Singraber, A. and Morawietz, T. and Behler, J. and Dellago, C.
JOURNAL OF PHYSICS CONDENSED MATTER. Volume: 30 (2018) - 2018 • 4990
Voltage dependent STM imaging of inorganic adsorbates
Zaum, C. and Morgenstern, K.
APPLIED PHYSICS LETTERS. Volume: 113 (2018) - 2018 • 4989
Deciphering the Surface Composition and the Internal Structure of Alloyed Silver–Gold Nanoparticles
Grasmik, V. and Rurainsky, C. and Loza, K. and Evers, M.V. and Prymak, O. and Heggen, M. and Tschulik, K. and Epple, M.
CHEMISTRY - A EUROPEAN JOURNAL. Volume: 24 (2018) - 2018 • 4988
H2 quantification based on selective pre-concentration and oxidative stripping at Pd modified microelectrodes
Koster, D. and Gutkowski, R. and Masa, J. and Schuhmann, W.
JOURNAL OF ELECTROANALYTICAL CHEMISTRY. Volume: 812 (2018) - 2018 • 4987
Local modification of the surface state properties at dilute coverages: CO/Cu(111)
Zaum, C. and Meyer-Auf-Der-Heide, K.M. and Morgenstern, K.
PHYSICAL REVIEW B. Volume: 97 (2018) - 2018 • 4986
Synthesis and biological characterization of alloyed silver-platinum nanoparticles: From compact core-shell nanoparticles to hollow nanoalloys
Grasmik, V. and Breisch, M. and Loza, K. and Heggen, M. and Köller, M. and Sengstock, C. and Epple, M.
RSC ADVANCES. Volume: 8 (2018) - 2018 • 4985
Imaging orbital-selective quasiparticles in the Hund’s metal state of FeSe
Kostin, A. and Sprau, P.O. and Kreisel, A. and Chong, Y.X. and Böhmer, A.E. and Canfield, P.C. and Hirschfeld, P.J. and Andersen, B.M. and Davis, J.C.S.
NATURE MATERIALS. Volume: 17 (2018) - 2018 • 4984
Understanding the Enhancement of Surface Diffusivity by Dimerization
Zaum, C. and Morgenstern, K.
PHYSICAL REVIEW LETTERS. Volume: 121 (2018) - 2018 • 4983
Influence of stoking on the combustion of beech wood particles of different shape in an agitated bed
Buß, F. and Wirtz, S. and Scherer, V.
EXPERIMENTAL THERMAL AND FLUID SCIENCE. Volume: 95 (2018) - 2018 • 4982
Theory of spin inertia in singly charged quantum dots
Smirnov, D.S. and Zhukov, E.A. and Kirstein, E. and Yakovlev, D.R. and Reuter, D. and Wieck, A.D. and Bayer, M. and Greilich, A. and Glazov, M.M.
PHYSICAL REVIEW B. Volume: 98 (2018) - 2018 • 4981
Molecular engineering of Ga-ketoiminates: Synthesis, structure and evaluation as precursors for the additive-free spin-coated deposition of gallium oxide thin films
O'Donoghue, R. and Rahman, S. and Mallick, B. and Winter, M. and Rogalla, D. and Becker, H.-W. and Devi, A.
NEW JOURNAL OF CHEMISTRY. Volume: 42 (2018) - 2018 • 4980
Utilization of the catalyst layer of dimensionally stable anodes. Part 2: Impact of spatial current distribution on electrocatalytic performance
Zeradjanin, A.R. and Ventosa, E. and Masa, J. and Schuhmann, W.
JOURNAL OF ELECTROANALYTICAL CHEMISTRY. Volume: 828 (2018) - 2018 • 4979
Oxygen Reduction Activity and Reversible Deactivation of Single Silver Nanoparticles during Particle Adsorption Events
Öhl, D. and Clausmeyer, J. and Barwe, S. and Botz, A. and Schuhmann, W.
CHEMELECTROCHEM. Volume: 5 (2018) - 2018 • 4978
Mechanical properties of sprayed overlayers on superalloy substrates, obtained via indentation testing
Campbell, J.E. and Kalfhaus, T. and Vassen, R. and Thompson, R.P. and Dean, J. and Clyne, T.W.
ACTA MATERIALIA. Volume: 154 (2018) - 2018 • 4977
Multiple slip dislocation patterning in a dislocation-based crystal plasticity finite element method
Grilli, N. and Janssens, K.G.F. and Nellessen, J. and Sandlöbes, S. and Raabe, D.
INTERNATIONAL JOURNAL OF PLASTICITY. Volume: 100 (2018) - 2018 • 4976
Optimized Ag Nanovoid Structures for Probing Electrocatalytic Carbon Dioxide Reduction Using Operando Surface-Enhanced Raman Spectroscopy
Öhl, D. and Kayran, Y.U. and Junqueira, J.R.C. and Eßmann, V. and Bobrowski, T. and Schuhmann, W.
LANGMUIR. Volume: 34 (2018) - 2018 • 4975
Fracture toughness of Mo2BC thin films: Intrinsic toughness versus system toughening
Soler, R. and Gleich, S. and Kirchlechner, C. and Scheu, C. and Schneider, J.M. and Dehm, G.
MATERIALS AND DESIGN. Volume: 154 (2018) - 2018 • 4974
In situ TEM observation of rebonding on fractured silicon carbide
Zhang, Z. and Cui, J. and Wang, B. and Jiang, H. and Chen, G. and Yu, J. and Lin, C. and Tang, C. and Hartmaier, A. and Zhang, J. and Luo, J. and Rosenkranz, A. and Jiang, N. and Guo, D.
NANOSCALE. Volume: 10 (2018) - 2018 • 4973
Testing of Ni-base superalloy single crystals with circular notched miniature tensile creep (CNMTC) specimens
Cao, L. and Bürger, D. and Wollgramm, P. and Neuking, K. and Eggeler, G.
MATERIALS SCIENCE AND ENGINEERING A. Volume: 712 (2018) - 2018 • 4972
Si micro-cantilever sensor chips for space-resolved stress measurements in physical and plasma-enhanced chemical vapour deposition
Grochla, D. and Banko, L. and Pfetzing-Micklich, J. and Behm, H. and Dahlmann, R. and Ludwig, Al.
SENSORS AND ACTUATORS, A: PHYSICAL. Volume: 270 (2018) - 2018 • 4971
Antiferromagnetic order in CaK(Fe1-xNix)4As4 and its interplay with superconductivity
Kreyssig, A. and Wilde, J.M. and Böhmer, A.E. and Tian, W. and Meier, W.R. and Li, B. and Ueland, B.G. and Xu, M. and Bud'Ko, S.L. and Canfield, P.C. and McQueeney, R.J. and Goldman, A.I.
PHYSICAL REVIEW B. Volume: 97 (2018) - 2018 • 4970
Microstructure and mechanical properties in the thin film system Cu-Zr
Oellers, T. and Raghavan, R. and Chakraborty, J. and Kirchlechner, C. and Kostka, A. and Liebscher, C.H. and Dehm, G. and Ludwig, Al.
THIN SOLID FILMS. Volume: 645 (2018) - 2018 • 4969
Microstructural and mechanical characterization of an equiatomic YGdTbDyHo high entropy alloy with hexagonal close-packed structure
Soler, R. and Evirgen, A. and Yao, M. and Kirchlechner, C. and Stein, F. and Feuerbacher, M. and Raabe, D. and Dehm, G.
ACTA MATERIALIA. Volume: 156 (2018) - 2018 • 4968
Calculating free energies of point defects from ab initio
Zhang, X. and Grabowski, B. and Hickel, T. and Neugebauer, J.
COMPUTATIONAL MATERIALS SCIENCE. Volume: 148 (2018) - 2018 • 4967
A fuzzy surrogate modelling approach for real-time predictions in mechanised tunnelling
Cao, B.T. and Freitag, S. and Meschke, G.
INTERNATIONAL JOURNAL OF RELIABILITY AND SAFETY. Volume: 12 (2018) - 2018 • 4966
How evolving multiaxial stress states affect the kinetics of rafting during creep of single crystal Ni-base superalloys
Cao, L. and Wollgramm, P. and Bürger, D. and Kostka, A. and Cailletaud, G. and Eggeler, G.
ACTA MATERIALIA. Volume: 158 (2018) - 2018 • 4965
Shear-density coupling for a compressible single-component yield-stress fluid
Gross, M. and Varnik, F.
SOFT MATTER. Volume: 14 (2018) - 2018 • 4964
Nanocasting in ball mills - Combining ultra-hydrophilicity and ordered mesoporosity in carbon materials
Zhang, E. and Hao, G.-P. and Casco, M.E. and Bon, V. and Grätz, S. and Borchardt, L.
JOURNAL OF MATERIALS CHEMISTRY A. Volume: 6 (2018) - 2018 • 4963
Origins of the Inverse Electrocaloric Effect
Grünebohm, A. and Ma, Y.-B. and Marathe, M. and Xu, B.-X. and Albe, K. and Kalcher, C. and Meyer, K.-C. and Shvartsman, V.V. and Lupascu, D.C. and Ederer, C.
ENERGY TECHNOLOGY. Volume: 6 (2018) - 2018 • 4962
Mechanochemical synthesis of porous carbon at room temperature with a highly ordered sp2 microstructure
Casco, M.E. and Badaczewski, F. and Grätz, S. and Tolosa, A. and Presser, V. and Smarsly, B.M. and Borchardt, L.
CARBON. Volume: 139 (2018) - 2018 • 4961
Temperature dependence of the stacking-fault Gibbs energy for Al, Cu, and Ni
Zhang, X. and Grabowski, B. and Körmann, F. and Ruban, A.V. and Gong, Y. and Reed, R.C. and Hickel, T. and Neugebauer, J.
PHYSICAL REVIEW B. Volume: 98 (2018) - 2018 • 4960
Monitoring the formation of PtNi nanoalloys supported on hollow graphitic spheres using: In situ pair distribution function analysis
Ortatatli, Ş. and Knossalla, J. and Schüth, F. and Weidenthaler, C.
PHYSICAL CHEMISTRY CHEMICAL PHYSICS. Volume: 20 (2018) - 2018 • 4959
Coherent transmission of superconducting carriers through a ∼2 μm polar semiconductor
Chakraborti, H. and Deb, S. and Schott, R. and Thakur, V. and Chatterjee, A. and Yadav, S. and Saroj, R.K. and Wieck, A. and Shivaprasad, S.M. and Das Gupta, K. and Dhar, S.
SUPERCONDUCTOR SCIENCE AND TECHNOLOGY. Volume: 31 (2018) - 2018 • 4958
Parameter free quantitative analysis of atom probe data by correlation functions: Application to the precipitation in Al-Zn-Mg-Cu
Zhao, H. and Gault, B. and Ponge, D. and Raabe, D. and De Geuser, F.
SCRIPTA MATERIALIA. Volume: 154 (2018) - 2018 • 4957
Segregation assisted grain boundary precipitation in a model Al-Zn-Mg-Cu alloy
Zhao, H. and De Geuser, F. and Kwiatkowski da Silva, A. and Szczepaniak, A. and Gault, B. and Ponge, D. and Raabe, D.
ACTA MATERIALIA. Volume: 156 (2018) - 2018 • 4956
Combinatorial Synthesis and High-Throughput Characterization of Fe-V-O Thin-Film Materials Libraries for Solar Water Splitting
Kumari, S. and Gutkowski, R. and Junqueira, J.R.C. and Kostka, A. and Hengge, K. and Scheu, C. and Schuhmann, W. and Ludwig, Al.
ACS COMBINATORIAL SCIENCE. Volume: 20 (2018) - 2018 • 4955
Light-induced formation of partially reduced oxygen species limits the lifetime of photosystem 1-based biocathodes
Zhao, F. and Hardt, S. and Hartmann, V. and Zhang, H. and Nowaczyk, M.M. and Rögner, M. and Plumeré, N. and Schuhmann, W. and Conzuelo, F.
NATURE COMMUNICATIONS. Volume: 9 (2018) - 2018 • 4954
Computational modeling of fiber flow during casting of fresh concrete
Gudžulić, V. and Dang, T.S. and Meschke, G.
COMPUTATIONAL MECHANICS. Volume: (2018) - 2018 • 4953
Phase-field modeling of pores and precipitates in polycrystalline systems
Kundin, J. and Sohaib, H. and Schiedung, R. and Steinbach, I.
MODELLING AND SIMULATION IN MATERIALS SCIENCE AND ENGINEERING. Volume: 26 (2018) - 2018 • 4952
Unravelling electron transfer processes at photosystem 2 embedded in an Os-complex modified redox polymer
Zhao, F. and Hartmann, V. and Ruff, A. and Nowaczyk, M.M. and Rögner, M. and Schuhmann, W. and Conzuelo, F.
ELECTROCHIMICA ACTA. Volume: 290 (2018) - 2018 • 4951
Building Information Modelling in mechanised shield tunnelling – A practitioner's outlook to the near future
Stascheit, J. and Ninić, J. and Meschke, G. and Hegemann, F. and Maidl, U.
GEOMECHANIK UND TUNNELBAU. Volume: 11 (2018) - 2018 • 4950
Competition between formation of carbides and reversed austenite during tempering of a medium-manganese steel studied by thermodynamic-kinetic simulations and atom probe tomography
Kwiatkowski da Silva, A. and Inden, G. and Kumar, A. and Ponge, D. and Gault, B. and Raabe, D.
ACTA MATERIALIA. Volume: 147 (2018) - 2018 • 4949
Dual properties of a hydrogen oxidation Ni-catalyst entrapped within a polymer promote self-defense against oxygen /639/638/77/886 /639/638/161/893 /639/638/675 /120 /128 /140/131 article
Oughli, A.A. and Ruff, A. and Boralugodage, N.P. and Rodríguez-Maciá, P. and Plumeré, N. and Lubitz, W. and Shaw, W.J. and Schuhmann, W. and Rüdiger, O.
NATURE COMMUNICATIONS. Volume: 9 (2018) - 2018 • 4948
Characterizing solute hydrogen and hydrides in pure and alloyed titanium at the atomic scale
Chang, Y. and Breen, A.J. and Tarzimoghadam, Z. and Kürnsteiner, P. and Gardner, H. and Ackerman, A. and Radecka, A. and Bagot, P.A.J. and Lu, W. and Li, T. and Jägle, E.A. and Herbig, M. and Stephenson, L.T. and Moody, M.P. and Rugg, D. and Dye, D. and Ponge, D. and Raabe, D. and Gault, B.
ACTA MATERIALIA. Volume: 150 (2018) - 2018 • 4947
Manipulation of matter by electric and magnetic fields: Toward novel synthesis and processing routes of inorganic materials
Guillon, O. and Elsässer, C. and Gutfleisch, O. and Janek, J. and Korte-Kerzel, S. and Raabe, D. and Volkert, C.A.
MATERIALS TODAY. Volume: 21 (2018) - 2018 • 4946
Phase nucleation through confined spinodal fluctuations at crystal defects evidenced in Fe-Mn alloys
Kwiatkowski Da Silva, A. and Ponge, D. and Peng, Z. and Inden, G. and Lu, Y. and Breen, A. and Gault, B. and Raabe, D.
NATURE COMMUNICATIONS. Volume: 9 (2018) - 2018 • 4945
Viologen-modified electrodes for protection of hydrogenases from high potential inactivation while performing H2 oxidation at low overpotential
Oughli, A.A. and Vélez, M. and Birrell, J.A. and Schuhmann, W. and Lubitz, W. and Plumeré, N. and Rüdiger, O.
DALTON TRANSACTIONS. Volume: 47 (2018) - 2018 • 4944
Spin inertia of resident and photoexcited carriers in singly charged quantum dots
Zhukov, E.A. and Kirstein, E. and Smirnov, D.S. and Yakovlev, D.R. and Glazov, M.M. and Reuter, D. and Wieck, A.D. and Bayer, M. and Greilich, A.
PHYSICAL REVIEW B. Volume: 98 (2018) - 2018 • 4943
A polytree-based adaptive polygonal finite element method for multi-material topology optimization
Chau, K.N. and Chau, K.N. and Ngo, T. and Hackl, K. and Nguyen-Xuan, H.
COMPUTER METHODS IN APPLIED MECHANICS AND ENGINEERING. Volume: 332 (2018) - 2018 • 4942
In situ atomic-scale observation of oxidation and decomposition processes in nanocrystalline alloys
Guo, J. and Haberfehlner, G. and Rosalie, J. and Li, L. and Duarte, M.J. and Kothleitner, G. and Dehm, G. and He, Y. and Pippan, R. and Zhang, Z.
NATURE COMMUNICATIONS. Volume: 9 (2018) - 2018 • 4941
Nonlinear observer-based recurrent wavelet neuro-controller in disturbance rejection control of flexible structures
Oveisi, A. and Jeronimo, M.B. and Nestorović, T.
ENGINEERING APPLICATIONS OF ARTIFICIAL INTELLIGENCE. Volume: 69 (2018) - 2018 • 4940
Robust nonfragile observer-based H2/H∞ controller
Oveisi, A. and Nestorović, T.
JVC/JOURNAL OF VIBRATION AND CONTROL. Volume: 24 (2018) - 2018 • 4939
Molecular statics simulation of CdTe grain boundary structures and energetics using a bond-order potential
Stechmann, G. and Zaefferer, S. and Raabe, D.
MODELLING AND SIMULATION IN MATERIALS SCIENCE AND ENGINEERING. Volume: 26 (2018) - 2018 • 4938
Hot cracking mechanism affecting a non-weldable Ni-based superalloy produced by selective electron Beam Melting
Chauvet, E. and Kontis, P. and Jägle, E.A. and Gault, B. and Raabe, D. and Tassin, C. and Blandin, J.-J. and Dendievel, R. and Vayre, B. and Abed, S. and Martin, G.
ACTA MATERIALIA. Volume: 142 (2018) - 2018 • 4937
PH-Dependence in facet-selective photo-deposition of metals and metal oxides on semiconductor particles
Guo, Y. and Siretanu, I. and Zhang, Y. and Mei, B. and Li, X. and Mugele, F. and Huang, H. and Mul, G.
JOURNAL OF MATERIALS CHEMISTRY A. Volume: 6 (2018) - 2018 • 4936
Observer-based repetitive model predictive control in active vibration suppression
Oveisi, A. and Hosseini-Pishrobat, M. and Nestorović, T. and Keighobadi, J.
STRUCTURAL CONTROL AND HEALTH MONITORING. Volume: 25 (2018) - 2018 • 4935
Misorientation distribution between martensite and austenite in Fe-31 wt%Ni-0.01 wt%C
Zilnyk, K.D. and Almeida Junior, D.R. and Sandim, H.R.Z. and Rios, P.R. and Raabe, D.
ACTA MATERIALIA. Volume: 143 (2018) - 2018 • 4934
Mechanical twinning induced alteration in the kinetics of martensitic phase transformation in TRIP-maraging steels
Gupta, S. and Ma, A. and Hartmaier, A.
INTERNATIONAL JOURNAL OF SOLIDS AND STRUCTURES. Volume: 155 (2018) - 2018 • 4933
On the origin of the improvement of shape memory effect by precipitating VC in Fe–Mn–Si-based shape memory alloys
Lai, M.J. and Li, Y.J. and Lillpopp, L. and Ponge, D. and Will, S. and Raabe, D.
ACTA MATERIALIA. Volume: 155 (2018) - 2018 • 4932
Finite element-based software-in-the-loop for offline post-processing and real-time simulations
Oveisi, A. and Sukhairi, T.A. and Nestorović, T.
STRUCTURAL ENGINEERING AND MECHANICS. Volume: 67 (2018) - 2018 • 4931
Application of High-Throughput Seebeck Microprobe Measurements on Thermoelectric Half-Heusler Thin Film Combinatorial Material Libraries
Ziolkowski, P. and Wambach, M. and Ludwig, Al. and Mueller, E.
ACS COMBINATORIAL SCIENCE. Volume: 20 (2018) - 2018 • 4930
Local dynamics of copper active sites in zeolite catalysts for selective catalytic reduction of NOx with NH3
Chen, P. and Khetan, A. and Jabłońska, M. and Simböck, J. and Muhler, M. and Palkovits, R. and Pitsch, H. and Simon, U.
APPLIED CATALYSIS B: ENVIRONMENTAL. Volume: 237 (2018) - 2018 • 4929
Precipitate-induced nonlinearities of diffusion along grain boundaries in Al-based alloys
Gupta, A. and Kulitcki, V. and Kavakbasi, B.T. and Buranova, Y. and Neugebauer, J. and Wilde, G. and Hickel, T. and Divinski, S.V.
PHYSICAL REVIEW MATERIALS. Volume: 2 (2018) - 2018 • 4928
ω phase acts as a switch between dislocation channeling and joint twinning- and transformation-induced plasticity in a metastable β titanium alloy
Lai, M.J. and Li, T. and Raabe, D.
ACTA MATERIALIA. Volume: 151 (2018) - 2018 • 4927
Optimal Input Excitation Design for Nonparametric Uncertainty Quantification of Multi-Input Multi-Output Systems
Oveisi, A. and Anderson, A. and Nestorović, T. and Montazeri, A.
IFAC-PAPERSONLINE. Volume: 51 (2018) - 2018 • 4926
Deformation mechanisms, activated slip systems and critical resolved shear stresses in an Mg-LPSO alloy studied by micro-pillar compression
Chen, R. and Sandlöbes, S. and Zehnder, C. and Zeng, X. and Korte-Kerzel, S. and Raabe, D.
MATERIALS AND DESIGN. Volume: 154 (2018) - 2018 • 4925
Frequency Domain Subspace Identification of Multivariable Dynamical Systems for Robust Control Design
Oveisi, A. and Nestorović, T. and Montazeri, A.
IFAC-PAPERSONLINE. Volume: 51 (2018) - 2018 • 4924
Molecular Dynamics Investigation of the Dielectric Decrement of Ion Solutions
Pache, D. and Schmid, R.
CHEMELECTROCHEM. Volume: 5 (2018) - 2018 • 4923
Printing structural colors via direct laser writing
Zyla, G. and Kovalev, A. and Gurevich, E.L. and Esen, C. and Ostendorf, A. and Gorb, S.
PROCEEDINGS OF SPIE - THE INTERNATIONAL SOCIETY FOR OPTICAL ENGINEERING. Volume: 10544 (2018) - 2018 • 4922
Compact continuous wave THz source based on monolithic two-color laser diode
Gwaro, J.O. and Brenner, C. and Sumpf, B. and Klehr, A. and Fricke, J. and Hofmann, M.R.
PROCEEDINGS OF SPIE - THE INTERNATIONAL SOCIETY FOR OPTICAL ENGINEERING. Volume: 10684 (2018) - 2018 • 4921
High-resolution 3D light fluence mapping for heterogeneous scattering media by localized sampling
Cherkashin, M.N. and Brenner, C. and Hofmann, M.R.
APPLIED OPTICS. Volume: 57 (2018) - 2018 • 4920
Elemental segregation to twin boundaries in a MnAl ferromagnetic Heusler alloy
Palanisamy, D. and Raabe, D. and Gault, B.
SCRIPTA MATERIALIA. Volume: 155 (2018) - 2018 • 4919
Charge Carrier Lifetimes in Cr-Fe-Al-O Thin Films
Stein, H.S. and Müller, S. and Schwarzburg, K. and Friedrich, D. and Ludwig, Al. and Eichberger, R.
ACS APPLIED MATERIALS AND INTERFACES. Volume: 10 (2018) - 2018 • 4918
Ingredients for the electronic nematic phase in FeSe revealed by its anisotropic optical response
Chinotti, M. and Pal, A. and Degiorgi, L. and Böhmer, A.E. and Canfield, P.C.
PHYSICAL REVIEW B. Volume: 98 (2018) - 2018 • 4917
Using controlled disorder to probe the interplay between charge order and superconductivity in NbSe2
Cho, K. and Kończykowski, M. and Teknowijoyo, S. and Tanatar, M.A. and Guss, J. and Gartin, P.B. and Wilde, J.M. and Kreyssig, A. and McQueeney, R.J. and Goldman, A.I. and Mishra, V. and Hirschfeld, P.J. and Prozorov, R.
NATURE COMMUNICATIONS. Volume: 9 (2018) - 2018 • 4916
Thermal activation parameters of plastic flow reveal deformation mechanisms in the CrMnFeCoNi high-entropy alloy
Laplanche, G. and Bonneville, J. and Varvenne, C. and Curtin, W.A. and George, E.P.
ACTA MATERIALIA. Volume: 143 (2018) - 2018 • 4915
On the nature of twin boundary-associated strengthening in Fe-Mn-C steel
Choi, W.S. and Sandlöbes, S. and Malyar, N.V. and Kirchlechner, C. and Korte-Kerzel, S. and Dehm, G. and Choi, P.-P. and Raabe, D.
SCRIPTA MATERIALIA. Volume: 156 (2018) - 2018 • 4914
Elastic moduli and thermal expansion coefficients of medium-entropy subsystems of the CrMnFeCoNi high-entropy alloy
Laplanche, G. and Gadaud, P. and Bärsch, C. and Demtröder, K. and Reinhart, C. and Schreuer, J. and George, E.P.
JOURNAL OF ALLOYS AND COMPOUNDS. Volume: 746 (2018) - 2018 • 4913
Carbon onion/sulfur hybrid cathodes: Via inverse vulcanization for lithium-sulfur batteries
Choudhury, S. and Srimuk, P. and Raju, K. and Tolosa, A. and Fleischmann, S. and Zeiger, M. and Ozoemena, K.I. and Borchardt, L. and Presser, V.
SUSTAINABLE ENERGY AND FUELS. Volume: 2 (2018) - 2018 • 4912
Phase stability and kinetics of σ-phase precipitation in CrMnFeCoNi high-entropy alloys
Laplanche, G. and Berglund, S. and Reinhart, C. and Kostka, A. and Fox, F. and George, E.P.
ACTA MATERIALIA. Volume: 161 (2018) - 2018 • 4911
On the influence of crystallography and dendritic microstructure on micro shear behavior of single crystal Ni-based superalloys
Laplanche, G. and Wieczorek, N. and Fox, F. and Berglund, S. and Pfetzing-Micklich, J. and Kishida, K. and Inui, H. and Eggeler, G.
ACTA MATERIALIA. Volume: 160 (2018) - 2018 • 4910
Increased Acid Dissociation at the Quartz/Water Interface
Parashar, S. and Lesnicki, D. and Sulpizi, M.
JOURNAL OF PHYSICAL CHEMISTRY LETTERS. Volume: 9 (2018) - 2018 • 4909
Anomalous Phonon Lifetime Shortening in Paramagnetic CrN Caused by Spin-Lattice Coupling: A Combined Spin and Ab Initio Molecular Dynamics Study
Stockem, I. and Bergman, A. and Glensk, A. and Hickel, T. and Körmann, F. and Grabowski, B. and Neugebauer, J. and Alling, B.
PHYSICAL REVIEW LETTERS. Volume: 121 (2018) - 2018 • 4908
Migration mechanisms of a faceted grain boundary
Hadian, R. and Grabowski, B. and Finnis, M.W. and Neugebauer, J.
PHYSICAL REVIEW MATERIALS. Volume: 2 (2018) - 2018 • 4907
Experimental and numerical research on damage localization in plate-like concrete structures using hybrid approach
Stojić, D. and Nestorović, T. and Marković, N. and Marjanović, M.
STRUCTURAL CONTROL AND HEALTH MONITORING. Volume: 25 (2018) - 2018 • 4906
Atomic/molecular layer deposition of Cu-organic thin films
Hagen, D.J. and Mai, L. and Devi, A. and Sainio, J. and Karppinen, M.
DALTON TRANSACTIONS. Volume: 47 (2018) - 2018 • 4905
Martensite aging in 〈0 0 1〉 oriented Co49Ni21Ga30 single crystals in tension
Lauhoff, C. and Krooß, P. and Langenkämper, D. and Somsen, C. and Eggeler, G. and Kireeva, I. and Chumlyakov, Y.I. and Niendorf, T.
FUNCTIONAL MATERIALS LETTERS. Volume: 11 (2018) - 2018 • 4904
Spectroscopical investigations on the redox chemistry of [FeFe]-hydrogenases in the presence of carbon monoxide
Laun, K. and Mebs, S. and Duan, J. and Wittkamp, F. and Apfel, U.-P. and Happe, T. and Winkler, M. and Haumann, M. and Stripp, S.T.
MOLECULES. Volume: 23 (2018) - 2018 • 4903
Connection between target poisoning and current waveforms in reactive high-power impulse magnetron sputtering of chromium
Layes, V. and Corbella, C. and Monjé, S. and Schulz-Von Der Gathen, V. and Von Keudell, A. and De Los Arcos, T.
PLASMA SOURCES SCIENCE AND TECHNOLOGY. Volume: 27 (2018) - 2018 • 4902
Simultaneous measurement of infrared absorption coefficient of Carbon doped Al0.33Ga0.67As thin film and thermal boundary resistance between thin film and heavily Zn doped GaAs substrate using spectrally-resolved modulated photothermal infrared radiometry
Pawlak, M. and Horny, N. and Scholz, S. and Ebler, C. and Ludwig, Ar. and Wieck, A.D.
THERMOCHIMICA ACTA. Volume: 667 (2018) - 2018 • 4901
Simultaneous measurement of thermal conductivity and diffusivity of an undoped Al0.33Ga0.67As thin film epitaxially grown on a heavily Zn doped GaAs using spectrally-resolved modulated photothermal infrared radiometry
Pawlak, M. and Pal, S. and Scholz, S. and Ludwig, Ar. and Wieck, A.D.
THERMOCHIMICA ACTA. Volume: 662 (2018) - 2018 • 4900
CVD-grown copper tungstate thin films for solar water splitting
Peeters, D. and Mendoza Reyes, O. and Mai, L. and Sadlo, A. and Cwik, S. and Rogalla, D. and Becker, H.-W. and Schütz, H.M. and Hirst, J. and Müller, S. and Friedrich, D. and Mitoraj, D. and Nagli, M. and Toroker, M.C. and Eichberger, R. and Beranek, R. and Devi, A.
JOURNAL OF MATERIALS CHEMISTRY A. Volume: 6 (2018) - 2018 • 4899
Function portability of molecular dynamics on heterogeneous parallel architectures with OpenCL
Halver, R. and Homberg, W. and Sutmann, G.
JOURNAL OF SUPERCOMPUTING. Volume: 74 (2018) - 2018 • 4898
Benchmarking molecular dynamics with OpenCL on many-core architectures
Halver, R. and Homberg, W. and Sutmann, G.
LECTURE NOTES IN COMPUTER SCIENCE (INCLUDING SUBSERIES LECTURE NOTES IN ARTIFICIAL INTELLIGENCE AND LECTURE NOTES IN BIOINFORMATICS). Volume: 10778 LNCS (2018) - 2018 • 4897
Dislocation multiplication in stage II deformation of fcc multi-slip single crystals
Stricker, M. and Sudmanns, M. and Schulz, K. and Hochrainer, T. and Weygand, D.
JOURNAL OF THE MECHANICS AND PHYSICS OF SOLIDS. Volume: 119 (2018) - 2018 • 4896
Temperature-induced transformations and martensitic reorientation processes in ultra-fine-grained Ni rich pseudoelastic NiTi wires studied by electrical resistance
Pelegrina, J.L. and Olbricht, J. and Yawny, A. and Eggeler, G.
JOURNAL OF ALLOYS AND COMPOUNDS. Volume: 735 (2018) - 2018 • 4895
Local Surface Modifications Investigated by Combining Scanning Electrochemical Microscopy and Surface-Enhanced Raman Scattering
Clausmeyer, J. and Nebel, M. and Grützke, S. and Kayran, Y.U. and Schuhmann, W.
CHEMPLUSCHEM. Volume: 83 (2018) - 2018 • 4894
Spin-orbit coupling, minimal model and potential Cooper-pairing from repulsion in BiS2-superconductors
Cobo-Lopez, S. and Bahramy, M.S. and Arita, R. and Akbari, A. and Eremin, I.
NEW JOURNAL OF PHYSICS. Volume: 20 (2018) - 2018 • 4893
On the detection of multiple events in atom probe tomography
Peng, Z. and Vurpillot, F. and Choi, P.-P. and Li, Y. and Raabe, D. and Gault, B.
ULTRAMICROSCOPY. Volume: 189 (2018) - 2018 • 4892
Structure and Chemical Organization in Damselfly Calopteryx haemorrhoidalis Wings: A Spatially Resolved FTIR and XRF Analysis with Synchrotron Radiation
Stuhr, S. and Truong, V.K. and Vongsvivut, J. and Senkbeil, T. and Yang, Y. and Al Kobaisi, M. and Baulin, V.A. and Werner, M. and Rubanov, S. and Tobin, M.J. and Cloetens, P. and Rosenhahn, A. and Lamb, R.N. and Luque, P. and Marchant, R. and Ivanova, E.P.
SCIENTIFIC REPORTS. Volume: 8 (2018) - 2018 • 4891
Equivalence analyses of dissolution profiles with the Mahalanobis distance: a regulatory perspective and a comparison with a parametric maximum deviation-based approach
Collignon, O. and Moellenhoff, K. and Dette, H.
BIOMETRICAL JOURNAL. Volume: (2018) - 2018 • 4890
Experimental confirmation of a new invariant for a non-linear chemical reaction
Peng, B. and Yablonsky, G.S. and Constales, D. and Marin, G.B. and Muhler, M.
CHEMICAL ENGINEERING SCIENCE. Volume: 191 (2018) - 2018 • 4889
Sodium enhances indium-gallium interdiffusion in copper indium gallium diselenide photovoltaic absorbers
Colombara, D. and Werner, F. and Schwarz, T. and Cañero Infante, I. and Fleming, Y. and Valle, N. and Spindler, C. and Vacchieri, E. and Rey, G. and Guennou, M. and Bouttemy, M. and Manjón, A.G. and Peral Alonso, I. and Melchiorre, M. and El Adib, B. and Gault, B. and Raabe, D. and Dale, P.J. and Siebentritt, S.
NATURE COMMUNICATIONS. Volume: 9 (2018) - 2018 • 4888
The “In Situ Electrolyte” Concept: Using Activation Chemicals as Electrolytes for Carbon-Based Supercapacitors
Leistenschneider, D. and Schneidermann, C. and Hippauf, F. and Grätz, S. and Borchardt, L.
ADVANCED SUSTAINABLE SYSTEMS. Volume: 2 (2018) - 2018 • 4887
Segregation-Induced Nanofaceting Transition at an Asymmetric Tilt Grain Boundary in Copper
Peter, N.J. and Frolov, T. and Duarte, M.J. and Hadian, R. and Ophus, C. and Kirchlechner, C. and Liebscher, C.H. and Dehm, G.
PHYSICAL REVIEW LETTERS. Volume: 121 (2018) - 2018 • 4886
Self-powered bioelectrochemical devices
Conzuelo, F. and Ruff, A. and Schuhmann, W.
CURRENT OPINION IN ELECTROCHEMISTRY. Volume: 12 (2018) - 2018 • 4885
Analysis of photosystem II electron transfer with natural PsbA-variants by redox polymer/protein biophotoelectrochemistry
Hartmann, V. and Ruff, A. and Schuhmann, W. and Rögner, M. and Nowaczyk, M.M.
PHOTOSYNTHETICA. Volume: (2018) - 2018 • 4884
The Open Circuit Voltage in Biofuel Cells: Nernstian Shift in Pseudocapacitive Electrodes
Conzuelo, F. and Marković, N. and Ruff, A. and Schuhmann, W.
ANGEWANDTE CHEMIE - INTERNATIONAL EDITION. Volume: 57 (2018) - 2018 • 4883
Wall effects on spatial correlations of non-affine strain in a 3D model glass
Hassani, M. and Engels, P. and Varnik, F.
EPL. Volume: 121 (2018) - 2018 • 4882
Deformation induced degradation of hot-dip aluminized steel
Lemmens, B. and Springer, H. and Peeters, M. and De Graeve, I. and De Strycker, J. and Raabe, D. and Verbeken, K.
MATERIALS SCIENCE AND ENGINEERING A. Volume: 710 (2018) - 2018 • 4881
Biological imaging with scanning electrochemical microscopy
Conzuelo, F. and Schulte, A. and Schuhmann, W.
PROCEEDINGS OF THE ROYAL SOCIETY A: MATHEMATICAL, PHYSICAL AND ENGINEERING SCIENCES. Volume: 474 (2018) - 2018 • 4880
Long-range strain correlations in 3D quiescent glass forming liquids
Hassani, M. and Zirdehi, E.M. and Kok, K. and Schall, P. and Fuchs, M. and Varnik, F.
EPL. Volume: 124 (2018) - 2018 • 4879
Hardness and modulus of Fe2B, Fe3(C,B), and Fe23(C,B)6 borides and carboborides in the Fe-C-B system
Lentz, J. and Röttger, A. and Theisen, W.
MATERIALS CHARACTERIZATION. Volume: 135 (2018) - 2018 • 4878
Enhancement of hardness, modulus and fracture toughness of the tetragonal (Fe,Cr)2B and orthorhombic (Cr,Fe)2B phases with addition of Cr
Lentz, J. and Röttger, A. and Großwendt, F. and Theisen, W.
MATERIALS AND DESIGN. Volume: 156 (2018) - 2018 • 4877
Atypical titration curves for GaAl12 Keggin-ions explained by a joint experimental and simulation approach
Sulpizi, M. and Lützenkirchen, J.
JOURNAL OF CHEMICAL PHYSICS. Volume: 148 (2018) - 2018 • 4876
Automated differentiation between meningioma and healthy brain tissue based on optical coherence tomography ex vivo images using texture features
Lenz, M. and Krug, R. and Dillmann, C. and Stroop, R. and Gerhardt, N.C. and Welp, H. and Schmieder, K. and Hofmann, M.R.
JOURNAL OF BIOMEDICAL OPTICS. Volume: 23 (2018) - 2018 • 4875
Multiscale modeling on biological systems
Sulpizi, M. and Faller, R. and Pantano, S.
BIOCHEMICAL AND BIOPHYSICAL RESEARCH COMMUNICATIONS. Volume: 498 (2018) - 2018 • 4874
Electrochemical C-H Cyanation of Electron-Rich (Hetero)Arenes
Hayrapetyan, D. and Rit, R.K. and Kratz, M. and Tschulik, K. and Gooßen, L.J.
CHEMISTRY - A EUROPEAN JOURNAL. Volume: (2018) - 2018 • 4873
Brain tissue analysis using texture features based on optical coherence tomography images
Lenz, M. and Krug, R. and DIllmann, C. and Gerhardt, N.C. and Welp, H. and Schmieder, K. and Hofmann, M.R.
PROGRESS IN BIOMEDICAL OPTICS AND IMAGING - PROCEEDINGS OF SPIE. Volume: 10483 (2018) - 2018 • 4872
Advanced crystallographic study of the columnar growth of YZS coatings produced by PS-PVD
He, W. and Mauer, G. and Schwedt, A. and Guillon, O. and Vaßen, R.
JOURNAL OF THE EUROPEAN CERAMIC SOCIETY. Volume: 38 (2018) - 2018 • 4871
Classification of brain tissue with optical coherence tomography by employing texture analysis
Lenz, M. and Krug, R. and Dillmann, C. and Gerhardt, N.C. and Welp, H. and Schmieder, K. and Hofmann, M.R.
PROCEEDINGS OF SPIE - THE INTERNATIONAL SOCIETY FOR OPTICAL ENGINEERING. Volume: 10679 (2018) - 2018 • 4870
Catalysis of Carbon Dioxide Photoreduction on Nanosheets: Fundamentals and Challenges
Sun, Z. and Talreja, N. and Tao, H. and Texter, J. and Muhler, M. and Strunk, J. and Chen, J.
ANGEWANDTE CHEMIE - INTERNATIONAL EDITION. Volume: 57 (2018) - 2018 • 4869
Direct Growth of MoS2 and WS2 Layers by Metal Organic Chemical Vapor Deposition
Cwik, S. and Mitoraj, D. and Mendoza Reyes, O. and Rogalla, D. and Peeters, D. and Kim, J. and Schütz, H.M. and Bock, C. and Beranek, R. and Devi, A.
ADVANCED MATERIALS INTERFACES. Volume: 5 (2018) - 2018 • 4868
A Microscopic Interpretation of Pump-Probe Vibrational Spectroscopy Using Ab Initio Molecular Dynamics
Lesnicki, D. and Sulpizi, M.
JOURNAL OF PHYSICAL CHEMISTRY B. Volume: 122 (2018) - 2018 • 4867
Spokes in high power impulse magnetron sputtering plasmas
Hecimovic, A. and Von Keudell, A.
JOURNAL OF PHYSICS D: APPLIED PHYSICS. Volume: 51 (2018) - 2018 • 4866
First-Principles Approach to Model Electrochemical Reactions: Understanding the Fundamental Mechanisms behind Mg Corrosion
Surendralal, S. and Todorova, M. and Finnis, M.W. and Neugebauer, J.
PHYSICAL REVIEW LETTERS. Volume: 120 (2018) - 2018 • 4865
Investigation of fractal MIMO concepts for radar imaging of bulk solids
Dahl, C. and Rolfes, I. and Vogt, M.
EUROPEAN MICROWAVE WEEK 2017: "A PRIME YEAR FOR A PRIME EVENT", EUMW 2017 - CONFERENCE PROCEEDINGS; 14TH EUROPEAN MICROWAVE CONFERENCE, EURAD 2017. Volume: 2018-January (2018) - 2018 • 4864
Terahertz Time-Domain Spectroscopy by Asynchronous Sampling with Modelocked Semiconductor Lasers
Surkamp, N. and Döpke, B. and Hu, Y. and Brenner, C. and Hofmann, M.R. and Klehr, A. and Knigge, A. and Tränkle, G.
2018 1ST INTERNATIONAL WORKSHOP ON MOBILE TERAHERTZ SYSTEMS, IWMTS 2018. Volume: (2018) - 2018 • 4863
Detuning dependence of Rabi oscillations in an InAs self-assembled quantum dot ensemble
Suzuki, T. and Singh, R. and Bayer, M. and Ludzwig, A. and Wieck, A.D. and Cundiff, S.T.
PHYSICAL REVIEW B. Volume: 97 (2018) - 2018 • 4862
A Shear-Slip Mesh Update – Immersed Boundary Finite Element model for computational simulations of material transport in EPB tunnel boring machines
Dang, T.S. and Meschke, G.
FINITE ELEMENTS IN ANALYSIS AND DESIGN. Volume: 142 (2018) - 2018 • 4861
Accelerated atomic-scale exploration of phase evolution in compositionally complex materials
Li, Y.J. and Savan, A. and Kostka, A. and Stein, H.S. and Ludwig, Al.
MATERIALS HORIZONS. Volume: 5 (2018) - 2018 • 4860
From Quasicrystals to Crystals with Interpenetrating Icosahedra in Ca-Au-Al: In Situ Variable-Temperature Transformation
Pham, J. and Meng, F. and Lynn, M.J. and Ma, T. and Kreyssig, A. and Kramer, M.J. and Goldman, A.I. and Miller, G.J.
JOURNAL OF THE AMERICAN CHEMICAL SOCIETY. Volume: 140 (2018) - 2018 • 4859
Dephasing of InAs quantum dot p -shell excitons studied using two-dimensional coherent spectroscopy
Suzuki, T. and Singh, R. and Moody, G. and Aßmann, M. and Bayer, M. and Ludwig, Ar. and Wieck, A.D. and Cundiff, S.T.
PHYSICAL REVIEW B. Volume: 98 (2018) - 2018 • 4858
Nucleation driving force for ω-assisted formation of α and associated ω morphology in β-Ti alloys
Li, T. and Kent, D. and Sha, G. and Liu, H. and Fries, S.G. and Ceguerra, A.V. and Dargusch, M.S. and Cairney, J.M.
SCRIPTA MATERIALIA. Volume: 155 (2018) - 2018 • 4857
Influence of preparation method and doping of zirconium oxide onto the material characteristics and catalytic activity for the HDO reaction in nickel on zirconium oxide catalysts
Pichler, C.M. and Gu, D. and Joshi, H. and Schüth, F.
JOURNAL OF CATALYSIS. Volume: 365 (2018) - 2018 • 4856
Finite-deformation phase-field chemomechanics for multiphase, multicomponent solids
Svendsen, B. and Shanthraj, P. and Raabe, D.
JOURNAL OF THE MECHANICS AND PHYSICS OF SOLIDS. Volume: 112 (2018) - 2018 • 4855
Atomic-scale investigation of fast oxidation kinetics of nanocrystalline CrMnFeCoNi thin films
Li, Y.J. and Kostka, A. and Savan, A. and Ludwig, Al.
JOURNAL OF ALLOYS AND COMPOUNDS. Volume: 766 (2018) - 2018 • 4854
Effect of tool wear evolution on chip formation during dry machining of Ti-6Al-4V alloy
Dargusch, M.S. and Sun, S. and Kim, J.W. and Li, T. and Trimby, P. and Cairney, J.
INTERNATIONAL JOURNAL OF MACHINE TOOLS AND MANUFACTURE. Volume: 126 (2018) - 2018 • 4853
Combinatorial metallurgical synthesis and processing of high-entropy alloys
Li, Z. and Ludwig, Al. and Savan, A. and Springer, H. and Raabe, D.
JOURNAL OF MATERIALS RESEARCH. Volume: (2018) - 2018 • 4852
A gas breathing hydrogen/air biofuel cell comprising a redox polymer/hydrogenase-based bioanode
Szczesny, J. and Marković, N. and Conzuelo, F. and Zacarias, S. and Pereira, I.A.C. and Lubitz, W. and Plumeré, N. and Schuhmann, W. and Ruff, A.
NATURE COMMUNICATIONS. Volume: 9 (2018) - 2018 • 4851
Numerical Benchmark of Phase-Field Simulations with Elastic Strains: Precipitation in the Presence of Chemo-Mechanical Coupling
Darvishi Kamachali, R. and Schwarze, C. and Lin, M. and Diehl, M. and Shanthraj, P. and Prahl, U. and Steinbach, I. and Raabe, D.
COMPUTATIONAL MATERIALS SCIENCE. Volume: 155 (2018) - 2018 • 4850
Atomic-scale insights into surface species of electrocatalysts in three dimensions
Li, T. and Kasian, O. and Cherevko, S. and Zhang, S. and Geiger, S. and Scheu, C. and Felfer, P. and Raabe, D. and Gault, B. and Mayrhofer, K.J.J.
NATURE CATALYSIS. Volume: 1 (2018) - 2018 • 4849
Segregation Phenomena in Size-Selected Bimetallic CuNi Nanoparticle Catalysts
Pielsticker, L. and Zegkinoglou, I. and Divins, N.J. and Mistry, H. and Chen, Y.-T. and Kostka, A. and Boscoboinik, J.A. and Cuenya, B.R.
JOURNAL OF PHYSICAL CHEMISTRY B. Volume: 122 (2018) - 2018 • 4848
Influence of biomass torrefaction parameters on fast pyrolysis products under flame-equivalent conditions
Pielsticker, S. and Möller, G. and Gövert, B. and Kreitzberg, T. and Hatzfeld, O. and Yönder, Ö. and Angenent, V. and Hättig, C. and Schmid, R. and Kneer, R.
BIOMASS AND BIOENERGY. Volume: 119 (2018) - 2018 • 4847
Basic Requirements of Spin-Flip Raman Scattering on Excitonic Resonances and Its Modulation through Additional High-Energy Illumination in Semiconductor Heterostructures
Debus, J. and Kudlacik, D. and Sapega, V.F. and Shamirzaev, T.S. and Yakovlev, D.R. and Reuter, D. and Wieck, A.D. and Waag, A. and Bayer, M.
PHYSICS OF THE SOLID STATE. Volume: 60 (2018) - 2018 • 4846
Numerical modelling of an optical belt sorter using a DEM–CFD approach coupled with particle tracking and comparison with experiments
Pieper, C. and Pfaff, F. and Maier, G. and Kruggel-Emden, H. and Wirtz, S. and Noack, B. and Gruna, R. and Scherer, V. and Hanebeck, U.D. and Längle, T. and Beyerer, J.
POWDER TECHNOLOGY. Volume: 340 (2018) - 2018 • 4845
Nucleic acid hybridization on an electrically reconfigurable network of gold-coated magnetic nanoparticles enables microRNA detection in blood
Tavallaie, R. and McCarroll, J. and Le Grand, M. and Ariotti, N. and Schuhmann, W. and Bakker, E. and Tilley, R.D. and Hibbert, D.B. and Kavallaris, M. and Gooding, J.J.
NATURE NANOTECHNOLOGY. Volume: 13 (2018) - 2018 • 4844
Strain-Induced Asymmetric Line Segregation at Faceted Si Grain Boundaries
Liebscher, C.H. and Stoffers, A. and Alam, M. and Lymperakis, L. and Cojocaru-Mirédin, O. and Gault, B. and Neugebauer, J. and Dehm, G. and Scheu, C. and Raabe, D.
PHYSICAL REVIEW LETTERS. Volume: 121 (2018) - 2018 • 4843
Influence of the Fe:Ni Ratio and Reaction Temperature on the Efficiency of (FexNi1-x)9S8 Electrocatalysts Applied in the Hydrogen Evolution Reaction
Piontek, S. and Andronescu, C. and Zaichenko, A. and Konkena, B. and Junge Puring, K. and Marler, B. and Antoni, H. and Sinev, I. and Muhler, M. and Mollenhauer, D. and Roldan Cuenya, B. and Schuhmann, W. and Apfel, U.-P.
ACS CATALYSIS. Volume: 8 (2018) - 2018 • 4842
Tuned Amperometric Detection of Reduced β-Nicotinamide Adenine Dinucleotide by Allosteric Modulation of the Reductase Component of the p -Hydroxyphenylacetate Hydroxylase Immobilized within a Redox Polymer
Teanphonkrang, S. and Janke, S. and Chaiyen, P. and Sucharitakul, J. and Suginta, W. and Khunkaewla, P. and Schuhmann, W. and Ruff, A. and Schulte, A.
ANALYTICAL CHEMISTRY. Volume: 90 (2018) - 2018 • 4841
Overview on micro- and nanomechanical testing: New insights in interface plasticity and fracture at small length scales
Dehm, G. and Jaya, B.N. and Raghavan, R. and Kirchlechner, C.
ACTA MATERIALIA. Volume: 142 (2018) - 2018 • 4840
Tetragonal fcc-Fe induced by κ -carbide precipitates: Atomic scale insights from correlative electron microscopy, atom probe tomography, and density functional theory
Liebscher, C.H. and Yao, M. and Dey, P. and Lipińska-Chwalek, M. and Berkels, B. and Gault, B. and Hickel, T. and Herbig, M. and Mayer, J. and Neugebauer, J. and Raabe, D. and Dehm, G. and Scheu, C.
PHYSICAL REVIEW MATERIALS. Volume: 2 (2018) - 2018 • 4839
Electronic Properties of a Two-Dimensional Electron Gas at the Interface between Transition Metal Complex Oxides
Piyanzina, I.I. and Lysogorskiy, Y.V. and Tayurskii, D.A. and Mamin, R.F.
BULLETIN OF THE RUSSIAN ACADEMY OF SCIENCES: PHYSICS. Volume: 82 (2018) - 2018 • 4838
Preparation-Dependent Orientation of Crystalline Ice Islands on Ag(111)
Heidorn, S.-C. and Lucht, K. and Bertram, C. and Morgenstern, K.
JOURNAL OF PHYSICAL CHEMISTRY B. Volume: 122 (2018) - 2018 • 4837
Comparative studies on the interaction of anticancer drug irinotecan with dsDNA and ssDNA
Temerk, Y. and Ibrahim, M. and Ibrahim, H. and Schuhmann, W.
RSC ADVANCES. Volume: 8 (2018) - 2018 • 4836
Low-Temperature Growth of Amorphous Water Ice on Ag(111)
Heidorn, S.-C. and Bertram, C. and Morgenstern, K.
JOURNAL OF PHYSICAL CHEMISTRY C. Volume: 122 (2018) - 2018 • 4835
Optimal discrimination designs for semiparametric models
Dette, H. and Guchenko, R. and Melas, V.B. and Wong, W.K.
BIOMETRIKA. Volume: 105 (2018) - 2018 • 4834
Demonstrating ultrafast polarization dynamics in spin-VCSELs
Lindemann, M. and Pusch, T. and Michalzik, R. and Gerhardt, N.C. and Hofmann, M.R.
PROCEEDINGS OF SPIE - THE INTERNATIONAL SOCIETY FOR OPTICAL ENGINEERING. Volume: 10552 (2018) - 2018 • 4833
Equivalence of Regression Curves
Dette, H. and Möllenhoff, K. and Volgushev, S. and Bretz, F.
JOURNAL OF THE AMERICAN STATISTICAL ASSOCIATION. Volume: (2018) - 2018 • 4832
Spin lasers for optical data communication
Lindemann, M. and Pusch, T. and Michalzik, R. and Gerhardt, N.C. and Hofmann, M.R.
PROCEEDINGS OF SPIE - THE INTERNATIONAL SOCIETY FOR OPTICAL ENGINEERING. Volume: 10682 (2018) - 2018 • 4831
Universality in random moment problems
Dette, H. and Tomecki, D. and Venker, M.
ELECTRONIC JOURNAL OF PROBABILITY. Volume: 23 (2018) - 2018 • 4830
An advanced high-temperature stable multipole resonance probe for industry compatible plasma diagnostics
Pohle, D. and Schulz, C. and Rolfes, I. and Oberberg, M. and Awakowicz, P. and Serwa, A. and Uhlig, P.
GEMIC 2018 - 2018 GERMAN MICROWAVE CONFERENCE. Volume: 2018-January (2018) - 2018 • 4829
Relevant change points in high dimensional time series
Dette, H. and Gösmann, J.
ELECTRONIC JOURNAL OF STATISTICS. Volume: 12 (2018) - 2018 • 4828
Progression of the Multipole Resonance Probe: Advanced Plasma Sensors Based on LTCC-Technology
Pohle, D. and Schulz, C. and Oberberg, M. and Serwa, A. and Uhlig, P. and Awakowicz, P. and Rolfes, I.
2018 48TH EUROPEAN MICROWAVE CONFERENCE, EUMC 2018. Volume: (2018) - 2018 • 4827
Maximum Likelihood Analysis of Reaction Coordinates during Solidification in Ni
Díaz Leines, G. and Rogal, J.
JOURNAL OF PHYSICAL CHEMISTRY B. Volume: 122 (2018) - 2018 • 4826
Surface reconstruction using thinned random arrays in mm-wave FMCW SAR imaging
Pohle, D. and Barowski, J. and Jebramcik, J. and Rolfes, I.
EUROPEAN MICROWAVE WEEK 2017: "A PRIME YEAR FOR A PRIME EVENT", EUMW 2017 - CONFERENCE PROCEEDINGS; 14TH EUROPEAN MICROWAVE CONFERENCE, EURAD 2017. Volume: 2018-January (2018) - 2018 • 4825
Velocity distribution of titanium neutrals in the target region of high power impulse magnetron sputtering discharges
Held, J. and Hecimovic, A. and Von Keudell, A. and Schulz-Von Der Gathen, V.
PLASMA SOURCES SCIENCE AND TECHNOLOGY. Volume: 27 (2018) - 2018 • 4824
Impact of Hydrophobic Organohybrid Silicas on the Stability of Ni2P Catalyst Phase in the Hydrodeoxygenation of Biophenols
Dierks, M. and Cao, Z. and Manayil, J.C. and Akilavasan, J. and Wilson, K. and Schüth, F. and Rinaldi, R.
CHEMCATCHEM. Volume: 10 (2018) - 2018 • 4823
Microstructure and mechanical properties of Al0.7CoCrFeNi high-entropy-alloy prepared by directional solidification
Liu, G. and Liu, L. and Liu, X. and Wang, Z. and Han, Z. and Zhang, G. and Kostka, A.
INTERMETALLICS. Volume: 93 (2018) - 2018 • 4822
Quantum Optics with Near-Lifetime-Limited Quantum-Dot Transitions in a Nanophotonic Waveguide
Thyrrestrup, H. and Kiršanske, G. and Le Jeannic, H. and Pregnolato, T. and Zhai, L. and Raahauge, L. and Midolo, L. and Rotenberg, N. and Javadi, A. and Schott, R. and Wieck, A.D. and Ludwig, Ar. and Löbl, M.C. and Söllner, I. and Warburton, R.J. and Lodahl, P.
NANO LETTERS. Volume: 18 (2018) - 2018 • 4821
Nuclear Quantum Effects in Sodium Hydroxide Solutions from Neural Network Molecular Dynamics Simulations
Hellström, M. and Ceriotti, M. and Behler, J.
JOURNAL OF PHYSICAL CHEMISTRY B. Volume: 122 (2018) - 2018 • 4820
Ultrasound-mediated deposition and cytocompatibility of apatite-like coatings on magnesium alloys
Liu, C.-N. and Böke, F. and Gebhard, M. and Devi, A. and Fischer, H. and Keller, A. and Grundmeier, G.
SURFACE AND COATINGS TECHNOLOGY. Volume: 345 (2018) - 2018 • 4819
Near lifetime-limited emitter in a nanophotonic waveguide
Thyrrestrup, H. and Kiršanskė, G. and Jeannic, H.L. and Pregnolato, T. and Zhai, L. and Midolo, L. and Rotenberg, N. and Javadi, A. and Schott, R. and Wieck, A.D. and Ludwig, Ar. and Löbl, M.C. and Söllner, I. and Warburton, R.J. and Lodahl, P.
OPTICS INFOBASE CONFERENCE PAPERS. Volume: Part F93-CLEO_QELS 2018 (2018) - 2018 • 4818
An integrated crystal plasticity-phase field model for spatially resolved twin nucleation, propagation, and growth in hexagonal materials
Liu, C. and Shanthraj, P. and Diehl, M. and Roters, F. and Dong, S. and Dong, J. and Ding, W. and Raabe, D.
INTERNATIONAL JOURNAL OF PLASTICITY. Volume: 106 (2018) - 2018 • 4817
Highly Efficient Photocatalytic Degradation of Dyes by a Copper–Triazolate Metal–Organic Framework
Liu, C.-X. and Zhang, W.-H. and Wang, N. and Guo, P. and Muhler, M. and Wang, Y. and Lin, S. and Chen, Z. and Yang, G.
CHEMISTRY - A EUROPEAN JOURNAL. Volume: 24 (2018) - 2018 • 4816
Hedgehog Spin-Vortex Crystal Antiferromagnetic Quantum Criticality in CaK (Fe1-xNix)4As4 Revealed by NMR
Ding, Q.-P. and Meier, W.R. and Cui, J. and Xu, M. and Böhmer, A.E. and Bud'Ko, S.L. and Canfield, P.C. and Furukawa, Y.
PHYSICAL REVIEW LETTERS. Volume: 121 (2018) - 2018 • 4815
Nanoimprint lithography of nanoporous carbon materials for micro-supercapacitor architectures
Lochmann, S. and Grothe, J. and Eckhardt, K. and Leistenschneider, D. and Borchardt, L. and Kaskel, S.
NANOSCALE. Volume: 10 (2018) - 2018 • 4814
Influence of microstructure morphology on multi-scale modeling of low-alloyed TRIP-steels
Prüger, S. and Gandhi, A. and Balzani, D.
ENGINEERING COMPUTATIONS (SWANSEA, WALES). Volume: 35 (2018) - 2018 • 4813
A Multiperspective Approach to Solvent Regulation of Enzymatic Activity: HMG-CoA Reductase
Dirkmann, M. and Iglesias-Fernández, J. and Muñoz, V. and Sokkar, P. and Rumancev, C. and von Gundlach, A. and Krenczyk, O. and Vöpel, T. and Nowack, J. and Schroer, M.A. and Ebbinghaus, S. and Herrmann, C. and Rosenhahn, A. and Sanchez-Garcia, E. and Schulz, F.
CHEMBIOCHEM. Volume: 19 (2018) - 2018 • 4812
Filament Growth and Resistive Switching in Hafnium Oxide Memristive Devices
Dirkmann, S. and Kaiser, J. and Wenger, C. and Mussenbrock, T.
ACS APPLIED MATERIALS AND INTERFACES. Volume: 10 (2018) - 2018 • 4811
Innovative test setup for the cyclic loading of multiple large-format concrete specimens
Przondziono, R. and Schulte-Schrepping, C. and Breitenbücher, R.
BETON- UND STAHLBETONBAU. Volume: 113 (2018) - 2018 • 4810
Dry Reforming of Methane at High Pressure in a Fixed-Bed Reactor with Axial Temperature Profile Determination
Tillmann, L. and Schulwitz, J. and van Veen, A. and Muhler, M.
CATALYSIS LETTERS. Volume: 148 (2018) - 2018 • 4809
Laser metal deposition of a refractory TiZrNbHfTa high-entropy alloy
Dobbelstein, H. and Gurevich, E.L. and George, E.P. and Ostendorf, A. and Laplanche, G.
ADDITIVE MANUFACTURING. Volume: 24 (2018) - 2018 • 4808
Discovery of a Multinary Noble Metal–Free Oxygen Reduction Catalyst
Löffler, T. and Meyer, H. and Savan, A. and Wilde, P. and Garzón Manjón, A. and Chen, Y.-T. and Ventosa, E. and Scheu, C. and Ludwig, Al. and Schuhmann, W.
ADVANCED ENERGY MATERIALS. Volume: 8 (2018) - 2018 • 4807
Electrical birefringence tuning of VCSELs
Pusch, T. and Lindemann, M. and Gerhardt, N.C. and Hofmann, M.R. and Michalzik, R.
PROCEEDINGS OF SPIE - THE INTERNATIONAL SOCIETY FOR OPTICAL ENGINEERING. Volume: 10552 (2018) - 2018 • 4806
Lessons learned from IDeAl - 33 recommendations from the IDeAl-net about design and analysis of small population clinical trials
Hilgers, R.-D. and Bogdan, M. and Burman, C.-F. and Dette, H. and Karlsson, M. and König, F. and Male, C. and Mentré, F. and Molenberghs, G. and Senn, S.
ORPHANET JOURNAL OF RARE DISEASES. Volume: 13 (2018) - 2018 • 4805
Evaluation of the intrinsic catalytic activity of nanoparticles without prior knowledge of the mass loading
Löffler, T. and Wilde, P. and Öhl, D. and Chen, Y.-T. and Tschulik, K. and Schuhmann, W.
FARADAY DISCUSSIONS. Volume: 210 (2018) - 2018 • 4804
Thermally-induced birefringence in VCSELs: Approaching the limits
Pusch, T. and Scherübl, S. and Lindemann, M. and Gerhardt, N.C. and Hofmann, M.R. and Michalzik, R.
PROCEEDINGS OF SPIE - THE INTERNATIONAL SOCIETY FOR OPTICAL ENGINEERING. Volume: 10682 (2018) - 2018 • 4803
Spectroelectrochemical studies on the effect of cations in the alkaline glycerol oxidation reaction over carbon nanotube-supported Pd nanoparticles
Hiltrop, D. and Cychy, S. and Elumeeva, K. and Schuhmann, W. and Muhler, M.
BEILSTEIN JOURNAL OF ORGANIC CHEMISTRY. Volume: 14 (2018) - 2018 • 4802
Maximally resolved anharmonic OH vibrational spectrum of the water/ZnO(10 1 0) interface from a high-dimensional neural network potential
Quaranta, V. and Hellström, M. and Behler, J. and Kullgren, J. and Mitev, P.D. and Hermansson, K.
JOURNAL OF CHEMICAL PHYSICS. Volume: 148 (2018) - 2018 • 4801
Potential pulse-assisted immobilization of Myrothecium verrucaria bilirubin oxidase at planar and nanoporous gold electrodes
Lopez, F. and Siepenkoetter, T. and Xiao, X. and Magner, E. and Schuhmann, W. and Salaj-Kosla, U.
JOURNAL OF ELECTROANALYTICAL CHEMISTRY. Volume: 812 (2018) - 2018 • 4800
An O2 Tolerant Polymer/Glucose Oxidase Based Bioanode as Basis for a Self-Powered Glucose Sensor
Lopez, F. and Zerria, S. and Ruff, A. and Schuhmann, W.
ELECTROANALYSIS. Volume: (2018) - 2018 • 4799
Antibacterial Efficacy of Sacrifical Anode Thin Films Combining Silver with Platinum Group Elements within a Bacteria-Containing Human Plasma Clot
Abuayyash, A. and Ziegler, N. and Gessmann, J. and Sengstock, C. and Schildhauer, T.A. and Ludwig, Al. and Köller, M.
ADVANCED ENGINEERING MATERIALS. Volume: 20 (2018) - 2018 • 4798
High-temperature ultrasound attenuation in langasite and langatate
Hirschle, C. and Schreuer, J.
IEEE TRANSACTIONS ON ULTRASONICS, FERROELECTRICS, AND FREQUENCY CONTROL. Volume: 65 (2018) - 2018 • 4797
Modulation infrared thermometry of caloric effects at up to kHz frequencies
Döntgen, J. and Rudolph, J. and Waske, A. and Hägele, D.
REVIEW OF SCIENTIFIC INSTRUMENTS. Volume: 89 (2018) - 2018 • 4796
Interplay of cation ordering and thermoelastic properties of spinel structure MgGa2O4
Hirschle, C. and Schreuer, J. and Galazka, Z.
JOURNAL OF APPLIED PHYSICS. Volume: 124 (2018) - 2018 • 4795
Change-Point Detection in Autoregressive Models with no Moment Assumptions
Akashi, F. and Dette, H. and Liu, Y.
JOURNAL OF TIME SERIES ANALYSIS. Volume: 39 (2018) - 2018 • 4794
Millisecond Dynamics of the Magnetocaloric Effect in a First- and Second-Order Phase Transition Material
Döntgen, J. and Rudolph, J. and Gottschall, T. and Gutfleisch, O. and Hägele, D.
ENERGY TECHNOLOGY. Volume: 6 (2018) - 2018 • 4793
Nonparametric inference of gradual changes in the jump behaviour of time-continuous processes
Hoffmann, M. and Vetter, M. and Dette, H.
STOCHASTIC PROCESSES AND THEIR APPLICATIONS. Volume: (2018) - 2018 • 4792
Formation of eta carbide in ferrous martensite by room temperature aging
Lu, W. and Herbig, M. and Liebscher, C.H. and Morsdorf, L. and Marceau, R.K.W. and Dehm, G. and Raabe, D.
ACTA MATERIALIA. Volume: 158 (2018) - 2018 • 4791
A multiscale model for high performance FRC
Timothy, J.J. and Iskhakov, T. and Zhan, Y. and Meschke, G.
RILEM BOOKSERIES. Volume: 15 (2018) - 2018 • 4790
Extraction of Relative Permittivity from Measured Reflection Coefficient of Dielectric Materials in the Frequency Range 207 - 247 GHz
Alawnch, I. and Barowski, J. and Rolfes, I.
2018 48TH EUROPEAN MICROWAVE CONFERENCE, EUMC 2018. Volume: (2018) - 2018 • 4789
Copper Supported on Hybrid C@SiO2 Hollow Submicron Spheres as Active Ethanol Dehydrogenation Catalyst
Lu, W.-D. and Wang, Q.-N. and He, L. and Li, W.-C. and Schüth, F. and Lu, A.-H.
CHEMNANOMAT. Volume: 4 (2018) - 2018 • 4788
Heisenberg model analysis on inelastic powder neutron scattering data using parent and K doped BaMn2As2 samples
Ramazanoglu, M. and Sapkota, A. and Pandey, A. and Lamsal, J. and Abernathy, D.L. and Niedziela, J.L. and Stone, M.B. and Salci, R. and Acar, D.A. and Oztirpan, F.O. and Ozonder, S. and Kreyssig, A. and Goldman, A.I. and Johnston, D.C. and McQueeney, R.J.
PHYSICA B: CONDENSED MATTER. Volume: 551 (2018) - 2018 • 4787
Effective Diffusivity of Porous Materials with Microcracks: Self-Similar Mean-Field Homogenization and Pixel Finite Element Simulations
Timothy, J.J. and Meschke, G.
TRANSPORT IN POROUS MEDIA. Volume: 125 (2018) - 2018 • 4786
Finite-temperature property-maps of Li-Mn-Ni-O cathode materials from: Ab initio calculations
Albina, J.-M. and Marusczyk, A. and Hammerschmidt, T. and Eckl, T. and Drautz, R.
JOURNAL OF MATERIALS CHEMISTRY A. Volume: 6 (2018) - 2018 • 4785
Bidirectional Transformation Enables Hierarchical Nanolaminate Dual-Phase High-Entropy Alloys
Lu, W. and Liebscher, C.H. and Dehm, G. and Raabe, D. and Li, Z.
ADVANCED MATERIALS. Volume: 30 (2018) - 2018 • 4784
Martensitic transformation hysteresis in Ni(Co)-Mn-Sn/MgO metamagnetic shape memory thin films
Alexandrakis, V. and Aseguinolaza, I.R. and Decker, P. and Salomon, S. and Barandiarán, J.M. and Ludwig, Al. and Chernenko, V.A.
SCRIPTA MATERIALIA. Volume: 156 (2018) - 2018 • 4783
Adhesion of plasma-deposited silicon oxide barrier layers on PDMS containing polypropylene
Hoppe, C. and Mitschker, F. and Awakowicz, P. and Kirchheim, D. and Dahlmann, R. and de los Arcos, T. and Grundmeier, G.
SURFACE AND COATINGS TECHNOLOGY. Volume: 335 (2018) - 2018 • 4782
On femtosecond laser shock peening of stainless steel AISI 316
Hoppius, J.S. and Kukreja, L.M. and Knyazeva, M. and Pöhl, F. and Walther, F. and Ostendorf, A. and Gurevich, E.L.
APPLIED SURFACE SCIENCE. Volume: 435 (2018) - 2018 • 4781
Sum frequency generation spectra from velocity-velocity correlation functions: New developments and applications
Rémi, K. and Marialore, S.
HIGH PERFORMANCE COMPUTING IN SCIENCE AND ENGINEERING' 17: TRANSACTIONS OF THE HIGH PERFORMANCE COMPUTING CENTER, STUTTGART (HLRS) 2017. Volume: (2018) - 2018 • 4780
Mode-locked diode laser with resonant ring amplifier
Ali Alloush, M. and Pilny, R.H. and Brenner, C. and Prziwarka, T. and Klehr, A. and Knigge, A. and Tränkle, G. and Hofmann, M.R.
PROCEEDINGS OF SPIE - THE INTERNATIONAL SOCIETY FOR OPTICAL ENGINEERING. Volume: 10682 (2018) - 2018 • 4779
Femtosecond laser crystallization of amorphous titanium oxide thin films
Hoppius, J.S. and Bialuschewski, D. and Mathur, S. and Ostendorf, A. and Gurevich, E.L.
APPLIED PHYSICS LETTERS. Volume: 113 (2018) - 2018 • 4778
Atomic Layer Deposition of Nickel on ZnO Nanowire Arrays for High-Performance Supercapacitors
Ren, Q.-H. and Zhang, Y. and Lu, H.-L. and Wang, Y.-P. and Liu, W.-J. and Ji, X.-M. and Devi, A. and Jiang, A.-Q. and Zhang, D.W.
ACS APPLIED MATERIALS AND INTERFACES. Volume: 10 (2018) - 2018 • 4777
Carbon doping - A key for the substitute of thoriated tungsten
Traxler, H. and Wesemann, I. and Knabl, W. and Nilius, M. and Morkel, M. and Höbing, T. and Mentel, J. and Awakowicz, P.
INTERNATIONAL JOURNAL OF REFRACTORY METALS AND HARD MATERIALS. Volume: 74 (2018) - 2018 • 4776
Combined experimental and theoretical description of direct current magnetron sputtering of Al by Ar and Ar/N2 plasma
Trieschmann, J. and Ries, S. and Bibinov, N. and Awakowicz, P. and Mráz, S. and Schneider, J.M. and Mussenbrock, T.
PLASMA SOURCES SCIENCE AND TECHNOLOGY. Volume: 27 (2018) - 2018 • 4775
Passive, active, and hybrid mode-locking in a self-optimized ultrafast diode laser
Alloush, M.A. and Pilny, R.H. and Brenner, C. and Klehr, A. and Knigge, A. and Tränkle, G. and Hofmann, M.R.
PROCEEDINGS OF SPIE - THE INTERNATIONAL SOCIETY FOR OPTICAL ENGINEERING. Volume: 10553 (2018) - 2018 • 4774
Hydrophilicity and Microsolvation of an Organic Molecule Resolved on the Sub-molecular Level by Scanning Tunneling Microscopy
Lucht, K. and Loose, D. and Ruschmeier, M. and Strotkötter, V. and Dyker, G. and Morgenstern, K.
ANGEWANDTE CHEMIE - INTERNATIONAL EDITION. Volume: 57 (2018) - 2018 • 4773
A TEM Investigation of Columnar-Structured Thermal Barrier Coatings Deposited by Plasma Spray-Physical Vapor Deposition (PS-PVD)
Rezanka, S. and Somsen, C. and Eggeler, G. and Mauer, G. and Vaßen, R. and Guillon, O.
PLASMA CHEMISTRY AND PLASMA PROCESSING. Volume: 38 (2018) - 2018 • 4772
Kinetic bandgap analysis of plasma photonic crystals
Trieschmann, J. and Mussenbrock, T.
JOURNAL OF APPLIED PHYSICS. Volume: 124 (2018) - 2018 • 4771
Imaging the Solvation of a One-Dimensional Solid on the Molecular Scale
Lucht, K. and Trosien, I. and Sander, W. and Morgenstern, K.
ANGEWANDTE CHEMIE - INTERNATIONAL EDITION. Volume: 57 (2018) - 2018 • 4770
An algorithm based on incompatible modes for the global tracking of strong discontinuities in shear localization analyses
Alsahly, A. and Callari, C. and Meschke, G.
COMPUTER METHODS IN APPLIED MECHANICS AND ENGINEERING. Volume: 330 (2018) - 2018 • 4769
Robust Linear Output Regulation Using Extended State Observer
Hosseini-Pishrobat, M. and Keighobadi, J. and Oveisi, A. and Nestorović, T.
MATHEMATICAL PROBLEMS IN ENGINEERING. Volume: 2018 (2018) - 2018 • 4768
Risk estimators for choosing regularization parameters in ill-posed problems - Properties and limitations
Lucka, F. and Proksch, K. and Brune, C. and Bissantz, N. and Burger, M. and Dette, H. and Wübbeling, F.
INVERSE PROBLEMS AND IMAGING. Volume: 12 (2018) - 2018 • 4767
Detecting sign-changing superconducting gap in LiFeAs using quasiparticle interference
Altenfeld, D. and Hirschfeld, P.J. and Mazin, I.I. and Eremin, I.
PHYSICAL REVIEW B. Volume: 97 (2018) - 2018 • 4766
Sign reversal of the order parameter in (Li1-x Fex)OHFe1-y Zny Se
Du, Z. and Yang, X. and Altenfeld, D. and Gu, Q. and Yang, H. and Eremin, I. and Hirschfeld, P.J. and Mazin, I.I. and Lin, H. and Zhu, X. and Wen, H.-H.
NATURE PHYSICS. Volume: 14 (2018) - 2018 • 4765
Effect of Pt and Au current collector in LiMn2O4 thin film for micro-batteries
Trócoli, R. and Dushina, A. and Borhani-Haghighi, S. and Ludwig, Al. and La Mantia, F.
NANOTECHNOLOGY. Volume: 29 (2018) - 2018 • 4764
Laser beam welding of dual-phase DP1000 steel
Alves, P.H.O.M. and Lima, M.S.F. and Raabe, D. and Sandim, H.R.Z.
JOURNAL OF MATERIALS PROCESSING TECHNOLOGY. Volume: 252 (2018) - 2018 • 4763
Crystallographic and spectroscopic assignment of the proton transfer pathway in [FeFe]-hydrogenases
Duan, J. and Senger, M. and Esselborn, J. and Engelbrecht, V. and Wittkamp, F. and Apfel, U.-P. and Hofmann, E. and Stripp, S.T. and Happe, T. and Winkler, M.
NATURE COMMUNICATIONS. Volume: 9 (2018) - 2018 • 4762
Light as Trigger for Biocatalysis: Photonic Wiring of Flavin Adenine Dinucleotide-Dependent Glucose Dehydrogenase to Quantum Dot-Sensitized Inverse Opal TiO2 Architectures via Redox Polymers
Riedel, M. and Parak, W.J. and Ruff, A. and Schuhmann, W. and Lisdat, F.
ACS CATALYSIS. Volume: 8 (2018) - 2018 • 4761
Processes at nanopores and bio-nanointerfaces: General discussion
Alzahrani, H. and Antoine, C. and Baker, L. and Balme, S. and Bhattacharya, G. and Bohn, P.W. and Cai, Q. and Chikere, C. and Crooks, R.M. and Das, N. and Edwards, M. and Ehi-Eromosele, C. and Ermann, N. and Jiang, L. and Kanoufi, F. and Kranz, C. and Long, Y. and Macpherson, J. and McKelvey, K. and Mirkin, M. and Nichols, R. and Nogala, W. and Pelta, J. and Ren, H. and Rudd, J. and Schuhmann, W. and Siwy, Z. and Tian, Z. and Unwin, P. and Wen, L. and White, H. and Willets, K. and Wu, Y. and Ying, Y.
FARADAY DISCUSSIONS. Volume: 210 (2018) - 2018 • 4760
Grain boundary-constrained reverse austenite transformation in nanostructured Fe alloy: Model and application
Huang, L. and Lin, W. and Wang, K. and Song, S. and Guo, C. and Chen, Y. and Li, Y. and Liu, F.
ACTA MATERIALIA. Volume: 154 (2018) - 2018 • 4759
Dynamics of nanointerfaces: General discussion
Alzahrani, H. and Bentley, C. and Burrows, R. and Cao, C. and Cai, Q. and Chikere, C. and Crooks, R.M. and Dunevall, J. and Edwards, M. and Ewing, A. and Gao, R. and Hillman, R. and Kahram, M. and Kanoufi, F. and Kranz, C. and Lemineur, J.-F. and Long, Y. and McKelvey, K. and Mirkin, M. and Moore, S. and Nogala, W. and Ren, H. and Schuhmann, W. and Unwin, P. and Vezzoli, A. and White, H. and Willets, K. and Yang, Z. and Ying, Y.
FARADAY DISCUSSIONS. Volume: 210 (2018) - 2018 • 4758
A machine learning approach to model solute grain boundary segregation
Huber, L. and Hadian, R. and Grabowski, B. and Neugebauer, J.
NPJ COMPUTATIONAL MATERIALS. Volume: 4 (2018) - 2018 • 4757
Spatially resolved characterization of a dc magnetron plasma using optical emission spectroscopy
Ries, S. and Bibinov, N. and Rudolph, M. and Schulze, J. and Mráz, S. and Schneider, J.M. and Awakowicz, P.
PLASMA SOURCES SCIENCE AND TECHNOLOGY. Volume: 27 (2018) - 2018 • 4756
Energy conversion at nanointerfaces: General discussion
Alzahrani, H. and Bentley, C. and Bohn, P.W. and Chikere, C. and Commandeur, D. and Crooks, R.M. and Ehi-Eromosele, C. and Ewing, A. and Galeyeva, A. and Hersbach, T. and Hillman, R. and Kanoufi, F. and Koper, M. and Kranz, C. and Löffler, T. and Long, Y. and Macpherson, J. and McKelvey, K. and Minteer, S. and Mirkin, M. and Nichols, R. and Nogala, W. and Öhl, D. and Pelta, J. and Ren, H. and Rudd, J. and Schuhmann, W. and Tian, Z. and Unwin, P. and Vezzoli, A. and Willets, K. and Wu, Y. and Yang, Z. and Zhan, D. and Zhao, C.
FARADAY DISCUSSIONS. Volume: 210 (2018) - 2018 • 4755
Phase Delay of Terahertz Fabry-Perot Resonator characterized by a Photonic Two-Tone Spectroscopy System with Self-Heterodyne Receiver
Dulme, S. and Schrinski, N. and Steeg, M. and Lu, P. and Khani, B. and Brenner, C. and Hofmann, M.R. and Stöhr, A.
INTERNATIONAL CONFERENCE ON INFRARED, MILLIMETER, AND TERAHERTZ WAVES, IRMMW-THZ. Volume: 2018-September (2018) - 2018 • 4754
Processes at nanoelectrodes: General discussion
Alzahrani, H. and Antoine, C. and Aoki, K. and Baker, L. and Balme, S. and Bentley, C. and Bhattacharya, G. and Bohn, P.W. and Cai, Q. and Cao, C. and Commandeur, D. and Crooks, R.M. and Edwards, M. and Ewing, A. and Fu, K. and Galeyeva, A. and Gao, R. and Hersbach, T. and Hillman, R. and Hu, Y.-X. and Jiang, L. and Kanoufi, F. and Kranz, C. and Liu, S. and Löffler, T. and Long, Y. and Macpherson, J. and McKelvey, K. and Minteer, S. and Mirkin, M. and Mount, A. and Nichols, R. and Nogala, W. and Öhl, D. and Qiu, K. and Ren, H. and Rudd, J. and Schuhmann, W. and Siwy, Z. and Tian, Z. and Unwin, P. and Wang, Y. and Wilde, P. and Wu, Y. and Yang, Z. and Ying, Y.
FARADAY DISCUSSIONS. Volume: 210 (2018) - 2018 • 4753
Influence of Temperature and Electrolyte Concentration on the Structure and Catalytic Oxygen Evolution Activity of Nickel–Iron Layered Double Hydroxide
Andronescu, C. and Seisel, S. and Wilde, P. and Barwe, S. and Masa, J. and Chen, Y.-T. and Ventosa, E. and Schuhmann, W.
CHEMISTRY - A EUROPEAN JOURNAL. Volume: 24 (2018) - 2018 • 4752
Impact of Co and Fe Doping on the Martensitic Transformation and the Magnetic Properties in Ni-Mn-Based Heusler Alloys
Dutta, B. and Körmann, F. and Hickel, T. and Neugebauer, J.
PHYSICA STATUS SOLIDI (B) BASIC RESEARCH. Volume: 255 (2018) - 2018 • 4751
Multiscale modelling of hydrogen transport and segregation in polycrystalline steels
Hüter, C. and Shanthraj, P. and McEniry, E. and Spatschek, R. and Hickel, T. and Tehranchi, A. and Guo, X. and Roters, F.
METALS. Volume: 8 (2018) - 2018 • 4750
Overcoming Ehrlich-Schwöbel barrier in (1 1 1)A GaAs molecular beam epitaxy
Ritzmann, J. and Schott, R. and Gross, K. and Reuter, D. and Ludwig, Ar. and Wieck, A.D.
JOURNAL OF CRYSTAL GROWTH. Volume: 481 (2018) - 2018 • 4749
A set-up for simultaneous measurement of second harmonic generation and streaming potential and some test applications
Lützenkirchen, J. and Scharnweber, T. and Ho, T. and Striolo, A. and Sulpizi, M. and Abdelmonem, A.
JOURNAL OF COLLOID AND INTERFACE SCIENCE. Volume: 529 (2018) - 2018 • 4748
Corrosion behavior of an equiatomic CoCrFeMnNi high-entropy alloy compared with 304 stainless steel in sulfuric acid solution
Luo, H. and Li, Z. and Mingers, A.M. and Raabe, D.
CORROSION SCIENCE. Volume: (2018) - 2018 • 4747
Reduction of the ordered magnetic moment and its relationship to Kondo coherence in Ce1-xLaxCu2Ge2
Ueland, B.G. and Jo, N.H. and Sapkota, A. and Tian, W. and Masters, M. and Hodovanets, H. and Downing, S.S. and Schmidt, C. and McQueeney, R.J. and Bud'Ko, S.L. and Kreyssig, A. and Canfield, P.C. and Goldman, A.I.
PHYSICAL REVIEW B. Volume: 97 (2018) - 2018 • 4746
Oxidative Deposition of Manganese Oxide Nanosheets on Nitrogen-Functionalized Carbon Nanotubes Applied in the Alkaline Oxygen Evolution Reaction
Antoni, H. and Morales, D.M. and Fu, Q. and Chen, Y.-T. and Masa, J. and Schuhmann, W. and Muhler, M.
ACS OMEGA. Volume: 3 (2018) - 2018 • 4745
Influence of composition and crystal structure on the fracture toughness of NbCo2 Laves phase studied by micro-cantilever bending tests
Luo, W. and Kirchlechner, C. and Fang, X. and Brinckmann, S. and Dehm, G. and Stein, F.
MATERIALS AND DESIGN. Volume: 145 (2018) - 2018 • 4744
Hydrogen embrittlement of an interstitial equimolar high-entropy alloy
Luo, H. and Li, Z. and Lu, W. and Ponge, D. and Raabe, D.
CORROSION SCIENCE. Volume: 136 (2018) - 2018 • 4743
Impact of chemical inhomogeneities on local material properties and hydrogen environment embrittlement in AISI 304L steels
Egels, G. and Mujica Roncery, L. and Fussik, R. and Theisen, W. and Weber, S.
INTERNATIONAL JOURNAL OF HYDROGEN ENERGY. Volume: (2018) - 2018 • 4742
On Shear Testing of Single Crystal Ni-Base Superalloys
Eggeler, G. and Wieczorek, N. and Fox, F. and Berglund, S. and Bürger, D. and Dlouhy, A. and Wollgramm, P. and Neuking, K. and Schreuer, J. and Agudo Jácome, L. and Gao, S. and Hartmaier, A. and Laplanche, G.
METALLURGICAL AND MATERIALS TRANSACTIONS A: PHYSICAL METALLURGY AND MATERIALS SCIENCE. Volume: (2018) - 2018 • 4741
Trendbericht Anorganische Chemie 2017: Koordinationschemie und Bioanorganik
Apfel, U.-P. and Demir, S. and Berkefeld, A.
NACHRICHTEN AUS DER CHEMIE. Volume: 66 (2018) - 2018 • 4740
Elastically frustrated rehybridization: Origin of chemical order and compositional limits in InGaN quantum wells
Lymperakis, L. and Schulz, T. and Freysoldt, C. and Anikeeva, M. and Chen, Z. and Zheng, X. and Shen, B. and Chèze, C. and Siekacz, M. and Wang, X.Q. and Albrecht, M. and Neugebauer, J.
PHYSICAL REVIEW MATERIALS. Volume: 2 (2018) - 2018 • 4739
Quantitative ptychographic bio-imaging in the water window
Rose, M. and Senkbeil, T. and Von Gundlach, A.R. and Stuhr, S. and Rumancev, C. and Dzhigaev, D. and Besedin, I. and Skopintsev, P. and Loetgering, L. and Viefhaus, J. and Rosenhahn, A. and Vartanyants, I.A.
OPTICS EXPRESS. Volume: 26 (2018) - 2018 • 4738
Pathways to electrochemical solar-hydrogen technologies
Ardo, S. and Fernandez Rivas, D. and Modestino, M.A. and Schulze Greiving, V. and Abdi, F.F. and Alarcon Llado, E. and Artero, V. and Ayers, K. and Battaglia, C. and Becker, J.-P. and Bederak, D. and Berger, A. and Buda, F. and Chinello, E. and Dam, B. and Di Palma, V. and Edvinsson, T. and Fujii, K. and Gardeniers, H. and Geerlings, H. and Hashemi, S.M. and Haussener, S. and Houle, F. and Huskens, J. and James, B.D. and Konrad, K. and Kudo, A. and Kunturu, P.P. and Lohse, D. and Mei, B. and Miller, E.L. and Moore, G.F. and Muller, J. and Orchard, K.L. and Rosser, T.E. and Saadi, F.H. and Schüttauf, J.-W. and Seger, B. and Sheehan, S.W. and Smith, W.A. and Spurgeon, J. and Tang, M.H. and Van De Krol, R. and Vesborg, P.C.K. and Westerik, P.
ENERGY AND ENVIRONMENTAL SCIENCE. Volume: 11 (2018) - 2018 • 4737
Temperature-dependent phonon spectra of magnetic random solid solutions
Ikeda, Y. and Körmann, F. and Dutta, B. and Carreras, A. and Seko, A. and Neugebauer, J. and Tanaka, I.
NPJ COMPUTATIONAL MATERIALS. Volume: 4 (2018) - 2018 • 4736
Tailoring the Electrocaloric Effect by Internal Bias Fields and Field Protocols
Ma, Y.-B. and Xu, B.-X. and Albe, K. and Grünebohm, A.
PHYSICAL REVIEW APPLIED. Volume: 10 (2018) - 2018 • 4735
Impact of chemical fluctuations on stacking fault energies of CrCoNi and CrMnFeCoNi high entropy alloys from first principles
Ikeda, Y. and Körmann, F. and Tanaka, I. and Neugebauer, J.
ENTROPY. Volume: 20 (2018) - 2018 • 4734
Lithium as energy carrier: CFD simulations of LI combustion in a 100 MW slag tap furnace
Maas, P. and Schiemann, M. and Scherer, V. and Fischer, P. and Taroata, D. and Schmid, G.
APPLIED ENERGY. Volume: 227 (2018) - 2018 • 4733
Non-equilibrium excitation of CO2 in an atmospheric pressure helium plasma jet
Urbanietz, T. and Böke, M. and Schulz-Von Der Gathen, V. and Von Keudell, A.
JOURNAL OF PHYSICS D: APPLIED PHYSICS. Volume: 51 (2018) - 2017 • 4732
Competitive bcc and fcc crystal nucleation from non-equilibrium liquids studied by phase-field crystal simulation
Tang, S. and Wang, J.C. and Svendsen, B. and Raabe, D.
ACTA MATERIALIA. Volume: 139 (2017) - 2017 • 4731
First Principles Neural Network Potentials for Reactive Simulations of Large Molecular and Condensed Systems
Behler, J.
ANGEWANDTE CHEMIE - INTERNATIONAL EDITION. Volume: 56 (2017) - 2017 • 4730
Atomic/molecular layer deposition of hybrid inorganic–organic thin films from erbium guanidinate precursor
Mai, L. and Giedraityte, Z. and Schmidt, M. and Rogalla, D. and Scholz, S. and Wieck, A.D. and Devi, A. and Karppinen, M.
JOURNAL OF MATERIALS SCIENCE. Volume: 52 (2017) - 2017 • 4729
Robust antiferromagnetic spin waves across the metal-insulator transition in hole-doped BaMn2As2
Ramazanoglu, M. and Sapkota, A. and Pandey, A. and Lamsal, J. and Abernathy, D.L. and Niedziela, J.L. and Stone, M.B. and Kreyssig, A. and Goldman, A.I. and Johnston, D.C. and McQueeney, R.J.
PHYSICAL REVIEW B. Volume: 95 (2017) - 2017 • 4728
Unearthing [3-(Dimethylamino)propyl]aluminium(III) Complexes as Novel Atomic Layer Deposition (ALD) Precursors for Al2O3: Synthesis, Characterization and ALD Process Development
Mai, L. and Gebhard, M. and de los Arcos, T. and Giner, I. and Mitschker, F. and Winter, M. and Parala, H. and Awakowicz, P. and Grundmeier, G. and Devi, A.
CHEMISTRY - A EUROPEAN JOURNAL. Volume: 23 (2017) - 2017 • 4727
Microstructural evolution and functional fatigue of a Ti–25Ta high-temperature shape memory alloy
Maier, H.J. and Karsten, E. and Paulsen, A. and Langenkämper, D. and Decker, P. and Frenzel, J. and Somsen, C. and Ludwig, Al. and Eggeler, G. and Niendorf, T.
JOURNAL OF MATERIALS RESEARCH. Volume: (2017) - 2017 • 4726
Grain boundary character distribution in electroplated nanotwinned copper
Ratanaphan, S. and Raabe, D. and Sarochawikasit, R. and Olmsted, D.L. and Rohrer, G.S. and Tu, K.N.
JOURNAL OF MATERIALS SCIENCE. Volume: 52 (2017) - 2017 • 4725
The three-dimensional structure of flexible resorcinol-formaldehyde aerogels investigated by means of holotomography
Tannert, R. and Schwan, M. and Rege, A. and Eggeler, M. and da Silva, J.C. and Bartsch, M. and Milow, B. and Itskov, M. and Ratke, L.
JOURNAL OF SOL-GEL SCIENCE AND TECHNOLOGY. Volume: (2017) - 2017 • 4724
Ultrastrong steel via minimal lattice misfit and high-density nanoprecipitation
Jiang, S. and Wang, H. and Wu, Y. and Liu, X. and Chen, H. and Yao, M. and Gault, B. and Ponge, D. and Raabe, D. and Hirata, A. and Chen, M. and Wang, Y. and Lu, Z.
NATURE. Volume: (2017) - 2017 • 4723
Nanoindentation testing as a powerful screening tool for assessing phase stability of nanocrystalline high-entropy alloys
Maier-Kiener, V. and Schuh, B. and George, E.P. and Clemens, H. and Hohenwarter, A.
MATERIALS AND DESIGN. Volume: 115 (2017) - 2017 • 4722
Hydrogen-assisted failure in Ni-based superalloy 718 studied under in situ hydrogen charging: The role of localized deformation in crack propagation
Tarzimoghadam, Z. and Ponge, D. and Klöwer, J. and Raabe, D.
ACTA MATERIALIA. Volume: 128 (2017) - 2017 • 4721
Insights into the deformation behavior of the CrMnFeCoNi high-entropy alloy revealed by elevated temperature nanoindentation
Maier-Kiener, V. and Schuh, B. and George, E.P. and Clemens, H. and Hohenwarter, A.
JOURNAL OF MATERIALS RESEARCH. Volume: 32 (2017) - 2017 • 4720
Parallel multiphase field simulations with OpenPhase
Tegeler, M. and Shchyglo, O. and Kamachali, R.D. and Monas, A. and Steinbach, I. and Sutmann, G.
COMPUTER PHYSICS COMMUNICATIONS. Volume: 215 (2017) - 2017 • 4719
Optimal designs for dose response curves with common parameters
Feller, C. and Schorning, K. and Dette, H. and Bermann, G. and Bornkamp, B.
ANNALS OF STATISTICS. Volume: 45 (2017) - 2017 • 4718
Synthesis and characterization of germanosilicate molecular sieves: GeO2/SiO2 ratio, H2O/TO2 ratio and temperature
Jiao, K. and Zhang, Z. and Xu, X. and Lv, Z. and Song, J. and Lin, C. and Sun, J. and He, M. and Gies, H.
DALTON TRANSACTIONS. Volume: 46 (2017) - 2017 • 4717
Growth and characterization of BaZnGa
Jo, N.H. and Lin, Q. and Nguyen, M.C. and Kaluarachchi, U.S. and Meier, W.R. and Manni, S. and Downing, S.S. and Böhmer, A.E. and Kong, T. and Sun, Y. and Taufour, V. and Wang, C.-Z. and Ho, K.-M. and Bud’ko, S.L. and Canfield, P.C.
PHILOSOPHICAL MAGAZINE. Volume: 97 (2017) - 2017 • 4716
VUV absorption spectroscopy of bacterial spores and DNA components
Fiebrandt, M. and Lackmann, J.-W. and Raguse, M. and Moeller, R. and Awakowicz, P. and Stapelmann, K.
PLASMA PHYSICS AND CONTROLLED FUSION. Volume: 59 (2017) - 2017 • 4715
Enhanced power coupling efficiency in inductive discharges with RF substrate bias driven at consecutive harmonics with adjustable phase
Berger, B. and Steinberger, T. and Schüngel, E. and Koepke, M. and Mussenbrock, T. and Awakowicz, P. and Schulze, J.
APPLIED PHYSICS LETTERS. Volume: 111 (2017) - 2017 • 4714
Comparison of Langmuir probe and multipole resonance probe measurements in argon, hydrogen, nitrogen, and oxygen mixtures in a double ICP discharge
Fiebrandt, M. and Oberberg, M. and Awakowicz, P.
JOURNAL OF APPLIED PHYSICS. Volume: 122 (2017) - 2017 • 4713
Fast broadband reflectometer for diagnostics of plasma processes based on spatially distributed multipole resonance probes
Mallach, M. and Oberberg, M. and Awakowicz, P. and Musch, T.
IEEE MTT-S INTERNATIONAL MICROWAVE SYMPOSIUM DIGEST. Volume: (2017) - 2017 • 4712
Fabrication of Oxide Dispersion Strengthened Bond Coats with Low Al2O3 Content
Bergholz, J. and Pint, B.A. and Unocic, K.A. and Vaßen, R.
JOURNAL OF THERMAL SPRAY TECHNOLOGY. Volume: (2017) - 2017 • 4711
Measurement of Ar resonance and metastable level number densities in argon containing plasmas
Fiebrandt, M. and Hillebrand, B. and Spiekermeier, S. and Bibinov, N. and Böke, M. and Awakowicz, P.
JOURNAL OF PHYSICS D: APPLIED PHYSICS. Volume: 50 (2017) - 2017 • 4710
Dislocation-twin boundary interaction in small scale Cu bi-crystals loaded in different crystallographic directions
Malyar, N.V. and Micha, J.-S. and Dehm, G. and Kirchlechner, C.
ACTA MATERIALIA. Volume: 129 (2017) - 2017 • 4709
Microstructural stability of a niobium single crystal deformed by equal channel angular pressing
Bernardi, H.H. and Sandim, H.R.Z. and Zilnyk, K.D. and Verlinden, B. and Raabe, D.
MATERIALS RESEARCH. Volume: 20 (2017) - 2017 • 4708
Depth-filtering in common-path digital holographic microscopy
Finkeldey, M. and Göring, L. and Brenner, C. and Hofmann, M. and Gerhardt, N.C.
OPTICS EXPRESS. Volume: 25 (2017) - 2017 • 4707
Size effect in bi-crystalline micropillars with a penetrable high angle grain boundary
Malyar, N.V. and Micha, J.S. and Dehm, G. and Kirchlechner, C.
ACTA MATERIALIA. Volume: 129 (2017) - 2017 • 4706
Effect of temperature on the fatigue-crack growth behavior of the high-entropy alloy CrMnFeCoNi
Thurston, K.V.S. and Gludovatz, B. and Hohenwarter, A. and Laplanche, G. and George, E.P. and Ritchie, R.O.
INTERMETALLICS. Volume: 88 (2017) - 2017 • 4705
Backside imaging of a microcontroller with common-path digital holography
Finkeldey, M. and Göring, L. and Schellenberg, F. and Gerhardt, N.C. and Hofmann, M.
PROCEEDINGS OF SPIE - THE INTERNATIONAL SOCIETY FOR OPTICAL ENGINEERING. Volume: 10127 (2017) - 2017 • 4704
Strain rate dependence of the slip transfer through a penetrable high angle grain boundary in copper
Malyar, N.V. and Dehm, G. and Kirchlechner, C.
SCRIPTA MATERIALIA. Volume: 138 (2017) - 2017 • 4703
Multimodal backside imaging of a microcontroller using confocal laser scanning and optical-beam-induced current imaging
Finkeldey, M. and Göring, L. and Schellenberg, F. and Brenner, C. and Gerhardt, N.C. and Hofmann, M.
PROCEEDINGS OF SPIE - THE INTERNATIONAL SOCIETY FOR OPTICAL ENGINEERING. Volume: 10110 (2017) - 2017 • 4702
Piezospectroscopic evaluation and damage identification for thermal barrier coatings subjected to simulated engine environments
Manero, A. and Selimov, A. and Fouliard, Q. and Knipe, K. and Wischek, J. and Meid, C. and Karlsson, A.M. and Bartsch, M. and Raghavan, S.
SURFACE AND COATINGS TECHNOLOGY. Volume: 323 (2017) - 2017 • 4701
Common-path holographic objective for conventional photographic camera
Besaga, V.R. and Gerhardt, N.C. and Maksimyak, P.P. and Hofmann, M.R.
PROCEEDINGS OF SPIE - THE INTERNATIONAL SOCIETY FOR OPTICAL ENGINEERING. Volume: 10376 (2017) - 2017 • 4700
A relaxation-based approach to damage modeling
Junker, P. and Schwarz, S. and Makowski, J. and Hackl, K.
CONTINUUM MECHANICS AND THERMODYNAMICS. Volume: 29 (2017) - 2017 • 4699
Investigation of the resistance of open-column-structured PS-PVD TBCs to erosive and high-temperature corrosive attack
Rezanka, S. and Mack, D.E. and Mauer, G. and Sebold, D. and Guillon, O. and Vaßen, R.
SURFACE AND COATINGS TECHNOLOGY. Volume: 324 (2017) - 2017 • 4698
Sequential kinetic modelling: A new tool decodes pulsed tectonic patterns in early hot orogens of Earth
Bhowmik, S.K. and Chakraborty, S.
EARTH AND PLANETARY SCIENCE LETTERS. Volume: 460 (2017) - 2017 • 4697
The relaxed-polar mechanism of locally optimal Cosserat rotations for an idealized nanoindentation and comparison with 3D-EBSD experiments
Fischle, A. and Neff, P. and Raabe, D.
ZEITSCHRIFT FUR ANGEWANDTE MATHEMATIK UND PHYSIK. Volume: 68 (2017) - 2017 • 4696
Coherent and robust high-fidelity generation of a biexciton in a quantum dot by rapid adiabatic passage
Kaldewey, T. and Lüker, S. and Kuhlmann, A.V. and Valentin, S.R. and Ludwig, Ar. and Wieck, A.D. and Reiter, D.E. and Kuhn, T. and Warburton, R.J.
PHYSICAL REVIEW B - CONDENSED MATTER AND MATERIALS PHYSICS. Volume: 95 (2017) - 2017 • 4695
Wavelength dependence of picosecond laser-induced periodic surface structures on copper
Maragkaki, S. and Derrien, T.J.-Y. and Levy, Y. and Bulgakova, N.M. and Ostendorf, A. and Gurevich, E.L.
APPLIED SURFACE SCIENCE. Volume: 417 (2017) - 2017 • 4694
Demonstrating the decoupling regime of the electron-phonon interaction in a quantum dot using chirped optical excitation
Kaldewey, T. and Lüker, S. and Kuhlmann, A.V. and Valentin, S.R. and Chauveau, J.-M. and Ludwig, Ar. and Wieck, A.D. and Reiter, D.E. and Kuhn, T. and Warburton, R.J.
PHYSICAL REVIEW B. Volume: 95 (2017) - 2017 • 4693
Electrocaloric effect in BaTiO3 at all three ferroelectric transitions: Anisotropy and inverse caloric effects
Marathe, M. and Renggli, D. and Sanlialp, M. and Karabasov, M.O. and Shvartsman, V.V. and Lupascu, D.C. and Grünebohm, A. and Ederer, C.
PHYSICAL REVIEW B. Volume: 96 (2017) - 2017 • 4692
Tailored Mesoporous Carbon/Vanadium Pentoxide Hybrid Electrodes for High Power Pseudocapacitive Lithium and Sodium Intercalation
Fleischmann, S. and Leistenschneider, D. and Lemkova, V. and Krüner, B. and Zeiger, M. and Borchardt, L. and Presser, V.
CHEMISTRY OF MATERIALS. Volume: 29 (2017) - 2017 • 4691
Pressure-induced half-collapsed-tetragonal phase in CaKFe4As4
Kaluarachchi, U.S. and Taufour, V. and Sapkota, A. and Borisov, V. and Kong, T. and Meier, W.R. and Kothapalli, K. and Ueland, B.G. and Kreyssig, A. and Valentí, R. and McQueeney, R.J. and Goldman, A.I. and Bud'Ko, S.L. and Canfield, P.C.
PHYSICAL REVIEW B. Volume: 96 (2017) - 2017 • 4690
Manufacturing of high performance solid oxide fuel cells (SOFCs) with atmospheric plasma spraying (APS) and plasma spray-physical vapor deposition (PS-PVD)
Marcano, D. and Mauer, G. and Vaßen, R. and Weber, A.
SURFACE AND COATINGS TECHNOLOGY. Volume: 318 (2017) - 2017 • 4689
A linear triple quantum dot system in isolated configuration
Flentje, H. and Bertrand, B. and Mortemousque, P.-A. and Thiney, V. and Ludwig, Ar. and Wieck, A.D. and Bäuerle, C. and Meunier, T.
APPLIED PHYSICS LETTERS. Volume: 110 (2017) - 2017 • 4688
Coherent long-distance displacement of individual electron spins
Flentje, H. and Mortemousque, P.-A. and Thalineau, R. and Ludwig, Ar. and Wieck, A.D. and Bäuerle, C. and Meunier, T.
NATURE COMMUNICATIONS. Volume: 8 (2017) - 2017 • 4687
Two-dimensional coherent spectroscopy of a THz quantum cascade laser: Observation of multiple harmonics
Markmann, S. and Nong, H. and Pal, S. and Fobbe, T. and Hekmat, N. and Mohandas, R.A. and Dean, P. and Li, L. and Linfield, E.H. and Davies, A.G. and Wieck, A.D. and Jukam, N.
OPTICS EXPRESS. Volume: 25 (2017) - 2017 • 4686
Redox Induced Configurational Isomerization of Bisphosphine-Tricarbonyliron(I) Complexes and the Difference a Ferrocene Makes
Ringenberg, M.R. and Wittkamp, F. and Apfel, U.-P. and Kaim, W.
INORGANIC CHEMISTRY. Volume: 56 (2017) - 2017 • 4685
Hybrid approach for two dimensional damage localization using piezoelectric smart aggregates
Marković, N. and Nestorović, T. and Stojić, D. and Marjanović, M. and Stojković, N.
MECHANICS RESEARCH COMMUNICATIONS. Volume: 85 (2017) - 2017 • 4684
Organometallic Fe–Fe Interactions: Beyond Common Metal–Metal Bonds and Inverse Mixed-Valent Charge Transfer
Ringenberg, M.R. and Schwilk, M. and Wittkamp, F. and Apfel, U.-P. and Kaim, W.
CHEMISTRY - A EUROPEAN JOURNAL. Volume: 23 (2017) - 2017 • 4683
The shear instability energy: A new parameter for materials design?
Kanani, M. and Hartmaier, A. and Janisch, R.
MODELLING AND SIMULATION IN MATERIALS SCIENCE AND ENGINEERING. Volume: 25 (2017) - 2017 • 4682
Oxygen activity and peroxide formation as charge compensation mechanisms in Li2MnO3
Marusczyk, A. and Albina, J.-M. and Hammerschmidt, T. and Drautz, R. and Eckl, T. and Henkelman, G.
JOURNAL OF MATERIALS CHEMISTRY A. Volume: 5 (2017) - 2017 • 4681
Effects of Potassium and Manganese Promoters on Nitrogen-Doped Carbon Nanotube-Supported Iron Catalysts for CO2 Hydrogenation
Kangvansura, P. and Chew, L.M. and Kongmark, C. and Santawaja, P. and Ruland, H. and Xia, W. and Schulz, H. and Worayingyong, A. and Muhler, M.
ENGINEERING. Volume: 3 (2017) - 2017 • 4680
Effect of annealing on the size dependent deformation behavior of thin cobalt films on flexible substrates
Marx, V.M. and Cordill, M.J. and Többens, D.M. and Kirchlechner, C. and Dehm, G.
THIN SOLID FILMS. Volume: 624 (2017) - 2017 • 4679
Influence of solvent mixture on the ablation rate of iron using femtosecond laser pulses
Kanitz, A. and Hoppius, J.S. and Ostendorf, A. and Gurevich, E.L.
PROCEEDINGS OF SPIE - THE INTERNATIONAL SOCIETY FOR OPTICAL ENGINEERING. Volume: 10092 (2017) - 2017 • 4678
Recurrent neural networks and proper orthogonal decomposition with interval data for real-time predictions of mechanised tunnelling processes
Freitag, S. and Cao, B.T. and Ninić, J. and Meschke, G.
COMPUTERS AND STRUCTURES. Volume: (2017) - 2017 • 4677
Impact of liquid environment on femtosecond laser ablation
Kanitz, A. and Hoppius, J.S. and Fiebrandt, M. and Awakowicz, P. and Esen, C. and Ostendorf, A. and Gurevich, E.L.
APPLIED PHYSICS A: MATERIALS SCIENCE AND PROCESSING. Volume: 123 (2017) - 2017 • 4676
Synthesis of Magnetic Nanoparticles by Ultrashort Pulsed Laser Ablation of Iron in Different Liquids
Kanitz, A. and Hoppius, J.S. and del Mar Sanz, M. and Maicas, M. and Ostendorf, A. and Gurevich, E.L.
CHEMPHYSCHEM. Volume: 18 (2017) - 2017 • 4675
Ultrathin High Surface Area Nickel Boride (NixB) Nanosheets as Highly Efficient Electrocatalyst for Oxygen Evolution
Masa, J. and Sinev, I. and Mistry, H. and Ventosa, E. and de la Mata, M. and Arbiol, J. and Muhler, M. and Roldan Cuenya, B. and Schuhmann, W.
ADVANCED ENERGY MATERIALS. Volume: (2017) - 2017 • 4674
Symposium on Theoretical Chemistry 2016: Chemistry in Solution [Symposium für theoretische Chemie 2016: Chemie in Lösung]
Römelt, M. and Behler, J.
NACHRICHTEN AUS DER CHEMIE. Volume: 65 (2017) - 2017 • 4673
Quantile spectral analysis for locally stationary time series
Birr, S. and Volgushev, S. and Kley, T. and Dette, H. and Hallin, M.
JOURNAL OF THE ROYAL STATISTICAL SOCIETY. SERIES B: STATISTICAL METHODOLOGY. Volume: 79 (2017) - 2017 • 4672
Synthesis and evaluation of new copper ketoiminate precursors for a facile and additive-free solution-based approach to nanoscale copper oxide thin films
Karle, S. and Rogalla, D. and Ludwig, A. and Becker, H. and Wieck, A. D. and Grafen, M. and Ostendorf, A. and Devi, A.
DALTON TRANSACTIONS. Volume: 46 (2017) - 2017 • 4671
Impact of processing conditions and feedstock characteristics on thermally sprayed MCrAlY bondcoat properties
Mauer, G. and Sebold, D. and Vaßen, R. and Hejrani, E. and Naumenko, D. and Quadakkers, W.J.
SURFACE AND COATINGS TECHNOLOGY. Volume: 318 (2017) - 2017 • 4670
The role of κ-carbides as hydrogen traps in high-Mn steels
Timmerscheidt, T.A. and Dey, P. and Bogdanovski, D. and von Appen, J. and Hickel, T. and Neugebauer, J. and Dronskowski, R.
METALS. Volume: 7 (2017) - 2017 • 4669
Recent developments in plasma spray processes for applications in energy technology
Mauer, G. and Jarligo, M.O. and Marcano, D. and Rezanka, S. and Zhou, D. and Vaßen, R.
IOP CONFERENCE SERIES: MATERIALS SCIENCE AND ENGINEERING. Volume: 181 (2017) - 2017 • 4668
Cascade continuum micromechanics model for the effective permeability of solids with distributed microcracks: Self-similar mean-field homogenization and image analysis
Timothy, J.J. and Meschke, G.
MECHANICS OF MATERIALS. Volume: 104 (2017) - 2017 • 4667
Diagnostics of Cold-Sprayed Particle Velocities Approaching Critical Deposition Conditions
Mauer, G. and Singh, R. and Rauwald, K.-H. and Schrüfer, S. and Wilson, S. and Vaßen, R.
JOURNAL OF THERMAL SPRAY TECHNOLOGY. Volume: (2017) - 2017 • 4666
The intrinsic permeability of microcracks in porous solids: Analytical models and Lattice Boltzmann simulations
Timothy, J.J. and Meschke, G.
INTERNATIONAL JOURNAL FOR NUMERICAL AND ANALYTICAL METHODS IN GEOMECHANICS. Volume: 41 (2017) - 2017 • 4665
Monitoring and Improving the Reliability of Plasma Spray Processes
Mauer, G. and Rauwald, K.-H. and Mücke, R. and Vaßen, R.
JOURNAL OF THERMAL SPRAY TECHNOLOGY. Volume: 26 (2017) - 2017 • 4664
Encapsulation of Bimetallic Metal Nanoparticles into Robust Zirconium-Based Metal-Organic Frameworks: Evaluation of the Catalytic Potential for Size-Selective Hydrogenation
Rösler, C. and Dissegna, S. and Rechac, V.L. and Kauer, M. and Guo, P. and Turner, S. and Ollegott, K. and Kobayashi, H. and Yamamoto, T. and Peeters, D. and Wang, Y. and Matsumura, S. and Van Tendeloo, G. and Kitagawa, H. and Muhler, M. and Llabrés i Xamena, F.X. and Fischer, R.A.
CHEMISTRY - A EUROPEAN JOURNAL. Volume: 23 (2017) - 2017 • 4663
Characterization of DC magnetron plasma in Ar/Kr/N2 mixture during deposition of (Cr,Al)N coating
Bobzin, K. and Bagcivan, N. and Theiß, S. and Brugnara, R. and Bibinov, N. and Awakowicz, P.
JOURNAL OF PHYSICS D: APPLIED PHYSICS. Volume: 50 (2017) - 2017 • 4662
Investigating the detrimental effects of low pressure plasma sterilization on the survival of bacillus subtilis spores using live cell microscopy
Fuchs, F.M. and Raguse, M. and Fiebrandt, M. and Madela, K. and Awakowicz, P. and Laue, M. and Stapelmann, K. and Moeller, R.
JOURNAL OF VISUALIZED EXPERIMENTS. Volume: 2017 (2017) - 2017 • 4661
Fundamental study of an industrial reactive HPPMS (Cr,Al)N process
Bobzin, K. and Brögelmann, T. and Kruppe, N.C. and Engels, M. and Von Keudell, A. and Hecimovic, A. and Ludwig, Al. and Grochla, D. and Banko, L.
JOURNAL OF APPLIED PHYSICS. Volume: 122 (2017) - 2017 • 4660
Graphene oxide reduction induced by femtosecond laser irradiation
Kasischke, M. and Maragkaki, S. and Volz, S. and Gurevich, E.L. and Ostendorf, A.
PROCEEDINGS OF SPIE - THE INTERNATIONAL SOCIETY FOR OPTICAL ENGINEERING. Volume: 10356 (2017) - 2017 • 4659
High-dynamic-range water window ptychography
Rose, M. and Dzhigaev, D. and Senkbeil, T. and Von Gundlach, A.R. and Stuhr, S. and Rumancev, C. and Besedin, I. and Skopintsev, P. and Viefhaus, J. and Rosenhahn, A. and Vartanyants, I.A.
JOURNAL OF PHYSICS: CONFERENCE SERIES. Volume: 849 (2017) - 2017 • 4658
Influence of Substrate Temperature and Film Thickness on Thermal, Electrical, and Structural Properties of HPPMS and DC Magnetron Sputtered Ge Thin Films
Furlan, A. and Grochla, D. and D'Acremont, Q. and Pernot, G. and Dilhaire, S. and Ludwig, Al.
ADVANCED ENGINEERING MATERIALS. Volume: (2017) - 2017 • 4657
In situ and operando observation of surface oxides during oxygen evolution reaction on copper
Toparli, C. and Sarfraz, A. and Wieck, A.D. and Rohwerder, M. and Erbe, A.
ELECTROCHIMICA ACTA. Volume: 236 (2017) - 2017 • 4656
Atomistic Simulations of the Crystallization and Aging of GeTe Nanowires
Gabardi, S. and Baldi, E. and Bosoni, E. and Campi, D. and Caravati, S. and Sosso, G.C. and Behler, J. and Bernasconi, M.
JOURNAL OF PHYSICAL CHEMISTRY C. Volume: 121 (2017) - 2017 • 4655
Hydrogen behaviour at twist {110} grain boundaries in α-Fe
McEniry, E.J. and Hickel, T. and Neugebauer, J.
PHILOSOPHICAL TRANSACTIONS OF THE ROYAL SOCIETY A: MATHEMATICAL, PHYSICAL AND ENGINEERING SCIENCES. Volume: 375 (2017) - 2017 • 4654
Micromechanical modeling of fatigue crack initiation in polycrystals
Boeff, M. and Hassan, H.U. and Hartmaier, A.
JOURNAL OF MATERIALS RESEARCH. Volume: (2017) - 2017 • 4653
Effect of Biaxial Strain on the Phase Transitions of Ca (Fe1-xCox)2As2
Böhmer, A.E. and Sapkota, A. and Kreyssig, A. and Bud'Ko, S.L. and Drachuck, G. and Saunders, S.M. and Goldman, A.I. and Canfield, P.C.
PHYSICAL REVIEW LETTERS. Volume: 118 (2017) - 2017 • 4652
Bridging Hydride at Reduced H-Cluster Species in [FeFe]-Hydrogenases Revealed by Infrared Spectroscopy, Isotope Editing, and Quantum Chemistry
Mebs, S. and Senger, M. and Duan, J. and Wittkamp, F. and Apfel, U.-P. and Happe, T. and Winkler, M. and Stripp, S.T. and Haumann, M.
JOURNAL OF THE AMERICAN CHEMICAL SOCIETY. Volume: 139 (2017) - 2017 • 4651
Compressed Bi-crystal micropillars showing a sigmoidal deformation state – A computational study
Toth, F. and Kirchlechner, C. and Fischer, F.D. and Dehm, G. and Rammerstorfer, F.G.
MATERIALS SCIENCE AND ENGINEERING A. Volume: 700 (2017) - 2017 • 4650
S+is superconductivity with incipient bands: Doping dependence and STM signatures
Böker, J. and Volkov, P.A. and Efetov, K.B. and Eremin, I.
PHYSICAL REVIEW B. Volume: 96 (2017) - 2017 • 4649
A global model of cylindrical and coaxial surface-wave discharges
Kemaneci, E. and Mitschker, F. and Rudolph, M. and Szeremley, D. and Eremin, D. and Awakowicz, P. and Peter Brinkmann, R.
JOURNAL OF PHYSICS D: APPLIED PHYSICS. Volume: 50 (2017) - 2017 • 4648
Ion-induced interdiffusion of surface GaN quantum dots
Rothfuchs, C. and Semond, F. and Portail, M. and Tottereau, O. and Courville, A. and Wieck, A.D. and Ludwig, Ar.
NUCLEAR INSTRUMENTS AND METHODS IN PHYSICS RESEARCH, SECTION B: BEAM INTERACTIONS WITH MATERIALS AND ATOMS. Volume: 409 (2017) - 2017 • 4647
A Self-Powered Ethanol Biosensor
Ruff, A. and Pinyou, P. and Nolten, M. and Conzuelo, F. and Schuhmann, W.
CHEMELECTROCHEM. Volume: 4 (2017) - 2017 • 4646
Material dependence of the contact behavior of oscillating microprobes-modeling and experimental evidence
Bohm, S. and Goj, B. and Dittrich, L. and Dressler, L. and Hoffmann, M.
JOURNAL OF MICRO AND NANO-MANUFACTURING. Volume: 5 (2017) - 2017 • 4645
Chalcogenide substitution in the [2Fe] cluster of [FeFe]-hydrogenases conserves high enzymatic activity
Kertess, L. and Wittkamp, F. and Sommer, C. and Esselborn, J. and Rüdiger, O. and Reijerse, E.J. and Hofmann, E. and Lubitz, W. and Winkler, M. and Happe, T. and Apfel, U.-P.
DALTON TRANSACTIONS. Volume: 46 (2017) - 2017 • 4644
Nanophase Segregation of Self-Assembled Monolayers on Gold Nanoparticles
Meena, S.K. and Goldmann, C. and Nassoko, D. and Seydou, M. and Marchandier, T. and Moldovan, S. and Ersen, O. and Ribot, F. and Chanéac, C. and Sanchez, C. and Portehault, D. and Tielens, F. and Sulpizi, M.
ACS NANO. Volume: 11 (2017) - 2017 • 4643
Protection and Reactivation of the [NiFeSe] Hydrogenase from Desulfovibrio vulgaris Hildenborough under Oxidative Conditions
Ruff, A. and Szczesny, J. and Zacarias, S. and Pereira, I.A.C. and Plumeré, N. and Schuhmann, W.
ACS ENERGY LETTERS. Volume: 2 (2017) - 2017 • 4642
Kinetic analysis of negative power deposition in inductive low pressure plasmas
Trieschmann, J. and Mussenbrock, T.
PLASMA SOURCES SCIENCE AND TECHNOLOGY. Volume: 26 (2017) - 2017 • 4641
Morphological analysis of cerium oxide stabilized nanoporous gold catalysts by soft X-ray ASAXS
Rumancev, C. and Von Gundlach, A.R. and Baier, S. and Wittstock, A. and Shi, J. and Benzi, F. and Senkbeil, T. and Stuhr, S. and Garamusx, V.M. and Grunwaldt, J.-D. and Rosenhahn, A.
RSC ADVANCES. Volume: 7 (2017) - 2017 • 4640
Particle-in-Cell/Test-Particle Simulations of Technological Plasmas: Sputtering Transport in Capacitive Radio Frequency Discharges
Trieschmann, J. and Schmidt, F. and Mussenbrock, T.
PLASMA PROCESSES AND POLYMERS. Volume: 14 (2017) - 2017 • 4639
On the pH Dependence of the Potential of Maximum Entropy of Ir(111) Electrodes
Ganassin, A. and Sebastian, P. and Climent, V. and Schuhmann, W. and Bandarenka, A.S. and Feliu, J.
SCIENTIFIC REPORTS. Volume: 7 (2017) - 2017 • 4638
Beyond Water Splitting: Efficiencies of Photo-Electrochemical Devices Producing Hydrogen and Valuable Oxidation Products
Mei, B. and Mul, G. and Seger, B.
ADVANCED SUSTAINABLE SYSTEMS. Volume: 1 (2017) - 2017 • 4637
Mechanochemical Friedel–Crafts Alkylation—A Sustainable Pathway Towards Porous Organic Polymers
Troschke, E. and Grätz, S. and Lübken, T. and Borchardt, L.
ANGEWANDTE CHEMIE - INTERNATIONAL EDITION. Volume: 56 (2017) - 2017 • 4636
Coverage-Induced Conformational Selectivity
Boom, K. and Stein, F. and Ernst, S. and Morgenstern, K.
JOURNAL OF PHYSICAL CHEMISTRY C. Volume: 121 (2017) - 2017 • 4635
Bioactivity and electrochemical behavior of hydroxyapatite-silicon-multi walled carbon nano-tubes composite coatings synthesized by EPD on NiTi alloys in simulated body fluid
Khalili, V. and Khalil-Allafi, J. and Frenzel, J. and Eggeler, G.
MATERIALS SCIENCE AND ENGINEERING C. Volume: 71 (2017) - 2017 • 4634
Salt templated synthesis of hierarchical covalent triazine frameworks
Troschke, E. and Grätz, S. and Borchardt, L. and Haubold, D. and Senkovska, I. and Eychmueller, A. and Kaskel, S.
MICROPOROUS AND MESOPOROUS MATERIALS. Volume: 239 (2017) - 2017 • 4633
Toward a molecular design of porous carbon materials
Borchardt, L. and Zhu, Q.-L. and Casco, M.E. and Berger, R. and Zhuang, X. and Kaskel, S. and Feng, X. and Xu, Q.
MATERIALS TODAY. Volume: 20 (2017) - 2017 • 4632
Rejuvenation of creep resistance of a Ni-base single-crystal superalloy by hot isostatic pressing
Ruttert, B. and Bürger, D. and Roncery, L.M. and Parsa, A.B. and Wollgramm, P. and Eggeler, G. and Theisen, W.
MATERIALS AND DESIGN. Volume: 134 (2017) - 2017 • 4631
Multiphase Characterization of Cu-In-Sn Alloys with 17 at.% Cu and Comparison with Calculated Phase Equilibria
Tumminello, S. and Del Negro, N. and Carrascal, C. and Fries, S.G. and Alonso, P.R. and Sommadossi, S.
JOURNAL OF PHASE EQUILIBRIA AND DIFFUSION. Volume: 38 (2017) - 2017 • 4630
Carbon nano-composites for lithium–sulfur batteries
Borchardt, L. and Althues, H. and Kaskel, S.
CURRENT OPINION IN GREEN AND SUSTAINABLE CHEMISTRY. Volume: 4 (2017) - 2017 • 4629
Oxidative photo-deposition of chromia: Tuning the activity for overall water splitting of the Rh/CrO: X co-catalyst system
Menze, J. and Mei, B. and Weide, P. and Muhler, M.
JOURNAL OF MATERIALS CHEMISTRY A. Volume: 5 (2017) - 2017 • 4628
3D discrete dislocation dynamics study of creep behavior in Ni-base single crystal superalloys by a combined dislocation climb and vacancy diffusion model
Gao, S. and Fivel, M. and Ma, A. and Hartmaier, A.
JOURNAL OF THE MECHANICS AND PHYSICS OF SOLIDS. Volume: 102 (2017) - 2017 • 4627
Combinatorial synthesis and high-throughput characterization of structural and photoelectrochemical properties of Fe:WO3 nanostructured libraries
Khare, C. and Sliozberg, K. and Stepanovich, A. and Schuhmann, W. and Ludwig, Al.
NANOTECHNOLOGY. Volume: 28 (2017) - 2017 • 4626
Diffusion-related SOFC stack degradation
Menzler, N.H. and Beez, A. and Grünwald, N. and Sebold, D. and Fang, Q. and Vaßen, R.
ECS TRANSACTIONS. Volume: 78 (2017) - 2017 • 4625
Sum Frequency Generation Spectra from Velocity-Velocity Correlation Functions
Khatib, R. and Sulpizi, M.
JOURNAL OF PHYSICAL CHEMISTRY LETTERS. Volume: 8 (2017) - 2017 • 4624
Simultaneous real-Time application and direct comparison of optical resonance sensing and fluorescence tagging techniques for biochemical component detection
Saetchnikov, A.V. and Saetchnikov, V.A. and Tcherniavskaia, E.A. and Ostendorf, A.
PROCEEDINGS OF SPIE - THE INTERNATIONAL SOCIETY FOR OPTICAL ENGINEERING. Volume: 10333 (2017) - 2017 • 4623
Microscopic insights into the fluorite/water interfaces from vibrational sum frequency generation spectroscopy
Khatib, R. and Sulpizi, M.
HIGH PERFORMANCE COMPUTING IN SCIENCE AND ENGINEERING '16: TRANSACTIONS OF THE HIGH PERFORMANCE COMPUTING CENTER STUTTGART (HLRS) 2016. Volume: (2017) - 2017 • 4622
Improved CO2 Electroreduction Performance on Plasma-Activated Cu Catalysts via Electrolyte Design: Halide Effect
Gao, D. and Scholten, F. and Roldan Cuenya, B.
ACS CATALYSIS. Volume: 7 (2017) - 2017 • 4621
Plasma-Activated Copper Nanocube Catalysts for Efficient Carbon Dioxide Electroreduction to Hydrocarbons and Alcohols
Gao, D. and Zegkinoglou, I. and Divins, N.J. and Scholten, F. and Sinev, I. and Grosse, P. and Roldan Cuenya, B.
ACS NANO. Volume: 11 (2017) - 2017 • 4620
On the competition between the stress-induced formation of martensite and dislocation plasticity during crack propagation in pseudoelastic NiTi shape memory alloys
Ungár, T. and Frenzel, J. and Gollerthan, S. and Ribárik, G. and Balogh, L. and Eggeler, G.
JOURNAL OF MATERIALS RESEARCH. Volume: (2017) - 2017 • 4619
Opto-electrochemical In Situ Monitoring of the Cathodic Formation of Single Cobalt Nanoparticles
Brasiliense, V. and Clausmeyer, J. and Dauphin, A.L. and Noël, J.-M. and Berto, P. and Tessier, G. and Schuhmann, W. and Kanoufi, F.
ANGEWANDTE CHEMIE - INTERNATIONAL EDITION. Volume: 56 (2017) - 2017 • 4618
Influence of nitrogen admixture to argon on the ion energy distribution in reactive high power pulsed magnetron sputtering of chromium
Breilmann, W. and Maszl, C. and Hecimovic, A. and Von Keudell, A.
JOURNAL OF PHYSICS D: APPLIED PHYSICS. Volume: 50 (2017) - 2017 • 4617
Simulation of the effect of the porous support on flux through an asymmetric oxygen transport membrane
Unije, U. and Mücke, R. and Niehoff, P. and Baumann, S. and Vaßen, R. and Guillon, O.
JOURNAL OF MEMBRANE SCIENCE. Volume: 524 (2017) - 2017 • 4616
Fast charge exchange ions in high power impulse magnetron sputtering of titanium as probes for the electrical potential
Breilmann, W. and Maszl, C. and von Keudell, A.
PLASMA SOURCES SCIENCE & TECHNOLOGY. Volume: 26 (2017) - 2017 • 4615
Complete Prevention of Dendrite Formation in Zn Metal Anodes by Means of Pulsed Charging Protocols
Garcia, G. and Ventosa, E. and Schuhmann, W.
ACS APPLIED MATERIALS AND INTERFACES. Volume: 9 (2017) - 2017 • 4614
Mössbauer spectroscopy evidence of intrinsic non-stoichiometry in iron telluride single crystals
Kiiamov, A.G. and Lysogorskiy, Y.V. and Vagizov, F.G. and Tagirov, L.R. and Tayurskii, D.A. and Croitori, D. and Tsurkan, V. and Loidl, A.
ANNALEN DER PHYSIK. Volume: 529 (2017) - 2017 • 4613
Alkali-silica reaction in concrete structures due to simultaneous cyclic loading and external supply of alkali: Results of the DFG research group FOR 1498
Breitenbücher, R.
STRUCTURAL CONCRETE. Volume: (2017) - 2017 • 4612
Machine learning molecular dynamics for the simulation of infrared spectra
Gastegger, M. and Behler, J. and Marquetand, P.
CHEMICAL SCIENCE. Volume: 8 (2017) - 2017 • 4611
Low-Temperature Atomic Layer Deposition of Cobalt Oxide as an Effective Catalyst for Photoelectrochemical Water-Splitting Devices
Kim, J. and Iivonen, T. and Hämäläinen, J. and Kemell, M. and Meinander, K. and Mizohata, K. and Wang, L. and Räisänen, J. and Beranek, R. and Leskelä, M. and Devi, A.
CHEMISTRY OF MATERIALS. Volume: 29 (2017) - 2017 • 4610
Scanning tunnelling spectroscopy as a probe of multi-Q magnetic states of itinerant magnets
Gastiasoro, M.N. and Eremin, I. and Fernandes, R.M. and Andersen, B.M.
NATURE COMMUNICATIONS. Volume: 8 (2017) - 2017 • 4609
Diffusional-displacive transformation enables formation of long-period stacking order in magnesium
Kim, J.-K. and Jin, L. and Sandlöbes, S. and Raabe, D.
SCIENTIFIC REPORTS. Volume: 7 (2017) - 2017 • 4608
Stress intensity factor dependence on anisotropy and geometry during micro-fracture experiments
Brinckmann, S. and Kirchlechner, C. and Dehm, G.
SCRIPTA MATERIALIA. Volume: 127 (2017) - 2017 • 4607
New amidinate complexes of indium(III): Promising CVD precursors for transparent and conductive In2O3 thin films
Gebhard, M. and Hellwig, M. and Kroll, A. and Rogalla, D. and Winter, M. and Mallick, B. and Ludwig, Ar. and Wiesing, M. and Wieck, A.D. and Grundmeier, G. and Devi, A.
DALTON TRANSACTIONS. Volume: 46 (2017) - 2017 • 4606
Modulation of plastic flow in metallic glasses via nanoscale networks of chemical heterogeneities
Kim, J. and Oh, H.S. and Kim, W. and Choi, P.-P. and Raabe, D. and Park, E.S.
ACTA MATERIALIA. Volume: 140 (2017) - 2017 • 4605
Micromechanical modeling approach to derive the yield surface for BCC and FCC steels using statistically informed microstructure models and nonlocal crystal plasticity
Vajragupta, N. and Ahmed, S. and Boeff, M. and Ma, A. and Hartmaier, A.
PHYSICAL MESOMECHANICS. Volume: 20 (2017) - 2017 • 4604
On the influence of microcantilever pre-crack geometries on the apparent fracture toughness of brittle materials
Brinckmann, S. and Matoy, K. and Kirchlechner, C. and Dehm, G.
ACTA MATERIALIA. Volume: 136 (2017) - 2017 • 4603
Measurements of lithium-ion concentration equilibration processes inside graphite electrodes
Kindermann, F.M. and Osswald, P.J. and Klink, S. and Ehlert, G. and Schuster, J. and Noel, A. and Erhard, S.V. and Schuhmann, W. and Jossen, A.
JOURNAL OF POWER SOURCES. Volume: 342 (2017) - 2017 • 4602
Towards prediction of springback in deep drawing using a micromechanical modeling scheme
Vajragupta, N. and Ul Hassan, H. and Hartmaier, A.
PROCEDIA ENGINEERING. Volume: 207 (2017) - 2017 • 4601
On the numerical evaluation of local curvature for diffuse interface models of microstructure evolution
Vakili, S. and Steinbach, I. and Varnik, F.
PROCEDIA COMPUTER SCIENCE. Volume: 108 (2017) - 2017 • 4600
Comparison of technologies for nano device prototyping with a special focus on ion beams: A review
Bruchhaus, L. and Mazarov, P. and Bischoff, L. and Gierak, J. and Wieck, A.D. and Hövel, H.
APPLIED PHYSICS REVIEWS. Volume: 4 (2017) - 2017 • 4599
Transport mechanisms through PE-CVD coatings: Influence of temperature, coating properties and defects on permeation of water vapour
Kirchheim, D. and Jaritz, M. and Mitschker, F. and Gebhard, M. and Brochhagen, M. and Hopmann, C. and Böke, M. and Devi, A. and Awakowicz, P. and Dahlmann, R.
JOURNAL OF PHYSICS D: APPLIED PHYSICS. Volume: 50 (2017) - 2017 • 4598
Numerical modelling of the czochralski growth of β-Ga2O3
Miller, W. and Böttcher, K. and Galazka, Z. and Schreuer, J.
CRYSTALS. Volume: 7 (2017) - 2017 • 4597
Temperature-dependent transport mechanisms through PE-CVD coatings: Comparison of oxygen and water vapour
Kirchheim, D. and Wilski, S. and Jaritz, M. and Mitschker, F. and Gebhard, M. and Brochhagen, M. and Böe, M. and Benedikt, J. and Awakowicz, P. and Devi, A. and Hopmann, C. and Dahlmann, R.
JOURNAL OF PHYSICS D: APPLIED PHYSICS. Volume: 50 (2017) - 2017 • 4596
Pre- and post-buckling behavior of bi-crystalline micropillars: Origin and consequences
Kirchlechner, C. and Toth, F. and Rammerstorfer, F.G. and Fischer, F.D. and Dehm, G.
ACTA MATERIALIA. Volume: 124 (2017) - 2017 • 4595
Size-dependent reactivity of gold-copper bimetallic nanoparticles during CO2 electroreduction
Mistry, H. and Reske, R. and Strasser, P. and Roldan Cuenya, B.
CATALYSIS TODAY. Volume: 288 (2017) - 2017 • 4594
High-frequency polarization dynamics in spin-lasers: Pushing the limits
Gerhardt, N.C. and Lindemann, M. and Pusch, T. and Michalzik, R. and Hofmann, M.R.
PROCEEDINGS OF SPIE - THE INTERNATIONAL SOCIETY FOR OPTICAL ENGINEERING. Volume: 10357 (2017) - 2017 • 4593
Indistinguishable and efficient single photons from a quantum dot in a planar nanobeam waveguide
Kiršanske, G. and Thyrrestrup, H. and Daveau, R.S. and Dreeßen, C.L. and Pregnolato, T. and Midolo, L. and Tighineanu, P. and Javadi, A. and Stobbe, Sø. and Schott, R. and Ludwig, Ar. and Wieck, A.D. and Park, S.I. and Song, J.D. and Kuhlmann, A.V. and Söllner, I. and Löbl, M.C. and Warburton, R.J. and Lodahl, P.
PHYSICAL REVIEW B. Volume: 96 (2017) - 2017 • 4592
Coarsening of Y-rich oxide particles in 9%Cr-ODS Eurofer steel annealed at 1350 °C
Sandim, M.J.R. and Souza Filho, I.R. and Bredda, E.H. and Kostka, A. and Raabe, D. and Sandim, H.R.Z.
JOURNAL OF NUCLEAR MATERIALS. Volume: 484 (2017) - 2017 • 4591
Surface optical phonon propagation in defect modulated nanowires
Venkatesan, S. and Mancabelli, T. and Krogstrup, P. and Hartschuh, A. and Dehm, G. and Scheu, C.
JOURNAL OF APPLIED PHYSICS. Volume: 121 (2017) - 2017 • 4590
Nonparametric tests for detecting breaks in the jump behaviour of a time-continuous process
Bucher, A. and Hoffmann, M. and Vetter, M. and Dette, H.
BERNOULLI. Volume: 23 (2017) - 2017 • 4589
A rare-earth free magnesium alloy with improved intrinsic ductility
Sandlöbes, S. and Friák, M. and Korte-Kerzel, S. and Pei, Z. and Neugebauer, J. and Raabe, D.
SCIENTIFIC REPORTS. Volume: 7 (2017) - 2017 • 4588
Solid electrolyte interphase (SEI) at TiO2 electrodes in li-ion batteries: Defining apparent and effective SEI based on evidence from X-ay photoemission spectroscopy and scanning electrochemical microscopy
Ventosa, E. and Madej, E. and Zampardi, G. and Mei, B. and Weide, P. and Antoni, H. and La Mantia, F. and Muhler, M. and Schuhmann, W.
ACS APPLIED MATERIALS AND INTERFACES. Volume: 9 (2017) - 2017 • 4587
Influence of PE-CVD and PE-ALD on defect formation in permeation barrier films on PET and correlation to atomic oxygen fluence
Mitschker, F. and Steves, S. and Gebhard, M. and Rudolph, M. and Schücke, L. and Kirchheim, D. and Jaritz, M. and Brochhagen, M. and Hoppe, C. and Dahlmann, R. and Böke, M. and Benedikt, J. and Giner, I. and De los Arcos, T. and Hopmann, C. and Grundmeier, G. and Devi, A. and Awakowicz, P.
JOURNAL OF PHYSICS D: APPLIED PHYSICS. Volume: 50 (2017) - 2017 • 4586
Effective One-Dimensional Coupling in the Highly Frustrated Square-Lattice Itinerant Magnet CaCo2-yAs2
Sapkota, A. and Ueland, B.G. and Anand, V.K. and Sangeetha, N.S. and Abernathy, D.L. and Stone, M.B. and Niedziela, J.L. and Johnston, D.C. and Kreyssig, A. and Goldman, A.I. and McQueeney, R.J.
PHYSICAL REVIEW LETTERS. Volume: 119 (2017) - 2017 • 4585
Development of bond-order potentials for BCC transition metals
Vitek, V. and Lin, Y.-S. and Mrovec, M.
SOLID STATE PHENOMENA. Volume: 258 SSP (2017) - 2017 • 4584
Electrical, optical and spectroscopic characterisation of a radio frequency discharge used for electrosurgical cutting
Bürger, I. and Bibinov, N. and Neugebauer, A. and Enderle, M. and Awakowicz, P.
PLASMA PROCESSES AND POLYMERS. Volume: 14 (2017) - 2017 • 4583
Diffusion processes during cementite precipitation and their impact on electrical and thermal conductivity of a heat-treatable steel
Klein, S. and Mujica Roncery, L. and Walter, M. and Weber, S. and Theisen, W.
JOURNAL OF MATERIALS SCIENCE. Volume: 52 (2017) - 2017 • 4582
Tissue differentiation by means of high resolution optical emission spectroscopy during electrosurgical intervention
Bürger, I. and Scharpf, M. and Hennenlotter, J. and Nüßle, D. and Spether, D. and Neugebauer, A. and Bibinov, N. and Stenzl, A. and Fend, F. and Enderle, M. and Awakowicz, P.
JOURNAL OF PHYSICS D: APPLIED PHYSICS. Volume: 50 (2017) - 2017 • 4581
Study of stability of microstructure and residual strain after thermal loading of plasma sprayed YSZ by through surface neutron scanning
Gibmeier, J. and Back, H.C. and Mutter, M. and Vollert, F. and Rebelo-Kornmeier, J. and Mücke, R. and Vaßen, R.
PHYSICA B: CONDENSED MATTER. Volume: (2017) - 2017 • 4580
Time-resolved impact electrochemistry for quantitative measurement of single-nanoparticle reaction kinetics
Saw, E.N. and Kratz, M. and Tschulik, K.
NANO RESEARCH. Volume: (2017) - 2017 • 4579
Hybrid N-Butylamine-Based Ligands for Switching the Colloidal Solubility and Regimentation of Inorganic-Capped Nanocrystals
Sayevich, V. and Guhrenz, C. and Dzhagan, V.M. and Sin, M. and Werheid, M. and Cai, B. and Borchardt, L. and Widmer, J. and Zahn, D.R.T. and Brunner, E. and Lesnyak, V. and Gaponik, N. and Eychmüller, A.
ACS NANO. Volume: 11 (2017) - 2017 • 4578
Tissue differentiation by means of high resolution optical emission spectroscopy during electrosurgical intervention
Burger, I. and Scharpf, M. and Hennenlotter, J. and Nussle, D. and Spether, D. and Neugebauer, A. and Bibinov, N. and Stenzl, A. and Fend, F. and Enderle, M. and Awakowicz, P.
JOURNAL OF PHYSICS D-APPLIED PHYSICS. Volume: 50 (2017) - 2017 • 4577
A kinetic Monte Carlo approach to diffusion-controlled thermal desorption spectroscopy
Schablitzki, T. and Rogal, J. and Drautz, R.
PHILOSOPHICAL TRANSACTIONS OF THE ROYAL SOCIETY A: MATHEMATICAL, PHYSICAL AND ENGINEERING SCIENCES. Volume: 375 (2017) - 2017 • 4576
Special Issue: Recent developments in thermo-mechanical fatigue
Klingelhöffer, H. and Affeldt, E. and Bache, M. and Bartsch, M. and Beck, T. and Christ, H.J. and Fedelich, B. and Hähner, P. and Holdsworth, S.R. and Lang, K.-H. and McGaw, M. and Olbricht, J. and Remy, L. and Skrotzki, B. and Stekovich, S.
INTERNATIONAL JOURNAL OF FATIGUE. Volume: 99 (2017) - 2017 • 4575
Modulation of the CO2 fixation in dinickel azacryptands
Möller, F. and Castañeda-Losada, L. and Junqueira, J.R.C. and Miller, R.G. and Reback, M.L. and Mallick, B. and Van Gastel, M. and Apfel, U.-P.
DALTON TRANSACTIONS. Volume: 46 (2017) - 2017 • 4574
Prussian Blue Analogues: A Versatile Framework for Solid-Contact Ion-Selective Electrodes with Tunable Potentials
Klink, S. and Ishige, Y. and Schuhmann, W.
CHEMELECTROCHEM. Volume: 4 (2017) - 2017 • 4573
Trimesic acid on Cu in ethanol: Potential-dependent transition from 2-D adsorbate to 3-D metal-organic framework
Schäfer, P. and Lalitha, A. and Sebastian, P. and Meena, S.K. and Feliu, J. and Sulpizi, M. and van der Veen, M.A. and Domke, K.F.
JOURNAL OF ELECTROANALYTICAL CHEMISTRY. Volume: 793 (2017) - 2017 • 4572
Wave dispersion and propagation in state-based peridynamics
Butt, S.N. and Timothy, J.J. and Meschke, G.
COMPUTATIONAL MECHANICS. Volume: 60 (2017) - 2017 • 4571
Combinatorial Alloy Design by Laser Additive Manufacturing
Knoll, H. and Ocylok, S. and Weisheit, A. and Springer, H. and Jägle, E. and Raabe, D.
STEEL RESEARCH INTERNATIONAL. Volume: 88 (2017) - 2017 • 4570
Nanostructure of and structural defects in a Mo2BC hard coating investigated by transmission electron microscopy and atom probe tomography
Gleich, S. and Fager, H. and Bolvardi, H. and Achenbach, J.-O. and Soler, R. and Pradeep, K.G. and Schneider, J.M. and Dehm, G. and Scheu, C.
JOURNAL OF APPLIED PHYSICS. Volume: 122 (2017) - 2017 • 4569
Hands-on Guide to the Synthesis of Mesoporous Hollow Graphitic Spheres and Core-Shell Materials
Knossalla, J. and Jalalpoor, D. and Schüth, F.
CHEMISTRY OF MATERIALS. Volume: 29 (2017) - 2017 • 4568
Atomic scale processes of phase transformations in nanocrystalline NiTi shape-memory alloys
Ko, W.-S. and Maisel, S.B. and Grabowski, B. and Jeon, J.B. and Neugebauer, J.
ACTA MATERIALIA. Volume: 123 (2017) - 2017 • 4567
Review Article: Unraveling synergistic effects in plasma-surface processes by means of beam experiments
Von Keudell, A. and Corbella, C.
JOURNAL OF VACUUM SCIENCE AND TECHNOLOGY A: VACUUM, SURFACES AND FILMS. Volume: 35 (2017) - 2017 • 4566
Introduction
Göken, M. and Eggeler, G. and Zhu, Y. and Lu, L.
JOURNAL OF MATERIALS RESEARCH. Volume: 32 (2017) - 2017 • 4565
Polymer conformations in ionic microgels in the presence of salt: Theoretical and mesoscale simulation results
Kobayashi, H. and Halver, R. and Sutmann, G. and Winkler, R.G.
POLYMERS. Volume: 9 (2017) - 2017 • 4564
Foundations of low-temperature plasma physics - An introduction
Von Keudell, A. and Schulz-Von Der Gathen, V.
PLASMA SOURCES SCIENCE AND TECHNOLOGY. Volume: 26 (2017) - 2017 • 4563
Lensless digital holographic microscope using in-line configuration and laser diode illumination
Göring, L. and Finkeldey, M. and Adinda-Ougba, A. and Gerhardt, N.C. and Hofmann, M.
PROCEEDINGS OF SPIE - THE INTERNATIONAL SOCIETY FOR OPTICAL ENGINEERING. Volume: 10127 (2017) - 2017 • 4562
Manufacturing of Composite Coatings by Atmospheric Plasma Spraying Using Different Feed-Stock Materials as YSZ and MoSi2
Koch, D. and Mauer, G. and Vaßen, R.
JOURNAL OF THERMAL SPRAY TECHNOLOGY. Volume: 26 (2017) - 2017 • 4561
Identification and separation of rectifier mechanisms in Si/SiGe ballistic cross junctions
Von Pock, J.F. and Salloch, D. and Wieser, U. and Hackbarth, T. and Kunze, U.
JOURNAL OF APPLIED PHYSICS. Volume: 121 (2017) - 2017 • 4560
Catalysis Meets Nonthermal Separation for the Production of (Alkyl)phenols and Hydrocarbons from Pyrolysis Oil
Cao, Z. and Engelhardt, J. and Dierks, M. and Clough, M.T. and Wang, G.-H. and Heracleous, E. and Lappas, A. and Rinaldi, R. and Schüth, F.
ANGEWANDTE CHEMIE - INTERNATIONAL EDITION. Volume: 56 (2017) - 2017 • 4559
Optical metrology for the investigation of buried technical structures
Göring, L. and Finkeldey, M. and Schellenberg, F. and Brenner, C. and Hofmann, M.R. and Gerhardt, N.
TECHNISCHES MESSEN. Volume: (2017) - 2017 • 4558
Development and characterization of a microsnap-fit for optical assembly
Köhler, J. and Kutlu, Y. and Ksouri, S.I. and Esen, C. and Ostendorf, A.
PROCEEDINGS OF SPIE - THE INTERNATIONAL SOCIETY FOR OPTICAL ENGINEERING. Volume: 10120 (2017) - 2017 • 4557
Input-Current Addition in Closely Positioned Dual-Stage Ballistic Rectifiers
Von Pock, J. and Wieser, U. and Kunze, U.
PHYSICAL REVIEW APPLIED. Volume: 7 (2017) - 2017 • 4556
Topological phase inversion after long-term thermal exposure of nickel-base superalloys: Experiment and phase-field simulation
Goerler, J.V. and Lopez-Galilea, I. and Mujica Roncery, L. and Shchyglo, O. and Theisen, W. and Steinbach, I.
ACTA MATERIALIA. Volume: 124 (2017) - 2017 • 4555
Optical assembly of microsnap-fits fabricated by two-photon polymerization
Köhler, J. and Kutlu, Y. and Zyla, G. and Ksouri, S.I. and Esen, C. and Gurevich, E.L. and Ostendorf, A.
OPTICAL ENGINEERING. Volume: 56 (2017) - 2017 • 4554
Promotional Effect of Fe Impurities in Graphene Precursors on the Activity of MnOX/Graphene Electrocatalysts for the Oxygen Evolution and Oxygen Reduction Reactions
Morales, D.M. and Masa, J. and Andronescu, C. and Schuhmann, W.
CHEMELECTROCHEM. Volume: 4 (2017) - 2017 • 4553
Upward mass transport and alloying during the growth of Co on Cu(111)
Vu, Q.H. and Morgenstern, K.
PHYSICAL REVIEW B - CONDENSED MATTER AND MATERIALS PHYSICS. Volume: 95 (2017) - 2017 • 4552
Effect of Pt substitution on the magnetocrystalline anisotropy of Ni2MnGa: A competition between chemistry and elasticity
Caron, L. and Dutta, B. and Devi, P. and Ghorbani Zavareh, M. and Hickel, T. and Cabassi, R. and Bolzoni, F. and Fabbrici, S. and Albertini, F. and Felser, C. and Singh, S.
PHYSICAL REVIEW B. Volume: 96 (2017) - 2017 • 4551
Antibacterial activity of microstructured sacrificial anode thin films by combination of silver with platinum group elements (platinum, palladium, iridium)
Köller, M. and Bellova, P. and Javid, S.M. and Motemani, Y. and Khare, C. and Sengstock, C. and Tschulik, K. and Schildhauer, T.A. and Ludwig, Al.
MATERIALS SCIENCE AND ENGINEERING C. Volume: 74 (2017) - 2017 • 4550
Hydratisierung von und auf Oberflächen: Eis unter dem Rastertunnelmikroskop
Morgenstern, K.
CHEMIE IN UNSERER ZEIT. Volume: 51 (2017) - 2017 • 4549
Verlet-like algorithms for Car-Parrinello molecular dynamics with unequal electronic occupations
Castañeda Medina, A. and Schmid, R.
JOURNAL OF CHEMICAL PHYSICS. Volume: 147 (2017) - 2017 • 4548
Hydration from and on surfaces: Ice under the scanning tunneling microscope
Morgenstern, K.
CHEMIE IN UNSERER ZEIT. Volume: 51 (2017) - 2017 • 4547
Mechanisms of severe sliding abrasion of single phase steels at elevated temperatures: Influence of lattice structure and microstructural parameters
Walter, M. and Weber, S. and Boes, J. and Egels, G. and Theisen, W.
WEAR. Volume: 376-377 (2017) - 2017 • 4546
Simulation of Reacting Moving Granular Material in Furnaces and Boilers An Overview on the Capabilities of the Discrete Element Method
Scherer, V. and Wirtz, S. and Krause, B. and Wissing, F.
ENERGY PROCEDIA. Volume: 120 (2017) - 2017 • 4545
Channel flow and localized fault bounded slice tectonics (LFBST): Insights from petrological, structural, geochronological and geospeedometric studies in the Sikkim Himalaya, NE India
Chakraborty, S. and Mukhopadhyay, D.K. and Chowdhury, P. and Rubatto, D. and Anczkiewicz, R. and Trepmann, C. and Gaidies, F. and Sorcar, N. and Dasgupta, S.
LITHOS. Volume: 282-283 (2017) - 2017 • 4544
Solar biosupercapacitor
González-Arribas, E. and Aleksejeva, O. and Bobrowski, T. and Toscano, M.D. and Gorton, L. and Schuhmann, W. and Shleev, S.
ELECTROCHEMISTRY COMMUNICATIONS. Volume: 74 (2017) - 2017 • 4543
Transparent, mediator- and membrane-free enzymatic fuel cell based on nanostructured chemically modified indium tin oxide electrodes
González-Arribas, E. and Bobrowski, T. and Di Bari, C. and Sliozberg, K. and Ludwig, R. and Toscano, M.D. and De Lacey, A.L. and Pita, M. and Schuhmann, W. and Shleev, S.
BIOSENSORS AND BIOELECTRONICS. Volume: 97 (2017) - 2017 • 4542
Optical screw-wrench for microassembly
Kohler, J. and Ksouri, S. I. and Esen, C. and Ostendorf, A.
MICROSYSTEMS & NANOENGINEERING. Volume: 3 (2017) - 2017 • 4541
Multi-phase-field model for surface and phase-boundary diffusion
Schiedung, R. and Kamachali, R.D. and Steinbach, I. and Varnik, F.
PHYSICAL REVIEW E. Volume: 96 (2017) - 2017 • 4540
Normal Radiative Emittance of Coal Ash Sulfates in the Context of Oxyfuel Combustion
Gorewoda, J. and Scherer, V.
ENERGY AND FUELS. Volume: 31 (2017) - 2017 • 4539
Confidence Corridors for Multivariate Generalized Quantile Regression
Chao, S.-K. and Proksch, K. and Dette, H. and Härdle, W.K.
JOURNAL OF BUSINESS AND ECONOMIC STATISTICS. Volume: 35 (2017) - 2017 • 4538
Key parameters for the radiative emittance of ashes of solid fuels
Gorewoda, J. and Scherer, V.
ENERGY PROCEDIA. Volume: 120 (2017) - 2017 • 4537
Influence of rhenium on γ′-strengthened cobalt-base superalloys
Kolb, M. and Zenk, C.H. and Kirzinger, A. and Povstugar, I. and Raabe, D. and Neumeier, S. and Göken, M.
JOURNAL OF MATERIALS RESEARCH. Volume: 32 (2017) - 2017 • 4536
Self-Diffusion of Surface Defects at Copper-Water Interfaces
Kondati Natarajan, S. and Behler, J.
JOURNAL OF PHYSICAL CHEMISTRY C. Volume: 121 (2017) - 2017 • 4535
On the bifunctional nature of Cu/ZrO2 catalysts applied in the hydrogenation of ethyl acetate
Schittkowski, J. and Tölle, K. and Anke, S. and Stürmer, S. and Muhler, M.
JOURNAL OF CATALYSIS. Volume: 352 (2017) - 2017 • 4534
Tracing the coupled atomic shear and shuffle for a cubic to a hexagonal crystal transition
Wang, H.L. and Hao, Y.L. and He, S.Y. and Du, K. and Li, T. and Obbard, E.G. and Hudspeth, J. and Wang, J.G. and Wang, Y.D. and Wang, Y. and Prima, F. and Lu, N. and Kim, M.J. and Cairney, J.M. and Li, S.J. and Yang, R.
SCRIPTA MATERIALIA. Volume: 133 (2017) - 2017 • 4533
Mechanochemical Suzuki polycondensation-from linear to hyperbranched polyphenylenes
Grätz, S. and Wolfrum, B. and Borchardt, L.
GREEN CHEMISTRY. Volume: 19 (2017) - 2017 • 4532
Elastically confined martensitic transformation at the nano-scale in a multifunctional titanium alloy
Wang, H.L. and Hao, Y.L. and He, S.Y. and Li, T. and Cairney, J.M. and Wang, Y.D. and Wang, Y. and Obbard, E.G. and Prima, F. and Du, K. and Li, S.J. and Yang, R.
ACTA MATERIALIA. Volume: 135 (2017) - 2017 • 4531
Avoiding Self-Poisoning: A Key Feature for the High Activity of Au/Mg(OH)2 Catalysts in Continuous Low-Temperature CO Oxidation
Wang, Y. and Widmann, D. and Lehnert, F. and Gu, D. and Schüth, F. and Behm, R.J.
ANGEWANDTE CHEMIE - INTERNATIONAL EDITION. Volume: 56 (2017) - 2017 • 4530
Room temperature deformation of LPSO structures by non-basal slip
Chen, R. and Sandlöbes, S. and Zeng, X. and Li, D. and Korte-Kerzel, S. and Raabe, D.
MATERIALS SCIENCE AND ENGINEERING A. Volume: 682 (2017) - 2017 • 4529
Optimized expression-based microdissection of formalin-fixed lung cancer tissue
Grafen, M. and Hofmann, T.R. and Scheel, A.H. and Beck, J. and Emmert, A. and Küffer, S. and Danner, B.C. and Schütz, E. and Büttner, R. and Ostendorf, A. and Ströbel, P. and Bohnenberger, H.
LABORATORY INVESTIGATION. Volume: 97 (2017) - 2017 • 4528
Metallic NiPS3@NiOOH Core-Shell Heterostructures as Highly Efficient and Stable Electrocatalyst for the Oxygen Evolution Reaction
Konkena, B. and Masa, J. and Botz, A. J. R. and Sinev, I. and Xia, W. and Kossmann, J. and Drautz, R. and Muhler, M. and Schuhmann, W.
ACS CATALYSIS. Volume: 7 (2017) - 2017 • 4527
High activity and negative apparent activation energy in low-temperature CO oxidation - Present on Au/Mg(OH)2, absent on Au/TiO2
Wang, Y. and Widmann, D. and Wittmann, M. and Lehnert, F. and Gu, D. and Schüth, F. and Behm, R.J.
CATALYSIS SCIENCE AND TECHNOLOGY. Volume: 7 (2017) - 2017 • 4526
Bayesian D-optimal designs for error-in-variables models
Konstantinou, M. and Dette, H.
APPLIED STOCHASTIC MODELS IN BUSINESS AND INDUSTRY. Volume: 33 (2017) - 2017 • 4525
An Electric Field Induced Breath for Metal-Organic Frameworks
Schmid, R.
ACS CENTRAL SCIENCE. Volume: 3 (2017) - 2017 • 4524
Experimental and Theoretical Understanding of Nitrogen-Doping-Induced Strong Metal-Support Interactions in Pd/TiO2 Catalysts for Nitrobenzene Hydrogenation
Chen, P. and Khetan, A. and Yang, F. and Migunov, V. and Weide, P. and Stürmer, S.P. and Guo, P. and Kähler, K. and Xia, W. and Mayer, J. and Pitsch, H. and Simon, U. and Muhler, M.
ACS CATALYSIS. Volume: 7 (2017) - 2017 • 4523
Dauerhafte Betonfahrbahndecken durch Verwendung unterschiedlicher Bindemittel in Ober- und Unterbeton
Müller, M. and Ludwig, H.-M. and Schulte-Schrepping, C. and Breitenbücher, R.
BETON- UND STAHLBETONBAU. Volume: 112 (2017) - 2017 • 4522
Durable concrete pavements using different binders in top and bottom lift
Müller, M. and Ludwig, H.-M. and Schulte-Schrepping, C. and Breitenbücher, R.
BETON- UND STAHLBETONBAU. Volume: 112 (2017) - 2017 • 4521
Linking transducer transfer function with multi-pulse excitation photoacoustic response
Cherkashin, M. and Brenner, C. and Putro, W.D. and Döpke, B. and Gerhardt, N.C. and Hofmann, M.R.
PROGRESS IN BIOMEDICAL OPTICS AND IMAGING - PROCEEDINGS OF SPIE. Volume: 10139 (2017) - 2017 • 4520
Cd and Impurity Redistribution at the CdS/CIGS Interface after Annealing of CIGS-Based Solar Cells Resolved by Atom Probe Tomography
Koprek, A. and Cojocaru-Miredin, O. and Wuerz, R. and Freysoldt, C. and Gault, B. and Raabe, D.
IEEE JOURNAL OF PHOTOVOLTAICS. Volume: 7 (2017) - 2017 • 4519
Microresonator array: A particular optical sensor
Weigel, T. and Schweiger, G. and Esen, C. and Ostendorf, A.
TECHNISCHES MESSEN. Volume: 84 (2017) - 2017 • 4518
Dynamics of the photoacoustic response of single-element PZT transducers to pulse burst excitation
Cherkashin, M. and Brenner, C. and Putro, W.D. and Döpke, B. and Gerhardt, N.C. and Hofmann, M.R.
PROGRESS IN BIOMEDICAL OPTICS AND IMAGING - PROCEEDINGS OF SPIE. Volume: 10064 (2017) - 2017 • 4517
Ultra-stiff metallic glasses through bond energy density design
Schnabel, V. and Köhler, M. and Music, D. and Bednarcik, J. and Clegg, W.J. and Raabe, D. and Schneider, J.M.
JOURNAL OF PHYSICS CONDENSED MATTER. Volume: 29 (2017) - 2017 • 4516
A monolithic micro-optical interferometer deep etched into fused silica
Weigel, C. and Markweg, E. and Müller, L. and Schulze, M. and Gargouri, H. and Hoffmann, M.
MICROELECTRONIC ENGINEERING. Volume: 174 (2017) - 2017 • 4515
Electronic and molecular structure relations in diiron compounds mimicking the [FeFe]-hydrogenase active site studied by X-ray spectroscopy and quantum chemistry
Kositzki, R. and Mebs, S. and Schüth, N. and Leidel, N. and Schwartz, L. and Karnahl, M. and Wittkamp, F. and Daunke, D. and Grohmann, A. and Apfel, U.-P. and Gloaguen, F. and Ott, S. and Haumann, M.
DALTON TRANSACTIONS. Volume: 46 (2017) - 2017 • 4514
Quantification of the influence of parameters determining radiative heat transfer in an oxy-fuel operated boiler
Gronarz, T. and Schulze, J. and Laemmerhold, M. and Graeser, P. and Gorewoda, J. and Kez, V. and Habermehl, M. and Schiemann, M. and Ströhle, J. and Epple, B. and Scherer, V. and Kneer, R.
FUEL PROCESSING TECHNOLOGY. Volume: 157 (2017) - 2017 • 4513
German Catalysis Society (GeCatS)
Muhler, M.
CHEMCATCHEM. Volume: 9 (2017) - 2017 • 4512
Magnetic properties of the CrMnFeCoNi high-entropy alloy
Schneeweiss, O. and Friák, M. and Dudová, M. and Holec, D. and Šob, M. and Kriegner, D. and Holý, V. and Beran, P. and George, E.P. and Neugebauer, J. and Dlouhý, A.
PHYSICAL REVIEW B. Volume: 96 (2017) - 2017 • 4511
Optical anisotropy in the electronic nematic phase of FeSe
Chinotti, M. and Pal, A. and Degiorgi, L. and Böhmer, A.E. and Canfield, P.C.
PHYSICAL REVIEW B. Volume: 96 (2017) - 2017 • 4510
Solvent-Free Mechanochemical Synthesis of Nitrogen-Doped Nanoporous Carbon for Electrochemical Energy Storage
Schneidermann, C. and Jäckel, N. and Oswald, S. and Giebeler, L. and Presser, V. and Borchardt, L.
CHEMSUSCHEM. Volume: 10 (2017) - 2017 • 4509
Dislocation interaction and twinning-induced plasticity in face-centered cubic Fe-Mn-C micro-pillars
Choi, W.S. and Sandlöbes, S. and Malyar, N.V. and Kirchlechner, C. and Korte-Kerzel, S. and Dehm, G. and De Cooman, B.C. and Raabe, D.
ACTA MATERIALIA. Volume: 132 (2017) - 2017 • 4508
The nature and evolution of the Main Central Thrust: Structural and geochronological constraints from the Sikkim Himalaya, NE India
Mukhopadhyay, D.K. and Chakraborty, S. and Trepmann, C. and Rubatto, D. and Anczkiewicz, R. and Gaidies, F. and Dasgupta, S. and Chowdhury, P.
LITHOS. Volume: 282-283 (2017) - 2017 • 4507
Carbon onion-sulfur hybrid cathodes for lithium-sulfur batteries
Choudhury, S. and Zeiger, M. and Massuti-Ballester, P. and Fleischmann, S. and Formanek, P. and Borchardt, L. and Presser, V.
SUSTAINABLE ENERGY AND FUELS. Volume: 1 (2017) - 2017 • 4506
Microporous novolac-derived carbon beads/sulfur hybrid cathode for lithium-sulfur batteries
Choudhury, S. and Krüner, B. and Massuti-Ballester, P. and Tolosa, A. and Prehal, C. and Grobelsek, I. and Paris, O. and Borchardt, L. and Presser, V.
JOURNAL OF POWER SOURCES. Volume: 357 (2017) - 2017 • 4505
Bone-like crack resistance in hierarchical metastable nanolaminate steels
Koyama, M. and Zhang, Z. and Wang, M. and Ponge, D. and Raabe, D. and Tsuzaki, K. and Noguchi, H. and Tasan, C.C.
SCIENCE. Volume: 355 (2017) - 2017 • 4504
Recent progress in microstructural hydrogen mapping in steels: quantification, kinetic analysis, and multi-scale characterisation
Koyama, M. and Rohwerder, M. and Tasan, C.C. and Bashir, A. and Akiyama, E. and Takai, K. and Raabe, D. and Tsuzaki, K.
MATERIALS SCIENCE AND TECHNOLOGY (UNITED KINGDOM). Volume: (2017) - 2017 • 4503
Correlative plasma-surface model for metastable Cr-Al-N: Frenkel pair formation and influence of the stress state on the elastic properties
Music, D. and Banko, L. and Ruess, H. and Engels, M. and Hecimovic, A. and Grochla, D. and Rogalla, D. and Brögelmann, T. and Ludwig, Al. and Von Keudell, A. and Bobzin, K. and Schneider, J.M.
JOURNAL OF APPLIED PHYSICS. Volume: 121 (2017) - 2017 • 4502
Focused ion beam supported growth of monocrystalline wurtzite InAs nanowires grown by molecular beam epitaxy
Scholz, S. and Schott, R. and Labud, P.A. and Somsen, C. and Reuter, D. and Ludwig, Ar. and Wieck, A.D.
JOURNAL OF CRYSTAL GROWTH. Volume: 470 (2017) - 2017 • 4501
Nondestructive evaluation of protective coatings for the conservation of industrial monuments
Welp, H. and Lenz, M. and Mazzon, C. and Dillmann, C. and Gerhardt, N.C. and Prange, M. and Hofmann, M.R.
PROCEEDINGS OF SPIE - THE INTERNATIONAL SOCIETY FOR OPTICAL ENGINEERING. Volume: 10331 (2017) - 2017 • 4500
Overview of hydrogen embrittlement in high-Mn steels
Koyama, M. and Akiyama, E. and Lee, Y.-K. and Raabe, D. and Tsuzaki, K.
INTERNATIONAL JOURNAL OF HYDROGEN ENERGY. Volume: (2017) - 2017 • 4499
Correlation of splat morphologies with porosity and residual stress in plasma-sprayed YSZ coatings
Mutter, M. and Mauer, G. and Mücke, R. and Guillon, O. and Vaßen, R.
SURFACE AND COATINGS TECHNOLOGY. Volume: 318 (2017) - 2017 • 4498
Optimal designs for active controlled dose-finding trials with efficacy-toxicity outcomes
Schorning, K. and Dette, H. and Kettelhake, K. and Wong, W.K. and Bretz, F.
BIOMETRIKA. Volume: 104 (2017) - 2017 • 4497
Self-healing atmospheric plasma sprayed Mn1.0Co1.9Fe0.1O4 protective interconnector coatings for solid oxide fuel cells
Grünwald, N. and Sebold, D. and Sohn, Y.J. and Menzler, N.H. and Vaßen, R.
JOURNAL OF POWER SOURCES. Volume: 363 (2017) - 2017 • 4496
Surface-Casting Synthesis of Mesoporous Zirconia with a CMK-5-Like Structure and High Surface Area
Gu, D. and Schmidt, W. and Pichler, C.M. and Bongard, H.-J. and Spliethoff, B. and Asahina, S. and Cao, Z. and Terasaki, O. and Schüth, F.
ANGEWANDTE CHEMIE - INTERNATIONAL EDITION. Volume: (2017) - 2017 • 4495
3D-DEM-CFD simulation of heat and mass transfer, gas combustion and calcination in an intermittent operating lime shaft kiln
Krause, B. and Liedmann, B. and Wiese, J. and Bucher, P. and Wirtz, S. and Piringer, H. and Scherer, V.
INTERNATIONAL JOURNAL OF THERMAL SCIENCES. Volume: 117 (2017) - 2017 • 4494
Thermo-mechanical stresses within switching contact systems after arcing events
Mutzel, T. and Ma, D. and Roters, F. and Raabe, D.
ELECTRICAL CONTACTS, PROCEEDINGS OF THE ANNUAL HOLM CONFERENCE ON ELECTRICAL CONTACTS. Volume: (2017) - 2017 • 4493
Oscillatory combustion of propene during in situ mechanical activation of solid catalysts
Schreyer, H. and Immohr, S. and Schüth, F.
JOURNAL OF MATERIALS SCIENCE. Volume: 52 (2017) - 2017 • 4492
Thermal bed mixing in rotary drums for different operational parameters
Nafsun, A.I. and Herz, F. and Specht, E. and Komossa, H. and Wirtz, S. and Scherer, V. and Liu, X.
CHEMICAL ENGINEERING SCIENCE. Volume: 160 (2017) - 2017 • 4491
Interfacial hydrogen localization in austenite/martensite dual-phase steel visualized through optimized silver decoration and scanning Kelvin probe force microscopy
Nagashima, T. and Koyama, M. and Bashir, A. and Rohwerder, M. and Tasan, C.C. and Akiyama, E. and Raabe, D. and Tsuzaki, K.
MATERIALS AND CORROSION. Volume: 68 (2017) - 2017 • 4490
Low-temperature features in the heat capacity of unary metals and intermetallics for the example of bulk aluminum and Al3 Sc
Gupta, A. and Kavakbasi, B.T. and Dutta, B. and Grabowski, B. and Peterlechner, M. and Hickel, T. and Divinski, S.V. and Wilde, G. and Neugebauer, J.
PHYSICAL REVIEW B - CONDENSED MATTER AND MATERIALS PHYSICS. Volume: 95 (2017) - 2017 • 4489
Robust Single-Shot Spin Measurement with 99.5% Fidelity in a Quantum Dot Array
Nakajima, T. and Delbecq, M.R. and Otsuka, T. and Stano, P. and Amaha, S. and Yoneda, J. and Noiri, A. and Kawasaki, K. and Takeda, K. and Allison, G. and Ludwig, Ar. and Wieck, A.D. and Loss, D. and Tarucha, S.
PHYSICAL REVIEW LETTERS. Volume: 119 (2017) - 2017 • 4488
Identification of a ternary μ-phase in the Co-Ti-W system – An advanced correlative thin-film and bulk combinatorial materials investigation
Naujoks, D. and Eggeler, Y.M. and Hallensleben, P. and Frenzel, J. and Fries, S.G. and Palumbo, M. and Koßmann, J. and Hammerschmidt, T. and Pfetzing-Micklich, J. and Eggeler, G. and Spiecker, E. and Drautz, R. and Ludwig, Al.
ACTA MATERIALIA. Volume: 138 (2017) - 2017 • 4487
NMR evidence for static local nematicity and its cooperative interplay with low-energy magnetic fluctuations in FeSe under pressure
Wiecki, P. and Nandi, M. and Böhmer, A.E. and Bud'Ko, S.L. and Canfield, P.C. and Furukawa, Y.
PHYSICAL REVIEW B. Volume: 96 (2017) - 2017 • 4486
Selective glycerol oxidation over ordered mesoporous copper aluminum oxide catalysts
Schünemann, S. and Schüth, F. and Tüysüz, H.
CATALYSIS SCIENCE AND TECHNOLOGY. Volume: 7 (2017) - 2017 • 4485
Optimizing Ni–Ti-based shape memory alloys for ferroic cooling
Wieczorek, A. and Frenzel, J. and Schmidt, M. and Maaß, B. and Seelecke, S. and Schütze, A. and Eggeler, G.
FUNCTIONAL MATERIALS LETTERS. Volume: 10 (2017) - 2017 • 4484
Unraveling compositional effects on the light-induced oxygen evolution in Bi(V-Mo-X)O4 material libraries
Gutkowski, R. and Khare, C. and Conzuelo, F. and Kayran, Y.U. and Ludwig, Al. and Schuhmann, W.
ENERGY AND ENVIRONMENTAL SCIENCE. Volume: 10 (2017) - 2017 • 4483
Terahertz frequency generation with monolithically integrated dual wavelength distributed Bragg reflector semiconductor laser diode
Gwaro, J. O. and Brenner, C. and Sumpf, B. and Klehr, A. and Fricke, J. and Hofmann, M. R.
IET OPTOELECTRONICS. Volume: 11 (2017) - 2017 • 4482
Role of Nanostructuring and Microstructuring in Silver Antimony Telluride Compounds for Thermoelectric Applications
Cojocaru-Mirédin, O. and Abdellaoui, L. and Nagli, M. and Zhang, S. and Yu, Y. and Scheu, C. and Raabe, D. and Wuttig, M. and Amouyal, Y.
ACS APPLIED MATERIALS AND INTERFACES. Volume: 9 (2017) - 2017 • 4481
Plasma diagnostics in dielectric deposition processes
Schulz, C. and Rolfes, I.
PROCEEDINGS OF IEEE SENSORS. Volume: (2017) - 2017 • 4480
Analysis of dispersive interactions at polymer/TiAlN interfaces by means of dynamic force spectroscopy
Wiesing, M. and De Los Arcos, T. and Gebhard, M. and Devi, A. and Grundmeier, G.
PHYSICAL CHEMISTRY CHEMICAL PHYSICS. Volume: 20 (2017) - 2017 • 4479
High-resolution analysis of photoanodes for water splitting by means of scanning photoelectrochemical microscopy
Conzuelo, F. and Sliozberg, K. and Gutkowski, R. and Grutzke, S. and Nebe, M. and Schuhmann, W.
ANALYTICAL CHEMISTRY. Volume: 89 (2017) - 2017 • 4478
A variational approach to the modelling of grooving in a three-dimensional setting
Hackl, K. and Fischer, F.D. and Svoboda, J.
ACTA MATERIALIA. Volume: 129 (2017) - 2017 • 4477
Collision frequency determination of low-pressure plasmas based on RF-reflectometry
Schulz, C. and Rolfes, I. and Oberberg, M. and Awakowicz, P.
EUROPEAN MICROWAVE WEEK 2016: "MICROWAVES EVERYWHERE", EUMW 2016 - CONFERENCE PROCEEDINGS; 46TH EUROPEAN MICROWAVE CONFERENCE, EUMC 2016. Volume: (2017) - 2017 • 4476
Electric potential screening on metal targets submitted to reactive sputtering
Corbella, C. and Marcak, A. and Von Keudell, A. and De Los Arcos, T.
JOURNAL OF VACUUM SCIENCE AND TECHNOLOGY A: VACUUM, SURFACES AND FILMS. Volume: 35 (2017) - 2017 • 4475
Effect of titania surface modification of mesoporous silica SBA-15 supported Au catalysts: Activity and stability in the CO oxidation reaction
Kučerová, G. and Strunk, J. and Muhler, M. and Behm, R.J.
JOURNAL OF CATALYSIS. Volume: 356 (2017) - 2017 • 4474
Precipitation of T1 and θ' phase in Al-4Cu-1Li-0.25Mn during age hardening: Microstructural investigation and phase-field simulation
Häusler, I. and Schwarze, C. and Bilal, M.U. and Ramirez, D.V. and Hetaba, W.d and Kamachali, R.D. and Skrotzki, B.
MATERIALS. Volume: 10 (2017) - 2017 • 4473
Influence of temperature and tempering condition on the thermal conductivity of hot work tool steels for hot stamping applications
Hafenstein, S. and Werner, E. and Wilzer, J. and Theisen, W. and Weber, S. and Sunderkötter, C. and Bachmann, M.
HTM - JOURNAL OF HEAT TREATMENT AND MATERIALS. Volume: 72 (2017) - 2017 • 4472
Perovskites as Precursors for Ni/La2O3 Catalysts in the Dry Reforming of Methane: Synthesis by Constant pH Co-Precipitation, Reduction Mechanism and Effect of Ru-Doping
Kühl, S. and Düdder, H. and Girgsdies, F. and Kähler, K. and Muhler, M. and Behrens, M.
ZEITSCHRIFT FUR ANORGANISCHE UND ALLGEMEINE CHEMIE. Volume: (2017) - 2017 • 4471
Enhanced strength and ductility in a friction stir processing engineered dual phase high entropy alloy
Nene, S.S. and Liu, K. and Frank, M. and Mishra, R.S. and Brennan, R.E. and Cho, K.C. and Li, Z. and Raabe, D.
SCIENTIFIC REPORTS. Volume: 7 (2017) - 2017 • 4470
Correlating Oxygen Evolution Catalysts Activity and Electronic Structure by a High-Throughput Investigation of Ni 1-y-z Fe y Cr z O x
Schwanke, C. and Stein, H.S. and Xi, L. and Sliozberg, K. and Schuhmann, W. and Ludwig, Al. and Lange, K.M.
SCIENTIFIC REPORTS. Volume: 7 (2017) - 2017 • 4469
Formation of nanometer-sized Cu-Sn-Se particles in Cu2ZnSnSe4 thin-films and their effect on solar cell efficiency
Schwarz, T. and Cojocaru-Mirédin, O. and Mousel, M. and Redinger, A. and Raabe, D. and Choi, P.-P.
ACTA MATERIALIA. Volume: 132 (2017) - 2017 • 4468
Absolutely calibrated mass spectrometry measurement of reactive and stable plasma chemistry products in the effluent of a He/H2O atmospheric plasma
Willems, G. and Benedikt, J. and Von Keudell, A.
JOURNAL OF PHYSICS D: APPLIED PHYSICS. Volume: 50 (2017) - 2017 • 4467
Magnetic fluctuations and superconducting properties of CaKFe4As4 studied by As 75 NMR
Cui, J. and Ding, Q.-P. and Meier, W.R. and Böhmer, A.E. and Kong, T. and Borisov, V. and Lee, Y. and Bud'Ko, S.L. and Valentí, R. and Canfield, P.C. and Furukawa, Y.
PHYSICAL REVIEW B. Volume: 96 (2017) - 2017 • 4466
Phase-field study of ripening and rearrangement of precipitates under chemomechanical coupling
Schwarze, C. and Gupta, A. and Hickel, T. and Darvishi Kamachali, R.
PHYSICAL REVIEW B. Volume: 95 (2017) - 2017 • 4465
Mechanisms of oxygen permeation through plastic films and barrier coatings
Wilski, S. and Wipperfürth, J. and Jaritz, M. and Kirchheim, D. and Mitschker, F. and Awakowicz, P. and Dahlmann, R. and Hopmann, C.
JOURNAL OF PHYSICS D: APPLIED PHYSICS. Volume: 50 (2017) - 2017 • 4464
Massive nanoprecipitation in an Fe-19Ni-xAl maraging steel triggered by the intrinsic heat treatment during laser metal deposition
Kürnsteiner, P. and Wilms, M.B. and Weisheit, A. and Barriobero-Vila, P. and Jägle, E.A. and Raabe, D.
ACTA MATERIALIA. Volume: 129 (2017) - 2017 • 4463
On the evolution of cast microstructures during processing of single crystal Ni-base superalloys using a Bridgman seed technique
Hallensleben, P. and Schaar, H. and Thome, P. and Jöns, N. and Jafarizadeh, A. and Steinbach, I. and Eggeler, G. and Frenzel, J.
MATERIALS AND DESIGN. Volume: 128 (2017) - 2017 • 4462
A Study on Microstructural Parameters for the Characterization of Granular Porous Ceramics Using a Combination of Stochastic and Mechanical Modeling
Kulosa, M. and Neumann, M. and Boeff, M. and Gaiselmann, G. and Schmidt, V. and Hartmaier, A.
INTERNATIONAL JOURNAL OF APPLIED MECHANICS. Volume: 9 (2017) - 2017 • 4461
Structure map including off-stoichiometric and ternary sp-d-valent compounds
Hammerschmidt, T. and Bialon, A.F. and Drautz, R.
MODELLING AND SIMULATION IN MATERIALS SCIENCE AND ENGINEERING. Volume: 25 (2017) - 2017 • 4460
The effects of prior austenite grain boundaries and microstructural morphology on the impact toughness of intercritically annealed medium Mn steel
Han, J. and da Silva, A.K. and Ponge, D. and Raabe, D. and Lee, S.-M. and Lee, Y.-K. and Lee, S.-I. and Hwang, B.
ACTA MATERIALIA. Volume: 122 (2017) - 2017 • 4459
Accumulating the hydride state in the catalytic cycle of [FeFe]-hydrogenases
Winkler, M. and Senger, M. and Duan, J. and Esselborn, J. and Wittkamp, F. and Hofmann, E. and Apfel, U.-P. and Stripp, S.T. and Happe, T.
NATURE COMMUNICATIONS. Volume: 8 (2017) - 2017 • 4458
Superplasticity in a lean Fe-Mn-Al steel
Han, J. and Kang, S.-H. and Lee, S.-J. and Kawasaki, M. and Lee, H.-J. and Ponge, D. and Raabe, D. and Lee, Y.-K.
NATURE COMMUNICATIONS. Volume: 8 (2017) - 2017 • 4457
Single electron-photon pair creation from a single polarization-entangled photon pair
Kuroyama, K. and Larsson, M. and Matsuo, S. and Fujita, T. and Valentin, S.R. and Ludwig, Ar. and Wieck, A.D. and Oiwa, A. and Tarucha, S.
SCIENTIFIC REPORTS. Volume: 7 (2017) - 2017 • 4456
A polytree-based adaptive approach to limit analysis of cracked structures
Nguyen-Xuan, H. and Nguyen-Hoang, S. and Rabczuk, T. and Hackl, K.
COMPUTER METHODS IN APPLIED MECHANICS AND ENGINEERING. Volume: 313 (2017) - 2017 • 4455
High-throughput heterodyne thermoreflectance: Application to thermal conductivity measurements of a Fe-Si-Ge thin film alloy library
D'Acremont, Q. and Pernot, G. and Rampnoux, J.-M. and Furlan, A. and Lacroix, D. and Ludwig, Al. and Dilhaire, S.
REVIEW OF SCIENTIFIC INSTRUMENTS. Volume: 88 (2017) - 2017 • 4454
Promoting Photocatalytic Overall Water Splitting by Controlled Magnesium Incorporation in SrTiO3 Photocatalysts
Han, K. and Lin, Y.-C. and Yang, C.-M. and Jong, R. and Mul, G. and Mei, B.
CHEMSUSCHEM. Volume: 10 (2017) - 2017 • 4453
Electron dynamics in transport and optical measurements of self-assembled quantum dots
Kurzmann, A. and Merkel, B. and Marquardt, B. and Beckel, A. and Ludwig, Ar. and Wieck, A.D. and Lorke, A. and Geller, M.
PHYSICA STATUS SOLIDI (B) BASIC RESEARCH. Volume: 254 (2017) - 2017 • 4452
Solvent-free hierarchization of zeolites by carbochlorination
Nichterwitz, M. and Grätz, S. and Nickel, W. and Borchardt, L.
JOURNAL OF MATERIALS CHEMISTRY A. Volume: 5 (2017) - 2017 • 4451
Transient Behavior of Ni@NiOx Functionalized SrTiO3 in Overall Water Splitting
Han, K. and Kreuger, T. and Mei, B. and Mul, G.
ACS CATALYSIS. Volume: 7 (2017) - 2017 • 4450
Fractal antenna arrays for MIMO radar applications
Dahl, C. and Vogt, M. and Rolfes, I.
INTERNATIONAL JOURNAL OF MICROWAVE AND WIRELESS TECHNOLOGIES. Volume: 9 (2017) - 2017 • 4449
High-throughput study of binary thin film tungsten alloys
Nikolić, V. and Wurster, S. and Savan, A. and Ludwig, Al. and Pippan, R.
INTERNATIONAL JOURNAL OF REFRACTORY METALS AND HARD MATERIALS. Volume: 69 (2017) - 2017 • 4448
Simulating municipal solid waste incineration with a DEM/CFD method – Influences of waste properties, grate and furnace design
Wissing, F. and Wirtz, S. and Scherer, V.
FUEL. Volume: 206 (2017) - 2017 • 4447
Spin dynamics of quadrupole nuclei in InGaAs quantum dots
Kuznetsova, M.S. and Cherbunin, R.V. and Gerlovin, I.Y. and Ignatiev, I.V. and Verbin, S.Y. and Yakovlev, D.R. and Reuter, D. and Wieck, A.D. and Bayer, M.
PHYSICAL REVIEW B. Volume: 95 (2017) - 2017 • 4446
CD44 mediates the catch-bond activated rolling of HEPG2Iso epithelial cancer cells on hyaluronan
Hanke-Roos, M. and Fuchs, K. and Maleschlijski, S. and Sleeman, J. and Orian-Rousseau, V. and Rosenhahn, A.
CELL ADHESION AND MIGRATION. Volume: (2017) - 2017 • 4445
Confined chemical and structural states at dislocations in Fe-9wt%Mn steels: A correlative TEM-atom probe study combined with multiscale modelling
Kwiatkowski da Silva, A. and Leyson, G. and Kuzmina, M. and Ponge, D. and Herbig, M. and Sandlöbes, S. and Gault, B. and Neugebauer, J. and Raabe, D.
ACTA MATERIALIA. Volume: 124 (2017) - 2017 • 4444
Simulation based evaluation of time-variant loadings acting on tunnel linings during mechanized tunnel construction
Ninić, J. and Meschke, G.
ENGINEERING STRUCTURES. Volume: 135 (2017) - 2017 • 4443
The effect of realistic heavy particle induced secondary electron emission coefficients on the electron power absorption dynamics in single- and dual-frequency capacitively coupled plasmas
Daksha, M. and Derzsi, A. and Wilczek, S. and Trieschmann, J. and Mussenbrock, T. and Awakowicz, P. and Donkó, Z. and Schulze, J.
PLASMA SOURCES SCIENCE AND TECHNOLOGY. Volume: 26 (2017) - 2017 • 4442
A hybrid finite element and surrogate modelling approach for simulation and monitoring supported TBM steering
Ninić, J. and Freitag, S. and Meschke, G.
TUNNELLING AND UNDERGROUND SPACE TECHNOLOGY. Volume: 63 (2017) - 2017 • 4441
A coupled computational approach for the simulation of soil excavation and transport in earth-pressure balance shield machines
Dang, T.S. and Wessels, N. and Nguyen, N.-S. and Hackl, K. and Meschke, G.
INTERNATIONAL JOURNAL FOR MULTISCALE COMPUTATIONAL ENGINEERING. Volume: 15 (2017) - 2017 • 4440
Stability, phase separation and oxidation of a supersaturated nanocrystalline Cu-33 at.% Cr thin film alloy
Harzer, T.P. and Dehm, G.
THIN SOLID FILMS. Volume: 623 (2017) - 2017 • 4439
Ab-initio study of C and N point defects in the C14-Fe2Nb phase
Ladines, A.N. and Drautz, R. and Hammerschmidt, T.
JOURNAL OF ALLOYS AND COMPOUNDS. Volume: 693 (2017) - 2017 • 4438
Electrode potential dependent desolvation and resolvation of germanium(100) in contact with aqueous perchlorate electrolytes
Niu, F. and Schulz, R. and Castañeda Medina, A. and Schmid, R. and Erbe, A.
PHYSICAL CHEMISTRY CHEMICAL PHYSICS. Volume: 19 (2017) - 2017 • 4437
Multiscale modeling of the HKUST-1/polyvinyl alcohol) interface: From an atomistic to a coarse graining approach
Semino, R. and Durholt, J.P. and Schmid, R. and Marin, G.
JOURNAL OF PHYSICAL CHEMISTRY C. Volume: 121 (2017) - 2017 • 4436
Multiscale characterization of White Etching Cracks (WEC) in a 100Cr6 bearing from a thrust bearing test rig
Danielsen, H.K. and Guzmán, F.G. and Dahl, K.V. and Li, Y.J. and Wu, J. and Jacobs, G. and Burghardt, G. and Fæster, S. and Alimadadi, H. and Goto, S. and Raabe, D. and Petrov, R.
WEAR. Volume: 370-371 (2017) - 2017 • 4435
In–situ TEM study of diffusion kinetics and electron irradiation effects on the Cr phase separation of a nanocrystalline Cu–4 at.% Cr thin film alloy
Harzer, T.P. and Duarte, M.J. and Dehm, G.
JOURNAL OF ALLOYS AND COMPOUNDS. Volume: 695 (2017) - 2017 • 4434
A triangular triple quantum dot with tunable tunnel couplings
Noiri, A. and Kawasaki, K. and Otsuka, T. and Nakajima, T. and Yoneda, J. and Amaha, S. and Delbecq, M.R. and Takeda, K. and Allison, G. and Ludwig, Ar. and Wieck, A.D. and Tarucha, S.
SEMICONDUCTOR SCIENCE AND TECHNOLOGY. Volume: 32 (2017) - 2017 • 4433
Proton-Coupled Reduction of the Catalytic [4Fe-4S] Cluster in [FeFe]-Hydrogenases
Senger, M. and Laun, K. and Wittkamp, F. and Duan, J. and Haumann, M. and Happe, T. and Winkler, M. and Apfel, U.-P. and Stripp, S.T.
ANGEWANDTE CHEMIE - INTERNATIONAL EDITION. Volume: 56 (2017) - 2017 • 4432
Inverse Ripening and Rearrangement of Precipitates under Chemomechanical Coupling
Darvishi Kamachali, R. and Schwarze, C.
COMPUTATIONAL MATERIALS SCIENCE. Volume: 130 (2017) - 2017 • 4431
Microwave near-field sensor for the contactless detection of material fluctuations
Hattenhorst, B. and Baer, C. and Musch, T. and Schulz, C. and Rolfes, I.
EUROPEAN MICROWAVE WEEK 2016: "MICROWAVES EVERYWHERE", EUMW 2016 - CONFERENCE PROCEEDINGS; 46TH EUROPEAN MICROWAVE CONFERENCE, EUMC 2016. Volume: (2017) - 2017 • 4430
Microfluidic accumulation assay probes attachment of biofilm forming diatom cells
Nolte, K.A. and Schwarze, J. and Rosenhahn, A.
BIOFOULING. Volume: 33 (2017) - 2017 • 4429
Core-shell nanoparticle arrays double the strength of steel
Seol, J.-B. and Na, S.-H. and Gault, B. and Kim, J.-E. and Han, J.-C. and Park, C.-G. and Raabe, D.
SCIENTIFIC REPORTS. Volume: 7 (2017) - 2017 • 4428
Tuning rule for linear control of nonlinear reactive sputter processes
Woelfel, C. and Awakowicz, P. and Lunze, J.
PROCEEDINGS OF THE 2017 21ST INTERNATIONAL CONFERENCE ON PROCESS CONTROL, PC 2017. Volume: (2017) - 2017 • 4427
Itinerant G-type antiferromagnetic order in SrCr2As2
Das, P. and Sangeetha, N.S. and Lindemann, G.R. and Heitmann, T.W. and Kreyssig, A. and Goldman, A.I. and McQueeney, R.J. and Johnston, D.C. and Vaknin, D.
PHYSICAL REVIEW B. Volume: 96 (2017) - 2017 • 4426
Dielectric phantom materials for broadband biomedical applications
Hattenhorst, B. and Mallach, M. and Baer, C. and Musch, T. and Barowski, J. and Rolfes, I.
2017 1ST IEEE MTT-S INTERNATIONAL MICROWAVE BIO CONFERENCE, IMBIOC 2017. Volume: (2017) - 2017 • 4425
Surface State Tunneling Signatures in the Two-Component Superconductor UPt3
Lambert, F. and Akbari, A. and Thalmeier, P. and Eremin, I.
PHYSICAL REVIEW LETTERS. Volume: 118 (2017) - 2017 • 4424
Accurate Neural Network Description of Surface Phonons in Reactive Gas-Surface Dynamics: N2 + Ru(0001)
Shakouri, K. and Behler, J. and Meyer, J. and Kroes, G.-J.
JOURNAL OF PHYSICAL CHEMISTRY LETTERS. Volume: 8 (2017) - 2017 • 4423
Crystal electric field excitations in the quasicrystal approximant TbCd6 studied by inelastic neutron scattering
Das, P. and Lory, P.-F. and Flint, R. and Kong, T. and Hiroto, T. and Bud'Ko, S.L. and Canfield, P.C. and De Boissieu, M. and Kreyssig, A. and Goldman, A.I.
PHYSICAL REVIEW B. Volume: 95 (2017) - 2017 • 4422
Interplay between CN- Ligands and the Secondary Coordination Sphere of the H-Cluster in [FeFe]-Hydrogenases
Lampret, O. and Adamska-Venkatesh, A. and Konegger, H. and Wittkamp, F. and Apfel, U.-P. and Reijerse, E.J. and Lubitz, W. and Rüdiger, O. and Happe, T. and Winkler, M.
JOURNAL OF THE AMERICAN CHEMICAL SOCIETY. Volume: 139 (2017) - 2017 • 4421
Collinear antiferromagnetism in trigonal SrMn2As2 revealed by single-crystal neutron diffraction
Das, P. and Sangeetha, N.S. and Pandey, A. and Benson, Z.A. and Heitmann, T.W. and Johnston, D.C. and Goldman, A.I. and Kreyssig, A.
JOURNAL OF PHYSICS CONDENSED MATTER. Volume: 29 (2017) - 2017 • 4420
Investigations on the Nature of Ceramic Deposits in Plasma Spray–Physical Vapor Deposition
He, W. and Mauer, G. and Gindrat, M. and Wäger, R. and Vaßen, R.
JOURNAL OF THERMAL SPRAY TECHNOLOGY. Volume: 26 (2017) - 2017 • 4419
Elasto-viscoplastic phase field modelling of anisotropic cleavage fracture
Shanthraj, P. and Svendsen, B. and Sharma, L. and Roters, F. and Raabe, D.
JOURNAL OF THE MECHANICS AND PHYSICS OF SOLIDS. Volume: 99 (2017) - 2017 • 4418
Excitation Temperature and Constituent Concentration Profiles of the Plasma Jet Under Plasma Spray-PVD Conditions
He, W. and Mauer, G. and Vaßen, R.
PLASMA CHEMISTRY AND PLASMA PROCESSING. Volume: 37 (2017) - 2017 • 4417
Low temperature growth of gallium oxide thin films via plasma enhanced atomic layer deposition
O'Donoghue, R. and Rechmann, J. and Aghaee, M. and Rogalla, D. and Becker, H.-W. and Creatore, M. and Wieck, A.D. and Devi, A.
DALTON TRANSACTIONS. Volume: 46 (2017) - 2017 • 4416
Dichotomy between in-plane magnetic susceptibility and resistivity anisotropies in extremely strained BaFe2As2
He, M. and Wang, L. and Ahn, F. and Hardy, F. and Wolf, T. and Adelmann, P. and Schmalian, J. and Eremin, I. and Meingast, C.
NATURE COMMUNICATIONS. Volume: 8 (2017) - 2017 • 4415
Shape memory micro-and nanowire libraries for the high-throughput investigation of scaling effects
Oellers, T. and König, D. and Kostka, A. and Xie, S. and Brugger, J. and Ludwig, Al.
ACS COMBINATORIAL SCIENCE. Volume: 19 (2017) - 2017 • 4414
Kinetic investigation of the ion angular distribution in capacitive radio-frequency plasmas
Shihab, M. and Mussenbrock, T.
PHYSICS OF PLASMAS. Volume: 24 (2017) - 2017 • 4413
Investigation of plasma spokes in reactive high power impulse magnetron sputtering discharge
Hecimovic, A. and Corbella, C. and Maszl, C. and Breilmann, W. and Von Keudell, A.
JOURNAL OF APPLIED PHYSICS. Volume: 121 (2017) - 2017 • 4412
Effect of temperature and texture on the reorientation of martensite variants in NiTi shape memory alloys
Laplanche, G. and Birk, T. and Schneider, S. and Frenzel, J. and Eggeler, G.
ACTA MATERIALIA. Volume: 127 (2017) - 2017 • 4411
High-Tc superconductivity in undoped ThFeAsN
Shiroka, T. and Shang, T. and Wang, C. and Cao, G.-H. and Eremin, I. and Ott, H.-R. and Mesot, J.
NATURE COMMUNICATIONS. Volume: 8 (2017) - 2017 • 4410
Efficiency enhancement of the coherent electron spin-flip Raman scattering through thermal phonons in (In,Ga)As/GaAs quantum dots
Debus, J. and Kudlacik, D. and Waldkirch, P. and Sapega, V.F. and Scholz, S. and Ludwig, Ar. and Wieck, A.D. and Bayer, M.
PHYSICAL REVIEW B. Volume: 95 (2017) - 2017 • 4409
Probing the electron density in HiPIMS plasmas by target inserts
Hecimovic, A. and Held, J. and Schulz-Von Der Gathen, V. and Breilmann, W. and Maszl, C. and Von Keudell, A.
JOURNAL OF PHYSICS D: APPLIED PHYSICS. Volume: 50 (2017) - 2017 • 4408
Reasons for the superior mechanical properties of medium-entropy CrCoNi compared to high-entropy CrMnFeCoNi
Laplanche, G. and Kostka, A. and Reinhart, C. and Hunfeld, J. and Eggeler, G. and George, E.P.
ACTA MATERIALIA. Volume: 128 (2017) - 2017 • 4407
On the alternating physicochemical characteristics of Colombian coal during pyrolysis
Wütscher, A. and Wedler, C. and Seibel, C. and Hiltrop, D. and Fieback, T.M. and Muhler, M. and Span, R.
JOURNAL OF ANALYTICAL AND APPLIED PYROLYSIS. Volume: 123 (2017) - 2017 • 4406
Spatially resolved measurements of the physical plasma parameters and the chemical modifications in a twin surface dielectric barrier discharge for gas flow purification
Offerhaus, B. and Lackmann, J.-W. and Kogelheide, F. and Bracht, V. and Smith, R. and Bibinov, N. and Stapelmann, K. and Awakowicz, P.
PLASMA PROCESSES AND POLYMERS. Volume: 14 (2017) - 2017 • 4405
Sunlight-Dependent Hydrogen Production by Photosensitizer/Hydrogenase Systems
Adam, D. and Bösche, L. and Castañeda-Losada, L. and Winkler, M. and Apfel, U.-P. and Happe, T.
CHEMSUSCHEM. Volume: 10 (2017) - 2017 • 4404
High-Throughput Structural and Functional Characterization of the Thin Film Materials System Ni-Co-Al
Decker, P. and Naujoks, D. and Langenkämper, D. and Somsen, C. and Ludwig, Al.
ACS COMBINATORIAL SCIENCE. Volume: 19 (2017) - 2017 • 4403
High-Performance Energy Storage and Conversion Materials Derived from a Single Metal-Organic Framework/Graphene Aerogel Composite
Xia, W. and Qu, C. and Liang, Z. and Zhao, B. and Dai, S. and Qiu, B. and Jiao, Y. and Zhang, Q. and Huang, X. and Guo, W. and Dang, D. and Zou, R. and Xia, D. and Xu, Q. and Liu, M.
NANO LETTERS. Volume: 17 (2017) - 2017 • 4402
Biofest: Bioinspired Chemistry, Biomaterials and Bioelectrochemistry
De Cola, L. and Schuhmann, W.
CHEMPLUSCHEM. Volume: 82 (2017) - 2017 • 4401
Special Focus on Next Generation Coatings for Gas Turbines
Lau, Y.C. and Dorfman, M. and Li, L. and Vaßen, R.
JOURNAL OF THERMAL SPRAY TECHNOLOGY. Volume: 26 (2017) - 2017 • 4400
Conversion from single photon to single electron spin using electrically controllable quantum dots
Oiwa, A. and Fujita, T. and Kiyama, H. and Allison, G. and Ludwig, Ar. and Wieck, A.D. and Tarucha, S.
JOURNAL OF THE PHYSICAL SOCIETY OF JAPAN. Volume: 86 (2017) - 2017 • 4399
Dome of magnetic order inside the nematic phase of sulfur-substituted FeSe under pressure
Xiang, L. and Kaluarachchi, U.S. and Böhmer, A.E. and Taufour, V. and Tanatar, M.A. and Prozorov, R. and Bud'Ko, S.L. and Canfield, P.C.
PHYSICAL REVIEW B. Volume: 96 (2017) - 2017 • 4398
Composite targets in HiPIMS plasmas: Correlation of in-vacuum XPS characterization and optical plasma diagnostics
Layes, V. and Monje, S. and Corbella, C. and Schulz-Von der Gathen, V. and Von Keudell, A. and De Los Arcos, T.
JOURNAL OF APPLIED PHYSICS. Volume: 121 (2017) - 2017 • 4397
Abnormal grain growth in Eurofer-97 steel in the ferrite phase field
Oliveira, V.B. and Sandim, H.R.Z. and Raabe, D.
JOURNAL OF NUCLEAR MATERIALS. Volume: 485 (2017) - 2017 • 4396
Low-cost fabrication of nanoimprint templates with tunable feature sizes at a constant pitch
Si, S. and Dittrich, L. and Hoffmann, M.
MICROELECTRONIC ENGINEERING. Volume: 170 (2017) - 2017 • 4395
Species transport on the target during high power impulse magnetron sputtering
Layes, V. and Monje, S. and Corbella, C. and Trieschmann, J. and De Los Arcos, T. and Von Keudell, A.
APPLIED PHYSICS LETTERS. Volume: 110 (2017) - 2017 • 4394
POD and Galerkin-based reduction of a wood chip drying model
Oliver Berner, M. and Sudbrock, F. and Scherer, V. and Mönnigmann, M.
IFAC-PAPERSONLINE. Volume: 50 (2017) - 2017 • 4393
Consecutive imprinting performance of large area UV nanoimprint lithography using Bi-layer soft stamps in ambient atmosphere
Si, S. and Hoffmann, M.
MICROELECTRONIC ENGINEERING. Volume: 176 (2017) - 2017 • 4392
Topographic cues guide the attachment of diatom cells and algal zoospores
Xiao, L. and Finlay, J.A. and Röhrig, M. and Mieszkin, S. and Worgull, M. and Hölscher, H. and Callow, J.A. and Callow, M.E. and Grunze, M. and Rosenhahn, A.
BIOFOULING. Volume: (2017) - 2017 • 4391
Transmission electron microscopy study of the microstructural evolution during higherature and low-stress (011) [11] shear creep deformation of the superalloy single crystal LEK 94
Agudo Jácome, L. and Göbenli, G. and Eggeler, G.
JOURNAL OF MATERIALS RESEARCH. Volume: 32 (2017) - 2017 • 4390
The NanoTuFe — Fabrication of large area periodic nanopatterns with tunable feature sizes at low cost
Si, S. and Dittrich, L. and Hoffmann, M.
MICROELECTRONIC ENGINEERING. Volume: 180 (2017) - 2017 • 4389
Recommended reading list of early publications on atomic layer deposition-Outcome of the "Virtual Project on the History of ALD"
Ahvenniemi, E. and Akbashev, A. R. and Ali, S. and Bechelany, M. and Berdova, M. and Boyadjiev, S. and Cameron, D. C. and Chen, R. and Chubarov, M. and Cremers, V. and Devi, A. and Drozd, V. and Elnikova, L. and Gottardi, G. and Grigoras, K. and Hausmann, D. M. and Hwang, C. S. and Jen, S. and Kallio, T. and Kanervo, J. and Khmelnitskiy, I. and Kim, D. H. and Klibanov, L. and Koshtyal, Y. and Krause, A. O. I. and Kuhs, J. and Kaerkkaenen, I. and Kaariainen, M. and Kaariainen, T. and Lamagna, L. and Lapicki, A. A. and Leskela, M. and Lipsanen, H. and Lyytinen, J. and Malkov, A. and Malygin, A. and Mennad, A. and Militzer, C. and Molarius, J. and Norek, M. and Ozgit-Akgun, C. and Panov, M. and Pedersen, H. and Piallat, F. and Popov, G. and Puurunen, R. L. and Rampelberg, G. and Ras, R. H. A. and Rauwel, E. and Roozeboom, F. and Sajavaara, T. and Salami, H. and Savin, H. and Schneider, N. and Seidel, T. E. and Sundqvist, J. and Suyatin, D. B. and Torndahl, T. and van Ommen, J. R. and Wiemer, C. and Ylivaara, O. M. E. and Yurkevich, O.
JOURNAL OF VACUUM SCIENCE & TECHNOLOGY A. Volume: 35 (2017) - 2017 • 4388
Review Article: Recommended reading list of early publications on atomic layer deposition - Outcome of the "virtual Project on the History of ALD"
Ahvenniemi, E. and Akbashev, A.R. and Ali, S. and Bechelany, M. and Berdova, M. and Boyadjiev, S. and Cameron, D.C. and Chen, R. and Chubarov, M. and Cremers, V. and Devi, A. and Drozd, V. and Elnikova, L. and Gottardi, G. and Grigoras, K. and Hausmann, D.M. and Hwang, C.S. and Jen, S.-H. and Kallio, T. and Kanervo, J. and Khmelnitskiy, I. and Kim, D.H. and Klibanov, L. and Koshtyal, Y. and Krause, A.O.I. and Kuhs, J. and Kärkkänen, I. and Kääriäinen, M.-L. and Kääriäinen, T. and Lamagna, L. and Łapicki, A.A. and Leskelä, M. and Lipsanen, H. and Lyytinen, J. and Malkov, A. and Malygin, A. and Mennad, A. and Militzer, C. and Molarius, J. and Norek, M. and Özgit-Akgün, Ç. and Panov, M. and Pedersen, H. and Piallat, F. and Popov, G. and Puurunen, R.L. and Rampelberg, G. and Ras, R.H.A. and Rauwel, E. and Roozeboom, F. and Sajavaara, T. and Salami, H. and Savin, H. and Schneider, N. and Seidel, T.E. and Sundqvist, J. and Suyatin, D.B. and Törndahl, T. and Van Ommen, J.R. and Wiemer, C. and Ylivaara, O.M.E. and Yurkevich, O.
JOURNAL OF VACUUM SCIENCE AND TECHNOLOGY A: VACUUM, SURFACES AND FILMS. Volume: 35 (2017) - 2017 • 4387
Monodispersed Mesoporous Silica Spheres Supported Co3O4 as Robust Catalyst for Oxygen Evolution Reaction
Deng, X. and Rin, R. and Tseng, J.-C. and Weidenthaler, C. and Apfel, U.-P. and Tüysüz, H.
CHEMCATCHEM. Volume: 9 (2017) - 2017 • 4386
Efficient and rapid transformation of high silica CHA zeolite from FAU zeolite in the absence of water
Xiong, X. and Yuan, D. and Wu, Q. and Chen, F. and Meng, X. and Lv, R. and Dai, D. and Maurer, S. and McGuire, R. and Feyen, M. and Müller, U. and Zhang, W. and Yokoi, T. and Bao, X. and Gies, H. and Marler, B. and De Vos, D.E. and Kolb, U. and Moini, A. and Xiao, F.-S.
JOURNAL OF MATERIALS CHEMISTRY A. Volume: 5 (2017) - 2017 • 4385
Topotactic Synthesis of Porous Cobalt Ferrite Platelets from a Layered Double Hydroxide Precursor and Their Application in Oxidation Catalysis
Ortega, K.F. and Anke, S. and Salamon, S. and Özcan, F. and Heese, J. and Andronescu, C. and Landers, J. and Wende, H. and Schuhmann, W. and Muhler, M. and Lunkenbein, T. and Behrens, M.
CHEMISTRY - A EUROPEAN JOURNAL. Volume: (2017) - 2017 • 4384
Metal–Organic Framework Derived Carbon Nanotube Grafted Cobalt/Carbon Polyhedra Grown on Nickel Foam: An Efficient 3D Electrode for Full Water Splitting
Aijaz, A. and Masa, J. and Rösler, C. and Xia, W. and Weide, P. and Fischer, R.A. and Schuhmann, W. and Muhler, M.
CHEMELECTROCHEM. Volume: 4 (2017) - 2017 • 4383
MOF-Templated Assembly Approach for Fe3C Nanoparticles Encapsulated in Bamboo-Like N-Doped CNTs: Highly Efficient Oxygen Reduction under Acidic and Basic Conditions
Aijaz, A. and Masa, J. and Rösler, C. and Antoni, H. and Fischer, R.A. and Schuhmann, W. and Muhler, M.
CHEMISTRY - A EUROPEAN JOURNAL. Volume: (2017) - 2017 • 4382
Hankel Determinants of Random Moment Sequences
Dette, H. and Tomecki, D.
JOURNAL OF THEORETICAL PROBABILITY. Volume: 30 (2017) - 2017 • 4381
Higher-order spin and charge dynamics in a quantum dot-lead hybrid system
Otsuka, T. and Nakajima, T. and Delbecq, M.R. and Amaha, S. and Yoneda, J. and Takeda, K. and Allison, G. and Stano, P. and Noiri, A. and Ito, T. and Loss, D. and Ludwig, Ar. and Wieck, A.D. and Tarucha, S.
SCIENTIFIC REPORTS. Volume: 7 (2017) - 2017 • 4380
Synergistic Effect of Cobalt and Iron in Layered Double Hydroxide Catalysts for the Oxygen Evolution Reaction
Yang, F. and Sliozberg, K. and Sinev, I. and Antoni, H. and Bähr, A. and Ollegott, K. and Xia, W. and Masa, J. and Grünert, W. and Cuenya, B.R. and Schuhmann, W. and Muhler, M.
CHEMSUSCHEM. Volume: 10 (2017) - 2017 • 4379
Optimal designs for comparing regression models with correlated observations
Dette, H. and Schorning, K. and Konstantinou, M.
COMPUTATIONAL STATISTICS AND DATA ANALYSIS. Volume: 113 (2017) - 2017 • 4378
T-optimal discriminating designs for Fourier regression models
Dette, H. and Melas, V.B. and Shpilev, P.
COMPUTATIONAL STATISTICS AND DATA ANALYSIS. Volume: 113 (2017) - 2017 • 4377
Co3O4@Co/NCNT Nanostructure Derived from a Dicyanamide-Based Metal-Organic Framework as an Efficient Bi-functional Electrocatalyst for Oxygen Reduction and Evolution Reactions
Sikdar, N. and Konkena, B. and Masa, J. and Schuhmann, W. and Maji, T.K.
CHEMISTRY - A EUROPEAN JOURNAL. Volume: 23 (2017) - 2017 • 4376
NH3 Post-Treatment Induces High Activity of Co-Based Electrocatalysts Supported on Carbon Nanotubes for the Oxygen Evolution Reaction
Yang, F. and Xia, W. and Maljusch, A. and Masa, J. and Hollmann, D. and Sinev, I. and Cuenya, B.R. and Schuhmann, W. and Muhler, M.
CHEMELECTROCHEM. Volume: 4 (2017) - 2017 • 4375
Efficient sampling in materials simulation - Exploring the parameter space of grain boundaries
Dette, H. and Gösmann, J. and Greiff, C. and Janisch, R.
ACTA MATERIALIA. Volume: 125 (2017) - 2017 • 4374
Crystal structures of Fe4C vs. Fe4N analysed by DFT calculations: Fcc-based interstitial superstructures explored
Leineweber, A. and Hickel, T. and Azimi-Manavi, B. and Maisel, S.B.
ACTA MATERIALIA. Volume: 140 (2017) - 2017 • 4373
Semi-analytical Modeling and Vibration Control of a Geometrically Nonlinear Plate
Oveisi, A. and Nestorović, T. and Nguyen, N.L.
INTERNATIONAL JOURNAL OF STRUCTURAL STABILITY AND DYNAMICS. Volume: 17 (2017) - 2017 • 4372
Effects of substrate roughness and spray-angle on deposition behavior of cold-sprayed Inconel 718
Singh, R. and Rauwald, K.-H. and Wessel, E. and Mauer, G. and Schruefer, S. and Barth, A. and Wilson, S. and Vassen, R.
SURFACE AND COATINGS TECHNOLOGY. Volume: 319 (2017) - 2017 • 4371
Optimal designs for thermal spraying
Dette, H. and Hoyden, L. and Kuhnt, S. and Schorning, K.
JOURNAL OF THE ROYAL STATISTICAL SOCIETY. SERIES C: APPLIED STATISTICS. Volume: 66 (2017) - 2017 • 4370
Mechanochemistry-assisted synthesis of hierarchical porous carbons applied as supercapacitors
Leistenschneider, D. and Jäckel, N. and Hippauf, F. and Presser, V. and Borchardt, L.
BEILSTEIN JOURNAL OF ORGANIC CHEMISTRY. Volume: 13 (2017) - 2017 • 4369
Transient response of an active nonlinear sandwich piezolaminated plate
Oveisi, A. and Nestorović, T.
COMMUNICATIONS IN NONLINEAR SCIENCE AND NUMERICAL SIMULATION. Volume: 45 (2017) - 2017 • 4368
Robust Bain distortion in the premartensite phase of a platinum-substituted Ni2MnGa magnetic shape memory alloy
Singh, S. and Dutta, B. and D'Souza, S.W. and Zavareh, M.G. and Devi, P. and Gibbs, A.S. and Hickel, T. and Chadov, S. and Felser, C. and Pandey, D.
NATURE COMMUNICATIONS. Volume: 8 (2017) - 2017 • 4367
Strengthening and strain hardening mechanisms in a precipitation-hardened high-Mn lightweight steel
Yao, M.J. and Welsch, E. and Ponge, D. and Haghighat, S.M.H. and Sandlöbes, S. and Choi, P. and Herbig, M. and Bleskov, I. and Hickel, T. and Lipinska-Chwalek, M. and Shanthraj, P. and Scheu, C. and Zaefferer, S. and Gault, B. and Raabe, D.
ACTA MATERIALIA. Volume: 140 (2017) - 2017 • 4366
A new approach to optimal designs for correlated observations
Dette, H. and Konstantinou, M. and Zhigljavsky, A.
ANNALS OF STATISTICS. Volume: 45 (2017) - 2017 • 4365
Isothermal and cyclic oxidation behavior of free standing MCrAlY coatings manufactured by high-velocity atmospheric plasma spraying
Hejrani, E. and Sebold, D. and Nowak, W.J. and Mauer, G. and Naumenko, D. and Vaßen, R. and Quadakkers, W.J.
SURFACE AND COATINGS TECHNOLOGY. Volume: 313 (2017) - 2017 • 4364
Disturbance rejection control based on state-reconstruction and persistence disturbance estimation
Oveisi, A. and Aldeen, M. and Nestorović, T.
JOURNAL OF THE FRANKLIN INSTITUTE. Volume: 354 (2017) - 2017 • 4363
Efficient Computation of Bayesian Optimal Discriminating Designs
Dette, H. and Guchenko, R. and Melas, V.B.
JOURNAL OF COMPUTATIONAL AND GRAPHICAL STATISTICS. Volume: 26 (2017) - 2017 • 4362
Natural (Non-)Informative Priors for Skew-symmetric Distributions
Dette, H. and Ley, C. and Rubio, F.
SCANDINAVIAN JOURNAL OF STATISTICS. Volume: (2017) - 2017 • 4361
Microstructure and texture evolution during severe plastic deformation of CrMnFeCoNi high-entropy alloy
Skrotzki, W. and Pukenas, A. and Joni, B. and Odor, E. and Ungar, T. and Hohenwarter, A. and Pippan, R. and George, E.P.
IOP CONFERENCE SERIES: MATERIALS SCIENCE AND ENGINEERING. Volume: 194 (2017) - 2017 • 4360
An Intrinsic Self-Charging Biosupercapacitor Comprised of a High-Potential Bioanode and a Low-Potential Biocathode
Alsaoub, S. and Ruff, A. and Conzuelo, F. and Ventosa, E. and Ludwig, R. and Shleev, S. and Schuhmann, W.
CHEMPLUSCHEM. Volume: (2017) - 2017 • 4359
Ab initio explanation of disorder and off-stoichiometry in Fe-Mn-Al-C κ carbides
Dey, P. and Nazarov, R. and Dutta, B. and Yao, M. and Herbig, M. and Friák, M. and Hickel, T. and Raabe, D. and Neugebauer, J.
PHYSICAL REVIEW B - CONDENSED MATTER AND MATERIALS PHYSICS. Volume: 95 (2017) - 2017 • 4358
Proton-Transfer-Driven Water Exchange Mechanism in the Na+ Solvation Shell
Hellström, M. and Behler, J.
JOURNAL OF PHYSICAL CHEMISTRY B. Volume: 121 (2017) - 2017 • 4357
Effect of silicon on the microstructure and growth kinetics of intermetallic phases formed during hot-dip aluminizing of ferritic steel
Lemmens, B. and Springer, H. and De Graeve, I. and De Strycker, J. and Raabe, D. and Verbeken, K.
SURFACE AND COATINGS TECHNOLOGY. Volume: 319 (2017) - 2017 • 4356
Nonequilibrium spin noise in a quantum dot ensemble
Smirnov, D.S. and Glasenapp, Ph. and Bergen, M. and Glazov, M.M. and Reuter, D. and Wieck, A.D. and Bayer, M. and Greilich, A.
PHYSICAL REVIEW B. Volume: 95 (2017) - 2017 • 4355
Structure of aqueous NaOH solutions: Insights from neural-network-based molecular dynamics simulations
Hellström, M. and Behler, J.
PHYSICAL CHEMISTRY CHEMICAL PHYSICS. Volume: 19 (2017) - 2017 • 4354
Atomistic insight into the non-classical nucleation mechanism during solidification in Ni
Díaz Leines, G. and Drautz, R. and Rogal, J.
JOURNAL OF CHEMICAL PHYSICS. Volume: 146 (2017) - 2017 • 4353
Surface phase diagram prediction from a minimal number of DFT calculations: Redox-active adsorbates on zinc oxide
Hellström, M. and Behler, J.
PHYSICAL CHEMISTRY CHEMICAL PHYSICS. Volume: 19 (2017) - 2017 • 4352
Spectral domain optical coherence tomography for non-destructive testing of protection coatings on metal substrates
Lenz, M. and Mazzon, C. and Dillmann, C. and Gerhardt, N.C. and Welp, H. and Prange, M. and Hofmann, M.R.
APPLIED SCIENCES (SWITZERLAND). Volume: 7 (2017) - 2017 • 4351
Spectroscopic optical coherence tomography for ex vivo brain tumor analysis
Lenz, M. and Krug, R. and Dillmann, C. and Gerling, A. and Gerhardt, N.C. and Welp, H. and Schmieder, K. and Hofmann, M.R.
PROGRESS IN BIOMEDICAL OPTICS AND IMAGING - PROCEEDINGS OF SPIE. Volume: 10054 (2017) - 2017 • 4350
Design of Mg alloys: The effects of Li concentration on the structure and elastic properties in the Mg-Li binary system by first principles calculations
Pavlic, O. and Ibarra-Hernandez, W. and Valencia-Jaime, I. and Singh, S. and Avendaño-Franco, G. and Raabe, D. and Romero, A.H.
JOURNAL OF ALLOYS AND COMPOUNDS. Volume: 691 (2017) - 2017 • 4349
Cascade Continuum Micromechanics model for the effective permeability of solids with distributed microcracks: Comparison with numerical homogenization
Leonhart, D. and Timothy, J.J. and Meschke, G.
MECHANICS OF MATERIALS. Volume: 115 (2017) - 2017 • 4348
On measurement of the thermal diffusivity of moderate and heavily doped semiconductor samples using modulated photothermal infrared radiometry
Pawlak, M. and Panas, A. and Ludwig, Ar. and Wieck, A.D.
THERMOCHIMICA ACTA. Volume: 650 (2017) - 2017 • 4347
Coupled Crystal Plasticity–Phase Field Fracture Simulation Study on Damage Evolution Around a Void: Pore Shape Versus Crystallographic Orientation
Diehl, M. and Wicke, M. and Shanthraj, P. and Roters, F. and Brueckner-Foit, A. and Raabe, D.
JOM. Volume: 69 (2017) - 2017 • 4346
Bio-oil upgrading via vapor-phase ketonization over nanostructured FeOx and MnOx: catalytic performance and mechanistic insight
Heracleous, E. and Gu, D. and Schüth, F. and Bennett, J.A. and Isaacs, M.A. and Lee, A.F. and Wilson, K. and Lappas, A.A.
BIOMASS CONVERSION AND BIOREFINERY. Volume: 7 (2017) - 2017 • 4345
Stress-modulated tilt actuator for tunable optical prisms
Leopold, S. and Paetz, D. and Sinzinger, S. and Hoffmann, M.
SENSORS AND ACTUATORS, A: PHYSICAL. Volume: 266 (2017) - 2017 • 4344
On the infrared absorption coefficient measurement of thick heavily Zn doped GaAs using spectrally resolved modulated photothermal infrared radiometry
Pawlak, M. and Pal, S. and Ludwig, Ar. and Wieck, A.D.
JOURNAL OF APPLIED PHYSICS. Volume: 122 (2017) - 2017 • 4343
Revealing the electronic character of the positive electrode/electrolyte interface in lithium-ion batteries
Zampardi, G. and Trocoli, R. and Schuhmann, W. and La Mantia, F.
PHYSICAL CHEMISTRY CHEMICAL PHYSICS. Volume: 19 (2017) - 2017 • 4342
Identifying Structure–Property Relationships Through DREAM.3D Representative Volume Elements and DAMASK Crystal Plasticity Simulations: An Integrated Computational Materials Engineering Approach
Diehl, M. and Groeber, M. and Haase, C. and Molodov, D.A. and Roters, F. and Raabe, D.
JOM. Volume: 69 (2017) - 2017 • 4341
Classical information transfer between distant quantum dots using individual electrons in fast moving quantum dots
Hermelin, S. and Bertrand, B. and Takada, S. and Yamamoto, M. and Tarucha, S. and Ludwig, Ar. and Wieck, A.D. and Bäuerle, C. and Meunier, T.
PHYSICA STATUS SOLIDI (B) BASIC RESEARCH. Volume: 254 (2017) - 2017 • 4340
Photoactive Zinc Ferrites Fabricated via Conventional CVD Approach
Peeters, D. and Taffa, D.H. and Kerrigan, M.M. and Ney, A. and Jöns, N. and Rogalla, D. and Cwik, S. and Becker, H.-W. and Grafen, M. and Ostendorf, A. and Winter, C.H. and Chakraborty, S. and Wark, M. and Devi, A.
ACS SUSTAINABLE CHEMISTRY AND ENGINEERING. Volume: 5 (2017) - 2017 • 4339
An enhanced lumped element electrical model of a double barrier memristive device
Solan, E. and Dirkmann, S. and Hansen, M. and Schroeder, D. and Kohlstedt, H. and Ziegler, M. and Mussenbrock, T. and Ochs, K.
JOURNAL OF PHYSICS D: APPLIED PHYSICS. Volume: 50 (2017) - 2017 • 4338
Operando Phonon Studies of the Protonation Mechanism in Highly Active Hydrogen Evolution Reaction Pentlandite Catalysts
Zegkinoglou, I. and Zendegani, A. and Sinev, I. and Kunze, S. and Mistry, H. and Jeon, H.S. and Zhao, J. and Hu, M.Y. and Alp, E.E. and Piontek, S. and Smialkowski, M. and Apfel, U.-P. and Körmann, F. and Neugebauer, J. and Hickel, T. and Roldan Cuenya, B.
JOURNAL OF THE AMERICAN CHEMICAL SOCIETY. Volume: 139 (2017) - 2017 • 4337
Crystal plasticity study on stress and strain partitioning in a measured 3D dual phase steel microstructure
Diehl, M. and An, D. and Shanthraj, P. and Zaefferer, S. and Roters, F. and Raabe, D.
PHYSICAL MESOMECHANICS. Volume: 20 (2017) - 2017 • 4336
Nanostructured Fe2O3 Processing via Water-Assisted ALD and Low-Temperature CVD from a Versatile Iron Ketoiminate Precursor
Peeters, D. and Sadlo, A. and Lowjaga, K. and Mendoza Reyes, O. and Wang, L. and Mai, L. and Gebhard, M. and Rogalla, D. and Becker, H.-W. and Giner, I. and Grundmeier, G. and Mitoraj, D. and Grafen, M. and Ostendorf, A. and Beranek, R. and Devi, A.
ADVANCED MATERIALS INTERFACES. Volume: (2017) - 2017 • 4335
A novel type of Co–Ti–Cr-base γ/γ′ superalloys with low mass density
Zenk, C.H. and Povstugar, I. and Li, R. and Rinaldi, F. and Neumeier, S. and Raabe, D. and Göken, M.
ACTA MATERIALIA. Volume: 135 (2017) - 2017 • 4334
Combinatorial screening of Pd-based quaternary electrocatalysts for oxygen reduction reaction in alkaline media
Li, J. and Stein, H.S. and Sliozberg, K. and Liu, J. and Liu, Y. and Sertic, G. and Scanley, E. and Ludwig, Al. and Schroers, J. and Schuhmann, W. and Taylor, A.D.
JOURNAL OF MATERIALS CHEMISTRY A. Volume: 5 (2017) - 2017 • 4333
Atomic structures of twin boundaries in hexagonal close-packed metallic crystals with particular focus on Mg
Pei, Z. and Zhang, X. and Hickel, T. and Friák, M. and Sandlöbes, S. and Dutta, B. and Neugebauer, J.
NPJ COMPUTATIONAL MATERIALS. Volume: 3 (2017) - 2017 • 4332
Adaptive crack modeling with interface solid elements for plain and fiber reinforced concrete structures
Zhan, Y. and Meschke, G.
MATERIALS. Volume: 10 (2017) - 2017 • 4331
Interstitial atoms enable joint twinning and transformation induced plasticity in strong and ductile high-entropy alloys
Li, Z. and Tasan, C.C. and Springer, H. and Gault, B. and Raabe, D.
SCIENTIFIC REPORTS. Volume: 7 (2017) - 2017 • 4330
Evaluation of Analysis Conditions for Laser-Pulsed Atom Probe Tomography: Example of Cemented Tungsten Carbide
Peng, Z. and Choi, P.-P. and Gault, B. and Raabe, D.
MICROSCOPY AND MICROANALYSIS. Volume: 23 (2017) - 2017 • 4329
Atomic scale characterization of white etching area and its adjacent matrix in a martensitic 100Cr6 bearing steel
Li, Y.J. and Herbig, M. and Goto, S. and Raabe, D.
MATERIALS CHARACTERIZATION. Volume: 123 (2017) - 2017 • 4328
Atomic diffusion induced degradation in bimetallic layer coated cemented tungsten carbide
Peng, Z. and Rohwerder, M. and Choi, P.-P. and Gault, B. and Meiners, T. and Friedrichs, M. and Kreilkamp, H. and Klocke, F. and Raabe, D.
CORROSION SCIENCE. Volume: 120 (2017) - 2017 • 4327
Spinel-Structured ZnCr2O4 with Excess Zn Is the Active ZnO/Cr2O3 Catalyst for High-Temperature Methanol Synthesis
Song, H. and Laudenschleger, D. and Carey, J.J. and Ruland, H. and Nolan, M. and Muhler, M.
ACS CATALYSIS. Volume: 7 (2017) - 2017 • 4326
Powder Catalyst Fixation for Post-Electrolysis Structural Characterization of NiFe Layered Double Hydroxide Based Oxygen Evolution Reaction Electrocatalysts
Andronescu, C. and Barwe, S. and Ventosa, E. and Masa, J. and Vasile, E. and Konkena, B. and Möller, S. and Schuhmann, W.
ANGEWANDTE CHEMIE - INTERNATIONAL EDITION. Volume: 56 (2017) - 2017 • 4325
NMR study of the new magnetic superconductor CaK(Fe0.951Ni0.049)4As4: Microscopic coexistence of the hedgehog spin-vortex crystal and superconductivity
Ding, Q.-P. and Meier, W.R. and Böhmer, A.E. and Bud'Ko, S.L. and Canfield, P.C. and Furukawa, Y.
PHYSICAL REVIEW B. Volume: 96 (2017) - 2017 • 4324
A TRIP-assisted dual-phase high-entropy alloy: Grain size and phase fraction effects on deformation behavior
Li, Z. and Tasan, C.C. and Pradeep, K.G. and Raabe, D.
ACTA MATERIALIA. Volume: 131 (2017) - 2017 • 4323
Method for the unique identification of hyperelastic material properties using full-field measures. Application to the passive myocardium material response
Perotti, L.E. and Ponnaluri, A.V.S. and Krishnamoorthi, S. and Balzani, D. and Ennis, D.B. and Klug, W.S.
INTERNATIONAL JOURNAL FOR NUMERICAL METHODS IN BIOMEDICAL ENGINEERING. Volume: 33 (2017) - 2017 • 4322
Interaction between phase transformations and dislocations at incipient plasticity of monocrystalline silicon under nanoindentation
Zhang, J. and Zhang, J. and Wang, Z. and Hartmaier, A. and Yan, Y. and Sun, T.
COMPUTATIONAL MATERIALS SCIENCE. Volume: 131 (2017) - 2017 • 4321
Resistive switching in memristive electrochemical metallization devices
Dirkmann, S. and Mussenbrock, T.
AIP ADVANCES. Volume: 7 (2017) - 2017 • 4320
Impact of Synthesis Parameters on the Formation of Defects in HKUST-1
Zhang, W. and Kauer, M. and Guo, P. and Kunze, S. and Cwik, S. and Muhler, M. and Wang, Y. and Epp, K. and Kieslich, G. and Fischer, R.A.
EUROPEAN JOURNAL OF INORGANIC CHEMISTRY. Volume: 2017 (2017) - 2017 • 4319
Ab initio assisted design of quinary dual-phase high-entropy alloys with transformation-induced plasticity
Li, Z. and Körmann, F. and Grabowski, B. and Neugebauer, J. and Raabe, D.
ACTA MATERIALIA. Volume: 136 (2017) - 2017 • 4318
Complexion-mediated martensitic phase transformation in Titanium
Zhang, J. and Tasan, C.C. and Lai, M.J. and Dippel, A.-C. and Raabe, D.
NATURE COMMUNICATIONS. Volume: 8 (2017) - 2017 • 4317
Microstructural evolution and solid state dewetting of epitaxial Al thin films on sapphire (α-Al2O3)
Hieke, S.W. and Breitbach, B. and Dehm, G. and Scheu, C.
ACTA MATERIALIA. Volume: 133 (2017) - 2017 • 4316
Annealing induced void formation in epitaxial Al thin films on sapphire (α-Al2O3)
Hieke, S.W. and Dehm, G. and Scheu, C.
ACTA MATERIALIA. Volume: 140 (2017) - 2017 • 4315
Mobile zinc increases rapidly in the retina after optic nerve injury and regulates ganglion cell survival and optic nerve regeneration
Li, Y. and Andereggen, L. and Yuki, K. and Omura, K. and Yin, Y. and Gilbert, H.-Y. and Erdogan, B. and Asdourian, M.S. and Shrock, C. and De Lima, S. and Apfel, U.-P. and Zhuo, Y. and Hershfinkel, M. and Lippard, S.J. and Rosenberg, P.A. and Benowitz, L.
PROCEEDINGS OF THE NATIONAL ACADEMY OF SCIENCES OF THE UNITED STATES OF AMERICA. Volume: 114 (2017) - 2017 • 4314
Dislocation mechanisms and 3D twin architectures generate exceptional strength-ductility-toughness combination in CrCoNi medium-entropy alloy
Zhang, Z. and Sheng, H. and Wang, Z. and Gludovatz, B. and Zhang, Z. and George, E.P. and Yu, Q. and Mao, S.X. and Ritchie, R.O.
NATURE COMMUNICATIONS. Volume: 8 (2017) - 2017 • 4313
Revealing the Presence of Mobile Molecules on the Surface
Antczak, G. and Boom, K. and Morgenstern, K.
JOURNAL OF PHYSICAL CHEMISTRY C. Volume: 121 (2017) - 2017 • 4312
Micrometer-Precise Determination of the Thin Electrolyte Layer of a Spectroelectrochemical Cell by Microelectrode Approach Curves
Hiltrop, D. and Masa, J. and Botz, A.J.R. and Lindner, A. and Schuhmann, W. and Muhler, M.
ANALYTICAL CHEMISTRY. Volume: 89 (2017) - 2017 • 4311
Influence of compositional inhomogeneity on mechanical behavior of an interstitial dual-phase high-entropy alloy
Li, Z. and Raabe, D.
MATERIALS CHEMISTRY AND PHYSICS. Volume: (2017) - 2017 • 4310
Accurate electronic free energies of the 3 d,4 d, and 5 d transition metals at high temperatures
Zhang, X. and Grabowski, B. and Körmann, F. and Freysoldt, C. and Neugebauer, J.
PHYSICAL REVIEW B - CONDENSED MATTER AND MATERIALS PHYSICS. Volume: 95 (2017) - 2017 • 4309
Tuning the oxidation state of manganese oxide nanoparticles on oxygen- and nitrogen-functionalized carbon nanotubes for the electrocatalytic oxygen evolution reaction
Antoni, H. and Xia, W. and Masa, J. and Schuhmann, W. and Muhler, M.
PHYSICAL CHEMISTRY CHEMICAL PHYSICS. Volume: 19 (2017) - 2017 • 4308
Large strain synergetic material deformation enabled by hybrid nanolayer architectures
Li, J. and Lu, W. and Zhang, S. and Raabe, D.
SCIENTIFIC REPORTS. Volume: 7 (2017) - 2017 • 4307
Carbon/Silicon Exchange at the Apex of Diphos- and Triphos-Derived Ligands – More Than Just a Substitute?
Petuker, A. and Reback, M.L. and Apfel, U.-P.
EUROPEAN JOURNAL OF INORGANIC CHEMISTRY. Volume: 2017 (2017) - 2017 • 4306
Strong and Ductile Non-equiatomic High-Entropy Alloys: Design, Processing, Microstructure, and Mechanical Properties
Li, Z. and Raabe, D.
JOM. Volume: 69 (2017) - 2017 • 4305
Spontaneous Si-C bond cleavage in (TriphosSi)-nickel complexes
Petuker, A. and Mebs, S. and Schüth, N. and Gerschel, P. and Reback, M.L. and Mallick, B. and Van Gastel, M. and Haumann, M. and Apfel, U.-P.
DALTON TRANSACTIONS. Volume: 46 (2017) - 2017 • 4304
In-situ tracking the structural and chemical evolution of nanostructured CuCr alloys
Zhang, Z. and Guo, J. and Dehm, G. and Pippan, R.
ACTA MATERIALIA. Volume: 138 (2017) - 2017 • 4303
Crystallisation of amorphous Fe – Ti – B alloys as a design pathway for nano-structured high modulus steels
Aparicio-Fernández, R. and Szczepaniak, A. and Springer, H. and Raabe, D.
JOURNAL OF ALLOYS AND COMPOUNDS. Volume: 704 (2017) - 2017 • 4302
Spectroscopic and reactivity differences in metal complexes derived from sulfur containing Triphos homologs
Petuker, A. and Gerschel, P. and Piontek, S. and Ritterskamp, N. and Wittkamp, F. and Iffland, L. and Miller, R. and Van Gastel, M. and Apfel, U.-P.
DALTON TRANSACTIONS. Volume: 46 (2017) - 2017 • 4301
Discovery of orbital-selective Cooper pairing in FeSe
Sprau, P.O. and Kostin, A. and Kreisel, A. and Böhmer, A.E. and Taufour, V. and Canfield, P.C. and Mukherjee, S. and Hirschfeld, P.J. and Andersen, B.M. and Davis, J.C.S.
SCIENCE. Volume: 357 (2017) - 2017 • 4300
Design and preparation of efficient hydroisomerization catalysts by the formation of stable SAPO-11 molecular sieve nanosheets with 10-20 nm thickness and partially blocked acidic sites
Zhang, F. and Liu, Y. and Sun, Q. and Dai, Z. and Gies, H. and Wu, Q. and Pan, S. and Bian, C. and Tian, Z. and Meng, X. and Zhang, Y. and Zou, X. and Yi, X. and Zheng, A. and Wang, L. and Xiao, F.-S.
CHEMICAL COMMUNICATIONS. Volume: 53 (2017) - 2017 • 4299
Anorganische Chemie 2016: Koordinationschemie und Bioanorganik
Apfel, U.-P. and Berkefeld, A. and Demir, S.
NACHRICHTEN AUS DER CHEMIE. Volume: 65 (2017) - 2017 • 4298
Synergistic effect of potassium hydroxide and steam co-treatment on the functionalization of carbon nanotubes applied as basic support in the Pd-catalyzed liquid-phase oxidation of ethanol
Dong, W. and Xia, W. and Xie, K. and Peng, B. and Muhler, M.
CARBON. Volume: 121 (2017) - 2017 • 4297
Improving multitarget tracking using orientation estimates for sorting bulk materials
Pfaff, F. and Kurz, G. and Pieper, C. and Maier, G. and Noack, B. and Kruggel-Emden, H. and Gruna, R. and Hanebeck, U.D. and Wirtz, S. and Scherer, V. and Langle, T. and Beyerer, J.
IEEE INTERNATIONAL CONFERENCE ON MULTISENSOR FUSION AND INTEGRATION FOR INTELLIGENT SYSTEMS. Volume: 2017-November (2017) - 2017 • 4296
Origin of Structural Modulations in Ultrathin Fe Films on Cu(001)
Zhang, X. and Hickel, T. and Rogal, J. and Neugebauer, J.
PHYSICAL REVIEW LETTERS. Volume: 118 (2017) - 2017 • 4295
Separation and characterization of carbonaceous particulate (soot and char) produced from fast pyrolysis of coal in inert and CO2 atmospheres
Apicella, B. and Senneca, O. and Russo, C. and Heuer, S. and Cortese, L. and Cerciello, F. and Scherer, V. and Schiemann, M. and Ciajolo, A.
FUEL. Volume: 201 (2017) - 2017 • 4294
Real-time motion prediction using the chromatic offset of line scan cameras
Pfaff, F. and Maier, G. and Aristov, M. and Noack, B. and Gruna, R. and Hanebeck, U.D. and Längle, T. and Beyerer, J. and Pieper, C. and Kruggel-Emden, H. and Wirtz, S. and Scherer, V.
AT-AUTOMATISIERUNGSTECHNIK. Volume: 65 (2017) - 2017 • 4293
Partial recrystallization of gum metal to achieve enhanced strength and ductility
Zhang, J.-L. and Tasan, C.C. and Lai, M.J. and Yan, D. and Raabe, D.
ACTA MATERIALIA. Volume: 135 (2017) - 2017 • 4292
Atomistic Modeling-Based Design of Novel Materials
Holec, D. and Zhou, L. and Riedl, H. and Koller, C.M. and Mayrhofer, P.H. and Friák, M. and Šob, M. and Körmann, F. and Neugebauer, J. and Music, D. and Hartmann, M.A. and Fischer, F.D.
ADVANCED ENGINEERING MATERIALS. Volume: 19 (2017) - 2017 • 4291
Electronic structure of metastable bcc Cu-Cr alloy thin films: Comparison of electron energy-loss spectroscopy and first-principles calculations
Liebscher, C.H. and Freysoldt, C. and Dennenwaldt, T. and Harzer, T.P. and Dehm, G.
ULTRAMICROSCOPY. Volume: 178 (2017) - 2017 • 4290
Stiff, light, strong and ductile: nano-structured High Modulus Steel
Springer, H. and Baron, C. and Szczepaniak, A. and Uhlenwinkel, V. and Raabe, D.
SCIENTIFIC REPORTS. Volume: 7 (2017) - 2017 • 4289
Designing duplex, ultrafine-grained Fe-Mn-Al-C steels by tuning phase transformation and recrystallization kinetics
Zhang, J. and Raabe, D. and Tasan, C.C.
ACTA MATERIALIA. Volume: 141 (2017) - 2017 • 4288
Collapsed tetragonal phase transition in LaRu2 P2
Drachuck, G. and Sapkota, A. and Jayasekara, W.T. and Kothapalli, K. and Bud'Ko, S.L. and Goldman, A.I. and Kreyssig, A. and Canfield, P.C.
PHYSICAL REVIEW B. Volume: 96 (2017) - 2017 • 4287
From the Precursor to the Active State: Monitoring Metamorphosis of Electrocatalysts During Water Oxidation by In Situ Spectroscopy
Hollmann, D. and Rockstroh, N. and Grabow, K. and Bentrup, U. and Rabeah, J. and Polyakov, M. and Surkus, A.-E. and Schuhmann, W. and Hoch, S. and Brückner, A.
CHEMELECTROCHEM. Volume: 4 (2017) - 2017 • 4286
Numerical Study on the Influence of Operational Settings on Refuse Derived Fuel Co-firing in Cement Rotary Kilns
Liedmann, B. and Wirtz, S. and Scherer, V. and Krüger, B.
ENERGY PROCEDIA. Volume: 120 (2017) - 2017 • 4285
High-Density Droplet Microarray of Individually Addressable Electrochemical Cells
Zhang, H. and Oellers, T. and Feng, W. and Abdulazim, T. and Saw, E.N. and Ludwig, Al. and Levkin, P.A. and Plumeré, N.
ANALYTICAL CHEMISTRY. Volume: 89 (2017) - 2017 • 4284
An approach to model the thermal conversion and flight behaviour of Refuse Derived Fuel
Liedmann, B. and Arnold, W. and Krüger, B. and Becker, A. and Krusch, S. and Wirtz, S. and Scherer, V.
FUEL. Volume: 200 (2017) - 2017 • 4283
Microcantilever Fracture Testing of Intermetallic Cu3Sn in Lead-Free Solder Interconnects
Philippi, B. and Matoy, K. and Zechner, J. and Kirchlechner, C. and Dehm, G.
JOURNAL OF ELECTRONIC MATERIALS. Volume: 46 (2017) - 2017 • 4282
Detection and Control of Spin-Orbit Interactions in a GaAs Hole Quantum Point Contact
Srinivasan, A. and Miserev, D.S. and Hudson, K.L. and Klochan, O. and Muraki, K. and Hirayama, Y. and Reuter, D. and Wieck, A.D. and Sushkov, O.P. and Hamilton, A.R.
PHYSICAL REVIEW LETTERS. Volume: 118 (2017) - 2017 • 4281
A novel versatile microbiosensor for local hydrogen detection by means of scanning photoelectrochemical microscopy
Zhao, F. and Conzuelo, F. and Hartmann, V. and Li, H. and Stapf, S. and Nowaczyk, M.M. and Rögner, M. and Plumeré, N. and Lubitz, W. and Schuhmann, W.
BIOSENSORS AND BIOELECTRONICS. Volume: 94 (2017) - 2017 • 4280
Kinetics and crystallization path of a Fe-based metallic glass alloy
Duarte, M.J. and Kostka, A. and Crespo, D. and Jimenez, J.A. and Dippel, A.-C. and Renner, F.U. and Dehm, G.
ACTA MATERIALIA. Volume: 127 (2017) - 2017 • 4279
Influence of organic surface chemistry on the nucleation of plasma deposited SiOx films
Hoppe, C. and Mitschker, F. and Giner, I. and De los Arcos, T. and Awakowicz, P. and Grundmeier, G.
JOURNAL OF PHYSICS D: APPLIED PHYSICS. Volume: 50 (2017) - 2017 • 4278
Comparison of pyrolysis test rigs for oxy-fuel conditions
Pielsticker, S. and Heuer, S. and Senneca, O. and Cerciello, F. and Salatino, P. and Cortese, L. and Gövert, B. and Hatzfeld, O. and Schiemann, M. and Scherer, V. and Kneer, R.
FUEL PROCESSING TECHNOLOGY. Volume: 156 (2017) - 2017 • 4277
A combined low-pressure hydrogen peroxide evaporation plus hydrogen plasma treatment method for sterilization – Part 1: Characterization of the condensation process and proof-of-concept
Stapelmann, K. and Fiebrandt, M. and Raguse, M. and Lackmann, J.-W. and Postema, M. and Moeller, R. and Awakowicz, P.
PLASMA PROCESSES AND POLYMERS. Volume: 14 (2017) - 2017 • 4276
Cu-exchanged Al-rich SSZ-13 zeolite from organotemplate-free synthesis as NH3-SCR catalyst: Effects of Na+ ions on the activity and hydrothermal stability
Zhao, Z. and Yu, R. and Zhao, R. and Shi, C. and Gies, H. and Xiao, F.-S. and De Vos, D. and Yokoi, T. and Bao, X. and Kolb, U. and Feyen, M. and McGuire, R. and Maurer, S. and Moini, A. and Müller, U. and Zhang, W.
APPLIED CATALYSIS B: ENVIRONMENTAL. Volume: 217 (2017) - 2017 • 4275
Adaptive grid semidefinite programming for finding optimal designs
Duarte, B.P.M. and Wong, W.K. and Dette, H.
STATISTICS AND COMPUTING. Volume: 28 (2017) - 2017 • 4274
Interrogation of a PS1-Based Photocathode by Means of Scanning Photoelectrochemical Microscopy
Zhao, F. and Plumeré, N. and Nowaczyk, M.M. and Ruff, A. and Schuhmann, W. and Conzuelo, F.
SMALL. Volume: 13 (2017) - 2017 • 4273
Investigations on polarization oscillation amplitudes in spin-VCSELs
Lindemann, M. and Pusch, T. and Michalzik, R. and Gerhardt, N.C. and Hofmann, M.R.
PROCEEDINGS OF SPIE - THE INTERNATIONAL SOCIETY FOR OPTICAL ENGINEERING. Volume: 10122 (2017) - 2017 • 4272
Numerical investigation of optical sorting using the discrete element method
Pieper, C. and Kruggel-Emden, H. and Wirtz, S. and Scherer, V. and Pfaff, F. and Noack, B. and Hanebeck, U.D. and Maier, G. and Gruna, R. and Längle, T. and Beyerer, J.
SPRINGER PROCEEDINGS IN PHYSICS. Volume: 188 (2017) - 2017 • 4271
Development of electrically conductive microstructures based on polymer/CNT nanocomposites via two-photon polymerization
Staudinger, U. and Zyla, G. and Krause, B. and Janke, A. and Fischer, D. and Esen, C. and Voit, B. and Ostendorf, A.
MICROELECTRONIC ENGINEERING. Volume: 179 (2017) - 2017 • 4270
A multiscale homogenization model for strength predictions of fully and partially frozen soils
Zhou, M.-M. and Meschke, G.
ACTA GEOTECHNICA. Volume: (2017) - 2017 • 4269
The influence of iron oxide on the oxidation kinetics of synthetic char derived from thermogravimetric analysis and fixed-bed experiments under isothermal and temperature-programmed conditions
Düdder, H. and Lotz, K. and Wütscher, A. and Muhler, M.
FUEL. Volume: 201 (2017) - 2017 • 4268
Femtosecond semiconductor laser system with resonator-internal dispersion adaptation
Pilny, R.H. and Döpke, B. and Balzer, J.C. and Brenner, C. and Klehr, A. and Knigge, A. and Tränkle, G. and Hofmann, M.R.
OPTICS LETTERS. Volume: 42 (2017) - 2017 • 4267
Efficient approach to compute melting properties fully from ab initio with application to Cu
Zhu, L.-F. and Grabowski, B. and Neugebauer, J.
PHYSICAL REVIEW B. Volume: 96 (2017) - 2017 • 4266
Ultrafast diode laser with self-adapting pulse-shaping in passive, active and hybrid mode-locking operation
Pilny, R.H. and Döpke, B. and Brenner, C. and Klehr, A. and Knigge, A. and Tränkle, G. and Hofmann, M.R.
OPTICS INFOBASE CONFERENCE PAPERS. Volume: Part F43-CLEO_AT 2017 (2017) - 2017 • 4265
A correlative investigation of grain boundary crystallography and electronic properties in CdTe thin film solar cells
Stechmann, G. and Zaefferer, S. and Schwarz, T. and Konijnenberg, P. and Raabe, D. and Gretener, C. and Kranz, L. and Perrenoud, J. and Buecheler, S. and Nath Tiwari, A.
SOLAR ENERGY MATERIALS AND SOLAR CELLS. Volume: 166 (2017) - 2017 • 4264
Intermediate Product Regulation in Tandem Solid Catalysts with Multimodal Porosity for High-Yield Synthetic Fuel Production
Duyckaerts, N. and Bartsch, M. and Trotuş, I.-T. and Pfänder, N. and Lorke, A. and Schüth, F. and Prieto, G.
ANGEWANDTE CHEMIE - INTERNATIONAL EDITION. Volume: 56 (2017) - 2017 • 4263
Preferential Carbon Monoxide Oxidation over Copper-Based Catalysts under In Situ Ball Milling
Eckert, R. and Felderhoff, M. and Schüth, F.
ANGEWANDTE CHEMIE - INTERNATIONAL EDITION. Volume: 56 (2017) - 2017 • 4262
Electronic properties of LaAlO3/SrTiO3 n-type interfaces: A GGA+U study
Piyanzina, I.I. and Kopp, T. and Lysogorskiy, Y.V. and Tayurskii, D.A. and Eyert, V.
JOURNAL OF PHYSICS CONDENSED MATTER. Volume: 29 (2017) - 2017 • 4261
Poly(benzoxazine) as an Immobilization Matrix for Miniaturized ATP and Glucose Biosensors
Ziller, C. and Lin, J. and Knittel, P. and Friedrich, L. and Andronescu, C. and Pöller, S. and Schuhmann, W. and Kranz, C.
CHEMELECTROCHEM. Volume: 4 (2017) - 2017 • 4260
Mesoscopic Field-Effect-Induced Devices in Depleted Two-Dimensional Electron Systems
Bachsoliani, N. and Platonov, S. and Wieck, A.D. and Ludwig, S.
PHYSICAL REVIEW APPLIED. Volume: 8 (2017) - 2017 • 4259
Multiscale inference for a multivariate density with applications to X-ray astronomy
Eckle, K. and Bissantz, N. and Dette, H. and Proksch, K. and Einecke, S.
ANNALS OF THE INSTITUTE OF STATISTICAL MATHEMATICS. Volume: (2017) - 2017 • 4258
Gold-Palladium Bimetallic Catalyst Stability: Consequences for Hydrogen Peroxide Selectivity
Pizzutilo, E. and Freakley, S.J. and Cherevko, S. and Venkatesan, S. and Hutchings, G.J. and Liebscher, C.H. and Dehm, G. and Mayrhofer, K.J.J.
ACS CATALYSIS. Volume: 7 (2017) - 2017 • 4257
Long-term thermal stability of nanoclusters in ODS-Eurofer steel: An atom probe tomography study
Zilnyk, K.D. and Pradeep, K.G. and Choi, P. and Sandim, H.R.Z. and Raabe, D.
JOURNAL OF NUCLEAR MATERIALS. Volume: 492 (2017) - 2017 • 4256
Residual stress depth distributions for atmospheric plasma sprayed MnCo1.9Fe0.1O4 spinel layers on crofer steel substrate
Back, H.C. and Mutter, M. and Gibmeier, J. and Mücke, R. and Vaßen, R.
MATERIALS SCIENCE FORUM. Volume: 905 MSF (2017) - 2017 • 4255
The Space Confinement Approach Using Hollow Graphitic Spheres to Unveil Activity and Stability of Pt-Co Nanocatalysts for PEMFC
Pizzutilo, E. and Knossalla, J. and Geiger, S. and Grote, J.-P. and Polymeros, G. and Baldizzone, C. and Mezzavilla, S. and Ledendecker, M. and Mingers, A. and Cherevko, S. and Schüth, F. and Mayrhofer, K.J.J.
ADVANCED ENERGY MATERIALS. Volume: 7 (2017) - 2017 • 4254
On the usability of low-cost inertial navigation systems for free-hand SAR imaging at GPR-frequencies
Baer, C. and Barowski, J. and Rolfes, I.
SAS 2017 - 2017 IEEE SENSORS APPLICATIONS SYMPOSIUM, PROCEEDINGS. Volume: (2017) - 2017 • 4253
Non-universal transmission phase behaviour of a large quantum dot
Edlbauer, H. and Takada, S. and Roussely, G. and Yamamoto, M. and Tarucha, S. and Ludwig, Ar. and Wieck, A.D. and Meunier, T. and Baüerle, C.
NATURE COMMUNICATIONS. Volume: 8 (2017) - 2017 • 4252
Dislocation activities at the martensite phase transformation interface in metastable austenitic stainless steel: An in-situ TEM study
Liu, J. and Chen, C. and Feng, Q. and Fang, X. and Wang, H. and Liu, F. and Lu, J. and Raabe, D.
MATERIALS SCIENCE AND ENGINEERING A. Volume: 703 (2017) - 2017 • 4251
Expediting combinatorial data set analysis by combining human and algorithmic analysis
Stein, H.S. and Jiao, S. and Ludwig, Al.
ACS COMBINATORIAL SCIENCE. Volume: 19 (2017) - 2017 • 4250
A new calibration method for the measurement of a multiport microwave tomography system with a 2-port vector network analyzer
Zimmermanns, M. and Rolfes, I. and Mallach, M. and Gebhardt, P. and Musch, T.
EUROPEAN MICROWAVE WEEK 2016: "MICROWAVES EVERYWHERE", EUMW 2016 - CONFERENCE PROCEEDINGS; 46TH EUROPEAN MICROWAVE CONFERENCE, EUMC 2016. Volume: (2017) - 2017 • 4249
Ground penetrating synthetic aperture radar imaging providing soil permittivity estimation
Baer, C. and Gutierrez, S. and Jebramcik, J. and Barowski, J. and Vega, F. and Rolfes, I.
IEEE MTT-S INTERNATIONAL MICROWAVE SYMPOSIUM DIGEST. Volume: (2017) - 2017 • 4248
Anisotropic layered Bi2 Te3 -In2 Te3 composites: Control of interface density for tuning of thermoelectric properties
Liu, D. and Li, X. and De Castro Borlido, P.M. and Botti, S. and Schmechel, R. and Rettenmayr, M.
SCIENTIFIC REPORTS. Volume: 7 (2017) - 2017 • 4247
Ab initio modelling of solute segregation energies to a general grain boundary
Huber, L. and Grabowski, B. and Militzer, M. and Neugebauer, J. and Rottler, J.
ACTA MATERIALIA. Volume: 132 (2017) - 2017 • 4246
Hybrid concrete elements with splitting fiber reinforcement under two-dimensional partial-area loading
Plückelmann, S. and Song, F. and Breitenbücher, R.
HIGH TECH CONCRETE: WHERE TECHNOLOGY AND ENGINEERING MEET - PROCEEDINGS OF THE 2017 FIB SYMPOSIUM. Volume: (2017) - 2017 • 4245
Quantum-Phase-Field Concept of Matter: Emergent Gravity in the Dynamic Universe
Steinbach, I.
ZEITSCHRIFT FUR NATURFORSCHUNG - SECTION A JOURNAL OF PHYSICAL SCIENCES. Volume: 72 (2017) - 2017 • 4244
Yb2Si2O7 Environmental Barrier Coatings Deposited by Various Thermal Spray Techniques: A Preliminary Comparative Study
Bakan, E. and Marcano, D. and Zhou, D. and Sohn, Y.J. and Mauer, G. and Vaßen, R.
JOURNAL OF THERMAL SPRAY TECHNOLOGY. Volume: 26 (2017) - 2017 • 4243
Electronic properties, low-energy Hamiltonian, and superconducting instabilities in CaKFe4As4
Lochner, F. and Ahn, F. and Hickel, T. and Eremin, I.
PHYSICAL REVIEW B. Volume: 96 (2017) - 2017 • 4242
Ceramic Top Coats of Plasma-Sprayed Thermal Barrier Coatings: Materials, Processes, and Properties
Bakan, E. and Vaßen, R.
JOURNAL OF THERMAL SPRAY TECHNOLOGY. Volume: 26 (2017) - 2017 • 4241
Modelling of grain boundary dynamics using amplitude equations
Hüter, C. and Neugebauer, J. and Boussinot, G. and Svendsen, B. and Prahl, U. and Spatschek, R.
CONTINUUM MECHANICS AND THERMODYNAMICS. Volume: 29 (2017) - 2017 • 4240
Narrow optical linewidths and spin pumping on charge-tunable close-to-surface self-assembled quantum dots in an ultrathin diode
Löbl, M.C. and Söllner, I. and Javadi, A. and Pregnolato, T. and Schott, R. and Midolo, L. and Kuhlmann, A.V. and Stobbe, Sø. and Wieck, A.D. and Lodahl, P. and Ludwig, Ar. and Warburton, R.J.
PHYSICAL REVIEW B. Volume: 96 (2017) - 2017 • 4239
Method for the quantification of rupture probability in soft collagenous tissues
Balzani, D. and Schmidt, T. and Ortiz, M.
INTERNATIONAL JOURNAL FOR NUMERICAL METHODS IN BIOMEDICAL ENGINEERING. Volume: 33 (2017) - 2017 • 4238
Data compression strategies for ptychographic diffraction imaging
Loetgering, L. and Rose, M. and Treffer, D. and Vartanyants, I.A. and Rosenhahn, A. and Wilhein, T.
ADVANCED OPTICAL TECHNOLOGIES. Volume: 6 (2017) - 2017 • 4237
A Unified Interdisciplinary Approach to Design Antibacterial Coatings for Fast Silver Release
El Arrassi, A. and Bellova, P. and Javid, S.M. and Motemani, Y. and Khare, C. and Sengstock, C. and Köller, M. and Ludwig, Al. and Tschulik, K.
CHEMELECTROCHEM. Volume: (2017) - 2017 • 4236
Correlating Atom Probe Tomography with Atomic-Resolved Scanning Transmission Electron Microscopy: Example of Segregation at Silicon Grain Boundaries
Stoffers, A. and Barthel, J. and Liebscher, C.H. and Gault, B. and Cojocaru-Mirédin, O. and Scheu, C. and Raabe, D.
MICROSCOPY AND MICROANALYSIS. Volume: (2017) - 2017 • 4235
Generation of bioinspired structural colors via two-photon polymerization
Zyla, G. and Kovalev, A. and Grafen, M. and Gurevich, E.L. and Esen, C. and Ostendorf, A. and Gorb, S.
SCIENTIFIC REPORTS. Volume: 7 (2017) - 2017 • 4234
Construction of statistically similar rves for the quantification of uncertainty associated with the material's microstructure morphology
Balzani, D. and Miska, N. and Pruger, S.
UNCECOMP 2017 - PROCEEDINGS OF THE 2ND INTERNATIONAL CONFERENCE ON UNCERTAINTY QUANTIFICATION IN COMPUTATIONAL SCIENCES AND ENGINEERING. Volume: 2017-January (2017) - 2017 • 4233
In situ measurement of VUV/UV radiation from low-pressure microwave-produced plasma in Ar/O2 gas mixtures
Iglesias, E.J. and Mitschker, F. and Fiebrandt, M. and Bibinov, N. and Awakowicz, P.
MEASUREMENT SCIENCE AND TECHNOLOGY. Volume: 28 (2017) - 2017 • 4232
A Polymer Multilayer Based Amperometric Biosensor for the Detection of Lactose in the Presence of High Concentrations of Glucose
Lopez, F. and Ma, S. and Ludwig, R. and Schuhmann, W. and Ruff, A.
ELECTROANALYSIS. Volume: 29 (2017) - 2017 • 4231
All-electrical measurement of the triplet-singlet spin relaxation time in self-assembled quantum dots
Eltrudis, K. and Al-Ashouri, A. and Beckel, A. and Ludwig, Ar. and Wieck, A.D. and Geller, M. and Lorke, A.
APPLIED PHYSICS LETTERS. Volume: 111 (2017) - 2017 • 4230
Cobalt boride modified with N-doped carbon nanotubes as a high-performance bifunctional oxygen electrocatalyst
Elumeeva, K. and Masa, J. and Medina, D. and Ventosa, E. and Seisel, S. and Kayran, Y.U. and Genç, A. and Bobrowski, T. and Weide, P. and Arbiol, J. and Muhler, M. and Schuhmann, W.
JOURNAL OF MATERIALS CHEMISTRY A. Volume: 5 (2017) - 2017 • 4229
Fermi-level pinning and intrinsic surface states of Al 1 - X in x N (10 1 - 0) surfaces
Portz, V. and Schnedler, M. and Lymperakis, L. and Neugebauer, J. and Eisele, H. and Carlin, J.-F. and Butté, R. and Grandjean, N. and Dunin-Borkowski, R.E. and Ebert, P.
APPLIED PHYSICS LETTERS. Volume: 110 (2017) - 2017 • 4228
Characterizing Dielectric Materials using Monostatic Transmission-and Reflection-Ellipsometry
Barowski, J. and Rolfes, I.
FREQUENZ. Volume: 71 (2017) - 2017 • 4227
Catalytic Oxidation of Soot Spray-Coated Lithium Zirconate in a Plate Reactor
Emmerich, T. and Lotz, K. and Sliozberg, K. and Schuhmann, W. and Muhler, M.
CHEMIE-INGENIEUR-TECHNIK. Volume: 89 (2017) - 2017 • 4226
Ultra-low charge and spin noise in self-assembled quantum dots
Ludwig, Ar. and Prechtel, J.H. and Kuhlmann, A.V. and Houel, J. and Valentin, S.R. and Warburton, R.J. and Wieck, A.D.
JOURNAL OF CRYSTAL GROWTH. Volume: 477 (2017) - 2017 • 4225
Probing Oxide Reduction and Phase Transformations at the Au-TiO2 Interface by Vibrational Spectroscopy
Pougin, A. and Lüken, A. and Klinkhammer, C. and Hiltrop, D. and Kauer, M. and Tölle, K. and Havenith-Newen, M. and Morgenstern, K. and Grünert, W. and Muhler, M. and Strunk, J.
TOPICS IN CATALYSIS. Volume: 60 (2017) - 2017 • 4224
Real-time imaging system for millimeter wave synthetic aperture radar sensors
Barowski, J. and Rolfes, I. and Baer, C.
2017 1ST IEEE MTT-S INTERNATIONAL MICROWAVE BIO CONFERENCE, IMBIOC 2017. Volume: (2017) - 2017 • 4223
Silicon surface modifications produced by non-equilibrium He, Ne and Kr plasma jets
Engelhardt, M. and Kartaschew, K. and Bibinov, N. and Havenith, M. and Awakowicz, P.
JOURNAL OF PHYSICS D-APPLIED PHYSICS. Volume: 50 (2017) - 2017 • 4222
The Influence of Water on the Performance of Molybdenum Carbide Catalysts in Hydrodeoxygenation Reactions: A Combined Theoretical and Experimental Study
Engelhardt, J. and Lyu, P. and Nachtigall, P. and Schüth, F. and García, Á.M.
CHEMCATCHEM. Volume: 9 (2017) - 2017 • 4221
Reply to the 'Comments on "dental lessons from past to present: Ultrastructure and composition of teeth from plesiosaurs, dinosaurs, extinct and recent sharks"' by H. Botella: Et al., RSC Adv., 2016, 6, 74384-74388
Luebke, A. and Loza, K. and Patnaik, R. and Enax, J. and Raabe, D. and Prymak, O. and Fabritius, H.-O. and Gaengler, P. and Epple, M.
RSC ADVANCES. Volume: 7 (2017) - 2017 • 4220
Modifications of aluminum film caused by micro-plasmoids and plasma spots in the effluent of an argon non-equilibrium plasma jet
Engelhardt, M. and Ries, S. and Hermanns, P. and Bibinov, N. and Awakowicz, P.
JOURNAL OF PHYSICS D: APPLIED PHYSICS. Volume: 50 (2017) - 2017 • 4219
Silicon surface modifications produced by non-equilibrium He, Ne and Kr plasma jets
Engelhardt, M. and Kartaschew, K. and Bibinov, N. and Havenith, M. and Awakowicz, P.
JOURNAL OF PHYSICS D: APPLIED PHYSICS. Volume: 50 (2017) - 2017 • 4218
Comparison of maraging steel micro- and nanostructure produced conventionally and by laser additive manufacturing
Jägle, E.A. and Sheng, Z. and Kürnsteiner, P. and Ocylok, S. and Weisheit, A. and Raabe, D.
MATERIALS. Volume: 10 (2017) - 2017 • 4217
Decoupling the Effects of High Crystallinity and Surface Area on the Photocatalytic Overall Water Splitting over β-Ga2O3 Nanoparticles by Chemical Vapor Synthesis
Lukic, S. and Menze, J. and Weide, P. and Busser, G.W. and Winterer, M. and Muhler, M.
CHEMSUSCHEM. Volume: 10 (2017) - 2017 • 4216
Observations of Surface Mode Influence on Plasma Uniformity in PIC/MCC Simulations of Large Capacitive Discharges
Eremin, D. and Brinkmann, R.P. and Mussenbrock, T.
PLASMA PROCESSES AND POLYMERS. Volume: 14 (2017) - 2017 • 4215
Hydrogen effects on microstructural evolution and passive film characteristics of a duplex stainless steel
Luo, H. and Li, Z. and Chen, Y.-H. and Ponge, D. and Rohwerder, M. and Raabe, D.
ELECTROCHEMISTRY COMMUNICATIONS. Volume: 79 (2017) - 2017 • 4214
Degradation in concrete structures due to cyclic loading and its effect on transport processes—Experiments and modeling
Przondziono, R. and Timothy, J.J. and Weise, F. and Krütt, E. and Breitenbücher, R. and Meschke, G. and Hofmann, M.
STRUCTURAL CONCRETE. Volume: 18 (2017) - 2017 • 4213
Polybenzoxazine-Derived N-doped Carbon as Matrix for Powder-Based Electrocatalysts
Barwe, S. and Andronescu, C. and Masa, J. and Ventosa, E. and Klink, S. and Genç, A. and Arbiol, J. and Schuhmann, W.
CHEMSUSCHEM. Volume: 10 (2017) - 2017 • 4212
Constitutive modeling of strain induced grain boundary migration via coupling crystal plasticity and phase-field methods
Jafari, M. and Jamshidian, M. and Ziaei-Rad, S. and Raabe, D. and Roters, F.
INTERNATIONAL JOURNAL OF PLASTICITY. Volume: 99 (2017) - 2017 • 4211
Hydrogen enhances strength and ductility of an equiatomic high-entropy alloy
Luo, H. and Li, Z. and Raabe, D.
SCIENTIFIC REPORTS. Volume: 7 (2017) - 2017 • 4210
Influence of Ni to Co ratio in mixed Co and Ni phosphides on their electrocatalytic oxygen evolution activity
Barwe, S. and Andronescu, C. and Vasile, E. and Masa, J. and Schuhmann, W.
ELECTROCHEMISTRY COMMUNICATIONS. Volume: 79 (2017) - 2017 • 4209
Adsorption and desorption of hydrogen at nonpolar GaN(1 1 00) surfaces: Kinetics and impact on surface vibrational and electronic properties
Lymperakis, L. and Neugebauer, J. and Himmerlich, M. and Krischok, S. and Rink, M. and Kröger, J. and Polyakov, V.M.
PHYSICAL REVIEW B. Volume: 95 (2017) - 2017 • 4208
Simple methods for the preparation of non-noble metal bulk-electrodes for electrocatalytic applications
Puring, K.J. and Piontek, S. and Smialkowski, M. and Burfeind, J. and Kaluza, S. and Doetsch, C. and Apfel, U.-P.
JOURNAL OF VISUALIZED EXPERIMENTS. Volume: 2017 (2017) - 2017 • 4207
Overcoming the Instability of Nanoparticle-Based Catalyst Films in Alkaline Electrolyzers by using Self-Assembling and Self-Healing Films
Barwe, S. and Masa, J. and Andronescu, C. and Mei, B. and Schuhmann, W. and Ventosa, E.
ANGEWANDTE CHEMIE - INTERNATIONAL EDITION. Volume: 56 (2017) - 2017 • 4206
Compositional fingerprint of soy sauces via hydrophobic surface interaction
Jakobi, V. and Salmen, P. and Paulus, M. and Tolan, M. and Rosenhahn, A.
FOOD CHEMISTRY. Volume: 218 (2017) - 2017 • 4205
Monolithic vertical-cavity surface-emitting laser with thermally tunable birefringence
Pusch, T. and La Tona, E. and Lindemann, M. and Gerhardt, N.C. and Hofmann, M.R. and Michalzik, R.
APPLIED PHYSICS LETTERS. Volume: 110 (2017) - 2017 • 4204
Irreversibility of dislocation motion under cyclic loading due to strain gradients
Stricker, M. and Weygand, D. and Gumbsch, P.
SCRIPTA MATERIALIA. Volume: 129 (2017) - 2017 • 4203
Alternating current-bipolar electrochemistry
Eßmann, V. and Clausmeyer, J. and Schuhmann, W.
ELECTROCHEMISTRY COMMUNICATIONS. Volume: 75 (2017) - 2017 • 4202
Amperometric Detection of dsDNA using an Acridine-Orange-Modified Glucose Oxidase
Jambrec, D. and Lammers, K. and Bobrowski, T. and Pöller, S. and Schuhmann, W. and Ruff, A.
CHEMPLUSCHEM. Volume: 82 (2017) - 2017 • 4201
In depth nano spectroscopic analysis on homogeneously switching double barrier memristive devices
Strobel, J. and Hansen, M. and Dirkmann, S. and Neelisetty, K.K. and Ziegler, M. and Haberfehlner, G. and Popescu, R. and Kothleitner, G. and Chakravadhanula, V.S.K. and Kübel, C. and Kohlstedt, H. and Mussenbrock, T. and Kienle, L.
JOURNAL OF APPLIED PHYSICS. Volume: 121 (2017) - 2017 • 4200
In Operando Investigation of Electrical Coupling of Photosystem 1 and Photosystem 2 by Means of Bipolar Electrochemistry
Eßmann, V. and Zhao, F. and Hartmann, V. and Nowaczyk, M.M. and Schuhmann, W. and Conzuelo, F.
ANALYTICAL CHEMISTRY. Volume: 89 (2017) - 2017 • 4199
Microstructure evolution in refill friction stir spot weld of a dissimilar Al–Mg alloy to Zn-coated steel
Suhuddin, U.F.H. and Fischer, V. and Kostka, A. and dos Santos, J.F.
SCIENCE AND TECHNOLOGY OF WELDING AND JOINING. Volume: (2017) - 2017 • 4198
Wireless light-emitting electrochemical rotors
Eßmann, V. and Voci, S. and Loget, G. and Sojic, N. and Schuhmann, W. and Kuhn, A.
JOURNAL OF PHYSICAL CHEMISTRY LETTERS. Volume: 8 (2017) - 2017 • 4197
Proton-Transfer Mechanisms at the Water-ZnO Interface: The Role of Presolvation
Quaranta, V. and Hellström, M. and Behler, J.
JOURNAL OF PHYSICAL CHEMISTRY LETTERS. Volume: 8 (2017) - 2017 • 4196
In situ Investigation of Magnetism in Metastable Phases of Levitated Fe83 B17 during Solidification in SITU INVESTIGATION of MAGNETISM in ... D. G. QUIRINALE et al.
Quirinale, D.G. and Messina, D. and Rustan, G.E. and Kreyssig, A. and Prozorov, R. and Goldman, A.I.
PHYSICAL REVIEW APPLIED. Volume: 8 (2017) - 2017 • 4195
Effects of Post Annealing Treatments on the Interfacial Chemical Properties and Band Alignment of AlN/Si Structure Prepared by Atomic Layer Deposition
Sun, L. and Lu, H. and Chen, H. and Wang, T. and Ji, X. and Liu, W. and Zhao, D. and Devi, A. and Ding, S. and Zhang, D. W.
NANOSCALE RESEARCH LETTERS. Volume: 12 (2017) - 2017 • 4194
CFD Simulation of a 100 MWth Lithium Combustion Slag Tap Furnace as a basis for an Energy Storage Process
Maas, P. and Schiemann, M. and Scherer, V. and Fischer, P. and Taroata, D. and Schmid, G.
ENERGY PROCEDIA. Volume: 105 (2017) - 2017 • 4193
The solidification of Al-Pd-Mn studied by high-energy X-ray diffraction from electrostatically levitated samples
Quirinale, D.G. and Kreyssig, A. and Saunders, S. and Messina, D. and Straszheim, W.E. and Canfield, P.C. and Kramer, M.J. and Goldman, A.I.
ZEITSCHRIFT FUR KRISTALLOGRAPHIE - CRYSTALLINE MATERIALS. Volume: 232 (2017) - 2017 • 4192
100 years public-private partnership in metallurgical and materials science research
Raabe, D. and Dehm, G. and Neugebauer, J. and Rohwerder, M.
MATERIALS TODAY. Volume: 20 (2017) - 2017 • 4191
Molecular Engineering of MnII Diamine Diketonate Precursors for the Vapor Deposition of Manganese Oxide Nanostructures
Maccato, C. and Bigiani, L. and Carraro, G. and Gasparotto, A. and Seraglia, R. and Kim, J. and Devi, A. and Tabacchi, G. and Fois, E. and Pace, G. and Di Noto, V. and Barreca, D.
CHEMISTRY - A EUROPEAN JOURNAL. Volume: 23 (2017) - 2017 • 4190
1 billion tons of nanostructure - Segregation engineering enables confined transformation effects at lattice defects in steels
Raabe, D. and Ponge, D. and Wang, M.-M. and Herbig, M. and Belde, M. and Springer, H.
IOP CONFERENCE SERIES: MATERIALS SCIENCE AND ENGINEERING. Volume: 219 (2017) - 2017 • 4189
Lifetime and failure modes of plasma sprayed thermal barrier coatings in thermal gradient rig tests with simultaneous CMAS injection
Mack, D.E. and Wobst, T. and Jarligo, M.O.D. and Sebold, D. and Vaßen, R.
SURFACE AND COATINGS TECHNOLOGY. Volume: 324 (2017) - 2017 • 4188
Cluster formation in stochastic disk systems
Sutmann, G. and Ganesan, H. and Begau, C.
AIP CONFERENCE PROCEEDINGS. Volume: 1863 (2017) - 2017 • 4187
Combinatorial study of Fe-Co-V hard magnetic thin films
Fackler, S.W. and Alexandrakis, V. and König, D. and Kusne, A.G. and Gao, T. and Kramer, M.J. and Stasak, D. and Lopez, K. and Zayac, B. and Mehta, A. and Ludwig, Al. and Takeuchi, I.
SCIENCE AND TECHNOLOGY OF ADVANCED MATERIALS. Volume: 18 (2017) - 2017 • 4186
Optimized growth and reorientation of anisotropic material based on evolution equations
Jantos, D.R. and Junker, P. and Hackl, K.
COMPUTATIONAL MECHANICS. Volume: (2017) - 2017 • 4185
Pulverized coal ignition testing under air-fired conditions using the Zelkowski method: Comparison of coals of different rank and provenience
Becker, A. and Schiemann, M. and Scherer, V. and Haxter, D. and Mayer, J.
ENERGY PROCEDIA. Volume: 120 (2017) - 2017 • 4184
The effect of UV radiation from oxygen and argon plasma on the adhesion of organosilicon coatings on polypropylene
Jaritz, M. and Behm, H. and Hopmann, C. and Kirchheim, D. and Mitschker, F. and Awakowicz, P. and Dahlmann, R.
JOURNAL OF PHYSICS D-APPLIED PHYSICS. Volume: 50 (2017) - 2017 • 4183
Comparative ignition tests of coal under oxy-fuel conditions in a standardized laboratory test rig
Becker, A. and Schiemann, M. and Scherer, V. and Shaddix, C. and Haxter, D. and Mayer, J.
FUEL. Volume: 208 (2017) - 2017 • 4182
Diffusion across the glass transition in silicate melts: Systematic correlations, new experimental data for Sr and Ba in calcium-aluminosilicate glasses and general mechanisms of ionic transport
Fanara, S. and Sengupta, P. and Becker, H.-W. and Rogalla, D. and Chakraborty, S.
JOURNAL OF NON-CRYSTALLINE SOLIDS. Volume: 455 (2017) - 2017 • 4181
Influence of residual stress on the adhesion and surface morphology of PECVD-coated polypropylene
Jaritz, M. and Hopmann, C. and Behm, H. and Kirchheim, D. and Wilski, S. and Grochla, D. and Banko, L. and Ludwig, Al. and Böke, M. and Winter, J. and Bahre, H. and Dahlmann, R.
JOURNAL OF PHYSICS D: APPLIED PHYSICS. Volume: 50 (2017) - 2017 • 4180
Mechanical size effects in a single crystalline equiatomic FeCrCoMnNi high entropy alloy
Raghavan, R. and Kirchlechner, C. and Jaya, B.N. and Feuerbacher, M. and Dehm, G.
SCRIPTA MATERIALIA. Volume: 129 (2017) - 2017 • 4179
Superelasticity and cryogenic linear shape memory effects of CaFe2As2
Sypek, J.T. and Yu, H. and Dusoe, K.J. and Drachuck, G. and Patel, H. and Giroux, A.M. and Goldman, A.I. and Kreyssig, A. and Canfield, P.C. and Bud'Ko, S.L. and Weinberger, C.R. and Lee, S.-W.
NATURE COMMUNICATIONS. Volume: 8 (2017) - 2017 • 4178
The effect of UV radiation from oxygen and argon plasma on the adhesion of organosilicon coatings on polypropylene
Jaritz, M. and Behm, H. and Hopmann, C. and Kirchheim, D. and Mitschker, F. and Awakowicz, P. and Dahlmann, R.
JOURNAL OF PHYSICS D: APPLIED PHYSICS. Volume: 50 (2017) - 2017 • 4177
Maintaining strength in supersaturated copper–chromium thin films annealed at 0.5 of the melting temperature of Cu
Raghavan, R. and Harzer, T.P. and Djaziri, S. and Hieke, S.W. and Kirchlechner, C. and Dehm, G.
JOURNAL OF MATERIALS SCIENCE. Volume: 52 (2017) - 2017 • 4176
Strengthening Fe – TiB2 based high modulus steels by precipitations
Szczepaniak, A. and Springer, H. and Aparicio-Fernández, R. and Baron, C. and Raabe, D.
MATERIALS AND DESIGN. Volume: 124 (2017) - 2017 • 4175
Positive centre voltage in T-branch junctions on n-type GaAs/AlGaAs based on hydrodynamics
Szelong, M. and Ludwig, Ar. and Wieck, A. and Kunze, U.
SEMICONDUCTOR SCIENCE AND TECHNOLOGY. Volume: 32 (2017) - 2017 • 4174
Resolved simulations of single char particle combustion in a laminar flow field
Farazi, S. and Sadr, M. and Kang, S. and Schiemann, M. and Vorobiev, N. and Scherer, V. and Pitsch, H.
FUEL. Volume: 201 (2017) - 2017 • 4173
Fracture behavior of nanostructured heavily cold drawn pearlitic steel wires before and after annealing
Jaya, B.N. and Goto, S. and Richter, G. and Kirchlechner, C. and Dehm, G.
MATERIALS SCIENCE AND ENGINEERING A. Volume: 707 (2017) - 2017 • 4172
Functional performance of Gd2Zr2O7/YSZ multi-layered thermal barrier coatings deposited by suspension plasma spray
Mahade, S. and Curry, N. and Björklund, S. and Markocsan, N. and Nylén, P. and Vaßen, R.
SURFACE AND COATINGS TECHNOLOGY. Volume: 318 (2017) - 2017 • 4171
Nanoporous Nitrogen-Doped Graphene Oxide/Nickel Sulfide Composite Sheets Derived from a Metal-Organic Framework as an Efficient Electrocatalyst for Hydrogen and Oxygen Evolution
Jayaramulu, K. and Masa, J. and Tomanec, O. and Peeters, D. and Ranc, V. and Schneemann, A. and Zboril, R. and Schuhmann, W. and Fischer, R.A.
ADVANCED FUNCTIONAL MATERIALS. Volume: (2017) - 2017 • 4170
Erosion Performance of Gadolinium Zirconate-Based Thermal Barrier Coatings Processed by Suspension Plasma Spray
Mahade, S. and Curry, N. and Björklund, S. and Markocsan, N. and Nylén, P. and Vaßen, R.
JOURNAL OF THERMAL SPRAY TECHNOLOGY. Volume: 26 (2017) - 2017 • 4169
Mesoscopic phase behavior in a quantum dot around crossover between single-level and multilevel transport regimes
Takada, S. and Yamamoto, M. and Bäuerle, C. and Ludwig, Ar. and Wieck, A.D. and Tarucha, S.
PHYSICAL REVIEW B. Volume: 95 (2017) - 2017 • 4168
Suppression of magnetic order in CaCo1.86As2 with Fe substitution: Magnetization, neutron diffraction, and x-ray diffraction studies of Ca(Co1-xFex)yAs2
Jayasekara, W.T. and Pandey, A. and Kreyssig, A. and Sangeetha, N.S. and Sapkota, A. and Kothapalli, K. and Anand, V.K. and Tian, W. and Vaknin, D. and Johnston, D.C. and McQueeney, R.J. and Goldman, A.I. and Ueland, B.G.
PHYSICAL REVIEW B. Volume: 95 (2017) - 2017 • 4167
Molecular dynamics simulations of entangled polymers: The effect of small molecules on the glass transition temperature
Mahmoudinezhad, E. and Marquardt, A. and Eggeler, G. and Varnik, F.
PROCEDIA COMPUTER SCIENCE. Volume: 108 (2017) - 2017 • 4166
Comparison of the quantitative analysis performance between pulsed voltage atom probe and pulsed laser atom probe
Takahashi, J. and Kawakami, K. and Raabe, D.
ULTRAMICROSCOPY. Volume: 175 (2017) - 2016 • 4165
Evaluation of kinetic constants on porous, non-noble catalyst layers for oxygen reduction - A comparative study between SECM and hydrodynamic methods
Dobrzeniecka, A. and Zeradjanin, A.R. and Masa, J. and Blicharska, M. and Wintrich, D. and Kulesza, P.J. and Schuhmann, W.
CATALYSIS TODAY. Volume: 262 (2016) - 2016 • 4164
Ultra-strong and damage tolerant metallic bulk materials: A lesson from nanostructured pearlitic steel wires
Hohenwarter, A. and Völker, B. and Kapp, M.W. and Li, Y. and Goto, S. and Raabe, D. and Pippan, R.
SCIENTIFIC REPORTS. Volume: 6 (2016) - 2016 • 4163
Self-supporting hierarchical porous PtAg alloy nanotubular aerogels as highly active and durable electrocatalysts
Liu, W. and Haubold, D. and Rutkowski, B. and Oschatz, M. and Hübner, R. and Werheid, M. and Ziegler, C. and Sonntag, L. and Liu, S. and Zheng, Z. and Herrmann, A.-K. and Geiger, D. and Terlan, B. and Gemming, T. and Borchardt, L. and Kaskel, S. and Czyrska-Filemonowicz, A. and Eychmüller, A.
CHEMISTRY OF MATERIALS. Volume: 28 (2016) - 2016 • 4162
Simulation-based evaluation of predictive tracking for sorting bulk materials
Pfaff, F. and Pieper, C. and Maier, G. and Noack, B. and Kruggel-Emden, H. and Gruna, R. and Hanebeck, U.D. and Wirtz, S. and Scherer, V. and Langle, T. and Beyerer, J.
IEEE INTERNATIONAL CONFERENCE ON MULTISENSOR FUSION AND INTEGRATION FOR INTELLIGENT SYSTEMS. Volume: 0 (2016) - 2016 • 4161
New materials for the light-induced hydrogen evolution reaction from the Cu-Si-Ti-O system
Stein, H.S. and Gutkowski, R. and Siegel, A. and Schuhmann, W. and Ludwig, Al.
JOURNAL OF MATERIALS CHEMISTRY A. Volume: 4 (2016) - 2016 • 4160
Influence of pore dimension on the host-guest interaction in metal-organic frameworks
Amirjalayer, S. and Schmid, R.
JOURNAL OF PHYSICAL CHEMISTRY C. Volume: 120 (2016) - 2016 • 4159
The Electrochemical Characterization of Single Core-Shell Nanoparticles
Holt, L.R. and Plowman, B.J. and Young, N.P. and Tschulik, K. and Compton, R.G.
ANGEWANDTE CHEMIE - INTERNATIONAL EDITION. Volume: 55 (2016) - 2016 • 4158
Performance improvements of polydisperse DEM simulations using a Loose Octree approach
Stein, G. and Wirtz, S. and Scherer, V.
ADVANCES IN PARALLEL COMPUTING. Volume: 27 (2016) - 2016 • 4157
PKa at Quartz/Electrolyte Interfaces
Pfeiffer-Laplaud, M. and Gaigeot, M.-P. and Sulpizi, M.
JOURNAL OF PHYSICAL CHEMISTRY LETTERS. Volume: 7 (2016) - 2016 • 4156
Timing jitter performance of mode-locked external cavity multi-quantum-well semiconductor lasers
Döpke, B. and Pilny, R.H. and Horstkemper, H. and Brenner, C. and Klehr, A. and Erbert, G. and Tränkle, G. and Hofmann, M.R.
PROCEEDINGS OF SPIE - THE INTERNATIONAL SOCIETY FOR OPTICAL ENGINEERING. Volume: 9767 (2016) - 2016 • 4155
Atom probe tomography of metallic nanostructures
Hono, K. and Raabe, D. and Ringer, S.P. and Seidman, D.N.
MRS BULLETIN. Volume: 41 (2016) - 2016 • 4154
Fe-Mg interdiffusion in orthopyroxene
Dohmen, R. and Ter Heege, J. H. and Becker, H. W. and Chakraborty, S.
AMERICAN MINERALOGIST. Volume: 101 (2016) - 2016 • 4153
Throughput optimization for laser micro structuring
Hoppius, J.S. and Kanitz, A. and Gurevich, E.L. and Ostendorf, A.
PROCEEDINGS OF SPIE - THE INTERNATIONAL SOCIETY FOR OPTICAL ENGINEERING. Volume: 9736 (2016) - 2016 • 4152
An Icosahedral Quasicrystal and Its 1/0 Crystalline Approximant in the Ca-Au-Al System
Pham, J. and Kreyssig, A. and Goldman, A.I. and Miller, G.J.
INORGANIC CHEMISTRY. Volume: 55 (2016) - 2016 • 4151
Quaternary Al-Cu-Mg-Si Q Phase: Sample Preparation, Heat Capacity Measurement and First-Principles Calculations
Löffler, A. and Zendegani, A. and Gröbner, J. and Hampl, M. and Schmid-Fetzer, R. and Engelhardt, H. and Rettenmayr, M. and Körmann, F. and Hickel, T. and Neugebauer, J.
JOURNAL OF PHASE EQUILIBRIA AND DIFFUSION. Volume: 37 (2016) - 2016 • 4150
Fracture toughness of intermetallic Cu6Sn5 in lead-free solder microelectronics
Philippi, B. and Matoy, K. and Zechner, J. and Kirchlechner, C. and Dehm, G.
SCRIPTA MATERIALIA. Volume: 123 (2016) - 2016 • 4149
CO Hydrogenation to Higher Alcohols over Cu–Co-Based Catalysts Derived from Hydrotalcite-Type Precursors
Anton, J. and Nebel, J. and Göbel, C. and Gabrysch, T. and Song, H. and Froese, C. and Ruland, H. and Muhler, M. and Kaluza, S.
TOPICS IN CATALYSIS. Volume: 59 (2016) - 2016 • 4148
Palladium Nanoparticles Supported on Nitrogen-Doped Carbon Nanotubes as a Release-and-Catch Catalytic System in Aerobic Liquid-Phase Ethanol Oxidation
Dong, W. and Chen, P. and Xia, W. and Weide, P. and Ruland, H. and Kostka, A. and Köhler, K. and Muhler, M.
CHEMCATCHEM. Volume: 8 (2016) - 2016 • 4147
Size and orientation dependent mechanical behavior of body-centered tetragonal Sn at 0.6 of the melting temperature
Philippi, B. and Kirchlechner, C. and Micha, J.S. and Dehm, G.
ACTA MATERIALIA. Volume: 115 (2016) - 2016 • 4146
The effect of sodium on the structure-activity relationships of cobalt-modified Cu/ZnO/Al2O3 catalysts applied in the hydrogenation of carbon monoxide to higher alcohols
Anton, J. and Nebel, J. and Song, H. and Froese, C. and Weide, P. and Ruland, H. and Muhler, M. and Kaluza, S.
JOURNAL OF CATALYSIS. Volume: 335 (2016) - 2016 • 4145
Magnetization and transport properties of single crystalline RPd2P2 (R=Y, La–Nd, Sm–Ho, Yb)
Drachuck, G. and Böhmer, A.E. and Bud'ko, S.L. and Canfield, P.C.
JOURNAL OF MAGNETISM AND MAGNETIC MATERIALS. Volume: 417 (2016) - 2016 • 4144
In-situ metal matrix composite steels: Effect of alloying and annealing on morphology, structure and mechanical properties of TiB2 particle containing high modulus steels
Aparicio-Fernández, R. and Springer, H. and Szczepaniak, A. and Zhang, H. and Raabe, D.
ACTA MATERIALIA. Volume: 107 (2016) - 2016 • 4143
The thermal stability of topologically close-packed phases in the single-crystal Ni-base superalloy ERBO/1
Lopez-Galilea, I. and Koßmann, J. and Kostka, A. and Drautz, R. and Mujica Roncery, L. and Hammerschmidt, T. and Huth, S. and Theisen, W.
JOURNAL OF MATERIALS SCIENCE. Volume: 51 (2016) - 2016 • 4142
Numerical Investigation of Third-Body Behavior in Dry and Wet Environments under Plane Shearing
Pieper, C. and Oschmann, T. and Markauskas, D. and Kempf, A. and Fischer, A. and Kruggel-Emden, H.
CHEMICAL ENGINEERING AND TECHNOLOGY. Volume: 39 (2016) - 2016 • 4141
Changes in volume during the four months’ remodelling period of iliac crest grafts in reconstruction of the alveolar ridge
Dreiseidler, T. and Kaunisaho, V. and Neugebauer, J. and Zöller, J.E. and Rothamel, D. and Kreppel, M.
BRITISH JOURNAL OF ORAL AND MAXILLOFACIAL SURGERY. Volume: 54 (2016) - 2016 • 4140
Numerical modeling of an automated optical belt sorter using the Discrete Element Method
Pieper, C. and Maier, G. and Pfaff, F. and Kruggel-Emden, H. and Wirtz, S. and Gruna, R. and Noack, B. and Scherer, V. and Längle, T. and Beyerer, J. and Hanebeck, U.D.
POWDER TECHNOLOGY. Volume: 301 (2016) - 2016 • 4139
Detection of damage to reinforced-concrete structures using piezoelectric smart aggregates
Stojić, D. and Nestorović, T. and Marković, N. and Cvetković, R. and Stojić, N.
GRADJEVINAR. Volume: 68 (2016) - 2016 • 4138
Finite element model updating using simulated annealing hybridized with unscented Kalman filter
Astroza, R. and Nguyen, L.T. and Nestorović, T.
COMPUTERS AND STRUCTURES. Volume: 177 (2016) - 2016 • 4137
From electronic structure to phase diagrams: A bottom-up approach to understand the stability of titanium–transition metal alloys
Huang, L.-F. and Grabowski, B. and Zhang, J. and Lai, M.-J. and Tasan, C.C. and Sandlöbes, S. and Raabe, D. and Neugebauer, J.
ACTA MATERIALIA. Volume: 113 (2016) - 2016 • 4136
A QM/MM approach for low-symmetry defects in metals
Huber, L. and Grabowski, B. and Militzer, M. and Neugebauer, J. and Rottler, J.
COMPUTATIONAL MATERIALS SCIENCE. Volume: 118 (2016) - 2016 • 4135
Oxidation characteristics of a cellulose-derived hydrochar in thermogravimetric and laminar flow burner experiments
Düdder, H. and Wütscher, A. and Vorobiev, N. and Schiemann, M. and Scherer, V. and Muhler, M.
FUEL PROCESSING TECHNOLOGY. Volume: 148 (2016) - 2016 • 4134
Nonlinear elastic effects in phase field crystal and amplitude equations: Comparison to ab initio simulations of bcc metals and graphene
Hüter, C. and Friák, M. and Weikamp, M. and Neugebauer, J. and Goldenfeld, N. and Svendsen, B. and Spatschek, R.
PHYSICAL REVIEW B - CONDENSED MATTER AND MATERIALS PHYSICS. Volume: 93 (2016) - 2016 • 4133
Interaction of phase and amplitude shaping in an external cavity semiconductor laser
Pilny, R.H. and Döpke, B. and Balzer, J.C. and Brenner, C. and Klehr, A. and Erbert, G. and Tränkle, G. and Hofmann, M.R.
PROCEEDINGS OF SPIE - THE INTERNATIONAL SOCIETY FOR OPTICAL ENGINEERING. Volume: 9767 (2016) - 2016 • 4132
Synthesis and characterization of lignite-like fuels obtained by hydrothermal carbonization of cellulose
Düdder, H. and Wütscher, A. and Stoll, R. and Muhler, M.
FUEL. Volume: 171 (2016) - 2016 • 4131
Barium sulfate micro- and nanoparticles as bioinert reference material in particle toxicology
Loza, K. and Föhring, I. and Bünger, J. and Westphal, G.A. and Köller, M. and Epple, M. and Sengstock, C.
NANOTOXICOLOGY. Volume: 10 (2016) - 2016 • 4130
Wiring of the aldehyde oxidoreductase PaoABC to electrode surfaces via entrapment in low potential phenothiazine-modified redox polymers
Pinyou, P. and Ruff, A. and Pöller, S. and Alsaoub, S. and Leimkühler, S. and Wollenberger, U. and Schuhmann, W.
BIOELECTROCHEMISTRY. Volume: 109 (2016) - 2016 • 4129
The Impact of Mesopores on the Mechanical Stability of HKUST-1: A Multiscale Investigation
Dürholt, J.P. and Keupp, J. and Schmid, R.
EUROPEAN JOURNAL OF INORGANIC CHEMISTRY. Volume: 2016 (2016) - 2016 • 4128
Precise synthesis of discrete and dispersible carbon-protected magnetic nanoparticles for efficient magnetic resonance imaging and photothermal therapy
Lu, A.-H. and Zhang, X.-Q. and Sun, Q. and Zhang, Y. and Song, Q. and Schüth, F. and Chen, C. and Cheng, F.
NANO RESEARCH. Volume: 9 (2016) - 2016 • 4127
Design of an Os Complex-Modified Hydrogel with Optimized Redox Potential for Biosensors and Biofuel Cells
Pinyou, P. and Ruff, A. and Pöller, S. and Ma, S. and Ludwig, R. and Schuhmann, W.
CHEMISTRY - A EUROPEAN JOURNAL. Volume: 22 (2016) - 2016 • 4126
Coarse graining of force fields for metal-organic frameworks
Dürholt, J.P. and Galvelis, R. and Schmid, R.
DALTON TRANSACTIONS. Volume: 45 (2016) - 2016 • 4125
Nano fabricated silicon nanorod array with titanium nitride coating for on-chip supercapacitors
Lu, P. and Ohlckers, P. and Müller, L. and Leopold, S. and Hoffmann, M. and Grigoras, K. and Ahopelto, J. and Prunnila, M. and Chen, X.
ELECTROCHEMISTRY COMMUNICATIONS. Volume: 70 (2016) - 2016 • 4124
Thermoresponsive amperometric glucose biosensor
Pinyou, P. and Ruff, A. and Pöller, S. and Barwe, S. and Nebel, M. and Alburquerque, N.G. and Wischerhoff, E. and Laschewsky, A. and Schmaderer, S. and Szeponik, J. and Plumeré, N. and Schuhmann, W.
BIOINTERPHASES. Volume: 11 (2016) - 2016 • 4123
Collective Atomic Displacements during Complex Phase Boundary Migration in Solid-Solid Phase Transformations
Duncan, J. and Harjunmaa, A. and Terrell, R. and Drautz, R. and Henkelman, G. and Rogal, J.
PHYSICAL REVIEW LETTERS. Volume: 116 (2016) - 2016 • 4122
Mode specific dynamics in the H2 + SH → H + H2S reaction
Lu, D. and Qi, J. and Yang, M. and Behler, J. and Song, H. and Li, J.
PHYSICAL CHEMISTRY CHEMICAL PHYSICS. Volume: 18 (2016) - 2016 • 4121
Analysis of Electronic and Structural Properties of Surfaces and Interfaces Based on LaAlO 3 and SrTiO 3
Piyanzina, I.I. and Lysogorskiy, Y.V. and Varlamova, I.I. and Kiiamov, A.G. and Kopp, T. and Eyert, V. and Nedopekin, O.V. and Tayurskii, D.A.
JOURNAL OF LOW TEMPERATURE PHYSICS. Volume: 185 (2016) - 2016 • 4120
Interface effects on the magnetic properties of layered Ni2MnGa/Ni2MnSn alloys: A first-principles investigation
Dutta, B. and Opahle, I. and Hickel, T.
FUNCTIONAL MATERIALS LETTERS. Volume: 9 (2016) - 2016 • 4119
Composites of fluoroapatite and methylmethacrylate-based polymers (PMMA) for biomimetic tooth replacement
Lübke, A. and Enax, J. and Wey, K. and Fabritius, H.-O. and Raabe, D. and Epple, M.
BIOINSPIRATION AND BIOMIMETICS. Volume: 11 (2016) - 2016 • 4118
Ab initio Prediction of Martensitic and Intermartensitic Phase Boundaries in Ni-Mn-Ga
Dutta, B. and Çaklr, A. and Giacobbe, C. and Al-Zubi, A. and Hickel, T. and Acet, M. and Neugebauer, J.
PHYSICAL REVIEW LETTERS. Volume: 116 (2016) - 2016 • 4117
In Situ Hydrocracking of Fischer-Tropsch Hydrocarbons: CO-Prompted Diverging Reaction Pathways for Paraffin and α-Olefin Primary Products
Duyckaerts, N. and Trotuş, I.-T. and Swertz, A.-C. and Schüth, F. and Prieto, G.
ACS CATALYSIS. Volume: 6 (2016) - 2016 • 4116
Intercalation Compounds as Inner Reference Electrodes for Reproducible and Robust Solid-Contact Ion-Selective Electrodes
Ishige, Y. and Klink, S. and Schuhmann, W.
ANGEWANDTE CHEMIE - INTERNATIONAL EDITION. Volume: 55 (2016) - 2016 • 4115
The structure and dynamics of chitin nanofibrils in an aqueous environment revealed by molecular dynamics simulations
Strelcova, Z. and Kulhanek, P. and Friak, M. and Fabritius, H. O. and Petrov, M. and Neugebauer, J. and Koca, J.
RSC ADVANCES. Volume: 6 (2016) - 2016 • 4114
Back-Illuminated Si-Based Photoanode with Nickel Cobalt Oxide Catalytic Protection Layer
Bae, D. and Mei, B. and Frydendal, R. and Pedersen, T. and Seger, B. and Hansen, O. and Vesborg, P.C.K. and Chorkendorff, I.
CHEMELECTROCHEM. Volume: 3 (2016) - 2016 • 4113
Tailoring Mixed-Halide, Wide-Gap Perovskites via Multistep Conversion Process
Bae, D. and Palmstrom, A. and Roelofs, K. and Mei, B. and Chorkendorff, I. and Bent, S.F. and Vesborg, P.C.K.
ACS APPLIED MATERIALS AND INTERFACES. Volume: 8 (2016) - 2016 • 4112
Silver-doped zinc oxide single nanowire multifunctional nanosensor with a significant enhancement in response
Lupan, O. and Cretu, V. and Postica, V. and Ahmadi, M. and Cuenya, B.R. and Chow, L. and Tiginyanu, I. and Viana, B. and Pauporté, T. and Adelung, R.
SENSORS AND ACTUATORS, B: CHEMICAL. Volume: 223 (2016) - 2016 • 4111
A polarimetrie, low ringing UWB antenna for ground penetrating radar operation
Baer, C. and Musch, T. and Schulz, C. and Rolfes, I.
2016 IEEE ANTENNAS AND PROPAGATION SOCIETY INTERNATIONAL SYMPOSIUM, APSURSI 2016 - PROCEEDINGS. Volume: (2016) - 2016 • 4110
On slip transmission and grain boundary yielding
Stricker, M. and Gagel, J. and Schmitt, S. and Schulz, K. and Weygand, D. and Gumbsch, P.
MECCANICA. Volume: 51 (2016) - 2016 • 4109
Analysis of composite materials with periodically aligned inclusions using 3D em field simulations
Baer, C. and Hattenhorst, B. and Schulz, C. and Will, B. and Rolfes, I. and Musch, T.
GEMIC 2016 - 2016 GERMAN MICROWAVE CONFERENCE. Volume: (2016) - 2016 • 4108
Origin of electron disproportionation in metallic sodium cobaltates
Lysogorskiy, Y.V. and Krivenko, S.A. and Mukhamedshin, I.R. and Nedopekin, O.V. and Tayurskii, D.A.
PHYSICAL REVIEW B. Volume: 94 (2016) - 2016 • 4107
Initial steps in reactions of aquathermolysis of cyclohexyl phenyl sulfide by means of ab initio calculations
Lysogorskiy, Y.V. and Aminova, R.M. and Tayurskii, D.A.
COMPUTATIONAL AND THEORETICAL CHEMISTRY. Volume: 1078 (2016) - 2016 • 4106
Demonstrating the steady performance of iron oxide composites over 2000 cycles at fast charge-rates for Li-ion batteries
Sun, Z. and Madej, E. and Genç, A. and Muhler, M. and Arbiol, J. and Schuhmann, W. and Ventosa, E.
CHEMICAL COMMUNICATIONS. Volume: 52 (2016) - 2016 • 4105
High temperature stability study of carbon supported high surface area catalysts—Expanding the boundaries of ex-situ diagnostics
Polymeros, G. and Baldizzone, C. and Geiger, S. and Grote, J.P. and Knossalla, J. and Mezzavilla, S. and Keeley, G.P. and Cherevko, S. and Zeradjanin, A.R. and Schüth, F. and Mayrhofer, K.J.J.
ELECTROCHIMICA ACTA. Volume: 211 (2016) - 2016 • 4104
The OpenCalphad thermodynamic software interface
Sundman, B. and Kattner, U.R. and Sigli, C. and Stratmann, M. and Le Tellier, R. and Palumbo, M. and Fries, S.G.
COMPUTATIONAL MATERIALS SCIENCE. Volume: 125 (2016) - 2016 • 4103
Positive and negative electrocaloric effect in BaTiO3 in the presence of defect dipoles
Ma, Y.-B. and Grünebohm, A. and Meyer, K.-C. and Albe, K. and Xu, B.-X.
PHYSICAL REVIEW B - CONDENSED MATTER AND MATERIALS PHYSICS. Volume: 94 (2016) - 2016 • 4102
Green's function enriched Poisson solver for electrostatics in many-particle systems
Sutmann, G.
AIP CONFERENCE PROCEEDINGS. Volume: 1738 (2016) - 2016 • 4101
Discrete element investigation of process models for batch screening under altered operational conditions
Elskamp, F. and Kruggel-Emden, H. and Hennig, M. and Teipel, U.
POWDER TECHNOLOGY. Volume: 301 (2016) - 2016 • 4100
A crystal plasticity smooth-particle hydrodynamics approach and its application to equal-channel angular pressing simulation
Ma, A. and Hartmaier, A.
MODELLING AND SIMULATION IN MATERIALS SCIENCE AND ENGINEERING. Volume: 24 (2016) - 2016 • 4099
Elemental partitioning, lattice misfit and creep behaviour of Cr containing gammaprime strengthened Co base superalloys
Povstugar, I. and Zenk, C.H. and Li, R. and Choi, P.-P. and Neumeier, S. and Dolotko, O. and Hoelzel, M. and Göken, M. and Raabe, D.
MATERIALS SCIENCE AND TECHNOLOGY (UNITED KINGDOM). Volume: 32 (2016) - 2016 • 4098
Perovskite-based bifunctional electrocatalysts for oxygen evolution and oxygen reduction in alkaline electrolytes
Elumeeva, K. and Masa, J. and Sierau, J. and Tietz, F. and Muhler, M. and Schuhmann, W.
ELECTROCHIMICA ACTA. Volume: 208 (2016) - 2016 • 4097
Crystal plasticity study of monocrystalline stochastic honeycombs under in-plane compression
Ma, D. and Eisenlohr, P. and Epler, E. and Volkert, C.A. and Shanthraj, P. and Diehl, M. and Roters, F. and Raabe, D.
ACTA MATERIALIA. Volume: 103 (2016) - 2016 • 4096
Coherent Control of the Exciton-Biexciton System in an InAs Self-Assembled Quantum Dot Ensemble
Suzuki, T. and Singh, R. and Bayer, M. and Ludwig, Ar. and Wieck, A.D. and Cundiff, S.T.
PHYSICAL REVIEW LETTERS. Volume: 117 (2016) - 2016 • 4095
A Simple Approach towards High-Performance Perovskite-Based Bifunctional Oxygen Electrocatalysts
Elumeeva, K. and Masa, J. and Tietz, F. and Yang, F. and Xia, W. and Muhler, M. and Schuhmann, W.
CHEMELECTROCHEM. Volume: 3 (2016) - 2016 • 4094
Nitrogen-Doped Hollow Amorphous Carbon Spheres@Graphitic Shells Derived from Pitch: New Structure Leads to Robust Lithium Storage
Ma, Q. and Wang, L. and Xia, W. and Jia, D. and Zhao, Z.
CHEMISTRY - A EUROPEAN JOURNAL. Volume: 22 (2016) - 2016 • 4093
Decoupling a hole spin qubit from the nuclear spins
Prechtel, J.H. and Kuhlmann, A.V. and Houel, J. and Ludwig, Ar. and Valentin, S.R. and Wieck, A.D. and Warburton, R.J.
NATURE MATERIALS. Volume: 15 (2016) - 2016 • 4092
Energetic and economic evaluation of membrane-based carbon capture routes for power plant processes
Maas, P. and Nauels, N. and Zhao, L. and Markewitz, P. and Scherer, V. and Modigell, M. and Stolten, D. and Hake, J.-F.
INTERNATIONAL JOURNAL OF GREENHOUSE GAS CONTROL. Volume: 44 (2016) - 2016 • 4091
Sterilization of beehive material with a double inductively coupled low pressure plasma
Priehn, M. and Denis, B. and Aumeier, P. and Kirchner, W.H. and Awakowicz, P. and Leichert, L.I.
JOURNAL OF PHYSICS D: APPLIED PHYSICS. Volume: 49 (2016) - 2016 • 4090
Numerical modeling of fluid–structure interaction in arteries with anisotropic polyconvex hyperelastic and anisotropic viscoelastic material models at finite strains
Balzani, D. and Deparis, S. and Fausten, S. and Forti, D. and Heinlein, A. and Klawonn, A. and Quarteroni, A. and Rheinbach, O. and Schröder, J.
INTERNATIONAL JOURNAL FOR NUMERICAL METHODS IN BIOMEDICAL ENGINEERING. Volume: 32 (2016) - 2016 • 4089
Precipitation Reactions in Age-Hardenable Alloys During Laser Additive Manufacturing
Jägle, E.A. and Sheng, Z. and Wu, L. and Lu, L. and Risse, J. and Weisheit, A. and Raabe, D.
JOM. Volume: 68 (2016) - 2016 • 4088
Hollow Nano- and Microstructures as Catalysts
Prieto, G. and Tüysüz, H. and Duyckaerts, N. and Knossalla, J. and Wang, G.-H. and Schüth, F.
CHEMICAL REVIEWS. Volume: 116 (2016) - 2016 • 4087
One-way and fully-coupled FE2 methods for heterogeneous elasticity and plasticity problems: Parallel scalability and an application to thermo-elastoplasticity of dual-phase steels
Balzani, D. and Gandhi, A. and Klawonn, A. and Lanser, M. and Rheinbach, O. and Schröder, J.
LECTURE NOTES IN COMPUTATIONAL SCIENCE AND ENGINEERING. Volume: 113 (2016) - 2016 • 4086
Recognition of pharmaceuticals with compact mini-Raman-spectrometer and automized pattern recognition algorithms
Jähme, H. and Di Florio, G. and Conti Nibali, V. and Esen, C. and Ostendorf, A. and Grafen, M. and Henke, E. and Soetebier, J. and Brenner, C. and Havenith, M. and Hofmann, M.R.
PROCEEDINGS OF SPIE - THE INTERNATIONAL SOCIETY FOR OPTICAL ENGINEERING. Volume: 9899 (2016) - 2016 • 4085
Interaction of an argon plasma jet with a silicon wafer
Engelhardt, M. and Pothiraja, R. and Kartaschew, K. and Bibinov, N. and Havenith, M. and Awakowicz, P.
JOURNAL OF PHYSICS D: APPLIED PHYSICS. Volume: 49 (2016) - 2016 • 4084
Structural and optical properties of (1122) InGaN quantum wells compared to (0001) and (1120)
Pristovsek, M. and Han, Y. and Zhu, T. and Oehler, F. and Tang, F. and Oliver, R.A. and Humphreys, C.J. and Tytko, D. and Choi, P.-P. and Raabe, D. and Brunner, F. and Weyers, M.
SEMICONDUCTOR SCIENCE AND TECHNOLOGY. Volume: 31 (2016) - 2016 • 4083
Development of carbon fibre-reinforced plastic (CFRP) crash absorbers with stable crushing behaviour considering the connection to the bumper system
Szlosarek, R. and Bombis, F. and Mühler, M. and Kröger, M. and Karall, T.
MATERIALWISSENSCHAFT UND WERKSTOFFTECHNIK. Volume: 47 (2016) - 2016 • 4082
Modeling of Microstructure Evolution with Dynamic Recrystallization in Finite Element Simulations of Martensitic Steel
Baron, T.J. and Khlopkov, K. and Pretorius, T. and Balzani, D. and Brands, D. and Schröder, J.
STEEL RESEARCH INTERNATIONAL. Volume: 87 (2016) - 2016 • 4081
Micro-plasmoids in self organized filamentary dielectric barrier discharges
Engelhardt, M. and Kogelheide, F. and Stapelmann, K. and Bibinov, N. and Awakowicz, P.
PLASMA PROCESSES AND POLYMERS. Volume: 14 (2016) - 2016 • 4080
Combinatorial screening of the microstructure–property relationships for Fe–B–X stiff, light, strong and ductile steels
Baron, C. and Springer, H. and Raabe, D.
MATERIALS AND DESIGN. Volume: 112 (2016) - 2016 • 4079
Modeling of low-alloyed trip-steels based on direct micro-macro simulations
Prüger, S. and Gandhi, A. and Balzani, D.
ECCOMAS CONGRESS 2016 - PROCEEDINGS OF THE 7TH EUROPEAN CONGRESS ON COMPUTATIONAL METHODS IN APPLIED SCIENCES AND ENGINEERING. Volume: 2 (2016) - 2016 • 4078
Low-temperature behavior of transmission phase shift across a Kondo correlated quantum dot
Takada, S. and Yamamoto, M. and Bäuerle, C. and Alex, A. and Von Delft, J. and Ludwig, Ar. and Wieck, A.D. and Tarucha, S.
PHYSICAL REVIEW B - CONDENSED MATTER AND MATERIALS PHYSICS. Volume: 94 (2016) - 2016 • 4077
Effects of Mn additions on microstructure and properties of Fe–TiB2 based high modulus steels
Baron, C. and Springer, H. and Raabe, D.
MATERIALS AND DESIGN. Volume: 111 (2016) - 2016 • 4076
Fast multitarget tracking via strategy switching for sensor-based sorting
Maier, G. and Pfaff, F. and Pieper, C. and Gruna, R. and Noack, B. and Kruggel-Emden, H. and Langle, T. and Hanebeck, U.D. and Wirtz, S. and Scherer, V. and Beyerer, J.
IEEE INTERNATIONAL CONFERENCE ON MULTISENSOR FUSION AND INTEGRATION FOR INTELLIGENT SYSTEMS. Volume: 0 (2016) - 2016 • 4075
Efficient liquid metallurgy synthesis of Fe-TiB2 high modulus steels via in-situ reduction of titanium oxides
Baron, C. and Springer, H. and Raabe, D.
MATERIALS AND DESIGN. Volume: 97 (2016) - 2016 • 4074
Potential-Pulse-Assisted Formation of Thiol Monolayers within Minutes for Fast and Controlled Electrode Surface Modification
Jambrec, D. and Conzuelo, F. and Estrada-Vargas, A. and Schuhmann, W.
CHEMELECTROCHEM. Volume: 3 (2016) - 2016 • 4073
Birefringence tuning of VCSELs
Pusch, T. and Bou Sanayeh, M. and Lindemann, M. and Gerhardt, N.C. and Hofmann, M.R.
PROCEEDINGS OF SPIE - THE INTERNATIONAL SOCIETY FOR OPTICAL ENGINEERING. Volume: 9892 (2016) - 2016 • 4072
Effect of intercritical deformation on microstructure and mechanical properties of a low-silicon aluminum-added hot-rolled directly quenched and partitioned steel
Tan, X.-D. and Xu, Y.-B. and Ponge, D. and Yang, X.-L. and Hu, Z.-P. and Peng, F. and Ju, X.-W. and Wu, D. and Raabe, D.
MATERIALS SCIENCE AND ENGINEERING A. Volume: 656 (2016) - 2016 • 4071
Monostatic and thickness-independent material characterisation based on microwave ellipsometry
Barowski, J. and Schultze, T. and Willms, I. and Rolfes, I.
GEMIC 2016 - 2016 GERMAN MICROWAVE CONFERENCE. Volume: (2016) - 2016 • 4070
On the physics of a large CCP discharge
Eremin, D. and Bienholz, S. and Szeremley, D. and Trieschmann, J. and Ries, S. and Awakowicz, P. and Mussenbrock, T. and Brinkmann, R.P.
PLASMA SOURCES SCIENCE AND TECHNOLOGY. Volume: 25 (2016) - 2016 • 4069
DNA Intercalators for Detection of DNA Hybridisation: SCS(MI)–MP2 Calculations and Electrochemical Impedance Spectroscopy
Jambrec, D. and Haddad, R. and Lauks, A. and Gebala, M. and Schuhmann, W. and Kokoschka, M.
CHEMPLUSCHEM. Volume: 81 (2016) - 2016 • 4068
Implementation of incremental variational formulations based on the numerical calculation of derivatives using hyper dual numbers
Tanaka, M. and Balzani, D. and Schröder, J.
COMPUTER METHODS IN APPLIED MECHANICS AND ENGINEERING. Volume: 301 (2016) - 2016 • 4067
Investigation on femto-second laser irradiation assisted shock peening of medium carbon (0.4% C) steel
Majumdar, J.D. and Gurevich, E.L. and Kumari, R. and Ostendorf, A.
APPLIED SURFACE SCIENCE. Volume: 364 (2016) - 2016 • 4066
The solidification products of levitated Fe83B17 studied by high-energy x-ray diffraction
Quirinale, D.G. and Rustan, G.E. and Kreyssig, A. and Lapidus, S.H. and Kramer, M.J. and Goldman, A.I.
JOURNAL OF APPLIED PHYSICS. Volume: 120 (2016) - 2016 • 4065
Robust numerical schemes for an efficient implementation of tangent matrices: Application to hyperelasticity, inelastic standard dissipative materials and thermo-mechanics at finite strains
Tanaka, M. and Balzani, D. and Schröder, J.
LECTURE NOTES IN APPLIED AND COMPUTATIONAL MECHANICS. Volume: 81 (2016) - 2016 • 4064
Imaging SPR combined with stereoscopic 3D tracking to study barnacle cyprid-surface interactions
Maleshlijski, S. and Sendra, G.H. and Aldred, N. and Clare, A.S. and Liedberg, B. and Grunze, M. and Ederth, T. and Rosenhahn, A.
SURFACE SCIENCE. Volume: 643 (2016) - 2016 • 4063
Phase sensitive properties and coherent manipulation of a photonic crystal microcavity
Quiring, W. and Jonas, B. and Förstner, J. and Rai, A.K. and Reuter, D. and Wieck, A.D. and Zrenner, A.
OPTICS EXPRESS. Volume: 24 (2016) - 2016 • 4062
Origin of the Resistivity Anisotropy in the Nematic Phase of FeSe
Tanatar, M.A. and Böhmer, A.E. and Timmons, E.I. and Schütt, M. and Drachuck, G. and Taufour, V. and Kothapalli, K. and Kreyssig, A. and Bud'Ko, S.L. and Canfield, P.C. and Fernandes, R.M. and Prozorov, R.
PHYSICAL REVIEW LETTERS. Volume: 117 (2016) - 2016 • 4061
Ab initio-guided design of twinning-induced plasticity steels
Raabe, D. and Roters, F. and Neugebauer, J. and Gutierrez-Urrutia, I. and Hickel, T. and Bleck, W. and Schneider, J.M. and Wittig, J.E. and Mayer, J.
MRS BULLETIN. Volume: 41 (2016) - 2016 • 4060
TiO2 quantum dots embedded in bamboo-like porous carbon nanotubes as ultra high power and long life anodes for lithium ion batteries
Tang, Y. and Liu, L. and Wang, X. and Jia, D. and Xia, W. and Zhao, Z. and Qiu, J.
JOURNAL OF POWER SOURCES. Volume: 319 (2016) - 2016 • 4059
Experimental evidence for the existence of interfaces in graphite and their relation to the observed metallic and superconducting behavior
Esquinazi, P.D. and Lysogorskiy, Y.V.
SPRINGER SERIES IN MATERIALS SCIENCE. Volume: 244 (2016) - 2016 • 4058
An evolutionary topology optimization approach with variationally controlled growth
Jantos, D.R. and Junker, P. and Hackl, K.
COMPUTER METHODS IN APPLIED MECHANICS AND ENGINEERING. Volume: 310 (2016) - 2016 • 4057
Advanced Evaluation of the Long-Term Stability of Oxygen Evolution Electrocatalysts
Maljusch, A. and Conradi, O. and Hoch, S. and Blug, M. and Schuhmann, W.
ANALYTICAL CHEMISTRY. Volume: 88 (2016) - 2016 • 4056
A structural view of synthetic cofactor integration into [FeFe]-hydrogenases
Esselborn, J. and Muraki, N. and Klein, K. and Engelbrecht, V. and Metzler-Nolte, N. and Apfel, U.-P. and Hofmann, E. and Kurisu, G. and Happe, T.
CHEMICAL SCIENCE. Volume: 7 (2016) - 2016 • 4055
Multi-scale and spatially resolved hydrogen mapping in a Ni-Nb model alloy reveals the role of the δ phase in hydrogen embrittlement of alloy 718
Tarzimoghadam, Z. and Rohwerder, M. and Merzlikin, S.V. and Bashir, A. and Yedra, L. and Eswara, S. and Ponge, D. and Raabe, D.
ACTA MATERIALIA. Volume: 109 (2016) - 2016 • 4054
Bipolar Electrochemistry for Concurrently Evaluating the Stability of Anode and Cathode Electrocatalysts and the Overall Cell Performance during Long-Term Water Electrolysis
Eßmann, V. and Barwe, S. and Masa, J. and Schuhmann, W.
ANALYTICAL CHEMISTRY. Volume: 88 (2016) - 2016 • 4053
Microscale Fracture Behavior of Single Crystal Silicon Beams at Elevated Temperatures
Jaya, B.N. and Wheeler, J.M. and Wehrs, J. and Best, J.P. and Soler, R. and Michler, J. and Kirchlechner, C. and Dehm, G.
NANO LETTERS. Volume: 16 (2016) - 2016 • 4052
Understanding of the importance of the spore coat structure and pigmentation in the Bacillus subtilis spore resistance to low-pressure plasma sterilization
Raguse, M. and Fiebrandt, M. and Denis, B. and Stapelmann, K. and Eichenberger, P. and Driks, A. and Eaton, P. and Awakowicz, P. and Moeller, R.
JOURNAL OF PHYSICS D: APPLIED PHYSICS. Volume: 49 (2016) - 2016 • 4051
Differentiation between Single- and Double-Stranded DNA through Local Capacitance Measurements
Estrada-Vargas, A. and Jambrec, D. and Kayran, Y.U. and Kuznetsov, V. and Schuhmann, W.
CHEMELECTROCHEM. Volume: 3 (2016) - 2016 • 4050
Coccospheres confer mechanical protection: New evidence for an old hypothesis
Jaya, B.N. and Hoffmann, R. and Kirchlechner, C. and Dehm, G. and Scheu, C. and Langer, G.
ACTA BIOMATERIALIA. Volume: 42 (2016) - 2016 • 4049
Improvement of biological indicators by uniformly distributing Bacillus subtilis spores in monolayers to evaluate enhanced spore decontamination technologies
Raguse, M. and Fiebrandt, M. and Stapelmann, K. and Madela, K. and Laue, M. and Lackmann, J.-W. and Thwaite, J.E. and Setlow, P. and Awakowicz, P. and Moeller, R.
APPLIED AND ENVIRONMENTAL MICROBIOLOGY. Volume: 82 (2016) - 2016 • 4048
Efficient parallelization of analytic bond-order potentials for large-scale atomistic simulations
Teijeiro, C. and Hammerschmidt, T. and Drautz, R. and Sutmann, G.
COMPUTER PHYSICS COMMUNICATIONS. Volume: 204 (2016) - 2016 • 4047
In Situ Characterization of Ultrathin Films by Scanning Electrochemical Impedance Microscopy
Estrada-Vargas, A. and Bandarenka, A. and Kuznetsov, V. and Schuhmann, W.
ANALYTICAL CHEMISTRY. Volume: 88 (2016) - 2016 • 4046
Spatially indirect transitions in electric field tunable quantum dot diodes
Rai, A.K. and Gordon, S. and Ludwig, Ar. and Wieck, A.D. and Zrenner, A. and Reuter, D.
PHYSICA STATUS SOLIDI (B) BASIC RESEARCH. Volume: 253 (2016) - 2016 • 4045
Complexity analysis of simulations with analytic bond-order potentials
Teijeiro, C. and Hammerschmidt, T. and Seiser, B. and Drautz, R. and Sutmann, G.
MODELLING AND SIMULATION IN MATERIALS SCIENCE AND ENGINEERING. Volume: 24 (2016) - 2016 • 4044
Enhancement of superconducting transition temperature by pointlike disorder and anisotropic energy gap in FeSe single crystals
Teknowijoyo, S. and Cho, K. and Tanatar, M.A. and Gonzales, J. and Böhmer, A.E. and Cavani, O. and Mishra, V. and Hirschfeld, P.J. and Bud'Ko, S.L. and Canfield, P.C. and Prozorov, R.
PHYSICAL REVIEW B. Volume: 94 (2016) - 2016 • 4043
X-Ray diffraction on large single crystals using a powder diffractometer
Jesche, A. and Fix, M. and Kreyssig, A. and Meier, W.R. and Canfield, P.C.
PHILOSOPHICAL MAGAZINE. Volume: 96 (2016) - 2016 • 4042
First-principles-based calculation of the electrocaloric effect in BaTiO3: A comparison of direct and indirect methods
Marathe, M. and Grünebohm, A. and Nishimatsu, T. and Entel, P. and Ederer, C.
PHYSICAL REVIEW B - CONDENSED MATTER AND MATERIALS PHYSICS. Volume: 93 (2016) - 2016 • 4041
On the origin of creep dislocations in a Ni-base, single-crystal superalloy: An ECCI, EBSD, and dislocation dynamics-based study
Ram, F. and Li, Z. and Zaefferer, S. and Hafez Haghighat, S.M. and Zhu, Z. and Raabe, D. and Reed, R.C.
ACTA MATERIALIA. Volume: 109 (2016) - 2016 • 4040
Square Wave Cathodic Adsorptive Stripping Voltammetric Determination of the Anticancer Drugs Flutamide and Irinotecan in Biological Fluids Using Renewable Pencil Graphite Electrodes
Temerk, Y.M. and Ibrahim, H. and Schuhmann, W.
ELECTROANALYSIS. Volume: 28 (2016) - 2016 • 4039
Attachment of Algal Cells to Zwitterionic Self-Assembled Monolayers Comprised of Different Anionic Compounds
Bauer, S. and Finlay, J.A. and Thomé, I. and Nolte, K. and Franco, S.C. and Ralston, E. and Swain, G.E. and Clare, A.S. and Rosenhahn, A.
LANGMUIR. Volume: 32 (2016) - 2016 • 4038
Functional adaptation of crustacean exoskeletal elements through structural and compositional diversity: A combined experimental and theoretical study
Fabritius, H.-O. and Ziegler, A. and Friák, M. and Nikolov, S. and Huber, J. and Seidl, B.H.M. and Ruangchai, S. and Alagboso, F.I. and Karsten, S. and Lu, J. and Janus, A.M. and Petrov, M. and Zhu, L.-F. and Hemzalová, P. and Hild, S. and Raabe, D. and Neugebauer, J.
BIOINSPIRATION AND BIOMIMETICS. Volume: 11 (2016) - 2016 • 4037
Controlling the stress state of La1-xSrxCoyFe1-yO3-δ oxygen transport membranes on porous metallic supports deposited by plasma spray-physical vapor process
Marcano, D. and Mauer, G. and Sohn, Y.J. and Vaßen, R. and Garcia-Fayos, J. and Serra, J.M.
JOURNAL OF MEMBRANE SCIENCE. Volume: 503 (2016) - 2016 • 4036
Resistance of Amphiphilic Polysaccharides against Marine Fouling Organisms
Bauer, S. and Alles, M. and Arpa-Sancet, M.P. and Ralston, E. and Swain, G.W. and Aldred, N. and Clare, A.S. and Finlay, J.A. and Callow, M.E. and Callow, J.A. and Rosenhahn, A.
BIOMACROMOLECULES. Volume: 17 (2016) - 2016 • 4035
The Role of Oxygen Partial Pressure in Controlling the Phase Composition of La1−xSrxCoyFe1−yO3−δ Oxygen Transport Membranes Manufactured by Means of Plasma Spray-Physical Vapor Deposition
Marcano, D. and Mauer, G. and Sohn, Y.J. and Vaßen, R. and Garcia-Fayos, J. and Serra, J.M.
JOURNAL OF THERMAL SPRAY TECHNOLOGY. Volume: 25 (2016) - 2016 • 4034
Crystal plasticity modeling of size effects in rolled multilayered Cu-Nb composites
Jia, N. and Raabe, D. and Zhao, X.
ACTA MATERIALIA. Volume: 111 (2016) - 2016 • 4033
Fermi surface reconstruction in FeSe under high pressure
Terashima, T. and Kikugawa, N. and Kiswandhi, A. and Graf, D. and Choi, E.-S. and Brooks, J.S. and Kasahara, S. and Watashige, T. and Matsuda, Y. and Shibauchi, T. and Wolf, T. and Böhmer, A.E. and Hardy, F. and Meingast, C. and Löhneysen, H.V. and Uji, S.
PHYSICAL REVIEW B. Volume: 93 (2016) - 2016 • 4032
A finite element model for propagating delamination in laminated composite plates based on the Virtual Crack Closure method
Marjanović, M. and Meschke, G. and Vuksanović, D.
COMPOSITE STRUCTURES. Volume: 150 (2016) - 2016 • 4031
Synthesis of nanosized Silicalite-1 in F-media
Jiao, K. and Xu, X. and Lv, Z. and Song, J. and He, M. and Gies, H.
MICROPOROUS AND MESOPOROUS MATERIALS. Volume: 225 (2016) - 2016 • 4030
Structure and properties of ITQ-8: A hydrous layer silicate with microporous silicate layers
Marler, B. and Müller, M. and Gies, H.
DALTON TRANSACTIONS. Volume: 45 (2016) - 2016 • 4029
A higher-order stress-based gradient-enhanced damage model based on isogeometric analysis
Thai, T. Q. and Rabczuk, T. and Bazilevs, Y. and Meschke, G.
COMPUTER METHODS IN APPLIED MECHANICS AND ENGINEERING. Volume: 304 (2016) - 2016 • 4028
An algorithmic scheme for the automated calculation of fiber orientations in arterial walls
Fausten, S. and Balzani, D. and Schröder, J.
COMPUTATIONAL MECHANICS. Volume: 58 (2016) - 2016 • 4027
An electronically reconfigurable reflectarray element based on binary phase shifters for K-band applications
Theissen, H. and Dahl, C. and Rolfes, I. and Musch, T.
GEMIC 2016 - 2016 GERMAN MICROWAVE CONFERENCE. Volume: (2016) - 2016 • 4026
Tempering behavior of a low nitrogen boron-added 9%Cr steel
Fedorova, I. and Kostka, A. and Tkachev, E. and Belyakov, A. and Kaibyshev, R.
MATERIALS SCIENCE AND ENGINEERING A. Volume: 662 (2016) - 2016 • 4025
Mesoporous nitrogen containing carbon materials for the simultaneous detection of ascorbic acid, dopamine and uric acid
Joshi, A. and Schuhmann, W. and Nagaiah, T.C.
SENSORS AND ACTUATORS, B: CHEMICAL. Volume: 230 (2016) - 2016 • 4024
Diffusion of small molecules in a shape memory polymer
Marquardt, A. and Mogharebi, S. and Neuking, K. and Varnik, F. and Eggeler, G.
JOURNAL OF MATERIALS SCIENCE. Volume: 51 (2016) - 2016 • 4023
Intracellular Hydrogen Peroxide Detection with Functionalised Nanoelectrodes
Marquitan, M. and Clausmeyer, J. and Actis, P. and Córdoba, A.L. and Korchev, Y. and Mark, M.D. and Herlitze, S. and Schuhmann, W.
CHEMELECTROCHEM. Volume: 3 (2016) - 2016 • 4022
Influence of the twin microstructure on the mechanical properties in magnetic shape memory alloys
Reinholz, B. and Brinckmann, S. and Hartmaier, A. and Muntifering, B. and Knowlton, W.B. and Müllner, P.
ACTA MATERIALIA. Volume: 108 (2016) - 2016 • 4021
A discontinuous phase field approach to variational growth-based topology optimization
Junker, P. and Hackl, K.
STRUCTURAL AND MULTIDISCIPLINARY OPTIMIZATION. Volume: 54 (2016) - 2016 • 4020
Erratum: “Perspective: Machine learning potentials for atomistic simulations” (The Journal of Chemical Physics (2016) 145 (170901) DOI: 10.1063/1.4966192)
Behler, J.
JOURNAL OF CHEMICAL PHYSICS. Volume: 145 (2016) - 2016 • 4019
Comparative evaluation of normal viscoelastic contact force models in low velocity impact situations
Kacianauskas, R. and Kruggel-Emden, H. and Zdancevicius, E. and Markauskas, D.
ADVANCED POWDER TECHNOLOGY. Volume: 27 (2016) - 2016 • 4018
Perspective: Machine learning potentials for atomistic simulations
Behler, J.
JOURNAL OF CHEMICAL PHYSICS. Volume: 145 (2016) - 2016 • 4017
Optimization of artificial ground freezing in tunneling in the presence of seepage flow
Marwan, A. and Zhou, M.-M. and Zaki Abdelrehim, M. and Meschke, G.
COMPUTERS AND GEOTECHNICS. Volume: 75 (2016) - 2016 • 4016
Polaron-induced lattice distortion of (In,Ga)As/GaAs quantum dots by optically excited carriers
Tiemeyer, S. and Bombeck, M. and Göhring, H. and Paulus, M. and Sternemann, C. and Nase, J. and Wirkert, F.J. and Möller, J. and Büning, T. and Seeck, O.H. and Reuter, D. and Wieck, A.D. and Bayer, M. and Tolan, M.
NANOTECHNOLOGY. Volume: 27 (2016) - 2016 • 4015
Theoretical Chemistry 2015 [Theoretische Chemie 2015]
Behler, J.
NACHRICHTEN AUS DER CHEMIE. Volume: 64 (2016) - 2016 • 4014
Strain-induced phase transformation of a thin Co film on flexible substrates
Marx, V.M. and Kirchlechner, C. and Breitbach, B. and Cordill, M.J. and Többens, D.M. and Waitz, T. and Dehm, G.
ACTA MATERIALIA. Volume: 121 (2016) - 2016 • 4013
Vessel microstructure design: A new approach for site-specific core-shell micromechanical tailoring of TRIP-assisted ultra-high strength steels
Belde, M. and Springer, H. and Raabe, D.
ACTA MATERIALIA. Volume: 113 (2016) - 2016 • 4012
Nonmonotonic pressure evolution of the upper critical field in superconducting FeSe
Kaluarachchi, U.S. and Taufour, V. and Böhmer, A.E. and Tanatar, M.A. and Bud'Ko, S.L. and Kogan, V.G. and Prozorov, R. and Canfield, P.C.
PHYSICAL REVIEW B. Volume: 93 (2016) - 2016 • 4011
Amorphous Cobalt Boride (Co2B) as a Highly Efficient Nonprecious Catalyst for Electrochemical Water Splitting: Oxygen and Hydrogen Evolution
Masa, J. and Weide, P. and Peeters, D. and Sinev, I. and Xia, W. and Sun, Z. Y. and Somsen, C. and Muhler, M. and Schuhmann, W.
ADVANCED ENERGY MATERIALS. Volume: 6 (2016) - 2016 • 4010
Investigation of magnetic phases in parent compounds of iron-chalcogenides via quasiparticle scattering interference
Kamble, B. and Akbari, A. and Eremin, I.
EPL. Volume: 114 (2016) - 2016 • 4009
Low Overpotential Water Splitting Using Cobalt-Cobalt Phosphide Nanoparticles Supported on Nickel Foam
Masa, J. and Barwe, S. and Andrpnescu, C. and Sinev, I. and Ruff, A. and Jayaramulu, K. and Elumeeva, K. and Konkena, B. and Cuenya, B. R. and Schuhmann, W.
ACS ENERGY LETTERS. Volume: 1 (2016) - 2016 • 4008
Nanocasting Design and Spatially Selective Sulfonation of Polystyrene-Based Polymer Networks as Solid Acid Catalysts
Richter, F.H. and Sahraoui, L. and Schüth, F.
CHEMISTRY - A EUROPEAN JOURNAL. Volume: 22 (2016) - 2016 • 4007
Electrocatalysis and bioelectrocatalysis – Distinction without a difference
Masa, J. and Schuhmann, W.
NANO ENERGY. Volume: 29 (2016) - 2016 • 4006
Common-path depth-filtered digital holography for high resolution imaging of buried semiconductor structures
Finkeldey, M. and Schellenberg, F. and Gerhardt, N.C. and Paar, C. and Hofmann, M.R.
PROCEEDINGS OF SPIE - THE INTERNATIONAL SOCIETY FOR OPTICAL ENGINEERING. Volume: 9771 (2016) - 2016 • 4005
Application of scanning electrochemical microscopy (SECM) to study electrocatalysis of oxygen reduction by MN4-macrocyclic complexes
Masa, J. and Ventosa, E. and Schuhmann, W.
ELECTROCHEMISTRY OF N4 MACROCYCLIC METAL COMPLEXES: VOLUME 1: ENERGY, SECOND EDITION. Volume: (2016) - 2016 • 4004
Stacking fault based analysis of shear mechanisms at interfaces in lamellar TiAl alloys
Kanani, M. and Hartmaier, A. and Janisch, R.
ACTA MATERIALIA. Volume: 106 (2016) - 2016 • 4003
Improved thermodynamic treatment of vacancy-mediated diffusion and creep
Fischer, F.D. and Hackl, K. and Svoboda, J.
ACTA MATERIALIA. Volume: 108 (2016) - 2016 • 4002
Heat flow, transport and fluctuations in etched semiconductor quantum wire structures
Riha, C. and Chiatti, O. and Buchholz, S.S. and Reuter, D. and Wieck, A.D. and Fischer, S.F.
PHYSICA STATUS SOLIDI (A) APPLICATIONS AND MATERIALS SCIENCE. Volume: 213 (2016) - 2016 • 4001
Product distribution of CO2 hydrogenation by K- and Mn-promoted Fe catalysts supported on N-functionalized carbon nanotubes
Kangvansura, P. and Chew, L.M. and Saengsui, W. and Santawaja, P. and Poo-arporn, Y. and Muhler, M. and Schulz, H. and Worayingyong, A.
CATALYSIS TODAY. Volume: 275 (2016) - 2016 • 4000
Influence of the liquid on femtosecond laser ablation of iron
Kanitz, A. and Hoppius, J.S. and Gurevich, E.L. and Ostendorf, A.
PHYSICS PROCEDIA. Volume: 83 (2016) - 2016 • 3999
High order path integrals made easy
Kapil, V. and Behler, J. and Ceriotti, M.
JOURNAL OF CHEMICAL PHYSICS. Volume: 145 (2016) - 2016 • 3998
Broadband terahertz dispersion control in hybrid waveguides
Fobbe, T. and Markmann, S. and Fobbe, F. and Hekmat, N. and Nong, H. and Pal, S. and Balzerwoski, P. and Savolainen, J. and Havenith, M. and Wieck, A.D. and Jukam, N.
OPTICS EXPRESS. Volume: 24 (2016) - 2016 • 3997
Electrostatic levitation facility optimized for neutron diffraction studies of high temperature liquids at a spallation neutron source
Mauro, N.A. and Vogt, A.J. and Derendorf, K.S. and Johnson, M.L. and Rustan, G.E. and Quirinale, D.G. and Kreyssig, A. and Lokshin, K.A. and Neuefeind, J.C. and An, K. and Wang, X.-L. and Goldman, A.I. and Egami, T. and Kelton, K.F.
REVIEW OF SCIENTIFIC INSTRUMENTS. Volume: 87 (2016) - 2016 • 3996
Growth of bainitic ferrite and carbon partitioning during the early stages of bainite transformation in a 2 mass% silicon steel studied by in situ neutron diffraction, TEM and APT
Timokhina, I.B. and Liss, K.D. and Raabe, D. and Rakha, K. and Beladi, H. and Xiong, X.Y. and Hodgson, P.D.
JOURNAL OF APPLIED CRYSTALLOGRAPHY. Volume: 49 (2016) - 2016 • 3995
Improving the Out-Coupling of a Metal-Metal Terahertz Frequency Quantum Cascade Laser Through Integration of a Hybrid Mode Section into the Waveguide
Fobbe, T. and Nong, H. and Schott, R. and Pal, S. and Markmann, S. and Hekmat, N. and Zhu, J. and Han, Y. and Li, L. and Dean, P. and Linfield, E.H. and Davies, A.G. and Wieck, A.D. and Jukam, N.
JOURNAL OF INFRARED, MILLIMETER, AND TERAHERTZ WAVES. Volume: 37 (2016) - 2016 • 3994
Superconducting phase diagram of itinerant antiferromagnets
Rømer, A.T. and Eremin, I. and Hirschfeld, P.J. and Andersen, B.M.
PHYSICAL REVIEW B - CONDENSED MATTER AND MATERIALS PHYSICS. Volume: 93 (2016) - 2016 • 3993
Cascade lattice micromechanics model for the effective permeability of materials with microcracks
Timothy, J.J. and Meschke, G.
JOURNAL OF NANOMECHANICS AND MICROMECHANICS. Volume: 6 (2016) - 2016 • 3992
A micromechanics model for molecular diffusion in materials with complex pore structure
Timothy, J.J. and Meschke, G.
INTERNATIONAL JOURNAL FOR NUMERICAL AND ANALYTICAL METHODS IN GEOMECHANICS. Volume: 40 (2016) - 2016 • 3991
Optical visualization of radiative recombination at partial dislocations in GaAs
Karin, T. and Linpeng, X. and Rai, A.K. and Ludwig, Ar. and Wieck, A.D. and Fu, K.-M.C.
CONFERENCE RECORD OF THE IEEE PHOTOVOLTAIC SPECIALISTS CONFERENCE. Volume: 2016-November (2016) - 2016 • 3990
A cascade continuum micromechanics model for the effective elastic properties of porous materials
Timothy, J.J. and Meschke, G.
INTERNATIONAL JOURNAL OF SOLIDS AND STRUCTURES. Volume: 83 (2016) - 2016 • 3989
Giant permanent dipole moment of two-dimensional excitons bound to a single stacking fault
Karin, T. and Linpeng, X. and Glazov, M.M. and Durnev, M.V. and Ivchenko, E.L. and Harvey, S. and Rai, A.K. and Ludwig, Ar. and Wieck, A.D. and Fu, K.-M.C.
PHYSICAL REVIEW B - CONDENSED MATTER AND MATERIALS PHYSICS. Volume: 94 (2016) - 2016 • 3988
Effect of Si on the acceleration of bainite transformation by pre-existing martensite
Toji, Y. and Matsuda, H. and Raabe, D.
ACTA MATERIALIA. Volume: 116 (2016) - 2016 • 3987
Interplay of the Open Circuit Potential-Relaxation and the Dissolution Behavior of a Single H2 Bubble Generated at a Pt Microelectrode
Karnbach, F. and Yang, X. and Mutschke, G. and Fröhlich, J. and Eckert, J. and Gebert, A. and Tschulik, K. and Eckert, K. and Uhlemann, M.
JOURNAL OF PHYSICAL CHEMISTRY C. Volume: 120 (2016) - 2016 • 3986
Linking mathematics with engineering applications at an early stage – implementation, experimental set-up and evaluation of a pilot project
Rooch, A. and Junker, P. and Härterich, J. and Hackl, K.
EUROPEAN JOURNAL OF ENGINEERING EDUCATION. Volume: 41 (2016) - 2016 • 3985
Fast spin information transfer between distant quantum dots using individual electrons
Bertrand, B. and Hermelin, S. and Takada, S. and Yamamoto, M. and Tarucha, S. and Ludwig, Ar. and Wieck, A.D. and Bäuerle, C. and Meunier, T.
NATURE NANOTECHNOLOGY. Volume: 11 (2016) - 2016 • 3984
Hollow Zn/Co Zeolitic Imidazolate Framework (ZIF) and Yolk-Shell Metal@Zn/Co ZIF Nanostructures
Rösler, C. and Aijaz, A. and Turner, S. and Filippousi, M. and Shahabi, A. and Xia, W. and Van Tendeloo, G. and Muhler, M. and Fischer, R.A.
CHEMISTRY - A EUROPEAN JOURNAL. Volume: 22 (2016) - 2016 • 3983
Injection of a single electron from static to moving quantum dots
Bertrand, B. and Hermelin, S. and Mortemousque, P.-A. and Takada, S. and Yamamoto, M. and Tarucha, S. and Ludwig, Ar. and Wieck, A.D. and Bäuerle, C. and Meunier, T.
NANOTECHNOLOGY. Volume: 27 (2016) - 2016 • 3982
Modeling of Gibbs energies of pure elements down to 0 K using segmented regression
Roslyakova, I. and Sundman, B. and Dette, H. and Zhang, L. and Steinbach, I.
CALPHAD: COMPUTER COUPLING OF PHASE DIAGRAMS AND THERMOCHEMISTRY. Volume: 55 (2016) - 2016 • 3981
Heat-Induced Phase Transformation of Three-Dimensional Nb3O7(OH) Superstructures: Effect of Atmosphere and Electron Beam
Betzler, S.B. and Harzer, T. and Ciston, J. and Dahmen, U. and Dehm, G. and Scheu, C.
CRYSTAL GROWTH AND DESIGN. Volume: 16 (2016) - 2016 • 3980
Electron and chemical reservoir corrections for point-defect formation energies
Freysoldt, C. and Lange, B. and Neugebauer, J. and Yan, Q. and Lyons, J.L. and Janotti, A. and Van De Walle, C.G.
PHYSICAL REVIEW B - CONDENSED MATTER AND MATERIALS PHYSICS. Volume: 93 (2016) - 2016 • 3979
Cold atmospheric-pressure plasma and bacteria: Understanding the mode of action using vibrational microspectroscopy
Kartaschew, K. and Baldus, S. and Mischo, M. and Bründermann, E. and Awakowicz, P. and Havenith, M.
JOURNAL OF PHYSICS D: APPLIED PHYSICS. Volume: 49 (2016) - 2016 • 3978
From Gold Nanoseeds to Nanorods: The Microscopic Origin of the Anisotropic Growth
Meena, S.K. and Sulpizi, M.
ANGEWANDTE CHEMIE - INTERNATIONAL EDITION. Volume: 55 (2016) - 2016 • 3977
On the origin of the improved ruthenium stability in RuO2-IrO2 mixed oxides
Kasian, O. and Geiger, S. and Stock, P. and Polymeros, G. and Breitbach, B. and Savan, A. and Ludwig, Al. and Cherevko, S. and Mayrhofer, K.J.J.
JOURNAL OF THE ELECTROCHEMICAL SOCIETY. Volume: 163 (2016) - 2016 • 3976
The role of halide ions in the anisotropic growth of gold nanoparticles: A microscopic, atomistic perspective
Meena, S.K. and Celiksoy, S. and Schäfer, P. and Henkel, A. and Sönnichsen, C. and Sulpizi, M.
PHYSICAL CHEMISTRY CHEMICAL PHYSICS. Volume: 18 (2016) - 2016 • 3975
Photoluminescence of gallium ion irradiated hexagonal and cubic GaN quantum dots
Rothfuchs, C. and Kukharchyk, N. and Koppe, T. and Semond, F. and Blumenthal, S. and Becker, H.-W. and As, D.J. and Hofsäss, H.C. and Wieck, A.D. and Ludwig, Ar.
NUCLEAR INSTRUMENTS AND METHODS IN PHYSICS RESEARCH, SECTION B: BEAM INTERACTIONS WITH MATERIALS AND ATOMS. Volume: 383 (2016) - 2016 • 3974
Structural Transitions in a Quasi-1D Wigner Solid on Liquid Helium
Beysengulov, N.R. and Rees, D.G. and Lysogorskiy, Y. and Galiullin, N.K. and Vazjukov, A.S. and Tayurskii, D.A. and Kono, K.
JOURNAL OF LOW TEMPERATURE PHYSICS. Volume: 182 (2016) - 2016 • 3973
Electrochemical Investigations of the Mechanism of Assembly of the Active-Site H-Cluster of [FeFe]-Hydrogenases
Megarity, C.F. and Esselborn, J. and Hexter, S.V. and Wittkamp, F. and Apfel, U.-P. and Happe, T. and Armstrong, F.A.
JOURNAL OF THE AMERICAN CHEMICAL SOCIETY. Volume: 138 (2016) - 2016 • 3972
Altering the luminescence properties of self-assembled quantum dots in GaAs by focused ion beam implantation
Rothfuchs, C. and Kukharchyk, N. and Greff, M.K. and Wieck, A.D. and Ludwig, Ar.
APPLIED PHYSICS B: LASERS AND OPTICS. Volume: 122 (2016) - 2016 • 3971
A higherature ferromagnetic topological insulating phase by proximity coupling
Katmis, F. and Lauter, V. and Nogueira, F.S. and Assaf, B.A. and Jamer, M.E. and Wei, P. and Satpati, B. and Freeland, J.W. and Eremin, I. and Heiman, D. and Jarillo-Herrero, P. and Moodera, J.S.
NATURE. Volume: 533 (2016) - 2016 • 3970
Physisorption versus chemisorption of oxygen molecules on Ag(100)
Mehlhorn, M. and Morgenstern, K.
JOURNAL OF CHEMICAL PHYSICS. Volume: 144 (2016) - 2016 • 3969
Three-Parameter Crystal-Structure Prediction for sp-d-Valent Compounds
Bialon, A.F. and Hammerschmidt, T. and Drautz, R.
CHEMISTRY OF MATERIALS. Volume: 28 (2016) - 2016 • 3968
Process flow to integrate nanostructures on silicon grass in surface micromachined systems
Mehner, H. and Müller, L. and Biermann, S. and Hänschke, F. and Hoffmann, M.
JOURNAL OF PHYSICS: CONFERENCE SERIES. Volume: 757 (2016) - 2016 • 3967
Gadolinium zirconate/YSZ thermal barrier coatings: Mixed-mode interfacial fracture toughness and sintering behavior
Frommherz, M. and Scholz, A. and Oechsner, M. and Bakan, E. and Vaßen, R.
SURFACE AND COATINGS TECHNOLOGY. Volume: 286 (2016) - 2016 • 3966
Synthesis of N-alkyl-4-vinylpyridinium-based cross-linked polymers and their catalytic performance for the conversion of fructose into 5-hydroxymethylfurfural
Ruby, M.-P. and Schüth, F.
GREEN CHEMISTRY. Volume: 18 (2016) - 2016 • 3965
Making the hydrogen evolution reaction in polymer electrolyte membrane electrolysers even faster
Tymoczko, J. and Calle-Vallejo, F. and Schuhmann, W. and Bandarenka, A.S.
NATURE COMMUNICATIONS. Volume: 7 (2016) - 2016 • 3964
Selection of Highly SERS-Active Nanostructures from a Size Gradient of Au Nanovoids on a Single Bipolar Electrode
Kayran, Y.U. and Eßmann, V. and Grützke, S. and Schuhmann, W.
CHEMELECTROCHEM. Volume: 3 (2016) - 2016 • 3963
Anisotropic thermodynamic and transport properties of single-crystalline CaKFe4As4
Meier, W.R. and Kong, T. and Kaluarachchi, U.S. and Taufour, V. and Jo, N.H. and Drachuck, G. and Böhmer, A.E. and Saunders, S.M. and Sapkota, A. and Kreyssig, A. and Tanatar, M.A. and Prozorov, R. and Goldman, A.I. and Balakirev, F.F. and Gurevich, A. and Bud'Ko, S.L. and Canfield, P.C.
PHYSICAL REVIEW B. Volume: 94 (2016) - 2016 • 3962
Signatures of hyperfine, spin-orbit, and decoherence effects in a Pauli spin blockade
Fujita, T. and Stano, P. and Allison, G. and Morimoto, K. and Sato, Y. and Larsson, M. and Park, J.-H. and Ludwig, Ar. and Wieck, A.D. and Oiwa, A. and Tarucha, S.
PHYSICAL REVIEW LETTERS. Volume: 117 (2016) - 2016 • 3961
Monitoring of low pressure plasmas with a calibrated probe
Runkel, J. and Schulz, C. and Rolfes, I. and Oberberg, M. and Awakowicz, P.
GEMIC 2016 - 2016 GERMAN MICROWAVE CONFERENCE. Volume: (2016) - 2016 • 3960
A computational analysis of the vibrational levels of molecular oxygen in low-pressure stationary and transient radio-frequency oxygen plasma
Kemaneci, E. and Booth, J.-P. and Chabert, P. and Van Dijk, J. and Mussenbrock, T. and Brinkmann, R.P.
PLASMA SOURCES SCIENCE AND TECHNOLOGY. Volume: 25 (2016) - 2016 • 3959
Control of High Power Pulsed Magnetron Discharge by Monitoring the Current Voltage Characteristics
von Keudell, A. and Hecimovic, A. and Maszl, C.
CONTRIBUTIONS TO PLASMA PHYSICS. Volume: 56 (2016) - 2016 • 3958
Long-Term functionalization of optical resonance sensor spots
Saetchnikov, V.A. and Tcherniavskaia, E.A. and Saetchnikov, A.V. and Schweiger, G. and Ostendorf, A.
PROCEEDINGS OF SPIE - THE INTERNATIONAL SOCIETY FOR OPTICAL ENGINEERING. Volume: 9884 (2016) - 2016 • 3957
Atomic-layer-controlled deposition of TEMAZ/O2-ZrO2 oxidation resistance inner surface coatings for solid oxide fuel cells
Keuter, T. and Mauer, G. and Vondahlen, F. and Iskandar, R. and Menzler, N.H. and Vaßen, R.
SURFACE AND COATINGS TECHNOLOGY. Volume: 288 (2016) - 2016 • 3956
Characterization of mechanical properties of hydroxyapatite-silicon-multi walled carbon nano tubes composite coatings synthesized by EPD on NiTi alloys for biomedical application
Khalili, V. and Khalil-Allafi, J. and Sengstock, C. and Motemani, Y. and Paulsen, A. and Frenzel, J. and Eggeler, G. and Köller, M.
JOURNAL OF THE MECHANICAL BEHAVIOR OF BIOMEDICAL MATERIALS. Volume: 59 (2016) - 2016 • 3955
High-Temperature Stable Ni Nanoparticles for the Dry Reforming of Methane
Mette, K. and Kühl, S. and Tarasov, A. and Willinger, M.G. and Kröhnert, J. and Wrabetz, S. and Trunschke, A. and Scherzer, M. and Girgsdies, F. and Düdder, H. and Kähler, K. and Ortega, K.F. and Muhler, M. and Schlögl, R. and Behrens, M. and Lunkenbein, T.
ACS CATALYSIS. Volume: 6 (2016) - 2016 • 3954
A new force field including charge directionality for TMAO in aqueous solution
Usui, K. and Nagata, Y. and Hunger, J. and Bonn, M. and Sulpizi, M.
JOURNAL OF CHEMICAL PHYSICS. Volume: 145 (2016) - 2016 • 3953
Benchmarking the Performance of Thin-Film Oxide Electrocatalysts for Gas Evolution Reactions at High Current Densities
Ganassin, A. and Maljusch, A. and Colic, V. and Spanier, L. and Brandl, K. and Schuhmann, W. and Bandarenka, A.
ACS CATALYSIS. Volume: 6 (2016) - 2016 • 3952
Preparing hydroxyapatite-silicon composite suspensions with homogeneous distribution of multi-walled carbon nano-tubes for electrophoretic coating of NiTi bone implant and their effect on the surface morphology
Khalili, V. and Khalil-Allafi, J. and Xia, W. and Parsa, A.B. and Frenzel, J. and Somsen, C. and Eggeler, G.
APPLIED SURFACE SCIENCE. Volume: 366 (2016) - 2016 • 3951
Ab Initio Study of Deformation Influence on the Electronic Properties of Graphene Structures Containing One-Dimensional Topological Defects
Valishina, A.A. and Lysogorskiy, Y.V. and Nedopekin, O.V. and Tayurskii, D.A.
JOURNAL OF LOW TEMPERATURE PHYSICS. Volume: 185 (2016) - 2016 • 3950
Smooth backfitting in additive inverse regression
Bissantz, N. and Dette, H. and Hildebrandt, T. and Bissantz, K.
ANNALS OF THE INSTITUTE OF STATISTICAL MATHEMATICS. Volume: 68 (2016) - 2016 • 3949
The influence of Si as reactive bonding agent in the electrophoretic coatings of HA–Si–MWCNTs on NiTi alloys
Khalili, V. and Khalil-Allafi, J. and Maleki-Ghaleh, H. and Paulsen, A. and Frenzel, J. and Eggeler, G.
JOURNAL OF MATERIALS ENGINEERING AND PERFORMANCE. Volume: 25 (2016) - 2016 • 3948
Ions and solvation at biointerfaces
Valtiner, M. and Erbe, A. and Rosenhahn, A.
BIOINTERPHASES. Volume: 11 (2016) - 2016 • 3947
One-step solvothermal synthesis of quasi-hexagonal Fe2O3 nanoplates/graphene composite as high performance electrode material for supercapacitor
Gao, Y. and Wu, D. L. and Wang, T. and Jia, D. Z. and Xia, W. and Lv, Y. and Cao, Y. L. and Tan, Y. Y. and Liu, P. G.
ELECTROCHIMICA ACTA. Volume: 191 (2016) - 2016 • 3946
Structure-Activity-Stability Relationships for Space-Confined PtxNiy Nanoparticles in the Oxygen Reduction Reaction
Mezzavilla, S. and Baldizzone, C. and Swertz, A.-C. and Hodnik, N. and Pizzutilo, E. and Polymeros, G. and Keeley, G.P. and Knossalla, J. and Heggen, M. and Mayrhofer, K.J.J. and Schüth, F.
ACS CATALYSIS. Volume: 6 (2016) - 2016 • 3945
The 2016 Thermal Spray Roadmap
Vardelle, A. and Moreau, C. and Akedo, J. and Ashrafizadeh, H. and Berndt, C.C. and Berghaus, J.O. and Boulos, M. and Brogan, J. and Bourtsalas, A.C. and Dolatabadi, A. and Dorfman, M. and Eden, T.J. and Fauchais, P. and Fisher, G. and Gaertner, F. and Gindrat, M. and Henne, R. and Hyland, M. and Irissou, E. and Jordan, E.H. and Khor, K.A. and Killinger, A. and Lau, Y.-C. and Li, C.-J. and Li, L. and Longtin, J. and Markocsan, N. and Masset, P.J. and Matejicek, J. and Mauer, G. and McDonald, A. and Mostaghimi, J. and Sampath, S. and Schiller, G. and Shinoda, K. and Smith, M.F. and Syed, A.A. and Themelis, N.J. and Toma, F.-L. and Trelles, J.P. and Vassen, R. and Vuoristo, P.
JOURNAL OF THERMAL SPRAY TECHNOLOGY. Volume: 25 (2016) - 2016 • 3944
Non-monotonic effect of confinement on the glass transition
Varnik, F. and Franosch, T.
JOURNAL OF PHYSICS CONDENSED MATTER. Volume: 28 (2016) - 2016 • 3943
A Three-Electrode, Battery-Type Swagelok Cell for the Evaluation of Secondary Alkaline Batteries: The Case of the Ni-Zn Battery
Garcia, G. and Schuhmann, W. and Ventosa, E.
CHEMELECTROCHEM. Volume: 3 (2016) - 2016 • 3942
Molecular Dynamics Simulations of SFG Librational Modes Spectra of Water at the Water-Air Interface
Khatib, R. and Hasegawa, T. and Sulpizi, M. and Backus, E.H.G. and Bonn, M. and Nagata, Y.
JOURNAL OF PHYSICAL CHEMISTRY C. Volume: 120 (2016) - 2016 • 3941
Advanced optical manipulation of carrier spins in (In,Ga)As quantum dots
Varwig, S. and Evers, E. and Greilich, A. and Yakovlev, D.R. and Reuter, D. and Wieck, A.D. and Meier, T. and Zrenner, A. and Bayer, M.
APPLIED PHYSICS B: LASERS AND OPTICS. Volume: 122 (2016) - 2016 • 3940
Impact of local magnetism on stacking fault energies: A first-principles investigation for fcc iron
Bleskov, I. and Hickel, T. and Neugebauer, J. and Ruban, A.
PHYSICAL REVIEW B - CONDENSED MATTER AND MATERIALS PHYSICS. Volume: 93 (2016) - 2016 • 3939
Water orientation and hydrogen-bond structure at the fluorite/water interface
Khatib, R. and Backus, E.H.G. and Bonn, M. and Perez-Haro, M.-J. and Gaigeot, M.-P. and Sulpizi, M.
SCIENTIFIC REPORTS. Volume: 6 (2016) - 2016 • 3938
Aging of atmospherically plasma sprayed chromium evaporation barriers
Vaßen, R. and Grünwald, N. and Marcano, D. and Menzler, N.H. and Mücke, R. and Sebold, D. and Sohn, Y.J. and Guillon, O.
SURFACE AND COATINGS TECHNOLOGY. Volume: 291 (2016) - 2016 • 3937
Comparing the accuracy of high-dimensional neural network potentials and the systematic molecular fragmentation method: A benchmark study for all-trans alkanes
Gastegger, M. and Kauffmann, C. and Behler, J. and Marquetand, P.
JOURNAL OF CHEMICAL PHYSICS. Volume: 144 (2016) - 2016 • 3936
Dispersibility of vapor phase oxygen and nitrogen functionalized multi-walled carbon nanotubes in various organic solvents
Khazaee, M. and Xia, W. and Lackner, G. and Mendes, R.G. and Rummeli, M. and Muhler, M. and Lupascu, D.C.
SCIENTIFIC REPORTS. Volume: 6 (2016) - 2016 • 3935
The role of silicon, vacancies, and strain in carbon distribution in low temperature bainite
Sampath, S. and Rementeria, R. and Huang, X. and Poplawsky, J.D. and Garcia-Mateo, C. and Caballero, F.G. and Janisch, R.
JOURNAL OF ALLOYS AND COMPOUNDS. Volume: 673 (2016) - 2016 • 3934
An efficient PE-ALD process for TiO2 thin films employing a new Ti-precursor
Gebhard, M. and Mitschker, F. and Wiesing, M. and Giner, I. and Torun, B. and De Los Arcos, T. and Awakowicz, P. and Grundmeier, G. and Devi, A.
JOURNAL OF MATERIALS CHEMISTRY C. Volume: 4 (2016) - 2016 • 3933
Ab Initio Determined Phase Diagram of Clean and Solvated Muscovite Mica Surfaces
Vatti, A.K. and Todorova, M. and Neugebauer, J.
LANGMUIR. Volume: 32 (2016) - 2016 • 3932
Highly selective plasma-activated copper catalysts for carbon dioxide reduction to ethylene
Mistry, H. and Varela, A.S. and Bonifacio, C.S. and Zegkinoglou, I. and Sinev, I. and Choi, Y.-W. and Kisslinger, K. and Stach, E.A. and Yang, J.C. and Strasser, P. and Cuenya, B.R.
NATURE COMMUNICATIONS. Volume: 7 (2016) - 2016 • 3931
Understanding surface reactivity of Si electrodes in Li-ion batteries by: In operando scanning electrochemical microscopy
Ventosa, E. and Wilde, P. and Zinn, A.-H. and Trautmann, M. and Ludwig, Al. and Schuhmann, W.
CHEMICAL COMMUNICATIONS. Volume: 52 (2016) - 2016 • 3930
Incomplete Bilayer Termination of the Ice (0001) Surface
Bockstedte, M. and Michl, A. and Kolb, M. and Mehlhorn, M. and Morgenstern, K.
JOURNAL OF PHYSICAL CHEMISTRY C. Volume: 120 (2016) - 2016 • 3929
The role of metastable LPSO building block clusters in phase transformations of an Mg-Y-Zn alloy
Kim, J.-K. and Ko, W.-S. and Sandlöbes, S. and Heidelmann, M. and Grabowski, B. and Raabe, D.
ACTA MATERIALIA. Volume: 112 (2016) - 2016 • 3928
Nanostructured electrocatalysts with tunable activity and selectivity
Mistry, H. and Varela, A. S. and Kuhl, S. and Strasser, P. and Cuenya, B. R.
NATURE REVIEWS MATERIALS. Volume: 1 (2016) - 2016 • 3927
Understanding memory effects in Li-ion batteries: Evidence of a kinetic origin in TiO2 upon hydrogen annealing
Ventosa, E. and Löffler, T. and La Mantia, F. and Schuhmann, W.
CHEMICAL COMMUNICATIONS. Volume: 52 (2016) - 2016 • 3926
Spectral analysis of the Moore-Penrose inverse of a large dimensional sample covariance matrix
Bodnar, T. and Dette, H. and Parolya, N.
JOURNAL OF MULTIVARIATE ANALYSIS. Volume: 148 (2016) - 2016 • 3925
Tuning Catalytic Selectivity at the Mesoscale via Interparticle Interactions
Mistry, H. and Behafarid, F. and Reske, R. and Varela, A.S. and Strasser, P. and Roldan Cuenya, B.
ACS CATALYSIS. Volume: 6 (2016) - 2016 • 3924
High-frequency operation of spin vertical-cavity surface-emitting lasers: Towards 100 GHz
Gerhardt, N.C. and Lindemann, M. and Pusch, T. and Michalzik, R. and Hofmann, M.R.
PROCEEDINGS OF SPIE - THE INTERNATIONAL SOCIETY FOR OPTICAL ENGINEERING. Volume: 9931 (2016) - 2016 • 3923
Simultaneous measurements of photocurrents and H2O2 evolution from solvent exposed photosystem 2 complexes
Vöpel, T. and Saw, E.N. and Hartmann, V. and Williams, R. and Müller, F. and Schuhmann, W. and Plumeré, N. and Nowaczyk, M. and Ebbinghaus, S. and Rögner, M.
BIOINTERPHASES. Volume: 11 (2016) - 2016 • 3922
Variation of transition temperatures and residual resistivity ratio in vapor-grown FeSe
Böhmer, A.E. and Taufour, V. and Straszheim, W.E. and Wolf, T. and Canfield, P.C.
PHYSICAL REVIEW B. Volume: 94 (2016) - 2016 • 3921
Birefringent vertical cavity surface-emitting lasers: Toward high-speed spin-lasers
Gerhardt, N.C. and Lindemann, M. and Pusch, T. and Michalzik, R. and Hofmann, M.R.
PROCEEDINGS OF SPIE - THE INTERNATIONAL SOCIETY FOR OPTICAL ENGINEERING. Volume: 9892 (2016) - 2016 • 3920
Electronic nematic susceptibility of iron-based superconductors
Böhmer, A.E. and Meingast, C.
COMPTES RENDUS PHYSIQUE. Volume: 17 (2016) - 2016 • 3919
Multi-spectral echo signal processing for improved detection and classification of radar targets
Vogt, M. and Neumann, T. and Gerding, M. and Dahl, C. and Rolfes, I.
GEMIC 2016 - 2016 GERMAN MICROWAVE CONFERENCE. Volume: (2016) - 2016 • 3918
Experimental and numerical study of fluidization and pressure drop of spherical and non-spherical particles in a model scale fluidized bed
Vollmari, K. and Jasevičius, R. and Kruggel-Emden, H.
POWDER TECHNOLOGY. Volume: 291 (2016) - 2016 • 3917
Carbon Materials for Lithium Sulfur Batteries - Ten Critical Questions
Borchardt, L. and Oschatz, M. and Kaskel, S.
CHEMISTRY - A EUROPEAN JOURNAL. Volume: 22 (2016) - 2016 • 3916
Interlayer expansion using metal-linker units: Crystalline microporous silicate zeolites with metal centers on specific framework sites
Gies, H. and Feyen, M. and De Baerdemaeker, T. and De Vos, D.E. and Yilmaz, B. and Müller, U. and Meng, X. and Xiao, F.-S. and Zhang, W. and Yokoi, T. and Tatsumi, T. and Bao, X.
MICROPOROUS AND MESOPOROUS MATERIALS. Volume: 222 (2016) - 2016 • 3915
Dioxygen binding to Fe-MOF-74: Microscopic insights from periodic QM/MM calculations
Moeljadi, A.M.P. and Schmid, R. and Hirao, H.
CANADIAN JOURNAL OF CHEMISTRY. Volume: 94 (2016) - 2016 • 3914
Electrochemistry at single bimetallic nanoparticles - using nano impacts for sizing and compositional analysis of individual AgAu alloy nanoparticles
Saw, E.N. and Grasmik, V. and Rurainsky, C. and Epple, M. and Tschulik, K.
FARADAY DISCUSSIONS. Volume: 193 (2016) - 2016 • 3913
Cyclic degradation in bamboo-like Fe-Mn-Al-Ni shape memory alloys - The role of grain orientation
Vollmer, M. and Krooß, P. and Kriegel, M.J. and Klemm, V. and Somsen, C. and Ozcan, H. and Karaman, I. and Weidner, A. and Rafaja, D. and Biermann, H. and Niendorf, T.
SCRIPTA MATERIALIA. Volume: 114 (2016) - 2016 • 3912
Illuminating solid gas storage in confined spaces-methane hydrate formation in porous model carbons
Borchardt, L. and Nickel, W. and Casco, M. and Senkovska, I. and Bon, V. and Wallacher, D. and Grimm, N. and Krause, S. and Silvestre-Albero, J.
PHYSICAL CHEMISTRY CHEMICAL PHYSICS. Volume: 18 (2016) - 2016 • 3911
Bimetallic nickel complexes for selective CO2 carbon capture and sequestration
Möller, F. and Merz, K. and Herrmann, C. and Apfel, U.-P.
DALTON TRANSACTIONS. Volume: 45 (2016) - 2016 • 3910
Partitioning of Cr and Si between cementite and ferrite derived from first-principles thermodynamics
Sawada, H. and Kawakami, K. and Körmann, F. and Grabowski, B. and Hickel, T. and Neugebauer, J.
ACTA MATERIALIA. Volume: 102 (2016) - 2016 • 3909
Use of small-angle X-ray scattering to resolve intracellular structure changes of Escherichia coli cells induced by antibiotic treatment
Von Gundlach, A.R. and Garamus, V.M. and Willey, T.M. and Ilavsky, J. and Hilpert, K. and Rosenhahn, A.
JOURNAL OF APPLIED CRYSTALLOGRAPHY. Volume: 49 (2016) - 2016 • 3908
Synthesis of nanostructured LiMn2O4 thin films by glancing angle deposition for Li-ion battery applications
Borhani-Haghighi, S. and Khare, C. and Trócoli, R. and Dushina, A. and Kieschnick, M. and Lamantia, F. and Ludwig, Al.
NANOTECHNOLOGY. Volume: 27 (2016) - 2016 • 3907
Small angle X-ray scattering as a high-throughput method to classify antimicrobial modes of action
Von Gundlach, A.R. and Garamus, V.M. and Gorniak, T. and Davies, H.A. and Reischl, M. and Mikut, R. and Hilpert, K. and Rosenhahn, A.
BIOCHIMICA ET BIOPHYSICA ACTA - BIOMEMBRANES. Volume: 1858 (2016) - 2016 • 3906
Quantization and anomalous structures in the conductance of Si/SiGe quantum point contacts
Von Pock, J.F. and Salloch, D. and Qiao, G. and Wieser, U. and Hackbarth, T. and Kunze, U.
JOURNAL OF APPLIED PHYSICS. Volume: 119 (2016) - 2016 • 3905
Microstructure design of tempered martensite by atomistically informed full-field simulation: From quenching to fracture
Borukhovich, E. and Du, G. and Stratmann, M. and Boeff, M. and Shchyglo, O. and Hartmaier, A. and Steinbach, I.
MATERIALS. Volume: 9 (2016) - 2016 • 3904
Experimentation for char combustion kinetics measurements: Bias from char preparation
Vorobiev, N. and Geier, M. and Schiemann, M. and Scherer, V.
FUEL PROCESSING TECHNOLOGY. Volume: 151 (2016) - 2016 • 3903
Exceptional damage-tolerance of a medium-entropy alloy CrCoNi at cryogenic temperatures
Gludovatz, B. and Hohenwarter, A. and Thurston, K.V.S. and Bei, H. and Wu, Z. and George, E.P. and Ritchie, R.O.
NATURE COMMUNICATIONS. Volume: 7 (2016) - 2016 • 3902
A coupled dissipation functional for modeling the functional fatigue in polycrystalline shape memory alloys
Waimann, J. and Junker, P. and Hackl, K.
EUROPEAN JOURNAL OF MECHANICS, A/SOLIDS. Volume: 55 (2016) - 2016 • 3901
Computational modeling of dual-phase steels based on representative three-dimensional microstructures obtained from EBSD data
Brands, D. and Balzani, D. and Scheunemann, L. and Schröder, J. and Richter, H. and Raabe, D.
ARCHIVE OF APPLIED MECHANICS. Volume: 86 (2016) - 2016 • 3900
Quantile spectral processes: Asymptotic analysis and inference
Kley, T. and Volgushev, S. and Dette, H. and Hallin, M.
BERNOULLI. Volume: 22 (2016) - 2016 • 3899
Autofluorescence imaging in recurrent oral squamous cell carcinoma
Scheer, M. and Fuss, J. and Derman, M.A. and Kreppel, M. and Neugebauer, J. and Rothamel, D. and Drebber, U. and Zoeller, J.E.
ORAL AND MAXILLOFACIAL SURGERY. Volume: 20 (2016) - 2016 • 3898
Near Net Shaping of Monolithic and Composite MAX Phases by Injection Molding
Gonzalez-Julian, J. and Classen, L. and Bram, M. and Vaßen, R. and Guillon, O.
JOURNAL OF THE AMERICAN CERAMIC SOCIETY. Volume: 99 (2016) - 2016 • 3897
From single cells to single molecules: general discussion
Gooding, J. and Magnussen, O. and Fermin, D. and Crooks, R. and Kanoufi, F. and Schuhmann, W. and Nichols, R. and Schmickler, W. and Tao, N. and Chen, S. and Actis, P. and Page, A. and Tschulik, K. and Faez, S. and Edwards, M. and Johnson, R. and Nogala, W. and Kranz, C. and Eikerling, M. and Unwin, P. and Thomas, B. and Prabhakaran, V. and Clausmeyer, J. and Vincent, K. and Koper, M. and Tian, Z. and Mount, A. and Alpuche-Aviles, M.A. and White, H. and Ewing, A. and Higgins, S. and Baker, L. and Zhan, D. and Ulstrup, J. and Bohn, P.W. and Lemay, S.
FARADAY DISCUSSIONS. Volume: 193 (2016) - 2016 • 3896
Few-layer graphene modified with nitrogen-rich metallo-macrocyclic complexes as precursor for bifunctional oxygen electrocatalysts
Morales, D.M. and Masa, J. and Andronescu, C. and Kayran, Y.U. and Sun, Z. and Schuhmann, W.
ELECTROCHIMICA ACTA. Volume: 222 (2016) - 2016 • 3895
Large laser spots and fault sensitivity analysis
Schellenberg, F. and Finkeldey, M. and Gerhardt, N. and Hofmann, M. and Moradi, A. and Paar, C.
PROCEEDINGS OF THE 2016 IEEE INTERNATIONAL SYMPOSIUM ON HARDWARE ORIENTED SECURITY AND TRUST, HOST 2016. Volume: (2016) - 2016 • 3894
First-principles modeling of energetic and mechanical properties of Ni-Cr, Ni-Re and Cr-Re random alloys
Breidi, A. and Fries, S.G. and Palumbo, M. and Ruban, A.V.
COMPUTATIONAL MATERIALS SCIENCE. Volume: 117 (2016) - 2016 • 3893
How van der waals interactions determine the unique properties of water
Morawietz, T. and Singraber, A. and Dellago, C. and Behler, J.
PROCEEDINGS OF THE NATIONAL ACADEMY OF SCIENCES OF THE UNITED STATES OF AMERICA. Volume: 113 (2016) - 2016 • 3892
Collective magnetic excitations of C4 -symmetric magnetic states in iron-based superconductors
Scherer, D.D. and Eremin, I. and Andersen, B.M.
PHYSICAL REVIEW B - CONDENSED MATTER AND MATERIALS PHYSICS. Volume: 94 (2016) - 2016 • 3891
Ideal compressive strength of fcc Co, Ni, and Ni-rich alloys along the (001) direction: A first-principles study
Breidi, A. and Fries, S.G. and Ruban, A.V.
PHYSICAL REVIEW B - CONDENSED MATTER AND MATERIALS PHYSICS. Volume: 93 (2016) - 2016 • 3890
Influence of carbonate decomposition on normal spectral radiative emittance in the context of oxyfuel combustion
Gorewoda, J. and Scherer, V.
ENERGY AND FUELS. Volume: 30 (2016) - 2016 • 3889
Devolatilization and volatiles reaction of individual coal particles in the context of FGM tabulated chemistry
Knappstein, R. and Kuenne, G. and Ketelheun, A. and Köser, J. and Becker, L. and Heuer, S. and Schiemann, M. and Scherer, V. and Dreizler, A. and Sadiki, A. and Janicka, J.
COMBUSTION AND FLAME. Volume: 169 (2016) - 2016 • 3888
Coupled DEM–CFD simulation of drying wood chips in a rotary drum – Baffle design and model reduction
Scherer, V. and Mönnigmann, M. and Berner, M.O. and Sudbrock, F.
FUEL. Volume: 184 (2016) - 2016 • 3887
Unraveling Self-Doping Effects in Thermoelectric TiNiSn Half-Heusler Compounds by Combined Theory and High-Throughput Experiments
Wambach, M. and Stern, R. and Bhattacharya, S. and Ziolkowski, P. and Muller, E. and Madsen, G. K. H. and Ludwig, Al.
ADVANCED ELECTRONIC MATERIALS. Volume: 2 (2016) - 2016 • 3886
A review on lithium combustion
Schiemann, M. and Bergthorson, J. and Fischer, P. and Scherer, V. and Taroata, D. and Schmid, G.
APPLIED ENERGY. Volume: 162 (2016) - 2016 • 3885
First-principles study of carbon segregation in bcc iron symmetrical tilt grain boundaries
Wang, J. and Janisch, R. and Madsen, G.K.H. and Drautz, R.
ACTA MATERIALIA. Volume: 115 (2016) - 2016 • 3884
Thermal shift of the resonance between an electron gas and quantum dots: What is the origin?
Brinks, F. and Wieck, A.D. and Ludwig, Ar.
NEW JOURNAL OF PHYSICS. Volume: 18 (2016) - 2016 • 3883
Multiple mechanisms of lath martensite plasticity
Morsdorf, L. and Jeannin, O. and Barbier, D. and Mitsuhara, M. and Raabe, D. and Tasan, C.C.
ACTA MATERIALIA. Volume: 121 (2016) - 2016 • 3882
Spectral TRIP enables ductile 1.1 GPa martensite
Wang, M.-M. and Tasan, C.C. and Ponge, D. and Raabe, D.
ACTA MATERIALIA. Volume: 111 (2016) - 2016 • 3881
Continuous synthesis of nanostructured silica based materials in a gas-liquid segmented flow tubular reactor
Knossalla, J. and Mezzavilla, S. and Schüth, F.
NEW JOURNAL OF CHEMISTRY. Volume: 40 (2016) - 2016 • 3880
Phase selection and nanocrystallization in Cu-free soft magnetic FeSiNbB amorphous alloy upon rapid annealing
Morsdorf, L. and Pradeep, K.G. and Herzer, G. and Kovács, A. and Dunin-Borkowski, R.E. and Povstugar, I. and Konygin, G. and Choi, P. and Raabe, D.
JOURNAL OF APPLIED PHYSICS. Volume: 119 (2016) - 2016 • 3879
Nanostructured Ti-Ta thin films synthesized by combinatorial glancing angle sputter deposition
Motemani, Y. and Khare, C. and Savan, A. and Hans, M. and Paulsen, A. and Frenzel, J. and Somsen, C. and Mücklich, F. and Eggeler, G. and Ludwig, Al.
NANOTECHNOLOGY. Volume: 27 (2016) - 2016 • 3878
The Temperature-Programmed Desorption of H2 from Cu/ZrO2
Schittkowski, J. and Buesen, D. and Toelle, K. and Muhler, M.
CATALYSIS LETTERS. Volume: 146 (2016) - 2016 • 3877
Co3O4 Nanoparticles Supported on Mesoporous Carbon for Selective Transfer Hydrogenation of α,β-Unsaturated Aldehydes
Wang, G.-H. and Deng, X. and Gu, D. and Chen, K. and Tüysüz, H. and Spliethoff, B. and Bongard, H.-J. and Weidenthaler, C. and Schmidt, W. and Schüth, F.
ANGEWANDTE CHEMIE - INTERNATIONAL EDITION. Volume: 55 (2016) - 2016 • 3876
Enhancement of the Superconducting Gap by Nesting in CaKFe4As4: A New High Temperature Superconductor
Mou, D. and Kong, T. and Meier, W.R. and Lochner, F. and Wang, L.-L. and Lin, Q. and Wu, Y. and Bud'Ko, S.L. and Eremin, I. and Johnson, D.D. and Canfield, P.C. and Kaminski, A.
PHYSICAL REVIEW LETTERS. Volume: 117 (2016) - 2016 • 3875
Nitrogen-Doped Ordered Mesoporous Carbon Supported Bimetallic PtCo Nanoparticles for Upgrading of Biophenolics
Wang, G.-H. and Cao, Z. and Gu, D. and Pfänder, N. and Swertz, A.-C. and Spliethoff, B. and Bongard, H.-J. and Weidenthaler, C. and Schmidt, W. and Rinaldi, R. and Schüth, F.
ANGEWANDTE CHEMIE - INTERNATIONAL EDITION. Volume: 55 (2016) - 2016 • 3874
Characterization of azimuthal and radial velocity fields induced by rotors in flows with a low Reynolds number
Köhler, J. and Friedrich, J. and Ostendorf, A. and Gurevich, E.L.
PHYSICAL REVIEW E - STATISTICAL, NONLINEAR, AND SOFT MATTER PHYSICS. Volume: 93 (2016) - 2016 • 3873
Discovery of an Unconventional Charge Density Wave at the Surface of K0.9Mo6 O17
Mou, D. and Sapkota, A. and Kung, H.-H. and Krapivin, V. and Wu, Y. and Kreyssig, A. and Zhou, X. and Goldman, A.I. and Blumberg, G. and Flint, R. and Kaminski, A.
PHYSICAL REVIEW LETTERS. Volume: 116 (2016) - 2016 • 3872
In Situ EPR Study of the Redox Properties of CuO-CeO2 Catalysts for Preferential CO Oxidation (PROX)
Wang, F. and Büchel, R. and Savitsky, A. and Zalibera, M. and Widmann, D. and Pratsinis, S.E. and Lubitz, W. and Schüth, F.
ACS CATALYSIS. Volume: 6 (2016) - 2016 • 3871
Mechanochemical polymerization-controlling a polycondensation reaction between a diamine and a dialdehyde in a ball mill
Grätz, S. and Borchardt, L.
RSC ADVANCES. Volume: 6 (2016) - 2016 • 3870
Optical screw-wrench for interlocking 2PP-microstructures
Köhler, J. and Zyla, G. and Ksouri, S.I. and Esen, C. and Ostendorf, A.
PROCEEDINGS OF SPIE - THE INTERNATIONAL SOCIETY FOR OPTICAL ENGINEERING. Volume: 9764 (2016) - 2016 • 3869
Complex phase diagram of Ba1-xNaxFe2As2: A multitude of phases striving for the electronic entropy
Wang, L. and Hardy, F. and Böhmer, A.E. and Wolf, T. and Schweiss, P. and Meingast, C.
PHYSICAL REVIEW B. Volume: 93 (2016) - 2016 • 3868
Performance testing of a mid-infrared spectroscopic system for clinical chemistry applications utilising an ultra-broadband tunable EC-QCL radiation source
Grafen, M. and Nalpantidis, K. and Ihrig, D. and Heise, H.M. and Ostendorf, A.
PROGRESS IN BIOMEDICAL OPTICS AND IMAGING - PROCEEDINGS OF SPIE. Volume: 9715 (2016) - 2016 • 3867
A differential scanning calorimetric study of carbide transition in 10cr tempered martensitic steels
Wang, H.C.
MATERIALS RESEARCH SOCIETY SYMPOSIUM PROCEEDINGS. Volume: 1754 (2016) - 2016 • 3866
Characterization of a multi-module tunable EC-QCL system for mid-infrared biofluid spectroscopy for hospital use and personalized diabetes technology
Grafen, M. and Nalpantidis, K. and Ostendorf, A. and Ihrig, D. and Heise, H.M.
PROGRESS IN BIOMEDICAL OPTICS AND IMAGING - PROCEEDINGS OF SPIE. Volume: 9715 (2016) - 2016 • 3865
Influence of magnetic excitations on the phase stability of metals and steels
Körmann, F. and Hickel, T. and Neugebauer, J.
CURRENT OPINION IN SOLID STATE AND MATERIALS SCIENCE. Volume: 20 (2016) - 2016 • 3864
Experimental methods for investigation of shape memory based elastocaloric cooling processes and model validation
Schmidt, M. and Ullrich, J. and Wieczorek, A. and Frenzel, J. and Eggeler, G. and Schütze, A. and Seelecke, S.
JOURNAL OF VISUALIZED EXPERIMENTS. Volume: 2016 (2016) - 2016 • 3863
Analytical and numerical modelling of a sub- and supersonic moving load front along a rod’s skin
Weber, W.E. and Fangye, Y.F. and Balzani, D. and Zastrau, B.W.
ADVANCED STRUCTURED MATERIALS. Volume: 60 (2016) - 2016 • 3862
Impact of magnetic fluctuations on lattice excitations in fcc nickel
Körmann, F. and Ma, P.-W. and Dudarev, S.L. and Neugebauer, J.
JOURNAL OF PHYSICS CONDENSED MATTER. Volume: 28 (2016) - 2016 • 3861
Relaxed incremental variational approach for the modeling of damage-induced stress hysteresis in arterial walls
Schmidt, T. and Balzani, D.
JOURNAL OF THE MECHANICAL BEHAVIOR OF BIOMEDICAL MATERIALS. Volume: 58 (2016) - 2016 • 3860
Tuning the Cell Adhesion on Biofunctionalized Nanoporous Organic Frameworks
Schmitt, S. and Hümmer, J. and Kraus, S. and Welle, A. and Grosjean, S. and Hanke-Roos, M. and Rosenhahn, A. and Bräse, S. and Wöll, C. and Lee-Thedieck, C. and Tsotsalas, M.
ADVANCED FUNCTIONAL MATERIALS. Volume: 26 (2016) - 2016 • 3859
Controlling the Photocorrosion of Zinc Sulfide Nanoparticles in Water by Doping with Chloride and Cobalt Ions
Weide, P. and Schulz, K. and Kaluza, S. and Rohe, M. and Beranek, R. and Muhler, M.
LANGMUIR. Volume: 32 (2016) - 2016 • 3858
FTIR spectroscopy of cysteine as a ready-to-use method for the investigation of plasma-induced chemical modifications of macromolecules
Kogelheide, F. and Kartaschew, K. and Strack, M. and Baldus, S. and Metzler-Nolte, N. and Havenith, M. and Awakowicz, P. and Stapelmann, K. and Lackmann, J.-W.
JOURNAL OF PHYSICS D: APPLIED PHYSICS. Volume: 49 (2016) - 2016 • 3857
Scanning micromirror for large, quasi-static 2D-deflections based on electrostatic driven rotation of a hemisphere
Bunge, F. and Leopold, S. and Bohm, S. and Hoffmann, M.
SENSORS AND ACTUATORS, A: PHYSICAL. Volume: 243 (2016) - 2016 • 3856
MEMS gas ionization sensor with palladium nanostructures for use at ambient pressure
Müller, L. and Mehner, H. and Hoffmann, M.
JOURNAL OF PHYSICS: CONFERENCE SERIES. Volume: 757 (2016) - 2016 • 3855
Adsorption of Helium Atoms on Two-Dimensional Substrates
Burganova, R. and Lysogorskiy, Y. and Nedopekin, O. and Tayurskii, D.
JOURNAL OF LOW TEMPERATURE PHYSICS. Volume: 185 (2016) - 2016 • 3854
On the Effect of Hot Isostatic Pressing on the Creep Life of a Single Crystal Superalloys
Mujica Roncery, L. and Lopez-Galilea, I. and Ruttert, B. and Bürger, D. and Wollgramm, P. and Eggeler, G. and Theisen, W.
ADVANCED ENGINEERING MATERIALS. Volume: 18 (2016) - 2016 • 3853
Linearized control of an uniaxial micromirror with electrostatic parallel-plate actuation
Weinberger, S. and Nguyen, T.T. and Lecomte, R. and Cheriguen, Y. and Ament, C. and Hoffmann, M.
MICROSYSTEM TECHNOLOGIES. Volume: 22 (2016) - 2016 • 3852
Electron spin dynamics in cubic GaN
Buß, J.H. and Schupp, T. and As, D.J. and Brandt, O. and Hägele, D. and Rudolph, J.
PHYSICAL REVIEW B - CONDENSED MATTER AND MATERIALS PHYSICS. Volume: 94 (2016) - 2016 • 3851
Scanning electrochemical microscopy: Visualization of local electrocatalytic activity of transition metals hexacyanoferrates
Komkova, M.A. and Maljusch, A. and Sliozberg, K. and Schuhmann, W. and Karyakin, A.A.
RUSSIAN JOURNAL OF ELECTROCHEMISTRY. Volume: 52 (2016) - 2016 • 3850
Electronic hybridisation implications for the damage-tolerance of thin film metallic glasses
Schnabel, V. and Jaya, B.N. and Köhler, M. and Music, D. and Kirchlechner, C. and Dehm, G. and Raabe, D. and Schneider, J.M.
SCIENTIFIC REPORTS. Volume: 6 (2016) - 2016 • 3849
Optical manipulation of a multilevel nuclear spin in ZnO: Master equation and experiment
Buß, J.H. and Rudolph, J. and Wassner, T.A. and Eickhoff, M. and Hägele, D.
PHYSICAL REVIEW B - CONDENSED MATTER AND MATERIALS PHYSICS. Volume: 93 (2016) - 2016 • 3848
Revealing the relationships between chemistry, topology and stiffness of ultrastrong Co-based metallic glass thin films: A combinatorial approach
Schnabel, V. and Köhler, M. and Evertz, S. and Gamcova, J. and Bednarcik, J. and Music, D. and Raabe, D. and Schneider, J.M.
ACTA MATERIALIA. Volume: 107 (2016) - 2016 • 3847
Strain hardening by dynamic slip band refinement in a high-Mn lightweight steel
Welsch, E. and Ponge, D. and Hafez Haghighat, S.M. and Sandlöbes, S. and Choi, P. and Herbig, M. and Zaefferer, S. and Raabe, D.
ACTA MATERIALIA. Volume: 116 (2016) - 2016 • 3846
MoSSe@reduced graphene oxide nanocomposite heterostructures as efficient and stable electrocatalysts for the hydrogen evolution reaction
Konkena, B. and Masa, J. and Xia, W. and Muhler, M. and Schuhmann, W.
NANO ENERGY. Volume: 29 (2016) - 2016 • 3845
Investigations on the Initial Stress Evolution During Atmospheric Plasma Spraying of YSZ by In Situ Curvature Measurement
Mutter, M. and Mauer, G. and Mücke, R. and Vaßen, R. and Back, H.C. and Gibmeier, J.
JOURNAL OF THERMAL SPRAY TECHNOLOGY. Volume: 25 (2016) - 2016 • 3844
Domain wall dynamics of periodic magnetic domain patterns in Co2MnGe-Heusler microstripes
Gross, K. and Westerholt, K. and Zabel, H.
NEW JOURNAL OF PHYSICS. Volume: 18 (2016) - 2016 • 3843
Pentlandite rocks as sustainable and stable efficient electrocatalysts for hydrogen generation
Konkena, B. and Puring, K.J. and Sinev, I. and Piontek, S. and Khavryuchenko, O. and Dürholt, J.P. and Schmid, R. and Tüysüz, H. and Muhler, M. and Schuhmann, W. and Apfel, U.-P.
NATURE COMMUNICATIONS. Volume: 7 (2016) - 2016 • 3842
Response to "Comment on 'Viscous coalescence of droplets: A lattice Boltzmann study'" [Phys. Fluids 28, 079101 (2016)]
Gross, M. and Steinbach, I. and Raabe, D. and Varnik, F.
PHYSICS OF FLUIDS. Volume: 28 (2016) - 2016 • 3841
Methods of gas purification and effect on the ion composition in an RF atmospheric pressure plasma jet investigated by mass spectrometry
Grosse-Kreul, S. and Hubner, S. and Schneider, S. and von Keudell, A. and Benedikt, J.
EPJ TECHNIQUES AND INSTRUMENTATION. Volume: 3 (2016) - 2016 • 3840
Heat Transfer Experiments in a Rotary Drum for a Variety of Granular Materials
Nafsun, A.I. and Herz, F. and Specht, E. and Scherer, V. and Wirtz, S.
EXPERIMENTAL HEAT TRANSFER. Volume: 29 (2016) - 2016 • 3839
Assessment of strain hardening in copper single crystals using in situ SEM microshear experiments
Wieczorek, N. and Laplanche, G. and Heyer, J.-K. and Parsa, A.B. and Pfetzing-Micklich, J. and Eggeler, G.
ACTA MATERIALIA. Volume: 113 (2016) - 2016 • 3838
Sampling of ions at atmospheric pressure: ion transmission and ion energy studied by simulation and experiment
Gross-Kreul, S. and Hubner, S. and Benedikt, J. and von Keudell, A.
EUROPEAN PHYSICAL JOURNAL D. Volume: 70 (2016) - 2016 • 3837
Production yield of rare-earth ions implanted into an optical crystal
Kornher, T. and Xia, K. and Kolesov, R. and Kukharchyk, N. and Reuter, R. and Siyushev, P. and Stöhr, R. and Schreck, M. and Becker, H.-W. and Villa, B. and Wieck, A.D. and Wrachtrup, J.
APPLIED PHYSICS LETTERS. Volume: 108 (2016) - 2016 • 3836
Experimental and modelling characterisation of residual stresses in cylindrical samples of rapidly cooled bulk metallic glass
Korsunsky, A.M. and Sui, T. and Salvati, E. and George, E.P. and Sebastiani, M.
MATERIALS AND DESIGN. Volume: 104 (2016) - 2016 • 3835
Screening of material libraries for electrochemical CO2 reduction catalysts – Improving selectivity of Cu by mixing with Co
Grote, J.-P. and Zeradjanin, A.R. and Cherevko, S. and Savan, A. and Breitbach, B. and Ludwig, Al. and Mayrhofer, K.J.J.
JOURNAL OF CATALYSIS. Volume: 343 (2016) - 2016 • 3834
Direction-dependent mechanical characterization of cellulose-based composite vulcanized fiber
Scholz, R. and Mittendorf, R. M. and Engels, J. K. and Hartmaier, A. and Kunne, B. and Walther, F.
MATERIALS TESTING. Volume: 58 (2016) - 2016 • 3833
Strong cooperative coupling of pressure-induced magnetic order and nematicity in FeSe
Kothapalli, K. and Böhmer, A.E. and Jayasekara, W.T. and Ueland, B.G. and Das, P. and Sapkota, A. and Taufour, V. and Xiao, Y. and Alp, E. and Bud'ko, S.L. and Canfield, P.C. and Kreyssig, A. and Goldman, A.I.
NATURE COMMUNICATIONS. Volume: 7 (2016) - 2016 • 3832
Magnetic microstructure in a stress-annealed Fe73.5Si15.5B7Nb3Cu1 soft magnetic alloy observed using off-axis electron holography and Lorentz microscopy
Kovács, A. and Pradeep, K.G. and Herzer, G. and Raabe, D. and Dunin-Borkowski, R.E.
AIP ADVANCES. Volume: 6 (2016) - 2016 • 3831
Neural network molecular dynamics simulations of solid-liquid interfaces: Water at low-index copper surfaces
Natarajan, S.K. and Behler, J.
PHYSICAL CHEMISTRY CHEMICAL PHYSICS. Volume: 18 (2016) - 2016 • 3830
Model selection versus model averaging in dose finding studies
Schorning, K. and Bornkamp, B. and Bretz, F. and Dette, H.
STATISTICS IN MEDICINE. Volume: 35 (2016) - 2016 • 3829
DEM/CFD modeling of the fuel conversion in a pellet stove
Wiese, J. and Wissing, F. and Höhner, D. and Wirtz, S. and Scherer, V. and Ley, U. and Behr, H.M.
FUEL PROCESSING TECHNOLOGY. Volume: 152 (2016) - 2016 • 3828
Hydrogen-assisted damage in austenite/martensite dual-phase steel
Koyama, M. and Tasan, C.C. and Nagashima, T. and Akiyama, E. and Raabe, D. and Tsuzaki, K.
PHILOSOPHICAL MAGAZINE LETTERS. Volume: 96 (2016) - 2016 • 3827
Phase Formation and Oxidation Behavior at 500 °c in a Ni-Co-Al Thin-Film Materials Library
Naujoks, D. and Richert, J. and Decker, P. and Weiser, M. and Virtanen, S. and Ludwig, Al.
ACS COMBINATORIAL SCIENCE. Volume: 18 (2016) - 2016 • 3826
Zeolite Beta Formation from Clear Sols: Silicate Speciation, Particle Formation and Crystallization Monitored by Complementary Analysis Methods
Castro, M. and Haouas, M. and Lim, I. and Bongard, H.J. and Schüth, F. and Taulelle, F. and Karlsson, G. and Alfredsson, V. and Breyneart, E. and Kirschhock, C.E.A. and Schmidt, W.
CHEMISTRY - A EUROPEAN JOURNAL. Volume: 22 (2016) - 2016 • 3825
On the rich magnetic phase diagram of (Ni, Co)-Mn-Sn Heusler alloys
Grünebohm, A. and Herper, H.C. and Entel, P.
JOURNAL OF PHYSICS D: APPLIED PHYSICS. Volume: 49 (2016) - 2016 • 3824
Spectroscopic studies of microwave plasmas containing hexamethyldisiloxane
Nave, A.S.C. and Mitschker, F. and Awakowicz, P. and Röpcke, J.
JOURNAL OF PHYSICS D: APPLIED PHYSICS. Volume: 49 (2016) - 2016 • 3823
Kinetic interpretation of resonance phenomena in low pressure capacitively coupled radio frequency plasmas
Wilczek, S. and Trieschmann, J. and Eremin, D. and Brinkmann, R.P. and Schulze, J. and Schuengel, E. and Derzsi, A. and Korolov, I. and Hartmann, P. and Donkó, Z. and Mussenbrock, T.
PHYSICS OF PLASMAS. Volume: 23 (2016) - 2016 • 3822
Strong discontinuity approaches: An algorithm for robust performance and comparative assessment of accuracy
Cazes, F. and Meschke, G. and Zhou, M.-M.
INTERNATIONAL JOURNAL OF SOLIDS AND STRUCTURES. Volume: 96 (2016) - 2016 • 3821
Tuning the caloric response of BaTiO3 by tensile epitaxial strain
Grünebohm, A. and Marathe, M. and Ederer, C.
EPL. Volume: 115 (2016) - 2016 • 3820
Unraveling the temperature dependence of the yield strength in single-crystal tungsten using atomistically-informed crystal plasticity calculations
Cereceda, D. and Diehl, M. and Roters, F. and Raabe, D. and Perlado, J.M. and Marian, J.
INTERNATIONAL JOURNAL OF PLASTICITY. Volume: 78 (2016) - 2016 • 3819
Influence of defects on ferroelectric and electrocaloric properties of BaTiO3
Grünebohm, A. and Nishimatsu, T.
PHYSICAL REVIEW B - CONDENSED MATTER AND MATERIALS PHYSICS. Volume: 93 (2016) - 2016 • 3818
Thermal energy and charge currents in multi-terminal nanorings
Kramer, T. and Kreisbeck, C. and Riha, C. and Chiatti, O. and Buchholz, S.S. and Wieck, A.D. and Reuter, D. and Fischer, S.F.
AIP ADVANCES. Volume: 6 (2016) - 2016 • 3817
A review of thermal history and timescales of tectonometamorphic processes in Sikkim Himalaya (NE India) and implications for rates of metamorphic processes
Chakraborty, S. and Anczkiewicz, R. and Gaidies, F. and Rubatto, D. and Sorcar, N. and Faak, K. and Mukhopadhyay, D.K. and Dasgupta, S.
JOURNAL OF METAMORPHIC GEOLOGY. Volume: 34 (2016) - 2016 • 3816
First-principles calculation of the elastic dipole tensor of a point defect: Application to hydrogen in α -zirconium
Nazarov, R. and Majevadia, J.S. and Patel, M. and Wenman, M.R. and Balint, D.S. and Neugebauer, J. and Sutton, A.P.
PHYSICAL REVIEW B - CONDENSED MATTER AND MATERIALS PHYSICS. Volume: 94 (2016) - 2016 • 3815
A novel mixed finite element for finite anisotropic elasticity; the SKA-element Simplified Kinematics for Anisotropy
Schröder, J. and Viebahn, N. and Balzani, D. and Wriggers, P.
COMPUTER METHODS IN APPLIED MECHANICS AND ENGINEERING. Volume: 310 (2016) - 2016 • 3814
Tool steel with improved properties for hot stamping tools
Wilzer, J.J. and Escher, C. and Kotzian, M. and Weber, S. and Theisen, W.
HTM - JOURNAL OF HEAT TREATMENT AND MATERIALS. Volume: 71 (2016) - 2016 • 3813
Development of Protective Coating of MoSi2 over TZM Alloy Substrate by Slurry Coating Technique
Chakraborty, S.P.
MATERIALS TODAY: PROCEEDINGS. Volume: 3 (2016) - 2016 • 3812
Unraveling the composition dependence of the martensitic transformation temperature: A first-principles study of Ti-Ta alloys
Chakraborty, T. and Rogal, J. and Drautz, R.
PHYSICAL REVIEW B - CONDENSED MATTER AND MATERIALS PHYSICS. Volume: 94 (2016) - 2016 • 3811
Glaucoma and structure-based mechanics of the lamina cribrosa at multiple scales
Grytz, R. and Meschke, G. and Jonas, J.B. and Downs, J.C.
STRUCTURE-BASED MECHANICS OF TISSUES AND ORGANS. Volume: (2016) - 2016 • 3810
Hydrogen diffusion and trapping in Ti-modified advanced high strength steels
Winzer, N. and Rott, O. and Thiessen, R. and Thomas, I. and Mraczek, K. and Höche, T. and Wright, L. and Mrovec, M.
MATERIALS AND DESIGN. Volume: 92 (2016) - 2016 • 3809
A Vibrant Science Lives from Within
Schüth, F.
ANGEWANDTE CHEMIE - INTERNATIONAL EDITION. Volume: 55 (2016) - 2016 • 3808
Gold on Different Manganese Oxides: Ultra-Low-Temperature CO Oxidation over Colloidal Gold Supported on Bulk-MnO2 Nanomaterials
Gu, D. and Tseng, J.-C. and Weidenthaler, C. and Bongard, H.-J. and Spliethoff, B. and Schmidt, W. and Soulimani, F. and Weckhuysen, B.M. and Schüth, F.
JOURNAL OF THE AMERICAN CHEMICAL SOCIETY. Volume: 138 (2016) - 2016 • 3807
Phosphine-ligated dinitrosyl iron complexes for redox-controlled NO release
Wittkamp, F. and Nagel, C. and Lauterjung, P. and Mallick, B. and Schatzschneider, U. and Apfel, U.-P.
DALTON TRANSACTIONS. Volume: 45 (2016) - 2016 • 3806
Structure and dynamics of shear bands in amorphous-crystalline nanolaminates
Guo, W. and Gan, B. and Molina-Aldareguia, J.M. and Poplawsky, J.D. and Raabe, D.
SCRIPTA MATERIALIA. Volume: 110 (2016) - 2016 • 3805
Flow-regime transitions in fluidized beds of non-spherical particles
Kruggel-Emden, H. and Vollmari, K.
PARTICUOLOGY. Volume: 29 (2016) - 2016 • 3804
Low cycle fatigue in aluminum single and bi-crystals: On the influence of crystal orientation
Nellessen, J. and Sandlöbes, S. and Raabe, D.
MATERIALS SCIENCE AND ENGINEERING A. Volume: 668 (2016) - 2016 • 3803
Diagnostics of plasma processes based on parallelized spatially resolved in-situ reflection measurements
Schulz, C. and Runkel, J. and Oberberg, M. and Awakowicz, P. and Rolfes, I.
IEEE TRANSACTIONS ON MICROWAVE THEORY AND TECHNIQUES. Volume: 64 (2016) - 2016 • 3802
PKU-20: A new silicogermanate constructed from sti and asv layers
Chen, Y. and Su, J. and Huang, S. and Liang, J. and Lin, X. and Liao, F. and Sun, J. and Wang, Y. and Lin, J. and Gies, H.
MICROPOROUS AND MESOPOROUS MATERIALS. Volume: 224 (2016) - 2016 • 3801
Early-stage nucleation of manganese sulfide particle and its processing evolution in Fe—3wt.%Si alloys
Guo, W. and Meng, L. and Wang, H. and Yan, G. and Mao, W.
FRONTIERS OF MATERIALS SCIENCE. Volume: 10 (2016) - 2016 • 3800
Direct numerical simulation of coupled fluid flow and heat transfer for single particles and particle packings by a LBM-approach
Kruggel-Emden, H. and Kravets, B. and Suryanarayana, M.K. and Jasevicius, R.
POWDER TECHNOLOGY. Volume: 294 (2016) - 2016 • 3799
Multiscale description of carbon-supersaturated ferrite in severely drawn pearlitic wires
Nematollahi, Gh.A. and Grabowski, B. and Raabe, D. and Neugebauer, J.
ACTA MATERIALIA. Volume: 111 (2016) - 2016 • 3798
Formation and Effect of NH4 + Intermediates in NH3-SCR over Fe-ZSM-5 Zeolite Catalysts
Chen, P. and Jabłońska, M. and Weide, P. and Caumanns, T. and Weirich, T. and Muhler, M. and Moos, R. and Palkovits, R. and Simon, U.
ACS CATALYSIS. Volume: 6 (2016) - 2016 • 3797
Electron heating in technological plasmas
Schulze, J. and Mussenbrock, T.
PLASMA SOURCES SCIENCE AND TECHNOLOGY. Volume: 25 (2016) - 2016 • 3796
The effect of Cu and Fe cations on NH3-supported proton transport in DeNO:X-SCR zeolite catalysts
Chen, P. and Rauch, D. and Weide, P. and Schönebaum, S. and Simons, T. and Muhler, M. and Moos, R. and Simon, U.
CATALYSIS SCIENCE AND TECHNOLOGY. Volume: 6 (2016) - 2016 • 3795
Identification of modal parameters for complex structures by experimental modal analysis approach
Nestorović, T. and Trajkov, M. and Patalong, M.
ADVANCES IN MECHANICAL ENGINEERING. Volume: 8 (2016) - 2016 • 3794
The effect of stress, temperature and loading direction on the creep behaviour of Ni-base single crystal superalloy miniature tensile specimens
Wollgramm, P. and Bürger, D. and Parsa, A.B. and Neuking, K. and Eggeler, G.
MATERIALS AT HIGH TEMPERATURES. Volume: 33 (2016) - 2016 • 3793
Traditional earth-abundant coal as new energy materials to catalyze the oxygen reduction reaction in alkaline solution
Chen, X. and Huang, X. and Wang, T. and Barwe, S. and Xie, K. and Kayran, Y.U. and Wintrich, D. and Schuhmann, W. and Masa, J.
ELECTROCHIMICA ACTA. Volume: 211 (2016) - 2016 • 3792
Electric field distribution and exciton recombination line shape in GaAs
Schuster, J. and Kim, T. Y. and Batke, E. and Reuter, D. and Wieck, A. D.
MATERIALS RESEARCH EXPRESS. Volume: 3 (2016) - 2016 • 3791
Characterisation of bifunctional electrocatalysts for oxygen reduction and evolution by means of SECM
Chen, X. and Botz, A.J.R. and Masa, J. and Schuhmann, W.
JOURNAL OF SOLID STATE ELECTROCHEMISTRY. Volume: 20 (2016) - 2016 • 3790
Plastic accommodation at homophase interfaces between nanotwinned and recrystallized grains in an austenitic duplex-microstructured steel
Gutierrez-Urrutia, I. and Archie, F. and Raabe, D. and Yan, F.-K. and Tao, N.-R. and Lu, K.
SCIENCE AND TECHNOLOGY OF ADVANCED MATERIALS. Volume: 17 (2016) - 2016 • 3789
Electrical properties of carbon nanotubes / WS2 nanotubes (nanoparticles) hybrid films
Ksenevich, V. K. and Gorbachuk, N. I. and Viet, H. and Shuba, M. V. and Kuzhir, P. P. and Maksimenko, S. A. and Paddubskaya, A. G. and Valusis, G. and Wieck, A. D. and Zak, A. and Tenne, R.
NANOSYSTEMS-PHYSICS CHEMISTRY MATHEMATICS. Volume: 7 (2016) - 2016 • 3788
Impact of lattice dynamics on the phase stability of metamagnetic FeRh: Bulk and thin films
Wolloch, M. and Gruner, M.E. and Keune, W. and Mohn, P. and Redinger, J. and Hofer, F. and Suess, D. and Podloucky, R. and Landers, J. and Salamon, S. and Scheibel, F. and Spoddig, D. and Witte, R. and Roldan Cuenya, B. and Gutfleisch, O. and Hu, M.Y. and Zhao, J. and Toellner, T. and Alp, E.E. and Siewert, M. and Entel, P. and Pentcheva, R. and Wende, H.
PHYSICAL REVIEW B - CONDENSED MATTER AND MATERIALS PHYSICS. Volume: 94 (2016) - 2016 • 3787
A crystal plasticity model for twinning- and transformation-induced plasticity
Wong, S.L. and Madivala, M. and Prahl, U. and Roters, F. and Raabe, D.
ACTA MATERIALIA. Volume: 118 (2016) - 2016 • 3786
Phase-field study of zener drag and pinning of cylindrical particles in polycrystalline materials
Schwarze, C. and Darvishi Kamachali, R. and Steinbach, I.
ACTA MATERIALIA. Volume: 106 (2016) - 2016 • 3785
Defect Recovery in Severely Deformed Ferrite Lamellae During Annealing and Its Impact on the Softening of Cold-Drawn Pearlitic Steel Wires
Chen, Y.Z. and Csiszár, G. and Cizek, J. and Shi, X.H. and Borchers, C. and Li, Y.J. and Liu, F. and Kirchheim, R.
METALLURGICAL AND MATERIALS TRANSACTIONS A: PHYSICAL METALLURGY AND MATERIALS SCIENCE. Volume: 47 (2016) - 2016 • 3784
Electrochemically induced sol-gel deposition of ZnO films on Pt-nanoparticle modified FTO surfaces for enhanced photoelectrocatalytic energy conversion
Gutkowski, R. and Schuhmann, W.
PHYSICAL CHEMISTRY CHEMICAL PHYSICS. Volume: 18 (2016) - 2016 • 3783
Influence of the sensitivity of an optical resonator with a surface layer by its properties
Schweiger, G. and Weigel, T. and Ostendorf, A.
PROCEEDINGS OF SPIE - THE INTERNATIONAL SOCIETY FOR OPTICAL ENGINEERING. Volume: 9727 (2016) - 2016 • 3782
Improved photoelectrochemical performance of electrodeposited metal-doped BiVO4 on Pt-nanoparticle modified FTO surfaces
Gutkowski, R. and Peeters, D. and Schuhmann, W.
JOURNAL OF MATERIALS CHEMISTRY A. Volume: 4 (2016) - 2016 • 3781
Double minimum creep of single crystal Ni-base superalloys
Wu, X. and Wollgramm, P. and Somsen, C. and Dlouhy, A. and Kostka, A. and Eggeler, G.
ACTA MATERIALIA. Volume: 112 (2016) - 2016 • 3780
Phase-field simulation of liquid phase migration in the WC-Co system during liquid phase sintering
Cheng, K. and Zhang, L. and Schwarze, C. and Steinbach, I. and Du, Y.
INTERNATIONAL JOURNAL OF MATERIALS RESEARCH. Volume: 107 (2016) - 2016 • 3779
Terahertz wave generation from dual wavelength monolithic integrated distributed Bragg reflector semiconductor laser diode
Gwaro, J.O. and Brenner, C. and Hofmann, M.R. and Sumpf, B. and Klehr, A. and Fricke, J.
GEMIC 2016 - 2016 GERMAN MICROWAVE CONFERENCE. Volume: (2016) - 2016 • 3778
Diffusion of solutes in fcc Cobalt investigated by diffusion couples and first principles kinetic Monte Carlo
Neumeier, S. and Rehman, H.U. and Neuner, J. and Zenk, C.H. and Michel, S. and Schuwalow, S. and Rogal, J. and Drautz, R. and Göken, M.
ACTA MATERIALIA. Volume: 106 (2016) - 2016 • 3777
From insect scales to sensor design: Modelling the mechanochromic properties of bicontinuous cubic structures
Wu, X. and Ma, D. and Eisenlohr, P. and Raabe, D. and Fabritius, H.-O.
BIOINSPIRATION AND BIOMIMETICS. Volume: 11 (2016) - 2016 • 3776
Nuclear Quantum Effects in Water at the Triple Point: Using Theory as a Link between Experiments
Cheng, B. and Behler, J. and Ceriotti, M.
JOURNAL OF PHYSICAL CHEMISTRY LETTERS. Volume: 7 (2016) - 2016 • 3775
Nanoscale nonlinear effects in Erbium-implanted Yttrium Orthosilicate
Kukharchyk, N. and Shvarkov, S. and Probst, S. and Xia, K. and Becker, H.-W. and Pal, S. and Markmann, S. and Kolesov, R. and Siyushev, P. and Wrachtrup, J. and Ludwig, Ar. and Ustinov, A.V. and Wieck, A.D. and Bushev, P.
JOURNAL OF LUMINESCENCE. Volume: 177 (2016) - 2016 • 3774
Combining sensor and protective functionalities in ferromagnetic nanocomposite thin films for applications in harsh environments
Seemann, K. and Leiste, H. and Stüber, M. and Krüger, K. and Brunken, H. and Ludwig, Al. and Thede, C. and Quandt, E.
ADVANCED ENGINEERING MATERIALS. Volume: 18 (2016) - 2016 • 3773
Oxygen and hydrogen evolution reactions on Ru, RuO2, Ir, and IrO2 thin film electrodes in acidic and alkaline electrolytes: A comparative study on activity and stability
Cherevko, S. and Geiger, S. and Kasian, O. and Kulyk, N. and Grote, J.-P. and Savan, A. and Shrestha, B.R. and Merzlikin, S. and Breitbach, B. and Ludwig, Al. and Mayrhofer, K.J.J.
CATALYSIS TODAY. Volume: 262 (2016) - 2016 • 3772
Development of yttrium alloy ion source and its application in nanofabrication
Kukharchyk, N. and Neumann, R. and Mazarov, S. and Bushev, P. and Wieck, A.D. and Mazarov, P.
APPLIED PHYSICS A: MATERIALS SCIENCE AND PROCESSING. Volume: 122 (2016) - 2016 • 3771
Role of the electron spin in determining the coherence of the nuclear spins in a quantum dot
Wüst, G. and Munsch, M. and Maier, F. and Kuhlmann, A.V. and Ludwig, Ar. and Wieck, A.D. and Loss, D. and Poggio, M. and Warburton, R.J.
NATURE NANOTECHNOLOGY. Volume: 11 (2016) - 2016 • 3770
Dynamics of double-pulse photoacoustic excitation
Cherkashin, M. and Brenner, C. and Göring, L. and Döpke, B. and Gerhardt, N.C. and Hofmann, M.R.
PROGRESS IN BIOMEDICAL OPTICS AND IMAGING - PROCEEDINGS OF SPIE. Volume: 9708 (2016) - 2016 • 3769
Unscented hybrid simulated annealing for fast inversion of tunnel seismic waves
Nguyen, L.T. and Nestorović, T.
COMPUTER METHODS IN APPLIED MECHANICS AND ENGINEERING. Volume: 301 (2016) - 2016 • 3768
On the role of the stability of functional groups in multi-walled carbon nanotubes applied as support in iron-based high-temperature Fischer-Tropsch synthesis
Chew, L.M. and Xia, W. and Düdder, H. and Weide, P. and Ruland, H. and Muhler, M.
CATALYSIS TODAY. Volume: 270 (2016) - 2016 • 3767
Atomistic migration mechanisms of atomically flat, stepped, and kinked grain boundaries
Hadian, R. and Grabowski, B. and Race, C.P. and Neugebauer, J.
PHYSICAL REVIEW B - CONDENSED MATTER AND MATERIALS PHYSICS. Volume: 94 (2016) - 2016 • 3766
Nonlinear Kalman Filters for Model Calibration of Soil Parameters for Geomechanical Modeling in Mechanized Tunneling
Nguyen, L.T. and NestoroviC, T.
JOURNAL OF COMPUTING IN CIVIL ENGINEERING. Volume: 30 (2016) - 2016 • 3765
Probing the Dynamic Structure and Chemical State of Au Nanocatalysts during the Electrochemical Oxidation of 2-Propanol
Choi, Y. and Sinev, I. and Mistry, H. and Zegkinoglou, I. and Roldan Cuenya, B.
ACS CATALYSIS. Volume: 6 (2016) - 2016 • 3764
Sorption measurements for determining surface effects and structure of solid fuels
Seibel, C. and Wedler, C. and Vorobiev, N. and Schiemann, M. and Scherer, V. and Span, R. and Fieback, T.M.
FUEL PROCESSING TECHNOLOGY. Volume: 153 (2016) - 2016 • 3763
Optical Blocking of Electron Tunneling into a Single Self-Assembled Quantum Dot
Kurzmann, A. and Merkel, B. and Labud, P.A. and Ludwig, Ar. and Wieck, A.D. and Lorke, A. and Geller, M. P.
PHYSICAL REVIEW LETTERS. Volume: 117 (2016) - 2016 • 3762
Interactions between metal species and nitrogen-functionalized carbon nanotubes
Xia, W.
CATALYSIS SCIENCE AND TECHNOLOGY. Volume: 6 (2016) - 2016 • 3761
Photoelectron generation and capture in the resonance fluorescence of a quantum dot
Kurzmann, A. and Ludwig, Ar. and Wieck, A.D. and Lorke, A. and Geller, M.
APPLIED PHYSICS LETTERS. Volume: 108 (2016) - 2016 • 3760
Promoting effect of nitrogen doping on carbon nanotube-supported RuO2 applied in the electrocatalytic oxygen evolution reaction
Xie, K. and Xia, W. and Masa, J. and Yang, F. and Weide, P. and Schuhmann, W. and Muhler, M.
JOURNAL OF ENERGY CHEMISTRY. Volume: 25 (2016) - 2016 • 3759
Superlattice effect for enhanced fracture toughness of hard coatings
Hahn, R. and Bartosik, M. and Soler, R. and Kirchlechner, C. and Dehm, G. and Mayrhofer, P.H.
SCRIPTA MATERIALIA. Volume: 124 (2016) - 2016 • 3758
Auger Recombination in Self-Assembled Quantum Dots: Quenching and Broadening of the Charged Exciton Transition
Kurzmann, A. and Ludwig, Ar. and Wieck, A.D. and Lorke, A. and Geller, M.
NANO LETTERS. Volume: 16 (2016) - 2016 • 3757
Superconductivity versus bound-state formation in a two-band superconductor with small Fermi energy: Applications to Fe pnictides/chalcogenides and doped SrTiO3
Chubukov, A.V. and Eremin, I. and Efremov, D.V.
PHYSICAL REVIEW B - CONDENSED MATTER AND MATERIALS PHYSICS. Volume: 93 (2016) - 2016 • 3756
Molecular dynamics simulations of chemically disordered ferroelectric (Ba,Sr)TiO3 with a semi-empirical effective Hamiltonian
Nishimatsu, T. and Grünebohm, A. and Waghmare, U.V. and Kubo, M.
JOURNAL OF THE PHYSICAL SOCIETY OF JAPAN. Volume: 85 (2016) - 2016 • 3755
Improvement of catalytic activity over Cu–Fe modified Al-rich Beta catalyst for the selective catalytic reduction of NOx with NH3
Xu, L. and Shi, C. and Chen, B. and Zhao, Q. and Zhu, Y. and Gies, H. and Xiao, F.-S. and De Vos, D. and Yokoi, T. and Bao, X. and Kolb, U. and Feyen, M. and Maurer, S. and Moini, A. and Müller, U. and Zhang, W.
MICROPOROUS AND MESOPOROUS MATERIALS. Volume: 236 (2016) - 2016 • 3754
Coherent electron-spin-resonance manipulation of three individual spins in a triple quantum dot
Noiri, A. and Yoneda, J. and Nakajima, T. and Otsuka, T. and Delbecq, M.R. and Takeda, K. and Amaha, S. and Allison, G. and Ludwig, Ar. and Wieck, A.D. and Tarucha, S.
APPLIED PHYSICS LETTERS. Volume: 108 (2016) - 2016 • 3753
Atomic structure of the i- R -Cd quasicrystals and consequences for magnetism
Yamada, T. and Takakura, H. and Kong, T. and Das, P. and Jayasekara, W.T. and Kreyssig, A. and Beutier, G. and Canfield, P.C. and De Boissieu, M. and Goldman, A.I.
PHYSICAL REVIEW B. Volume: 94 (2016) - 2016 • 3752
A new mixed finite-element approach for the elastoplastic analysis of Mindlin plates
Kutlu, A. and Meschke, G. and Omurtag, M.H.
JOURNAL OF ENGINEERING MATHEMATICS. Volume: 99 (2016) - 2016 • 3751
Probabilistic lifetime model for atmospherically plasma sprayed thermal barrier coating systems
Nordhorn, C. and Mücke, R. and Mack, D.E. and Vaßen, R.
MECHANICS OF MATERIALS. Volume: 93 (2016) - 2016 • 3750
Elemental distributions within multiphase quaternary Pb chalcogenide thermoelectric materials determined through three-dimensional atom probe tomography
Yamini, A. and Li, T. and Mitchell, D.R.G. and Cairney, J.M.
NANO ENERGY. Volume: 26 (2016) - 2016 • 3749
Electrochemical bromination of organosulfur containing species for the determination of the strength of garlic (A. sativum)
Hall, E.M. and Tschulik, K. and Batchelor-McAuley, C. and Compton, R.G.
FOOD CHEMISTRY. Volume: 199 (2016) - 2016 • 3748
Kinetic passivation effect of localized differential aeration on brass
Kuznetsov, V. and Estrada-Vargas, A. and Maljusch, A. and Berkes, B.B. and Bandarenka, A.S. and Souto, R.M. and Schuhmann, W.
CHEMPLUSCHEM. Volume: 81 (2016) - 2016 • 3747
MoxC/CNT Composites as Active Electrocatalysts for the Hydrogen Evolution Reaction under Alkaline Conditions
Yang, F. and Sliozberg, K. and Antoni, H. and Xia, W. and Muhler, M.
ELECTROANALYSIS. Volume: 28 (2016) - 2016 • 3746
Measuring stationarity in long-memory processes
Sen, K. and Preuß, P. and Dette, H.
STATISTICA SINICA. Volume: 26 (2016) - 2016 • 3745
Ferrocene Aryl Derivatives for the Redox Tagging of Graphene Nanoplatelets
Yang, M. and Batchelor-Mcauley, C. and MoreiraGonçalves, L. and Lima, C.F.R.A.C. and Vyskočil, V. and Tschulik, K. and Compton, R.G.
ELECTROANALYSIS. Volume: 28 (2016) - 2016 • 3744
Multi-threaded construction of neighbour lists for particle systems in openMP
Halver, R. and Sutmann, G.
LECTURE NOTES IN COMPUTER SCIENCE (INCLUDING SUBSERIES LECTURE NOTES IN ARTIFICIAL INTELLIGENCE AND LECTURE NOTES IN BIOINFORMATICS). Volume: 9574 (2016) - 2016 • 3743
A combined low-pressure hydrogen peroxide evaporation plus hydrogen plasma treatment method for sterilization - Part 2: An intercomparison study of different biological systems
Lackmann, J.-W. and Fiebrandt, M. and Raguse, M. and Kartaschew, K. and Havenith, M. and Bandow, J.E. and Moeller, R. and Awakowicz, P. and Stapelmann, K.
PLASMA PROCESSES AND POLYMERS. Volume: 14 (2016) - 2016 • 3742
[FeFe]-Hydrogenase with Chalcogenide Substitutions at the H-Cluster Maintains Full H2Evolution Activity
Noth, J. and Esselborn, J. and Güldenhaupt, J. and Brünje, A. and Sawyer, A. and Apfel, U.-P. and Gerwert, K. and Hofmann, E. and Winkler, M. and Happe, T.
ANGEWANDTE CHEMIE - INTERNATIONAL EDITION. Volume: 55 (2016) - 2016 • 3741
Stepwise isotope editing of [FeFe]-hydrogenases exposes cofactor dynamics
Senger, M. and Mebs, S. and Duan, J. and Wittkamp, F. and Apfel, U.-P. and Heberle, J. and Haumann, M. and Stripp, S.T.
PROCEEDINGS OF THE NATIONAL ACADEMY OF SCIENCES OF THE UNITED STATES OF AMERICA. Volume: 113 (2016) - 2016 • 3740
Crystal-structure analysis with moments of the density-of-states: Application to intermetallic topologically close-packed phases
Hammerschmidt, T. and Ladines, A.N. and Koßmann, J. and Drautz, R.
CRYSTALS. Volume: 6 (2016) - 2016 • 3739
In vivo and in situ synchrotron radiation-based μ-XRF reveals elemental distributions during the early attachment phase of barnacle larvae and juvenile barnacles
Senkbeil, T. and Mohamed, T. and Simon, R. and Batchelor, D. and Di Fino, A. and Aldred, N. and Clare, A.S. and Rosenhahn, A.
ANALYTICAL AND BIOANALYTICAL CHEMISTRY. Volume: 408 (2016) - 2016 • 3738
Combined atom probe tomography and density functional theory investigation of the Al off-stoichiometry of κ-carbides in an austenitic Fe-Mn-Al-C low density steel
Yao, M.J. and Dey, P. and Seol, J.-B. and Choi, P. and Herbig, M. and Marceau, R.K.W. and Hickel, T. and Neugebauer, J. and Raabe, D.
ACTA MATERIALIA. Volume: 106 (2016) - 2016 • 3737
On the mechanism of {332} twinning in metastable β titanium alloys
Lai, M.J. and Tasan, C.C. and Raabe, D.
ACTA MATERIALIA. Volume: 111 (2016) - 2016 • 3736
Effects of CO2 on submicronic carbon particulate (soot) formed during coal pyrolysis in a drop tube reactor
Senneca, O. and Apicella, B. and Heuer, S. and Schiemann, M. and Scherer, V. and Stanzione, F. and Ciajolo, A. and Russo, C.
COMBUSTION AND FLAME. Volume: 172 (2016) - 2016 • 3735
On Local Phase Equilibria and the Appearance of Nanoparticles in the Microstructure of Single-Crystal Ni-Base Superalloys
Yardley, V. and Povstugar, I. and Choi, P.-P. and Raabe, D. and Parsa, A.B. and Kostka, A. and Somsen, C. and Dlouhy, A. and Neuking, K. and George, E.P. and Eggeler, G.
ADVANCED ENGINEERING MATERIALS. Volume: 18 (2016) - 2016 • 3734
Catch bond interaction allows cells to attach to strongly hydrated interfaces
Hanke-Roos, M. and Meseck, G.R. and Rosenhahn, A.
BIOINTERPHASES. Volume: 11 (2016) - 2016 • 3733
Detection of individual nanoparticle impacts using etched carbon nanoelectrodes
Clausmeyer, J. and Wilde, P. and Löffler, T. and Ventosa, E. and Tschulik, K. and Schuhmann, W.
ELECTROCHEMISTRY COMMUNICATIONS. Volume: 73 (2016) - 2016 • 3732
Superelasticity and Tunable Thermal Expansion across a Wide Temperature Range
Hao, Y. L. and Wang, H. L. and Li, T. and Cairney, J. M. and Ceguerra, A. V. and Wang, Y. D. and Wang, Y. and Wang, D. and Obbard, E. G. and Li, S. J. and Yang, R.
JOURNAL OF MATERIALS SCIENCE & TECHNOLOGY. Volume: 32 (2016) - 2016 • 3731
Quasiparticle interference from different impurities on the surface of pyrochlore iridates: Signatures of the Weyl phase
Lambert, F. and Schnyder, A.P. and Moessner, R. and Eremin, I.
PHYSICAL REVIEW B - CONDENSED MATTER AND MATERIALS PHYSICS. Volume: 94 (2016) - 2016 • 3730
Wave digital emulation of a double barrier memristive device
Ochs, K. and Solan, E. and Dirkmann, S. and Mussenbrock, T.
MIDWEST SYMPOSIUM ON CIRCUITS AND SYSTEMS. Volume: 0 (2016) - 2016 • 3729
A phase field model for damage in elasto-viscoplastic materials
Shanthraj, P. and Sharma, L. and Svendsen, B. and Roters, F. and Raabe, D.
COMPUTER METHODS IN APPLIED MECHANICS AND ENGINEERING. Volume: 312 (2016) - 2016 • 3728
Nanoelectrodes: Applications in electrocatalysis, single-cell analysis and high-resolution electrochemical imaging
Clausmeyer, J. and Schuhmann, W.
TRAC - TRENDS IN ANALYTICAL CHEMISTRY. Volume: 79 (2016) - 2016 • 3727
Design of Hierarchically Porous Carbons with Interlinked Hydrophilic and Hydrophobic Surface and Their Capacitive Behavior
Hao, G.-P. and Zhang, Q. and Sin, M. and Hippauf, F. and Borchardt, L. and Brunner, E. and Kaskel, S.
CHEMISTRY OF MATERIALS. Volume: 28 (2016) - 2016 • 3726
The oxygen reduction reaction at the three-phase boundary: nanoelectrodes modified with Ag nanoclusters
Clausmeyer, J. and Botz, A. and Öhl, D. and Schuhmann, W.
FARADAY DISCUSSIONS. Volume: 193 (2016) - 2016 • 3725
Strong correlations, strong coupling, and s -wave superconductivity in hole-doped BaFe2As2 single crystals
Hardy, F. and Böhmer, A.E. and De'Medici, L. and Capone, M. and Giovannetti, G. and Eder, R. and Wang, L. and He, M. and Wolf, T. and Schweiss, P. and Heid, R. and Herbig, A. and Adelmann, P. and Fisher, R.A. and Meingast, C.
PHYSICAL REVIEW B. Volume: 94 (2016) - 2016 • 3724
Systematic molecular engineering of Zn-ketoiminates for application as precursors in atomic layer depositions of zinc oxide
O'Donoghue, R. and Peeters, D. and Rogalla, D. and Becker, H.-W. and Rechmann, J. and Henke, S. and Winter, M. and Devi, A.
DALTON TRANSACTIONS. Volume: 45 (2016) - 2016 • 3723
Nanoelectrodes reveal the electrochemistry of single nickelhydroxide nanoparticles
Clausmeyer, J. and Masa, J. and Ventosa, E. and Öhl, D. and Schuhmann, W.
CHEMICAL COMMUNICATIONS. Volume: 52 (2016) - 2016 • 3722
Springback prediction and reduction in deep drawing under influence of unloading modulus degradation
ul Hassan, H. and Maqbool, F. and Güner, A. and Hartmaier, A. and Ben Khalifa, N. and Tekkaya, A.E.
INTERNATIONAL JOURNAL OF MATERIAL FORMING. Volume: 9 (2016) - 2016 • 3721
Atomistically informed extended Gibbs energy description for phase-field simulation of tempering of martensitic steel
Shchyglo, O. and Hammerschmidt, T. and Čak, M. and Drautz, R. and Steinbach, I.
MATERIALS. Volume: 9 (2016) - 2016 • 3720
Anisotropic spin fluctuations in Sr2RuO4: Role of spin-orbit coupling and induced strain
Cobo, S. and Ahn, F. and Eremin, I. and Akbari, A.
PHYSICAL REVIEW B - CONDENSED MATTER AND MATERIALS PHYSICS. Volume: 94 (2016) - 2016 • 3719
Localized plastic deformation in a model metallic glass: A survey of free volume and local force distributions
Hassani, M. and Engels, P. and Raabe, D. and Varnik, F.
JOURNAL OF STATISTICAL MECHANICS: THEORY AND EXPERIMENT. Volume: 2016 (2016) - 2016 • 3718
Lattice Distortions in the FeCoNiCrMn High Entropy Alloy Studied by Theory and Experiment
Oh, H. S. and Ma, D. and Leyson, G. P. and Grabowski, B. and Park, E. S. and Kormann, F. and Raabe, D.
ENTROPY. Volume: 18 (2016) - 2016 • 3717
The Formation and Morphology of Nanoparticle Supracrystals
Haubold, D. and Reichhelm, A. and Weiz, A. and Borchardt, L. and Ziegler, C. and Bahrig, L. and Kaskel, S. and Ruck, M. and Eychmüller, A.
ADVANCED FUNCTIONAL MATERIALS. Volume: 26 (2016) - 2016 • 3716
(Nbx, Zr1-x)4AlC3 MAX Phase Solid Solutions: Processing, Mechanical Properties, and Density Functional Theory Calculations
Lapauw, T. and Tytko, D. and Vanmeensel, K. and Huang, S. and Choi, P.-P. and Raabe, D. and Caspi, E.N. and Ozeri, O. and To Baben, M. and Schneider, J.M. and Lambrinou, K. and Vleugels, J.
INORGANIC CHEMISTRY. Volume: 55 (2016) - 2016 • 3715
Atomic displacement in the CrMnFeCoNi high-entropy alloy - A scaling factor to predict solid solution strengthening
Okamoto, N.L. and Yuge, K. and Tanaka, K. and Inui, H. and George, E.P.
AIP ADVANCES. Volume: 6 (2016) - 2016 • 3714
Exploring the mineral-water interface: Reduction and reaction kinetics of single hematite (α-Fe2O3) nanoparticles
Shimizu, K. and Tschulik, K. and Compton, R.G.
CHEMICAL SCIENCE. Volume: 7 (2016) - 2016 • 3713
Experimental Evidence for a Three-Body Interaction between Diffusing CO Molecules
Zaum, C. and Morgenstern, K.
NANO LETTERS. Volume: 16 (2016) - 2016 • 3712
Microstructure evolution and critical stress for twinning in the CrMnFeCoNi high-entropy alloy
Laplanche, G. and Kostka, A. and Horst, O.M. and Eggeler, G. and George, E.P.
ACTA MATERIALIA. Volume: 118 (2016) - 2016 • 3711
Size effect, critical resolved shear stress, stacking fault energy, and solid solution strengthening in the CrMnFeCoNi high-entropy alloy
Okamoto, N.L. and Fujimoto, S. and Kambara, Y. and Kawamura, M. and Chen, Z.M.T. and Matsunoshita, H. and Tanaka, K. and Inui, H. and George, E.P.
SCIENTIFIC REPORTS. Volume: 6 (2016) - 2016 • 3710
Temperature calibration for diffusion experiments to sub-Kelvin precision
Zaum, C. and Bertram, C. and Meyer Auf Der Heide, K.M. and Mehlhorn, M. and Morgenstern, K.
REVIEW OF SCIENTIFIC INSTRUMENTS. Volume: 87 (2016) - 2016 • 3709
Interrogation of immunoassay platforms by SERS and SECM after enzyme-catalyzed deposition of silver nanoparticles
Conzuelo, F. and Grützke, S. and Stratmann, L. and Pingarrón, J.M. and Schuhmann, W.
MICROCHIMICA ACTA. Volume: 183 (2016) - 2016 • 3708
Spoke rotation reversal in magnetron discharges of aluminium, chromium and titanium
Hecimovic, A. and Maszl, C. and Schulz-von der Gathen, V. and Boke, M. and von Keudell, A.
PLASMA SOURCES SCIENCE & TECHNOLOGY. Volume: 25 (2016) - 2016 • 3707
Oxidation Behavior of the CrMnFeCoNi High-Entropy Alloy
Laplanche, G. and Volkert, U.F. and Eggeler, G. and George, E.P.
OXIDATION OF METALS. Volume: 85 (2016) - 2016 • 3706
First principles characterisation of brittle transgranular fracture of titanium hydrides
Olsson, P.A.T. and Mrovec, M. and Kroon, M.
ACTA MATERIALIA. Volume: 118 (2016) - 2016 • 3705
Intermediate Co/Ni-base model superalloys - Thermophysical properties, creep and oxidation
Zenk, C.H. and Neumeier, S. and Engl, N.M. and Fries, S.G. and Dolotko, O. and Weiser, M. and Virtanen, S. and Göken, M.
SCRIPTA MATERIALIA. Volume: 112 (2016) - 2016 • 3704
Revising secondary electron yields of ion-sputtered metal oxides
Corbella, C. and Marcak, A. and de los Arcos, T. and von Keudell, A.
JOURNAL OF PHYSICS D-APPLIED PHYSICS. Volume: 49 (2016) - 2016 • 3703
Plasticity of the ω-Al7Cu2Fe phase
Laplanche, G. and Bonneville, J. and Joulain, A. and Gauthier-Brunet, V. and Dubois, S.
JOURNAL OF ALLOYS AND COMPOUNDS. Volume: 665 (2016) - 2016 • 3702
Stabilizing nuclear spins around semiconductor electrons via the interplay of optical coherent population trapping and dynamic nuclear polarization
Onur, A.R. and De Jong, J.P. and O'Shea, D. and Reuter, D. and Wieck, A.D. and Van Der Wal, C.H.
PHYSICAL REVIEW B - CONDENSED MATTER AND MATERIALS PHYSICS. Volume: 93 (2016) - 2016 • 3701
Phase field modeling of intercalation kinetics: A finite interface dissipation approach
Zerihun, N.A. and Steinbach, I.
MRS COMMUNICATIONS. Volume: 6 (2016) - 2016 • 3700
Multilevel computational model for failure analysis of steel-fiber-reinforced concrete structures
Zhan, Y. and Meschke, G.
JOURNAL OF ENGINEERING MECHANICS. Volume: 142 (2016) - 2016 • 3699
The fractal dimension of ice on the nanoscale
Heidorn, S.-C. and Bertram, C. and Morgenstern, K.
CHEMICAL PHYSICS LETTERS. Volume: 665 (2016) - 2016 • 3698
Interplay between interstitial displacement and displacive lattice transformations
Zhang, X. and Hickel, T. and Rogal, J. and Neugebauer, J.
PHYSICAL REVIEW B - CONDENSED MATTER AND MATERIALS PHYSICS. Volume: 94 (2016) - 2016 • 3697
Active contour extraction method for objects with a rough surface using single-chip FMCW radars
Damyanov, D. and Friederich, B. and Schultze, T. and Willms, I. and Salman, R. and Barowski, J. and Rolfes, I.
2016 IEEE RADAR CONFERENCE, RADARCONF 2016. Volume: (2016) - 2016 • 3696
Atom probe tomography of intermetallic phases and interfaces formed in dissimilar joining between Al alloys and steel
Lemmens, B. and Springer, H. and Duarte, M.J. and De Graeve, I. and De Strycker, J. and Raabe, D. and Verbeken, K.
MATERIALS CHARACTERIZATION. Volume: 120 (2016) - 2016 • 3695
Simultaneous introduction of various palladium active sites into MOF: Via one-pot synthesis: Pd at[Cu3- xPdx(BTC)2]n
Zhang, W. and Chen, Z. and Al-Naji, M. and Guo, P. and Cwik, S. and Halbherr, O. and Wang, Y. and Muhler, M. and Wilde, N. and Gläser, R. and Fischer, R.A.
DALTON TRANSACTIONS. Volume: 45 (2016) - 2016 • 3694
Ruthenium Metal–Organic Frameworks with Different Defect Types: Influence on Porosity, Sorption, and Catalytic Properties
Zhang, W. and Kauer, M. and Halbherr, O. and Epp, K. and Guo, P. and Gonzalez, M.I. and Xiao, D.J. and Wiktor, C. and LIabrés i Xamena, F.X. and Wöll, C. and Wang, Y. and Muhler, M. and Fischer, R.A.
CHEMISTRY - A EUROPEAN JOURNAL. Volume: 22 (2016) - 2016 • 3693
Mechanism of the Fe3(B,C) and Fe23(C,B)6 solid-state transformation in the hypoeutectic region of the Fe-C-B system
Lentz, J. and Röttger, A. and Theisen, W.
ACTA MATERIALIA. Volume: 119 (2016) - 2016 • 3692
Interactions Between Electrolytes and Carbon-Based Materials—NMR Studies on Electrical Double-Layer Capacitors, Lithium-Ion Batteries, and Fuel Cells
Oschatz, M. and Borchardt, L. and Hippauf, F. and Nickel, W. and Kaskel, S. and Brunner, E.
ANNUAL REPORTS ON NMR SPECTROSCOPY. Volume: 87 (2016) - 2016 • 3691
Improving the mechanical properties of Fe – TiB2 high modulus steels through controlled solidification processes
Zhang, H. and Springer, H. and Aparicio-Fernández, R. and Raabe, D.
ACTA MATERIALIA. Volume: 118 (2016) - 2016 • 3690
Ex vivo brain tumor analysis using spectroscopic optical coherence tomography
Lenz, M. and Krug, R. and Welp, H. and Schmieder, K. and Hofmann, M.R.
PROGRESS IN BIOMEDICAL OPTICS AND IMAGING - PROCEEDINGS OF SPIE. Volume: 9697 (2016) - 2016 • 3689
Development and verification of a resolved 3D inner particle heat transfer model for the Discrete Element Method (DEM)
Oschmann, T. and Schiemann, M. and Kruggel-Emden, H.
POWDER TECHNOLOGY. Volume: 291 (2016) - 2016 • 3688
Imaging the Bonds of Dehalogenated Benzene Radicals on Cu(111) and Au(111)
Simic-Milosevic, V. and Mehlhorn, M. and Morgenstern, K.
CHEMPHYSCHEM. Volume: (2016) - 2016 • 3687
Autonomous Filling of Grain-Boundary Cavities during Creep Loading in Fe-Mo Alloys
Zhang, S. and Fang, H. and Gramsma, M.E. and Kwakernaak, C. and Sloof, W.G. and Tichelaar, F.D. and Kuzmina, M. and Herbig, M. and Raabe, D. and Brück, E. and van der Zwaag, S. and van Dijk, N.H.
METALLURGICAL AND MATERIALS TRANSACTIONS A: PHYSICAL METALLURGY AND MATERIALS SCIENCE. Volume: 47 (2016) - 2016 • 3686
A CSF-SPH method for simulating drainage and imbibition at pore-scale resolution while tracking interfacial areas
Sivanesapillai, R. and Falkner, N. and Hartmaier, A. and Steeb, H.
ADVANCES IN WATER RESOURCES. Volume: 95 (2016) - 2016 • 3685
A virtual laboratory using high resolution crystal plasticity simulations to determine the initial yield surface for sheet metal forming operations
Zhang, H. and Diehl, M. and Roters, F. and Raabe, D.
INTERNATIONAL JOURNAL OF PLASTICITY. Volume: 80 (2016) - 2016 • 3684
Spearhead Nanometric Field-Effect Transistor Sensors for Single-Cell Analysis
Zhang, Y. and Clausmeyer, J. and Babakinejad, B. and López Córdoba, A. and Ali, T. and Shevchuk, A. and Takahashi, Y. and Novak, P. and Edwards, C. and Lab, M. and Gopal, S. and Chiappini, C. and Anand, U. and Magnani, L. and Coombes, R.C. and Gorelik, J. and Matsue, T. and Schuhmann, W. and Klenerman, D. and Sviderskaya, E.V. and Korchev, Y.
ACS NANO. Volume: 10 (2016) - 2016 • 3683
Concentration-Dependent Proton Transfer Mechanisms in Aqueous NaOH Solutions: From Acceptor-Driven to Donor-Driven and Back
Hellström, M. and Behler, J.
JOURNAL OF PHYSICAL CHEMISTRY LETTERS. Volume: 7 (2016) - 2016 • 3682
Atomic-scale characterization and modeling of 60°dislocations in a high-entropy alloy
Smith, T.M. and Hooshmand, M.S. and Esser, B.D. and Otto, F. and Mccomb, D.W. and George, E.P. and Ghazisaeidi, M. and Mills, M.J.
ACTA MATERIALIA. Volume: 110 (2016) - 2016 • 3681
Development of a post-synthetic method for tuning the Al content of OSDA-free Beta as a catalyst for conversion of methanol to olefins
Otomo, R. and Müller, U. and Feyen, M. and Yilmaz, B. and Meng, X. and Xiao, F.-S. and Gies, H. and Bao, X. and Zhang, W. and De Vos, D. and Yokoi, T.
CATALYSIS SCIENCE AND TECHNOLOGY. Volume: 6 (2016) - 2016 • 3680
Reconstruction of nuclear quadrupole interaction in (In,Ga)As/GaAs quantum dots observed by transmission electron microscopy
Sokolov, P.S. and Petrov, M.Y. and Mehrtens, T. and Müller-Caspary, K. and Rosenauer, A. and Reuter, D. and Wieck, A.D.
PHYSICAL REVIEW B - CONDENSED MATTER AND MATERIALS PHYSICS. Volume: 93 (2016) - 2016 • 3679
Absence of nematic order in the pressure-induced intermediate phase of the iron-based superconductor B a0.85 K0.15 F e2 A s2
Zheng, Y. and Tam, P.M. and Hou, J. and Böhmer, A.E. and Wolf, T. and Meingast, C. and Lortz, R.
PHYSICAL REVIEW B. Volume: 93 (2016) - 2016 • 3678
Structural and multifunctional properties of magnetron-sputtered Fe-P(-Mn) thin films
Decker, P. and Stein, H.S. and Salomon, S. and Brüssing, F. and Savan, A. and Hamann, S. and Ludwig, Al.
THIN SOLID FILMS. Volume: 603 (2016) - 2016 • 3677
Silver nanoparticles with different size and shape: Equal cytotoxicity, but different antibacterial effects
Helmlinger, J. and Sengstock, C. and Groß-Heitfeld, C. and Mayer, C. and Schildhauer, T.A. and Köller, M. and Epple, M.
RSC ADVANCES. Volume: 6 (2016) - 2016 • 3676
Multiscale modeling of hydrogen enhanced homogeneous dislocation nucleation
Leyson, G.P.M. and Grabowski, B. and Neugebauer, J.
ACTA MATERIALIA. Volume: 107 (2016) - 2016 • 3675
Single-electron spin resonance in a quadruple quantum dot
Otsuka, T. and Nakajima, T. and Delbecq, M.R. and Amaha, S. and Yoneda, J. and Takeda, K. and Allison, G. and Ito, T. and Sugawara, R. and Noiri, A. and Ludwig, Ar. and Wieck, A.D. and Tarucha, S.
SCIENTIFIC REPORTS. Volume: 6 (2016) - 2016 • 3674
Micro-Venturi injector: Design, experimental and simulative examination
Degenhardt, S. and Cheriguen, Y. and Geiling, T. and Hoffmann, M.
JOURNAL OF PHYSICS: CONFERENCE SERIES. Volume: 757 (2016) - 2016 • 3673
Experimentelle Siebklassierung und deren Abbildung in der DEM
Hennig, M. and Elskamp, F. and Teipel, U. and Kruggel-Emden, H.
CHEMIE-INGENIEUR-TECHNIK. Volume: 88 (2016) - 2016 • 3672
The role of ω in the precipitation of α in near-β Ti alloys
Li, T. and Kent, D. and Sha, G. and Cairney, J.M. and Dargusch, M.S.
SCRIPTA MATERIALIA. Volume: 117 (2016) - 2016 • 3671
Decomposition of the single-phase high-entropy alloy CrMnFeCoNi after prolonged anneals at intermediate temperatures
Otto, F. and Dlouhý, A. and Pradeep, K.G. and Kuběnová, M. and Raabe, D. and Eggeler, G. and George, E.P.
ACTA MATERIALIA. Volume: 112 (2016) - 2016 • 3670
Tailoring the Catalytic Properties of Metal Nanoparticles via Support Interactions
Ahmadi, M. and Mistry, H. and Roldan Cuenya, B.
JOURNAL OF PHYSICAL CHEMISTRY LETTERS. Volume: 7 (2016) - 2016 • 3669
New insights into the phase transformations to isothermal ω and ω-assisted α in near β-Ti alloys
Li, T. and Kent, D. and Sha, G. and Stephenson, L.T. and Ceguerra, A.V. and Ringer, S.P. and Dargusch, M.S. and Cairney, J.M.
ACTA MATERIALIA. Volume: 106 (2016) - 2016 • 3668
Fe-doped Beta zeolite from organotemplate-free synthesis for NH3-SCR of NOX
Zhu, Y. and Chen, B. and Zhao, R. and Zhao, Q. and Gies, H. and Xiao, F.-S. and De Vos, D. and Yokoi, T. and Bao, X. and Kolb, U. and Feyen, M. and Maurer, S. and Moini, A. and Müller, U. and Shi, C. and Zhang, W.
CATALYSIS SCIENCE AND TECHNOLOGY. Volume: 6 (2016) - 2016 • 3667
Size-dependent adhesion energy of shape-selected Pd and Pt nanoparticles
Ahmadi, M. and Behafarid, F. and Cuenya, B.R.
NANOSCALE. Volume: 8 (2016) - 2016 • 3666
Formation of nanosized grain structure in martensitic 100Cr6 bearing steels upon rolling contact loading studied by atom probe tomography
Li, Y.J. and Herbig, M. and Goto, S. and Raabe, D.
MATERIALS SCIENCE AND TECHNOLOGY (UNITED KINGDOM). Volume: 32 (2016) - 2016 • 3665
Robust observer-based adaptive fuzzy sliding mode controller
Oveisi, A. and Nestorović, T.
MECHANICAL SYSTEMS AND SIGNAL PROCESSING. Volume: 76-77 (2016) - 2016 • 3664
Cr2O3 Nanoparticles on Ba5Ta4O15 as a Noble-Metal-Free Oxygen Evolution Co-Catalyst for Photocatalytic Overall Water Splitting
Soldat, J. and Busser, G.W. and Muhler, M. and Wark, M.
CHEMCATCHEM. Volume: 8 (2016) - 2016 • 3663
Quantum Dephasing in a Gated GaAs Triple Quantum Dot due to Nonergodic Noise
Delbecq, M.R. and Nakajima, T. and Stano, P. and Otsuka, T. and Amaha, S. and Yoneda, J. and Takeda, K. and Allison, G. and Ludwig, Ar. and Wieck, A.D. and Tarucha, S.
PHYSICAL REVIEW LETTERS. Volume: 116 (2016) - 2016 • 3662
Metastable high-entropy dual-phase alloys overcome the strength-ductility trade-off
Li, Z. and Pradeep, K.G. and Deng, Y. and Raabe, D. and Tasan, C.C.
NATURE. Volume: 534 (2016) - 2016 • 3661
Co@Co3O4 Encapsulated in Carbon Nanotube-Grafted Nitrogen-Doped Carbon Polyhedra as an Advanced Bifunctional Oxygen Electrode
Aijaz, A. and Masa, J. and Rösler, C. and Xia, W. and Weide, P. and Botz, A.J.R. and Fischer, R.A. and Schuhmann, W. and Muhler, M.
ANGEWANDTE CHEMIE - INTERNATIONAL EDITION. Volume: 55 (2016) - 2016 • 3660
Dynamic high-temperature tensile characterization of an iridium alloy
Song, B. and Nelson, K. and Lipinski, R. and Bignell, J. and Ulrich, G.B. and George, E.P.
CONFERENCE PROCEEDINGS OF THE SOCIETY FOR EXPERIMENTAL MECHANICS SERIES. Volume: 85 (2016) - 2016 • 3659
ZnPd/ZnO Aerogels as Potential Catalytic Materials
Ziegler, C. and Klosz, S. and Borchardt, L. and Oschatz, M. and Kaskel, S. and Friedrich, M. and Kriegel, R. and Keilhauer, T. and Armbrüster, M. and Eychmüller, A.
ADVANCED FUNCTIONAL MATERIALS. Volume: 26 (2016) - 2016 • 3658
Selective Methane Oxidation Catalyzed by Platinum Salts in Oleum at Turnover Frequencies of Large-Scale Industrial Processes
Zimmermann, T. and Soorholtz, M. and Bilke, M. and Schüth, F.
JOURNAL OF THE AMERICAN CHEMICAL SOCIETY. Volume: 138 (2016) - 2016 • 3657
Considerations on the symmetry of pure silica ITQ-7 zeolite (ISV) derived from 29Si MAS NMR and Rietveld analysis
Paillaud, J.-L. and Tzanis, L. and Marler, B. and Rigolet, S. and Patarin, J. and Gies, H.
MICROPOROUS AND MESOPOROUS MATERIALS. Volume: 219 (2016) - 2016 • 3656
Local Platinum Environments in a Solid Analogue of the Molecular Periana Catalyst
Soorholtz, M. and Jones, L.C. and Samuelis, D. and Weidenthaler, C. and White, R.J. and Titirici, M.-M. and Cullen, D.A. and Zimmermann, T. and Antonietti, M. and Maier, J. and Palkovits, R. and Chmelka, B.F. and Schüth, F.
ACS CATALYSIS. Volume: 6 (2016) - 2016 • 3655
Multiport calibration for microwave tomography systems
Zimmermanns, M. and Rolfes, I. and Mallach, M. and Gebhardt, P. and Musch, T.
GEMIC 2016 - 2016 GERMAN MICROWAVE CONFERENCE. Volume: (2016) - 2016 • 3654
The impact of carbon and oxygen in alpha-titanium: Ab initio study of solution enthalpies and grain boundary segregation
Aksyonov, D.A. and Hickel, T. and Neugebauer, J. and Lipnitskii, A.G.
JOURNAL OF PHYSICS CONDENSED MATTER. Volume: 28 (2016) - 2016 • 3653
Single Nanoparticle Voltammetry: Contact Modulation of the Mediated Current
Li, X. and Batchelor-Mcauley, C. and Whitby, S.A.I. and Tschulik, K. and Shao, L. and Compton, R.G.
ANGEWANDTE CHEMIE - INTERNATIONAL EDITION. Volume: 55 (2016) - 2016 • 3652
Probing indirect exciton complexes in a quantum dot molecule via capacitance-voltage spectroscopy
Pal, S. and Junggebauer, C. and Valentin, S.R. and Eickelmann, P. and Scholz, S. and Ludwig, Ar. and Wieck, A.D.
PHYSICAL REVIEW B - CONDENSED MATTER AND MATERIALS PHYSICS. Volume: 94 (2016) - 2016 • 3651
Thermodynamic and kinetic solid-liquid interface properties from transition path sampling
Şopu, D. and Rogal, J. and Drautz, R.
JOURNAL OF CHEMICAL PHYSICS. Volume: 145 (2016) - 2016 • 3650
Model Study of Thermoresponsive Behavior of Metal-Organic Frameworks Modulated by Linker Functionalization
Alaghemandi, M. and Schmid, R.
JOURNAL OF PHYSICAL CHEMISTRY C. Volume: 120 (2016) - 2016 • 3649
Atomic mobility in the overheated amorphous GeTe compound for phase change memories
Sosso, G.C. and Behler, J. and Bernasconi, M.
PHYSICA STATUS SOLIDI (A) APPLICATIONS AND MATERIALS SCIENCE. Volume: 213 (2016) - 2016 • 3648
Effect of Plasma Enthalpy on the Structure of La2Zr2O7 Coatings Prepared by Suspension Plasma Spraying
Zotov, N. and Guignard, A. and Mauer, G. and Vaßen, R.
JOURNAL OF THE AMERICAN CERAMIC SOCIETY. Volume: 99 (2016) - 2016 • 3647
Optimal designs for regression models with autoregressive errors
Dette, H. and Pepelyshev, A. and Zhigljavsky, A.
STATISTICS AND PROBABILITY LETTERS. Volume: 116 (2016) - 2016 • 3646
Antenna induced hot restrike of a ceramic metal halide lamp recorded by high-speed photography
Hermanns, P. and Hoebing, T. and Bergner, A. and Ruhrmann, C. and Awakowicz, P. and Mentel, J.
JOURNAL OF APPLIED PHYSICS. Volume: 119 (2016) - 2016 • 3645
Optimal designs for comparing curves
Dette, H. and Schorning, K.
ANNALS OF STATISTICS. Volume: 44 (2016) - 2016 • 3644
Reactions at the nanoscale: general discussion
Hersbach, T. and MacPherson, J. and Magnussen, O. and Crooks, R. and Higgins, S. and Fermin, D. and Kanoufi, F. and Schuhmann, W. and Nichols, R. and Mitra, S. and Schmickler, W. and Tschulik, K. and Bartlett, P. and Faez, S. and Nogala, W. and Eikerling, M. and Kranz, C. and Unwin, P. and Koper, M. and Lemay, S. and Mount, A. and Ewing, A. and Tian, Z. and White, H. and Chen, S. and Clausmeyer, J. and Krischer, K.
FARADAY DISCUSSIONS. Volume: 193 (2016) - 2016 • 3643
Importance of inclusion of the effect of s electrons into bond-order potentials for transition bcc metals with d-band mediated bonding
Lin, Y.-S. and Mrovec, M. and Vitek, V.
MODELLING AND SIMULATION IN MATERIALS SCIENCE AND ENGINEERING. Volume: 24 (2016) - 2016 • 3642
Microstructural analysis in the Fe-30.5Mn-8.0Al-1.2C and Fe-30.5Mn-2.1Al-1.2C steels upon cold rolling
Souza, F.M. and Padilha, A.F. and Gutierrez-Urrutia, I. and Raabe, D.
REVISTA ESCOLA DE MINAS. Volume: 69 (2016) - 2016 • 3641
Detecting relevant changes in time series models
Dette, H. and Wied, D.
JOURNAL OF THE ROYAL STATISTICAL SOCIETY. SERIES B: STATISTICAL METHODOLOGY. Volume: 78 (2016) - 2016 • 3640
Bond-order potential for magnetic body-centered-cubic iron and its transferability
Lin, Y.-S. and Mrovec, M. and Vitek, V.
PHYSICAL REVIEW B - CONDENSED MATTER AND MATERIALS PHYSICS. Volume: 93 (2016) - 2016 • 3639
Scale bridging description of coherent phase equilibria in the presence of surfaces and interfaces
Spatschek, R. and Gobbi, G. and Hüter, C. and Chakrabarty, A. and Aydin, U. and Brinckmann, S. and Neugebauer, J.
PHYSICAL REVIEW B - CONDENSED MATTER AND MATERIALS PHYSICS. Volume: 94 (2016) - 2016 • 3638
Electrochemistry of single nanoparticles: general discussion
Albrecht, T. and MacPherson, J. and Magnussen, O. and Fermin, D. and Crooks, R. and Gooding, J. and Hersbach, T. and Kanoufi, F. and Schuhmann, W. and Bentley, C. and Tao, N. and Mitra, S. and Krischer, K. and Tschulik, K. and Faez, S. and Nogala, W. and Unwin, P. and Long, Y. and Koper, M. and Tian, Z. and Alpuche-Aviles, M.A. and White, H. and Brasiliense, V. and Kranz, C. and Schmickler, W. and Stevenson, K. and Jing, C. and Edwards, M.
FARADAY DISCUSSIONS. Volume: 193 (2016) - 2016 • 3637
Testing multivariate economic restrictions using quantiles: The example of Slutsky negative semidefiniteness
Dette, H. and Hoderlein, S. and Neumeyer, N.
JOURNAL OF ECONOMETRICS. Volume: 191 (2016) - 2016 • 3636
Effects of oxy-fuel conditions on the products of pyrolysis in a drop tube reactor
Heuer, S. and Senneca, O. and Wütscher, A. and Düdder, H. and Schiemann, M. and Muhler, M. and Scherer, V.
FUEL PROCESSING TECHNOLOGY. Volume: 150 (2016) - 2016 • 3635
Hydrodynamics in adaptive resolution particle simulations: Multiparticle collision dynamics
Alekseeva, U. and Winkler, R.G. and Sutmann, G.
JOURNAL OF COMPUTATIONAL PHYSICS. Volume: 314 (2016) - 2016 • 3634
Optimal Designs in Regression with Correlated Errors
Dette, H. and Pepelyshev, A. and Zhigljavsky, A.
ANNALS OF STATISTICS. Volume: 44 (2016) - 2016 • 3633
Modelling of flow behaviour and dynamic recrystallization during hot deformation of MS-W 1200 using the phase field framework
Hiebeler, J. and Khlopkov, K. and Shchyglo, O. and Pretorius, T. and Steinbach, I.
MATEC WEB OF CONFERENCES. Volume: 80 (2016) - 2016 • 3632
Comparison of minimum-action and steepest-descent paths in gradient systems
Díaz Leines, G. and Rogal, J.
PHYSICAL REVIEW E - STATISTICAL, NONLINEAR, AND SOFT MATTER PHYSICS. Volume: 93 (2016) - 2016 • 3631
Frequency tuning of polarization oscillations: Toward high-speed spin-lasers
Lindemann, M. and Pusch, T. and Michalzik, R. and Gerhardt, N.C. and Hofmann, M.R.
APPLIED PHYSICS LETTERS. Volume: 108 (2016) - 2016 • 3630
A Nernstian Biosupercapacitor
Pankratov, D. and Conzuelo, F. and Pinyou, P. and Alsaoub, S. and Schuhmann, W. and Shleev, S.
ANGEWANDTE CHEMIE - INTERNATIONAL EDITION. Volume: 55 (2016) - 2016 • 3629
Change of the arc attachment mode and its effect on the lifetime in automotive high intensity discharge lamps
Alexejev, A. and Flesch, P. and Mentel, J. and Awakowicz, P.
JOURNAL OF APPLIED PHYSICS. Volume: 120 (2016) - 2016 • 3628
Frequency tuning of polarization oscillations in spin-lasers
Lindemann, M. and Gerhardt, N.C. and Hofmann, M.R. and Pusch, T. and Michalzik, R.
PROCEEDINGS OF SPIE - THE INTERNATIONAL SOCIETY FOR OPTICAL ENGINEERING. Volume: 9931 (2016) - 2016 • 3627
Transmission electron microscopy of a CMSX-4 Ni-base superalloy produced by selective electron beam melting
Parsa, A.B. and Ramsperger, M. and Kostka, A. and Somsen, C. and Körner, C. and Eggeler, G.
METALS. Volume: 6 (2016) - 2016 • 3626
Efficient additive manufacturing production of oxide- and nitride-dispersion-strengthened materials through atmospheric reactions in liquid metal deposition
Springer, H. and Baron, C. and Szczepaniak, A. and Jägle, E.A. and Wilms, M.B. and Weisheit, A. and Raabe, D.
MATERIALS AND DESIGN. Volume: 111 (2016) - 2016 • 3625
Quantitative screening of an extended oxidative coupling of methane catalyst library
Alexiadis, V.I. and Chaar, M. and van Veen, A. and Muhler, M. and Thybaut, J.W. and Marin, G.B.
APPLIED CATALYSIS B: ENVIRONMENTAL. Volume: 199 (2016) - 2016 • 3624
Directions for new developments on statistical design and analysis of small population group trials
Hilgers, R.-D. and Roes, K. and Stallard, N. and Alberti, C. and Van Baal, C. and Benda, N. and Biesheuvel, E. and Burmann, C.F. and Bogdan, M. and Comets, E. and Day, S. and Dette, H. and Dmitrienko, A. and Friede, T. and Graf, A. and Karlsson, M. and Koch, A. and König, F. and Van Der Lee, J.H. and Lentz, F. and Madan, J. and Male, C. and Mentré, F. and Miller, F. and Molenberghs, G. and Neuenschwander, B. and Posch, M. and Oosterwijk, C. and Röver, C. and Senn, S. and Torres, F. and Zohar, S.
ORPHANET JOURNAL OF RARE DISEASES. Volume: 11 (2016) - 2016 • 3623
Frequency tuning of polarization oscillations in spin-polarized vertical-cavity surface-emitting lasers
Lindemann, M. and Pusch, T. and Michalzik, R. and Gerhardt, N.C. and Hofmann, M.R.
PROCEEDINGS OF SPIE - THE INTERNATIONAL SOCIETY FOR OPTICAL ENGINEERING. Volume: 9892 (2016) - 2016 • 3622
Molecular Mechanism of Crystal Growth Inhibition at the Calcium Oxalate/Water Interfaces
Parvaneh, L.S. and Donadio, D. and Sulpizi, M.
JOURNAL OF PHYSICAL CHEMISTRY C. Volume: 120 (2016) - 2016 • 3621
Combinatorial design of transitory constitution steels: Coupling high strength with inherent formability and weldability through sequenced austenite stability
Springer, H. and Belde, M. and Raabe, D.
MATERIALS AND DESIGN. Volume: 90 (2016) - 2016 • 3620
Strong impact of lattice vibrations on electronic and magnetic properties of paramagnetic Fe revealed by disordered local moments molecular dynamics
Alling, B. and Körmann, F. and Grabowski, B. and Glensk, A. and Abrikosov, I.A. and Neugebauer, J.
PHYSICAL REVIEW B - CONDENSED MATTER AND MATERIALS PHYSICS. Volume: 93 (2016) - 2016 • 3619
Pd deposited on functionalized carbon nanotubes for the electrooxidation of ethanol in alkaline media
Hiltrop, D. and Masa, J. and Maljusch, A. and Xia, W. and Schuhmann, W. and Muhler, M.
ELECTROCHEMISTRY COMMUNICATIONS. Volume: 63 (2016) - 2016 • 3618
Influence of birefringence splitting on ultrafast polarization oscillations in VCSELs
Lindemann, M. and Gerhardt, N.C. and Hofmann, M.R. and Pusch, T. and Michalzik, R.
PROCEEDINGS OF SPIE - THE INTERNATIONAL SOCIETY FOR OPTICAL ENGINEERING. Volume: 9766 (2016) - 2016 • 3617
Double-Q spin-density wave in iron arsenide superconductors
Allred, J.M. and Taddei, K.M. and Bugaris, D.E. and Krogstad, M.J. and Lapidus, S.H. and Chung, D.Y. and Claus, H. and Kanatzidis, M.G. and Brown, D.E. and Kang, J. and Fernandes, R.M. and Eremin, I. and Rosenkranz, S. and Chmaissem, O. and Osborn, R.
NATURE PHYSICS. Volume: 12 (2016) - 2016 • 3616
Towards a continuous adsorption process for the enrichment of ACE-inhibiting peptides from food protein hydrolysates
Hippauf, F. and Huettner, C. and Lunow, D. and Borchardt, L. and Henle, T. and Kaskel, S.
CARBON. Volume: 107 (2016) - 2016 • 3615
The role of ion transport phenomena in memristive double barrier devices
Dirkmann, S. and Hansen, M. and Ziegler, M. and Kohlstedt, H. and Mussenbrock, T.
SCIENTIFIC REPORTS. Volume: 6 (2016) - 2016 • 3614
The Importance of Pore Size and Surface Polarity for Polysulfide Adsorption in Lithium Sulfur Batteries
Hippauf, F. and Nickel, W. and Hao, G.-P. and Schwedtmann, K. and Giebeler, L. and Oswald, S. and Borchardt, L. and Doerfler, S. and Weigand, J.J. and Kaskel, S.
ADVANCED MATERIALS INTERFACES. Volume: 3 (2016) - 2016 • 3613
First-principles investigation of hydrogen interaction with TiC precipitates in α -Fe
Di Stefano, D. and Nazarov, R. and Hickel, T. and Neugebauer, J. and Mrovec, M. and Elsässer, C.
PHYSICAL REVIEW B - CONDENSED MATTER AND MATERIALS PHYSICS. Volume: 93 (2016) - 2016 • 3612
Importance and Challenges of Electrochemical in Situ Liquid Cell Electron Microscopy for Energy Conversion Research
Hodnik, N. and Dehm, G. and Mayrhofer, K.J.J.
ACCOUNTS OF CHEMICAL RESEARCH. Volume: 49 (2016) - 2016 • 3611
Advanced finite element modeling of excavation and advancement processes in mechanized tunneling
Alsahly, A. and Stascheit, J. and Meschke, G.
ADVANCES IN ENGINEERING SOFTWARE. Volume: 100 (2016) - 2016 • 3610
Surface Structure and Photocatalytic Properties of Bi2WO6 Nanoplatelets Modified by Molybdena Islands from Chemical Vapor Deposition
Dittmer, A. and Menze, J. and Mei, B. and Strunk, J. and Luftman, H.S. and Gutkowski, R. and Wachs, I.E. and Schuhmann, W. and Muhler, M.
JOURNAL OF PHYSICAL CHEMISTRY C. Volume: 120 (2016) - 2016 • 3609
The anodic emitter effect and its inversion demonstrated by temperature measurements at doped and undoped tungsten electrodes
Hoebing, T. and Bergner, A. and Hermanns, P. and Mentel, J. and Awakowicz, P.
JOURNAL OF PHYSICS D: APPLIED PHYSICS. Volume: 49 (2016) - 2016 • 3608
Beam-induced atomic migration at Ag-containing nanofacets at an asymmetric Cu grain boundary
Peter, N.J. and Liebscher, C.H. and Kirchlechner, C. and Dehm, G.
JOURNAL OF MATERIALS RESEARCH. Volume: 32 (2016) - 2016 • 3607
3-Dimensional microstructural characterization of CdTe absorber layers from CdTe/CdS thin film solar cells
Stechmann, G. and Zaefferer, S. and Konijnenberg, P. and Raabe, D. and Gretener, C. and Kranz, L. and Perrenoud, J. and Buecheler, S. and Tiwari, A.N.
SOLAR ENERGY MATERIALS AND SOLAR CELLS. Volume: 151 (2016) - 2016 • 3606
Deformation-Induced Martensite: A New Paradigm for Exceptional Steels
Djaziri, S. and Li, Y. and Nematollahi, G.A. and Grabowski, B. and Goto, S. and Kirchlechner, C. and Kostka, A. and Doyle, S. and Neugebauer, J. and Raabe, D. and Dehm, G.
ADVANCED MATERIALS. Volume: 28 (2016) - 2016 • 3605
Are Mo2BC nanocrystalline coatings damage resistant? Insights from comparative tension experiments
Djaziri, S. and Gleich, S. and Bolvardi, H. and Kirchlechner, C. and Hans, M. and Scheu, C. and Schneider, J.M. and Dehm, G.
SURFACE AND COATINGS TECHNOLOGY. Volume: 289 (2016) - 2016 • 3604
Controlled Flexible Coordination in Tripodal Iron(II) Phosphane Complexes: Effects on Reactivity
Petuker, A. and Merz, K. and Merten, C. and Apfel, U.-P.
INORGANIC CHEMISTRY. Volume: 55 (2016) - 2016 • 3603
Direct metal deposition of refractory high entropy alloy MoNbTaW
Dobbelstein, H. and Thiele, M. and Gurevich, E.L. and George, E.P. and Ostendorf, A.
PHYSICS PROCEDIA. Volume: 83 (2016) - 2016 • 3602
Improving optical sorting of bulk materials using sophisticated motion models
Pfaff, F. and Pieper, C. and Maier, G. and Noack, B. and Kruggel-Emden, H. and Gruna, R. and Hanebeck, U.D. and Wirtz, S. and Scherer, V. and Längle, T. and Beyerer, J.
TECHNISCHES MESSEN. Volume: 83 (2016) - 2015 • 3601
The evolution of microstructure and mechanical properties of Ti-5Al-5Mo-5V-2Cr-1Fe during ageing
Ahmed, M. and Li, T. and Casillas, G. and Cairney, J.M. and Wexler, D. and Pereloma, E.V.
JOURNAL OF ALLOYS AND COMPOUNDS. Volume: 629 (2015) - 2015 • 3600
Bayesian T-optimal discriminating designs
Dette, H. and Melas, V.B. and Guchenko, R.
ANNALS OF STATISTICS. Volume: 43 (2015) - 2015 • 3599
Amphiphilic Pickering Emulsifiers Based on Mushroom-Type Janus Particles
Passas-Lagos, E. and Schüth, F.
LANGMUIR. Volume: 31 (2015) - 2015 • 3598
Co3 O4 -MnO2 -CNT Hybrids Synthesized by HNO3 Vapor Oxidation of Catalytically Grown CNTs as OER Electrocatalysts
Xie, K. and Masa, J. and Madej, E. and Yang, F. and Weide, P. and Dong, W. and Muhler, M. and Schuhmann, W. and Xia, W.
CHEMCATCHEM. Volume: 7 (2015) - 2015 • 3597
Multiscale description of dislocation induced nano-hydrides
Leyson, G.P.M. and Grabowski, B. and Neugebauer, J.
ACTA MATERIALIA. Volume: 89 (2015) - 2015 • 3596
A reevaluation of the correlation between the synthesis parameters and structure and properties of nitrogen-doped carbon nanotubes
Xie, K. and Yang, F. and Ebbinghaus, P. and Erbe, A. and Muhler, M. and Xia, W.
JOURNAL OF ENERGY CHEMISTRY. Volume: 24 (2015) - 2015 • 3595
Kinetic simulation of filament growth dynamics in memristive electrochemical metallization devices
Dirkmann, S. and Ziegler, M. and Hansen, M. and Kohlstedt, H. and Trieschmann, J. and Mussenbrock, T.
JOURNAL OF APPLIED PHYSICS. Volume: 118 (2015) - 2015 • 3594
Structural stability and Lewis acidity of tetravalent Ti, Sn, or Zr-linked interlayer-expanded zeolite COE-4: A DFT study
Li, H. and Wang, J. and Zhou, D. and Tian, D. and Shi, C. and Müller, U. and Feyen, M. and Gies, H. and Xiao, F.-S. and De Vos, D. and Yokoi, T. and Bao, X. and Zhang, W.
MICROPOROUS AND MESOPOROUS MATERIALS. Volume: 218 (2015) - 2015 • 3593
Jahn-Teller versus quantum effects in the spin-orbital material LuVO3
Skoulatos, M. and Toth, S. and Roessli, B. and Enderle, M. and Habicht, K. and Sheptyakov, D. and Cervellino, A. and Freeman, P.G. and Reehuis, M. and Stunault, A. and McIntyre, G.J. and Tung, L.D. and Marjerrison, C. and Pomjakushina, E. and Brown, P.J. and Khomskii, D.I. and Rüegg, C. and Kreyssig, A. and Goldman, A.I. and Goff, J.P.
PHYSICAL REVIEW B - CONDENSED MATTER AND MATERIALS PHYSICS. Volume: 91 (2015) - 2015 • 3592
First-principles investigation of quantum mechanical effects on the diffusion of hydrogen in iron and nickel
Di Stefano, D. and Mrovec, M. and Elsässer, C.
PHYSICAL REVIEW B - CONDENSED MATTER AND MATERIALS PHYSICS. Volume: 92 (2015) - 2015 • 3591
The influence of partitioning on the growth of intragranular α in near-β Ti alloys
Li, T. and Ahmed, M. and Sha, G. and Shi, R. and Casillas, G. and Yen, H.-W. and Wang, Y. and Pereloma, E.V. and Cairney, J.M.
JOURNAL OF ALLOYS AND COMPOUNDS. Volume: 643 (2015) - 2015 • 3590
High-Throughput Screening of Thin-Film Semiconductor Material Libraries I: System Development and Case Study for Ti-W-O
Sliozberg, K. and Schäfer, D. and Erichsen, T. and Meyer, R. and Khare, C. and Ludwig, Al. and Schuhmann, W.
CHEMSUSCHEM. Volume: 8 (2015) - 2015 • 3589
Atomic layer-by-layer construction of Pd on nanoporous gold via underpotential deposition and displacement reaction
Yan, X. and Xiong, H. and Bai, Q. and Frenzel, J. and Si, C. and Chen, X. and Eggeler, G. and Zhang, Z.
RSC ADVANCES. Volume: 5 (2015) - 2015 • 3588
First-principles investigation of hydrogen trapping and diffusion at grain boundaries in nickel
Di Stefano, D. and Mrovec, M. and Elsässer, C.
ACTA MATERIALIA. Volume: 98 (2015) - 2015 • 3587
The mechanism of ω-assisted α phase formation in near β-Ti alloys
Li, T. and Kent, D. and Sha, G. and Dargusch, M.S. and Cairney, J.M.
SCRIPTA MATERIALIA. Volume: 104 (2015) - 2015 • 3586
Errautm: From generalized stacking fault energies to dislocation properties: Five-energy-point approach and solid solution effects in magnesium (Physical Review B - Condensed Matter and Materials Physics (2015) 92 (064107))
Pei, Z. and Ma, D. and Friák, M. and Svendsen, B. and Raabe, D. and Neugebauer, J.
PHYSICAL REVIEW B - CONDENSED MATTER AND MATERIALS PHYSICS. Volume: 92 (2015) - 2015 • 3585
A combinatorial study of photoelectrochemical properties of Fe-W-O thin films
Sliozberg, K. and Schäfer, D. and Meyer, R. and Ludwig, Al. and Schuhmann, W.
CHEMPLUSCHEM. Volume: 80 (2015) - 2015 • 3584
High resolution in situ mapping of microstrain and microstructure evolution reveals damage resistance criteria in dual phase steels
Yan, D. and Tasan, C.C. and Raabe, D.
ACTA MATERIALIA. Volume: 96 (2015) - 2015 • 3583
Combinatorial Development of Fe-Co-Nb Thin Film Magnetic Nanocomposites
Alexandrakis, V. and Wallisch, W. and Hamann, S. and Varvaro, G. and Fidler, J. and Ludwig, Al.
ACS COMBINATORIAL SCIENCE. Volume: 17 (2015) - 2015 • 3582
Atomic scale investigation of non-equilibrium segregation of boron in a quenched Mo-free martensitic steel
Li, Y.J. and Ponge, D. and Choi, P. and Raabe, D.
ULTRAMICROSCOPY. Volume: 159 (2015) - 2015 • 3581
Rapid theory-guided prototyping of ductile Mg alloys: From binary to multi-component materials
Pei, Z. and Friák, M. and Sandlöbes, S. and Nazarov, R. and Svendsen, B. and Raabe, D. and Neugebauer, J.
NEW JOURNAL OF PHYSICS. Volume: 17 (2015) - 2015 • 3580
Fe-cr-al containing oxide semiconductors as potential solar water-splitting materials
Sliozberg, K. and Stein, H.S. and Khare, C. and Parkinson, B.A. and Ludwig, Al. and Schuhmann, W.
ACS APPLIED MATERIALS AND INTERFACES. Volume: 7 (2015) - 2015 • 3579
Influence of water on supra-molecular assembly of 4, 4′-dihydroxy azobenzene on Ag(111)
Henzl, J. and Boom, K. and Morgenstern, K.
JOURNAL OF CHEMICAL PHYSICS. Volume: 142 (2015) - 2015 • 3578
Mechanisms of subgrain coarsening and its effect on the mechanical properties of carbon-supersaturated nanocrystalline hypereutectoid steel
Li, Y.J. and Kostka, A. and Choi, P. and Goto, S. and Ponge, D. and Kirchheim, R. and Raabe, D.
ACTA MATERIALIA. Volume: 84 (2015) - 2015 • 3577
From generalized stacking fault energies to dislocation properties: Five-energy-point approach and solid solution effects in magnesium
Pei, Z. and Ma, D. and Friák, M. and Svendsen, B. and Raabe, D. and Neugebauer, J.
PHYSICAL REVIEW B - CONDENSED MATTER AND MATERIALS PHYSICS. Volume: 92 (2015) - 2015 • 3576
Combining structural and chemical information at the nanometer scale by correlative transmission electron microscopy and atom probe tomography
Herbig, M. and Choi, P. and Raabe, D.
ULTRAMICROSCOPY. Volume: 153 (2015) - 2015 • 3575
Segregation of boron at prior austenite grain boundaries in a quenched martensitic steel studied by atom probe tomography
Li, Y.J. and Ponge, D. and Choi, P. and Raabe, D.
SCRIPTA MATERIALIA. Volume: 96 (2015) - 2015 • 3574
Reversible or Not? Distinguishing Agglomeration and Aggregation at the Nanoscale
Sokolov, S.V. and Tschulik, K. and Batchelor-McAuley, C. and Jurkschat, K. and Compton, R.G.
ANALYTICAL CHEMISTRY. Volume: 87 (2015) - 2015 • 3573
Microfluidic detachment assay to probe the adhesion strength of diatoms
Alles, M. and Rosenhahn, A.
BIOFOULING. Volume: 31 (2015) - 2015 • 3572
Microstructure in plasticity, a comparison between theory and experiment
Dmitrieva, O. and Raabe, D. and Müller, S. and Dondl, P.W.
LECTURE NOTES IN APPLIED AND COMPUTATIONAL MECHANICS. Volume: 78 (2015) - 2015 • 3571
Grain boundary segregation in Fe-Mn-C twinning-induced plasticity steels studied by correlative electron backscatter diffraction and atom probe tomography
Herbig, M. and Kuzmina, M. and Haase, C. and Marceau, R.K.W. and Gutierrez-Urrutia, I. and Haley, D. and Molodov, D.A. and Choi, P. and Raabe, D.
ACTA MATERIALIA. Volume: 83 (2015) - 2015 • 3570
Rotational and translational dynamics of CO2 adsorbed in MOF Zn2(bdc)2(dabco)
Peksa, M. and Burrekaew, S. and Schmid, R. and Lang, J. and Stallmach, F.
MICROPOROUS AND MESOPOROUS MATERIALS. Volume: 216 (2015) - 2015 • 3569
Are Nanoparticles Spherical or Quasi-Spherical?
Sokolov, S.V. and Batchelor-Mcauley, C. and Tschulik, K. and Fletcher, S. and Compton, R.G.
CHEMISTRY - A EUROPEAN JOURNAL. Volume: 21 (2015) - 2015 • 3568
[001] Preferentially-oriented 2D tungsten disulfide nanosheets as anode materials for superior lithium storage
Yang, W. and Wang, J. and Si, C. and Peng, Z. and Frenzel, J. and Eggeler, G. and Zhang, Z.
JOURNAL OF MATERIALS CHEMISTRY A. Volume: 3 (2015) - 2015 • 3567
Transformation activity in ultrafine grained pseudoelastic NiTi wires during small amplitude loading/unloading experiments
Pelegrina, J.L. and Yawny, A. and Olbricht, J. and Eggeler, G.
JOURNAL OF ALLOYS AND COMPOUNDS. Volume: 651 (2015) - 2015 • 3566
Effects of Ru on elemental partitioning and precipitation of topologically close-packed phases in Ni-based superalloys
Peng, Z. and Povstugar, I. and Matuszewski, K. and Rettig, R. and Singer, R. and Kostka, A. and Choi, P.-P. and Raabe, D.
SCRIPTA MATERIALIA. Volume: 101 (2015) - 2015 • 3565
Poly(benzoxazine)s Modified with Osmium Complexes as a Class of Redox Polymers for Wiring of Enzymes to Electrode Surfaces
Alsaoub, S. and Barwe, S. and Andronescu, C. and Pöller, S. and Ruff, A. and Schuhmann, W.
CHEMPLUSCHEM. Volume: 80 (2015) - 2015 • 3564
Ultra-small Palladium Nanoparticle Decorated Carbon Nanotubes: Conductivity and Reactivity
Li, X. and Batchelor-McAuley, C. and Tschulik, K. and Shao, L. and Compton, R.G.
CHEMPHYSCHEM. Volume: 16 (2015) - 2015 • 3563
Predictions of a Large Magnetocaloric Effect in Co- and Cr-Substituted Heusler Alloys Using First-Principles and Monte Carlo Approaches
Sokolovskiy, V.V. and Buchelnikov, V.D. and Zagrebin, M.A. and Grünebohm, A. and Entel, P.
PHYSICS PROCEDIA. Volume: 75 (2015) - 2015 • 3562
Temperature dependent low-field measurements of the magnetocaloric Δ T with sub-mK resolution in small volume and thin film samples
Döntgen, J. and Rudolph, J. and Gottschall, T. and Gutfleisch, O. and Salomon, S. and Ludwig, Al. and Hägele, D.
APPLIED PHYSICS LETTERS. Volume: 106 (2015) - 2015 • 3561
Phase and amplitude calibration of dualmask spatial light modulator for highresolution femtosecond pulse shaping
Döpke, B. and Balzer, J.C. and Hofmann, M.R.
ELECTRONICS LETTERS. Volume: 51 (2015) - 2015 • 3560
The topical use of non-thermal dielectric barrier discharge (DBD): Nitric oxide related effects on human skin
Heuer, K. and Hoffmanns, M.A. and Demir, E. and Baldus, S. and Volkmar, C.M. and Röhle, M. and Fuchs, P.C. and Awakowicz, P. and Suschek, C.V. and Opländer, C.
NITRIC OXIDE - BIOLOGY AND CHEMISTRY. Volume: 44 (2015) - 2015 • 3559
Self-optimizing femtosecond semiconductor laser
Döpke, B. and Pilny, R.H. and Brenner, C. and Klehr, A. and Erbert, G. and Tränkle, G. and Balzer, J.C. and Hofmann, M.R.
OPTICS EXPRESS. Volume: 23 (2015) - 2015 • 3558
Ultrashort pulse generation with semiconductor lasers using intracavity phase- and amplitude pulse shaping
Döpke, B. and Balzer, J.C. and Pilny, R.H. and Brenner, C. and Klehr, A. and Erbert, G. and Tränkle, G. and Hofmann, M.R.
PROCEEDINGS OF SPIE - THE INTERNATIONAL SOCIETY FOR OPTICAL ENGINEERING. Volume: 9382 (2015) - 2015 • 3557
Chromatic confocal matrix sensor with actuated pinhole arrays
Hillenbrand, M. and Weiss, R. and Endrödy, C. and Grewe, A. and Hoffmann, M. and Sinzinger, S.
APPLIED OPTICS. Volume: 54 (2015) - 2015 • 3556
Insights into the molecular assembly of zeolitic imidazolate frameworks by ESI-MS
Lim, I.H. and Schrader, W. and Schüth, F.
CHEMISTRY OF MATERIALS. Volume: 27 (2015) - 2015 • 3555
Modulation of extrasynaptic NMDA receptors by synaptic and tonic zinc
Anderson, C.T. and Radford, R.J. and Zastrow, M.L. and Zhang, D.Y. and Apfel, U.-P. and Lippard, S.J. and Tzounopoulos, T.
PROCEEDINGS OF THE NATIONAL ACADEMY OF SCIENCES OF THE UNITED STATES OF AMERICA. Volume: 112 (2015) - 2015 • 3554
Enhancing ACE-inhibition of food protein hydrolysates by selective adsorption using porous carbon materials
Hippauf, F. and Lunow, D. and Huettner, C. and Nickel, W. and Borchardt, L. and Henle, T. and Kaskel, S.
CARBON. Volume: 87 (2015) - 2015 • 3553
Influence of Adsorption Kinetics upon the Electrochemically Reversible Hydrogen Oxidation Reaction
Lin, C. and Jiao, X. and Tschulik, K. and Batchelor-Mcauley, C. and Compton, R.G.
JOURNAL OF PHYSICAL CHEMISTRY C. Volume: 119 (2015) - 2015 • 3552
Theoretical and experimental study of the core structure and mobility of dislocations and their influence on the ferroelectric polarization in perovskite KNbO3
Hirel, P. and Mark, A.F. and Castillo-Rodriguez, M. and Sigle, W. and Mrovec, M. and Elsässer, C.
PHYSICAL REVIEW B - CONDENSED MATTER AND MATERIALS PHYSICS. Volume: 92 (2015) - 2015 • 3551
Complex Surface Diffusion Mechanisms of Cobalt Phthalocyanine Molecules on Ag(100)
Antczak, G. and Kamiński, W. and Sabik, A. and Zaum, C. and Morgenstern, K.
JOURNAL OF THE AMERICAN CHEMICAL SOCIETY. Volume: 137 (2015) - 2015 • 3550
Robust determination of the superconducting gap sign structure via quasiparticle interference
Hirschfeld, P.J. and Altenfeld, D. and Eremin, I. and Mazin, I.I.
PHYSICAL REVIEW B - CONDENSED MATTER AND MATERIALS PHYSICS. Volume: 92 (2015) - 2015 • 3549
Ultrafast polarization dynamics with controlled polarization oscillations in vertical-cavity surface-emitting lasers
Lindemann, M. and Höpfner, H. and Gerhardt, N.C. and Hofmann, M.R. and Pusch, T. and Michalzik, R.
PROCEEDINGS OF SPIE - THE INTERNATIONAL SOCIETY FOR OPTICAL ENGINEERING. Volume: 9381 (2015) - 2015 • 3548
Modulating sonogashira cross-coupling reactivity in four-coordinate nickel complexes by using geometric control
Petuker, A. and Merten, C. and Apfel, U.-P.
EUROPEAN JOURNAL OF INORGANIC CHEMISTRY. Volume: 2015 (2015) - 2015 • 3547
Dynamic high-temperature Kolsky tension bar techniques
Song, B. and Nelson, K. and Lipinski, R. and Bignell, J. and Ulrich, G.B. and George, E.P.
EPJ WEB OF CONFERENCES. Volume: 94 (2015) - 2015 • 3546
Structure-activity relationships of Co-modified Cu/ZnO/Al2 O3 catalysts applied in the synthesis of higher alcohols from synthesis gas
Anton, J. and Nebel, J. and Song, H. and Froese, C. and Weide, P. and Ruland, H. and Muhler, M. and Kaluza, S.
APPLIED CATALYSIS A: GENERAL. Volume: 505 (2015) - 2015 • 3545
Investigation of the flickering of La2O3 and ThO2 doped tungsten cathodes
Hoebing, T. and Hermanns, P. and Bergner, A. and Ruhrmann, C. and Traxler, H. and Wesemann, I. and Knabl, W. and Mentel, J. and Awakowicz, P.
JOURNAL OF APPLIED PHYSICS. Volume: 118 (2015) - 2015 • 3544
Towards high frequency operation of polarization oscillations in spin vertical-cavity surface-emitting lasers
Lindemann, M. and Höpfner, H. and Gerhardt, N.C. and Hofmann, M.R. and Pusch, T. and Michalzik, R.
PROCEEDINGS OF SPIE - THE INTERNATIONAL SOCIETY FOR OPTICAL ENGINEERING. Volume: 9551 (2015) - 2015 • 3543
Interface engineering and nanoscale characterization of Zn(S,O) alternative buffer layer for CIGS thin film solar cells
Soni, P. and Cojocaru-Miredin, O. and Raabe, D.
2015 IEEE 42ND PHOTOVOLTAIC SPECIALIST CONFERENCE, PVSC 2015. Volume: (2015) - 2015 • 3542
Fast and Reproducible Testing of Cu-Co-Based Catalysts Applied in the Conversion of Synthesis Gas to Ethanol and Higher Alcohols
Anton, J. and Ruland, H. and Kaluza, S. and Muhler, M.
CATALYSIS LETTERS. Volume: 145 (2015) - 2015 • 3541
Controlled switching and frequency tuning of polarization oscillations in vertical-cavity surface-emitting lasers
Lindemann, M. and Höpfner, H. and Gerhardt, N.C. and Hofmann, M.R. and Pusch, T. and Michalzik, R.
PROCEEDINGS OF SPIE - THE INTERNATIONAL SOCIETY FOR OPTICAL ENGINEERING. Volume: 9551 (2015) - 2015 • 3540
Bimodal Acidity at the Amorphous Silica/Water Interface
Pfeiffer-Laplaud, M. and Costa, D. and Tielens, F. and Gaigeot, M.-P. and Sulpizi, M.
JOURNAL OF PHYSICAL CHEMISTRY C. Volume: 119 (2015) - 2015 • 3539
Heterogeneous crystallization of the phase change material GeTe via atomistic simulations
Sosso, G.C. and Salvalaglio, M. and Behler, J. and Bernasconi, M. and Parrinello, M.
JOURNAL OF PHYSICAL CHEMISTRY C. Volume: 119 (2015) - 2015 • 3538
Pattern recognition with TiOx-based memristive devices
Zahari, F. and Hansen, M. and Mussenbrock, T. and Ziegler, M. and Kohlstedt, H.
AIMS MATERIALS SCIENCE. Volume: 2 (2015) - 2015 • 3537
The effect of the Au loading on the liquid-phase aerobic oxidation of ethanol over Au/TiO2 catalysts prepared by pulsed laser ablation
Dong, W. and Reichenberger, S. and Chu, S. and Weide, P. and Ruland, H. and Barcikowski, S. and Wagener, P. and Muhler, M.
JOURNAL OF CATALYSIS. Volume: 330 (2015) - 2015 • 3536
A study on the influence of particle shape on the mechanical interactions of granular media in a hopper using the Discrete Element Method
Höhner, D. and Wirtz, S. and Scherer, V.
POWDER TECHNOLOGY. Volume: 278 (2015) - 2015 • 3535
Orientation dependent deformation by slip and twinning in magnesium during single crystal indentation
Zambaldi, C. and Zehnder, C. and Raabe, D.
ACTA MATERIALIA. Volume: 91 (2015) - 2015 • 3534
Three-dimensional Cu foam-supported single crystalline mesoporous Cu2 O nanothorn arrays for ultra-highly sensitive and efficient nonenzymatic detection of glucose
Dong, C. and Zhong, H. and Kou, T. and Frenzel, J. and Eggeler, G. and Zhang, Z.
ACS APPLIED MATERIALS AND INTERFACES. Volume: 7 (2015) - 2015 • 3533
Combined AFM/SECM Investigation of the Solid Electrolyte Interphase in Li-Ion Batteries
Zampardi, G. and Klink, S. and Kuznetsov, V. and Erichsen, T. and Maljusch, A. and LaMantia, F. and Schuhmann, W. and Ventosa, E.
CHEMELECTROCHEM. Volume: 2 (2015) - 2015 • 3532
The influence of stacking fault energy on the microstructural and strain-hardening evolution of Fe-Mn-Al-Si steels during tensile deformation
Pierce, D.T. and Jiménez, J.A. and Bentley, J. and Raabe, D. and Wittig, J.E.
ACTA MATERIALIA. Volume: 100 (2015) - 2015 • 3531
In-operando evaluation of the effect of vinylene carbonate on the insulating character of the solid electrolyte interphase
Zampardi, G. and La Mantia, F. and Schuhmann, W.
ELECTROCHEMISTRY COMMUNICATIONS. Volume: 58 (2015) - 2015 • 3530
Scanning Electrochemical Microscopy Applied to the Investigation of Lithium (De-)Insertion in TiO2
Zampardi, G. and Ventosa, E. and La Mantia, F. and Schuhmann, W.
ELECTROANALYSIS. Volume: 27 (2015) - 2015 • 3529
Bond-order potentials: Derivation and parameterization for refractory elements
Drautz, R. and Hammerschmidt, T. and Čák, M. and Pettifor, D.G.
MODELLING AND SIMULATION IN MATERIALS SCIENCE AND ENGINEERING. Volume: 23 (2015) - 2015 • 3528
Intercalation of Proflavine in ssDNA Aptamers: Effect on Binding of the Specific Target Chloramphenicol
Pilehvar, S. and Jambrec, D. and Gebala, M. and Schuhmann, W. and De Wael, K.
ELECTROANALYSIS. Volume: 27 (2015) - 2015 • 3527
On the role of zinc on the formation and growth of intermetallic phases during interdiffusion between steel and aluminium alloys
Springer, H. and Szczepaniak, A. and Raabe, D.
ACTA MATERIALIA. Volume: 96 (2015) - 2015 • 3526
Determination of the formation and range of stability of the SEI on glassy carbon by local electrochemistry
Zampardi, G. and La Mantia, F. and Schuhmann, W.
RSC ADVANCES. Volume: 5 (2015) - 2015 • 3525
Coupling of an enzymatic biofuel cell to an electrochemical cell for self-powered glucose sensing with optical readout
Pinyou, P. and Conzuelo, F. and Sliozberg, K. and Vivekananthan, J. and Contin, A. and Pöller, S. and Plumeré, N. and Schuhmann, W.
BIOELECTROCHEMISTRY. Volume: 106 (2015) - 2015 • 3524
Microstructure refinement for high modulus in-situ metal matrix composite steels via controlled solidification of the system Fe-TiB2
Springer, H. and Aparicio Fernandez, R. and Duarte, M.J. and Kostka, A. and Raabe, D.
ACTA MATERIALIA. Volume: 96 (2015) - 2015 • 3523
Differences between thermal and laser-induced diffusion
Zaum, C. and Meyer-Auf-Der-Heide, K.M. and Mehlhorn, M. and McDonough, S. and Schneider, W.F. and Morgenstern, K.
PHYSICAL REVIEW LETTERS. Volume: 114 (2015) - 2015 • 3522
Optimization of Os-complex modified redox polymers for improving biocatalysis of PQQ-sGDH based electrodes
Pinyou, P. and Pöller, S. and Chen, X. and Schuhmann, W.
ELECTROANALYSIS. Volume: 27 (2015) - 2015 • 3521
A novel roll-bonding methodology for the cross-scale analysis of phase properties and interactions in multiphase structural materials
Springer, H. and Tasan, C. and Raabe, D.
INTERNATIONAL JOURNAL OF MATERIALS RESEARCH. Volume: 106 (2015) - 2015 • 3520
MAXNET Energy - Focusing Research in Chemical Energy Conversion on the Electrocatlytic Oxygen Evolution
Auer, A.A. and Cap, S. and Antonietti, M. and Cherevko, S. and Deng, X. and Papakonstantinou, G. and Sundmacher, K. and Brüller, S. and Antonyshyn, I. and Dimitratos, N. and Davis, R.J. and Böhm, K.-H. and Fechler, N. and Freakley, S. and Grin, Y. and Gunnoe, B.T. and Haj-Hariri, H. and Hutchings, G. and Liang, H. and Mayrhofer, K.J.J. and Müllen, K. and Neese, F. and Ranjan, C. and Sankar, M. and Schlögl, R. and Schüth, F. and Spanos, I. and Stratmann, M. and Tüysüz, H. and Vidakovic-Koch, T. and Yi, Y. and Zangari, G.
GREEN. Volume: 5 (2015) - 2015 • 3519
Energy storage technologies as options to a secure energy supply
Ausfelder, F. and Beilmann, C. and Bertau, M. and Bräuninger, S. and Heinzel, A. and Hoer, R. and Koch, W. and Mahlendorf, F. and Metzelthin, A. and Peuckert, M. and Plass, L. and Räuchle, K. and Reuter, M. and Schaub, G. and Schiebahn, S. and Schwab, E. and Schüth, F. and Stolten, D. and Teßmer, G. and Wagemann, K. and Ziegahn, K.-F.
CHEMIE-INGENIEUR-TECHNIK. Volume: 87 (2015) - 2015 • 3518
Capping agent promoted oxidation of gold nanoparticles: cetyl trimethylammonium bromide
Plowman, B.J. and Tschulik, K. and Young, N.P. and Compton, R.G.
PHYSICAL CHEMISTRY CHEMICAL PHYSICS. Volume: 17 (2015) - 2015 • 3517
Shear-flow-controlled mode selection in a nonlinear autocatalytic medium
Ayodele, S.G. and Raabe, D. and Varnik, F.
PHYSICAL REVIEW E - STATISTICAL, NONLINEAR, AND SOFT MATTER PHYSICS. Volume: 91 (2015) - 2015 • 3516
Modelling and proper evaluation of volumetric kinetics of hydrogen desorption by metal hydrides
Drozdov, I.V. and Kochubey, V. and Meng, L. and Mauer, G. and Vaßen, R. and Stöver, D.
INTERNATIONAL JOURNAL OF HYDROGEN ENERGY. Volume: 40 (2015) - 2015 • 3515
The fate of nano-silver in aqueous media
Plowman, B.J. and Tschulik, K. and Walport, E. and Young, N.P. and Compton, R.G.
NANOSCALE. Volume: 7 (2015) - 2015 • 3514
The peculiarity of the metal-ceramic interface
Zhang, Z. and Long, Y. and Cazottes, S. and Daniel, R. and Mitterer, C. and Dehm, G.
SCIENTIFIC REPORTS. Volume: 5 (2015) - 2015 • 3513
Modelling and evaluation of hydrogen desorption kinetics controlled by surface reaction and bulk diffusion for magnesium hydride
Drozdov, I.V. and Vaßen, R. and Stöver, D.
RSC ADVANCES. Volume: 5 (2015) - 2015 • 3512
Implications of electron heating and non-uniformities in a VHF-CCP for sterilization of medical instruments
Stapelmann, K. and Fiebrandt, M. and Styrnoll, T. and Baldus, S. and Bibinov, N. and Awakowicz, P.
PLASMA SOURCES SCIENCE AND TECHNOLOGY. Volume: 24 (2015) - 2015 • 3511
Structural transformations among austenite, ferrite and cementite in Fe-C alloys: A unified theory based on ab initio simulations
Zhang, X. and Hickel, T. and Rogal, J. and Fähler, S. and Drautz, R. and Neugebauer, J.
ACTA MATERIALIA. Volume: 99 (2015) - 2015 • 3510
On the human blood permittivity: Model parameters and substitution material for mmWave applications
Baer, C. and Schulz, C. and Notzon, G. and Rolfes, I. and Musch, T.
2015 IEEE MTT-S INTERNATIONAL MICROWAVE WORKSHOP SERIES ON RF AND WIRELESS TECHNOLOGIES FOR BIOMEDICAL AND HEALTHCARE APPLICATIONS, IMWS-BIO 2015 - PROCEEDINGS. Volume: (2015) - 2015 • 3509
Nanoscale origins of the damage tolerance of the high-entropy alloy CrMnFeCoNi
Zhang, Z. and Mao, M.M. and Wang, J. and Gludovatz, B. and Zhang, Z. and Mao, S.X. and George, E.P. and Yu, Q. and Ritchie, R.O.
NATURE COMMUNICATIONS. Volume: 6 (2015) - 2015 • 3508
Dielectric waveguides for industrial radar applications
Baer, C. and Schulz, C. and Rolfes, I. and Musch, T.
INTERNATIONAL JOURNAL OF MICROWAVE AND WIRELESS TECHNOLOGIES. Volume: 7 (2015) - 2015 • 3507
Improved method of calculating ab initio high-temperature thermodynamic properties with application to ZrC
Duff, A.I. and Davey, T. and Korbmacher, D. and Glensk, A. and Grabowski, B. and Neugebauer, J. and Finnis, M.W.
PHYSICAL REVIEW B - CONDENSED MATTER AND MATERIALS PHYSICS. Volume: 91 (2015) - 2015 • 3506
Effect of reduction–oxidation treatment on structure and catalytic properties of ordered mesoporous Cu–Mg–Al composite oxides
Lu, J. and Zhang, Y. and Jiao, C. and Megarajan, S.K. and Gu, D. and Yang, G. and Jiang, H. and Jia, C. and Schüth, F.
SCIENCE BULLETIN. Volume: 60 (2015) - 2015 • 3505
Influence of Defects Introduced by Irradiation with 4-9 MeV Helium Ions on Impedance of Silicon Diodes
Poklonski, N.A. and Gorbachuk, N.I. and Nha, V.Q. and Shpakovski, S.V. and Filipenya, V.A. and Skuratov, V.A. and Kotunowicz, T.N. and Kukharchyk, N. and Becker, H.-W. and Wieck, A.
ACTA PHYSICA POLONICA A. Volume: 128 (2015) - 2015 • 3504
Atomistic investigation of wear mechanisms of a copper bi-crystal
Zhang, J. and Begau, C. and Geng, L. and Hartmaier, A.
WEAR. Volume: 332-333 (2015) - 2015 • 3503
Effective permittivity determination of randomized mixed materials using 3D electromagnetic simulations
Baer, C. and Hattenhorst, B. and Schulz, C. and Will, B. and Rolfes, I. and Musch, T.
2015 IEEE MTT-S INTERNATIONAL MICROWAVE SYMPOSIUM, IMS 2015. Volume: (2015) - 2015 • 3502
Ab initio-based bulk and surface thermodynamics of InGaN alloys: Investigating the effects of strain and surface polarity
Duff, A.I. and Lymperakis, L. and Neugebauer, J.
PHYSICA STATUS SOLIDI (B) BASIC RESEARCH. Volume: 252 (2015) - 2015 • 3501
Effects of boron on the fracture behavior and ductility of cast Ti-6Al-4V alloys
Luan, J.H. and Jiao, Z.B. and Heatherly, L. and George, E.P. and Chen, G. and Liu, C.T.
SCRIPTA MATERIALIA. Volume: 100 (2015) - 2015 • 3500
Difference in linear polarization of biaxially strained i nx G a1-x N alloys on nonpolar a -plane and m -plane GaN
Zhang, S. and Cui, Y. and Griffiths, J.T. and Fu, W.Y. and Freysoldt, C. and Neugebauer, J. and Humphreys, C.J. and Oliver, R.A.
PHYSICAL REVIEW B - CONDENSED MATTER AND MATERIALS PHYSICS. Volume: 92 (2015) - 2015 • 3499
Dental lessons from past to present: Ultrastructure and composition of teeth from plesiosaurs, dinosaurs, extinct and recent sharks
Lübke, A. and Enax, J. and Loza, K. and Prymak, O. and Gaengler, P. and Fabritius, H.-O. and Raabe, D. and Epple, M.
RSC ADVANCES. Volume: 5 (2015) - 2015 • 3498
Single graphene nanoplatelets: Capacitance, potential of zero charge and diffusion coefficient
Poon, J. and Batchelor-Mcauley, C. and Tschulik, K. and Compton, R.G.
CHEMICAL SCIENCE. Volume: 6 (2015) - 2015 • 3497
Autonomous Repair Mechanism of Creep Damage in Fe-Au and Fe-Au-B-N Alloys
Zhang, S. and Kwakernaak, C. and Tichelaar, F.D. and Sloof, W.G. and Kuzmina, M. and Herbig, M. and Raabe, D. and Brück, E. and van der Zwaag, S. and van Dijk, N.H.
METALLURGICAL AND MATERIALS TRANSACTIONS A: PHYSICAL METALLURGY AND MATERIALS SCIENCE. Volume: 46 (2015) - 2015 • 3496
Interplay of strain and interdiffusion in Heusler alloy bilayers
Dutta, B. and Hickel, T. and Neugebauer, J. and Behler, C. and Fähler, S. and Behler, A. and Waske, A. and Teichert, N. and Schmalhorst, J.-M. and Hütten, A.
PHYSICA STATUS SOLIDI - RAPID RESEARCH LETTERS. Volume: 9 (2015) - 2015 • 3495
On the role of gold nanoparticles in the selective photooxidation of 2-propanol over Au/TiO2
Lüken, A. and Muhler, M. and Strunk, J.
PHYSICAL CHEMISTRY CHEMICAL PHYSICS. Volume: 17 (2015) - 2015 • 3494
Bifunctional redox tagging of carbon nanoparticles
Poon, J. and Batchelor-McAuley, C. and Tschulik, K. and Palgrave, R.G. and Compton, R.G.
NANOSCALE. Volume: 7 (2015) - 2015 • 3493
A study on the geometry of dislocation patterns in the surrounding of nanoindents in a TWIP steel using electron channeling contrast imaging and discrete dislocation dynamics simulations
Zhang, J.-L. and Zaefferer, S. and Raabe, D.
MATERIALS SCIENCE AND ENGINEERING A. Volume: 636 (2015) - 2015 • 3492
Film Stress of Amorphous Hydrogenated Carbon on Biaxially Oriented Polyethylene Terephthalate
Bahre, H. and Behm, H. and Grochla, D. and Böke, M., and Dahlmann, R., and Hopmann, C., and Ludwig, Al., and Winter, J.
PLASMA PROCESSES AND POLYMERS. Volume: 12 (2015) - 2015 • 3491
Mesoporous Sulfonated Carbon Materials Prepared by Spray Pyrolysis
Duyckaerts, N. and Trotuş, I.-T. and Nese, V. and Swertz, A.-C. and Auris, S. and Wiggers, H. and Schüth, F.
CHEMCATCHEM. Volume: 7 (2015) - 2015 • 3490
Damage resistance in gum metal through cold work-induced microstructural heterogeneity
Zhang, J.-L. and Tasan, C.C. and Lai, M.L. and Zhang, J. and Raabe, D.
JOURNAL OF MATERIALS SCIENCE. Volume: 50 (2015) - 2015 • 3489
Porosity-Property Relationships of Plasma-Sprayed Gd2 Zr2 O7 /YSZ Thermal Barrier Coatings
Bakan, E. and Mack, D.E. and Mauer, G. and Mücke, R. and Vaßen, R.
JOURNAL OF THE AMERICAN CERAMIC SOCIETY. Volume: 98 (2015) - 2015 • 3488
Structural and magnetic phase transitions near optimal superconductivity in BaFe2 (As1-x Px)2
Hu, D. and Lu, X. and Zhang, W. and Luo, H. and Li, S. and Wang, P. and Chen, G. and Han, F. and Banjara, S.R. and Sapkota, A. and Kreyssig, A. and Goldman, A.I. and Yamani, Z. and Niedermayer, C. and Skoulatos, M. and Georgii, R. and Keller, T. and Wang, P. and Yu, W. and Dai, P.
PHYSICAL REVIEW LETTERS. Volume: 114 (2015) - 2015 • 3487
Dynamic strain-induced transformation: An atomic scale investigation
Zhang, H. and Pradeep, K.G. and Mandal, S. and Ponge, D. and Springer, H. and Raabe, D.
SCRIPTA MATERIALIA. Volume: 109 (2015) - 2015 • 3486
Importance of coordination number and bond length in titanium revealed by electronic structure investigations
Huang, L.-F. and Grabowski, B. and Mceniry, E. and Trinkle, D.R. and Neugebauer, J.
PHYSICA STATUS SOLIDI (B) BASIC RESEARCH. Volume: 252 (2015) - 2015 • 3485
A structure zone diagram obtained by simultaneous deposition on a novel step heater: A case study for Cu2O thin films
Stein, H. and Naujoks, D. and Grochla, D. and Khare, C. and Gutkowski, R. and Grützke, S. and Schuhmann, W. and Ludwig, Al.
PHYSICA STATUS SOLIDI (A) APPLICATIONS AND MATERIALS SCIENCE. Volume: 212 (2015) - 2015 • 3484
Incorporating the CALPHAD sublattice approach of ordering into the phase-field model with finite interface dissipation
Zhang, L. and Stratmann, M. and Du, Y. and Sundman, B. and Steinbach, I.
ACTA MATERIALIA. Volume: 88 (2015) - 2015 • 3483
Activation of carbon-supported catalysts by ozonized acidic solutions for the direct implementation in (electro-)chemical reactors
Baldizzone, C. and Mezzavilla, S. and Hodnik, N. and Zeradjanin, A.R. and Kostka, A. and Schüth, F. and Mayrhofer, K.J.J.
CHEMICAL COMMUNICATIONS. Volume: 51 (2015) - 2015 • 3482
On the reactions of cyclohexyl phenyl sulfide with water by means of density functional theory
Lysogorskiy, Yu.V. and Aminova, R.M. and Tayurskii, D.A.
AIP CONFERENCE PROCEEDINGS. Volume: 1702 (2015) - 2015 • 3481
Diamond single micro-crystals and graphitic micro-balls' formation in plasmoids under atmospheric pressure
Pothiraja, R. and Kartaschew, K. and Bibinov, N. and Havenith, M. and Awakowicz, P.
JOURNAL OF PHYSICS D: APPLIED PHYSICS. Volume: 48 (2015) - 2015 • 3480
Light Induced H2 Evolution from a Biophotocathode Based on Photosystem 1 - Pt Nanoparticles Complexes Integrated in Solvated Redox Polymers Films
Zhao, F. and Conzuelo, F. and Hartmann, V. and Li, H. and Nowaczyk, M.M. and Plumeré, N. and Rögner, M. and Schuhmann, W.
JOURNAL OF PHYSICAL CHEMISTRY B. Volume: 119 (2015) - 2015 • 3479
Stability of Dealloyed Porous Pt/Ni Nanoparticles
Baldizzone, C. and Gan, L. and Hodnik, N. and Keeley, G.P. and Kostka, A. and Heggen, M. and Strasser, P. and Mayrhofer, K.J.J.
ACS CATALYSIS. Volume: 5 (2015) - 2015 • 3478
Vibrational and magnetic properties of crystalline CuTe2O5
Lysogorskiy, Y.V. and Eremina, R.M. and Gavrilova, T.P. and Nedopekin, O.V. and Tayurskii, D.A.
JETP LETTERS. Volume: 100 (2015) - 2015 • 3477
Molecular-scale hybridization of clay monolayers and conducting polymer for thin-film supercapacitors
Zhao, J. and Xu, S. and Tschulik, K. and Compton, R.G. and Wei, M. and O'Hare, D. and Evans, D.G. and Duan, X.
ADVANCED FUNCTIONAL MATERIALS. Volume: 25 (2015) - 2015 • 3476
Phase resolved analysis of the homogeneity of a diffuse dielectric barrier discharge
Baldus, S. and Kogelheide, F. and Bibinov, N. and Stapelmann, K. and Awakowicz, P.
JOURNAL OF PHYSICS D: APPLIED PHYSICS. Volume: 48 (2015) - 2015 • 3475
Atomic scale study of CU clustering and pseudo-homogeneous Fe-Si nanocrystallization in soft magnetic FeSiNbB(CU) alloys
Pradeep, K.G. and Herzer, G. and Raabe, D.
ULTRAMICROSCOPY. Volume: 159 (2015) - 2015 • 3474
Very low amount of TiO2 on N-doped carbon nanotubes significantly improves oxygen reduction activity and stability of supported Pt nanoparticles
Zhao, A. and Masa, J. and Xia, W.
PHYSICAL CHEMISTRY CHEMICAL PHYSICS. Volume: 17 (2015) - 2015 • 3473
Atomic oxygen dynamics in an air dielectric barrier discharge: A combined diagnostic and modeling approach
Baldus, S. and Schröder, D. and Bibinov, N. and Schulz-Von Der Gathen, V. and Awakowicz, P.
JOURNAL OF PHYSICS D: APPLIED PHYSICS. Volume: 48 (2015) - 2015 • 3472
A study of deformation and phase transformation coupling for TRIP-assisted steels
Ma, A. and Hartmaier, A.
INTERNATIONAL JOURNAL OF PLASTICITY. Volume: 64 (2015) - 2015 • 3471
Non-equiatomic high entropy alloys: Approach towards rapid alloy screening and property-oriented design
Pradeep, K.G. and Tasan, C.C. and Yao, M.J. and Deng, Y. and Springer, H. and Raabe, D.
MATERIALS SCIENCE AND ENGINEERING A. Volume: 648 (2015) - 2015 • 3470
Numerical calculation of thermo-mechanical problems at large strains based on complex step derivative approximation of tangent stiffness matrices
Balzani, D. and Gandhi, A. and Tanaka, M. and Schröder, J.
COMPUTATIONAL MECHANICS. Volume: 55 (2015) - 2015 • 3469
Ab initio thermodynamics of the CoCrFeMnNi high entropy alloy: Importance of entropy contributions beyond the configurational one
Ma, D. and Grabowski, B. and Körmann, F. and Neugebauer, J. and Raabe, D.
ACTA MATERIALIA. Volume: 100 (2015) - 2015 • 3468
Atom probe informed simulations of dislocation-precipitate interactions reveal the importance of local interface curvature
Prakash, A. and Guénolé, J. and Wang, J. and Müller, J. and Spiecker, E. and Mills, M.J. and Povstugar, I. and Choi, P. and Raabe, D. and Bitzek, E.
ACTA MATERIALIA. Volume: 92 (2015) - 2015 • 3467
Tuning the tunneling probability between low-dimensional electron systems by momentum matching
Zhou, D. and Beckel, A. and Ludwig, Ar. and Wieck, A.D. and Geller, M. and Lorke, A.
APPLIED PHYSICS LETTERS. Volume: 106 (2015) - 2015 • 3466
Comparative analysis of damage functions for soft tissues: Properties at damage initialization
Balzani, D. and Schmidt, T.
MATHEMATICS AND MECHANICS OF SOLIDS. Volume: 20 (2015) - 2015 • 3465
Ab initio study of compositional trends in solid solution strengthening in metals with low Peierls stresses
Ma, D. and Friák, M. and Von Pezold, J. and Neugebauer, J. and Raabe, D.
ACTA MATERIALIA. Volume: 98 (2015) - 2015 • 3464
Mutual Independence of Critical Temperature and Superfluid Density under Pressure in Optimally Electron-Doped Superconducting LaFeAsO1-xFx
Prando, G. and Hartmann, Th. and Schottenhamel, W. and Guguchia, Z. and Sanna, S. and Ahn, F. and Nekrasov, I. and Blum, C.G.F. and Wolter, A.U.B. and Wurmehl, S. and Khasanov, R. and Eremin, I. and Büchner, B.
PHYSICAL REVIEW LETTERS. Volume: 114 (2015) - 2015 • 3463
Interstitial iron impurities at cores of dissociated dislocations in silicon
Ziebarth, B. and Mrovec, M. and Elsässer, C. and Gumbsch, P.
PHYSICAL REVIEW B - CONDENSED MATTER AND MATERIALS PHYSICS. Volume: 92 (2015) - 2015 • 3462
Non-Thermal Dielectric Barrier Discharge (DBD) effects on proliferation and differentiation of human fibroblasts are primary mediated by hydrogen peroxide
Balzer, J. and Heuer, K. and Demir, E. and Hoffmanns, M.A. and Baldus, S. and Fuchs, P.C. and Awakowicz, P. and Suschek, C.V. and Opländer, C.
PLOS ONE. Volume: 10 (2015) - 2015 • 3461
The effect of insulator nano-sheath thickness on the steady state current at a micro-disc electrode
Ellison, J. and Eloul, S. and Batchelor-Mcauley, C. and Tschulik, K. and Salter, C. and Compton, R.G.
JOURNAL OF ELECTROANALYTICAL CHEMISTRY. Volume: 745 (2015) - 2015 • 3460
Computationally efficient and quantitatively accurate multiscale simulation of solid-solution strengthening by ab initio calculation
Ma, D. and Friák, M. and Von Pezold, J. and Raabe, D. and Neugebauer, J.
ACTA MATERIALIA. Volume: 85 (2015) - 2015 • 3459
Electrically tunable hole g factor of an optically active quantum dot for fast spin rotations
Prechtel, J.H. and Maier, F. and Houel, J. and Kuhlmann, A.V. and Ludwig, Ar. and Wieck, A.D. and Loss, D. and Warburton, R.J.
PHYSICAL REVIEW B - CONDENSED MATTER AND MATERIALS PHYSICS. Volume: 91 (2015) - 2015 • 3458
Influence of dislocation strain fields on the diffusion of interstitial iron impurities in silicon
Ziebarth, B. and Mrovec, M. and Elsässer, C. and Gumbsch, P.
PHYSICAL REVIEW B - CONDENSED MATTER AND MATERIALS PHYSICS. Volume: 92 (2015) - 2015 • 3457
Passively mode-locked diode laser with optimized dispersion management
Balzer, J.C. and Pilny, R.H. and Döpke, B. and Klehr, A. and Erbert, G. and Tränkle, G. and Brenner, C. and Hofmann, M.R.
IEEE JOURNAL ON SELECTED TOPICS IN QUANTUM ELECTRONICS. Volume: 21 (2015) - 2015 • 3456
In Situ TEM Microcompression of Single and Bicrystalline Samples: Insights and Limitations
Imrich, P.J. and Kirchlechner, C. and Kiener, D. and Dehm, G.
JOM. Volume: 67 (2015) - 2015 • 3455
Analytical bounds of in-plane Young's modulus and full-field simulations of two-dimensional monocrystalline stochastic honeycomb structures
Ma, D. and Eisenlohr, P. and Shanthraj, P. and Diehl, M. and Roters, F. and Raabe, D.
COMPUTATIONAL MATERIALS SCIENCE. Volume: 109 (2015) - 2015 • 3454
Detection of Multiple Structural Breaks in Multivariate Time Series
Preuss, P. and Puchstein, R. and Dette, H.
JOURNAL OF THE AMERICAN STATISTICAL ASSOCIATION. Volume: 110 (2015) - 2015 • 3453
Complex Nanotwin Substructure of an Asymmetric Σ9 Tilt Grain Boundary in a Silicon Polycrystal
Stoffers, A. and Ziebarth, B. and Barthel, J. and Cojocaru-Mirédin, O. and Elsässer, C. and Raabe, D.
PHYSICAL REVIEW LETTERS. Volume: 115 (2015) - 2015 • 3452
Interstitial iron impurities at grain boundaries in silicon: A first-principles study
Ziebarth, B. and Mrovec, M. and Elsässer, C. and Gumbsch, P.
PHYSICAL REVIEW B - CONDENSED MATTER AND MATERIALS PHYSICS. Volume: 91 (2015) - 2015 • 3451
Diffusional impacts of nanoparticles on microdisc and microwire electrodes: The limit of detection and first passage statistics
Eloul, S. and Kätelhön, E. and Batchelor-McAuley, C. and Tschulik, K. and Compton, R.G.
JOURNAL OF ELECTROANALYTICAL CHEMISTRY. Volume: 755 (2015) - 2015 • 3450
Influence of inclined twin boundaries on the deformation behavior of Cu micropillars
Imrich, P.J. and Kirchlechner, C. and Dehm, G.
MATERIALS SCIENCE AND ENGINEERING A. Volume: 642 (2015) - 2015 • 3449
Phase stability of non-equiatomic CoCrFeMnNi high entropy alloys
Ma, D. and Yao, M. and Pradeep, K.G. and Tasan, C.C. and Springer, H. and Raabe, D.
ACTA MATERIALIA. Volume: 98 (2015) - 2015 • 3448
Grain boundary segregation in multicrystalline silicon: Correlative characterization by EBSD, EBIC, and atom probe tomography
Stoffers, A. and Cojocaru-Mirédin, O. and Seifert, W. and Zaefferer, S. and Riepe, S. and Raabe, D.
PROGRESS IN PHOTOVOLTAICS: RESEARCH AND APPLICATIONS. Volume: 23 (2015) - 2015 • 3447
Formation and stabilization of ZnO nanoparticles inside MCM-48 porous support via post-synthetic organometallic route
Bandyopadhyay, M. and Gies, H. and Grünert, W. and Van Den Berg, M. and Birkner, A.
ADVANCED MATERIALS LETTERS. Volume: 6 (2015) - 2015 • 3446
Diffusional nanoimpacts: The stochastic limit
Eloul, S. and Kätelhön, E. and Batchelor-Mcauley, C. and Tschulik, K. and Compton, R.G.
JOURNAL OF PHYSICAL CHEMISTRY C. Volume: 119 (2015) - 2015 • 3445
Internal and external stresses: In situ TEM compression of Cu bicrystals containing a twin boundary
Imrich, P.J. and Kirchlechner, C. and Kiener, D. and Dehm, G.
SCRIPTA MATERIALIA. Volume: 100 (2015) - 2015 • 3444
Hybrid architecture for shallow accumulation mode AlGaAs/GaAs heterostructures with epitaxial gates
Macleod, S.J. and See, A.M. and Hamilton, A.R. and Farrer, I. and Ritchie, D.A. and Ritzmann, J. and Ludwig, Ar. and Wieck, A.D.
APPLIED PHYSICS LETTERS. Volume: 106 (2015) - 2015 • 3443
Bridging the gap between insightful simplicity and successful complexity: From fundamental studies on model systems to technical catalysts
Prieto, G. and Schüth, F.
JOURNAL OF CATALYSIS. Volume: 328 (2015) - 2015 • 3442
Martensitic transformation in Eurofer-97 and ODS-Eurofer steels: A comparative study
Zilnyk, K.D. and Oliveira, V.B. and Sandim, H.R.Z. and Möslang, A. and Raabe, D.
JOURNAL OF NUCLEAR MATERIALS. Volume: 462 (2015) - 2015 • 3441
The nucleation of Mo-rich Laves phase particles adjacent to M23C6 micrograin boundary carbides in 12% Cr tempered martensite ferritic steels
Isik, M.I. and Kostka, A. and Yardley, V.A. and Pradeep, K.G. and Duarte, M.J. and Choi, P.P. and Raabe, D. and Eggeler, G.
ACTA MATERIALIA. Volume: 90 (2015) - 2015 • 3440
Effect of the specific surface area on thermodynamic and kinetic properties of nanoparticle anatase TiO2 in lithium-ion batteries
Madej, E. and Klink, S. and Schuhmann, W. and Ventosa, E. and La Mantia, F.
JOURNAL OF POWER SOURCES. Volume: 297 (2015) - 2015 • 3439
The Yin and Yang in the development of catalytic processes: Catalysis research and reaction engineering
Prieto, G. and Schüth, F.
ANGEWANDTE CHEMIE - INTERNATIONAL EDITION. Volume: 54 (2015) - 2015 • 3438
Electrochemical detection of synthetic DNA and native 16S rRNA fragments on a microarray using a biotinylated intercalator as coupling site for an enzyme label
Zimdars, A. and Gebala, M. and Hartwich, G. and Neugebauer, S. and Schuhmann, W.
TALANTA. Volume: 143 (2015) - 2015 • 3437
Benchmarking of process models for continuous screening based on discrete element simulations
Elskamp, F. and Kruggel-Emden, H. and Hennig, M. and Teipel, U.
MINERALS ENGINEERING. Volume: 83 (2015) - 2015 • 3436
Review and benchmarking of process models for batch screening based on discrete element simulations
Elskamp, F. and Kruggel-Emden, H.
ADVANCED POWDER TECHNOLOGY. Volume: 26 (2015) - 2015 • 3435
Confidence bands for multivariate and time dependent inverse regression models
Proksch, K. and Bissantz, N. and Dette, H.
BERNOULLI. Volume: 21 (2015) - 2015 • 3434
Characterizing surface profiles utilizing mm-wave FMCW SAR imaging
Barowski, J. and Pohle, D. and Jaeschke, T. and Pohl, N. and Rolfes, I.
EUROPEAN MICROWAVE WEEK 2015: "FREEDOM THROUGH MICROWAVES", EUMW 2015 - CONFERENCE PROCEEDINGS; 2015 45TH EUROPEAN MICROWAVE CONFERENCE PROCEEDINGS, EUMC. Volume: (2015) - 2015 • 3433
Degradations in concrete due to cyclic loading and its effects on transport processes with regard to ASR damage
Przondziono, R. and Timothy, J.J. and Nguyen, M. and Weise, F. and Breitenbücher, R. and Meschke, G. and Meng, B.
BETON- UND STAHLBETONBAU. Volume: 110 (2015) - 2015 • 3432
Non-destructive Patterning of Carbon Electrodes by Using the Direct Mode of Scanning Electrochemical Microscopy
Stratmann, L. and Clausmeyer, J. and Schuhmann, W.
CHEMPHYSCHEM. Volume: 16 (2015) - 2015 • 3431
Vertical-cavity surface-emitting lasers with birefringence splitting above 250 GHz
Pusch, T. and Lindemann, M. and Gerhardt, N.C. and Hofmann, M.R. and Michalzik, R.
ELECTRONICS LETTERS. Volume: 51 (2015) - 2015 • 3430
Linear micromechanical stepping drive for pinhole array positioning
Endrödy, C. and Mehner, H. and Grewe, A. and Hoffmann, M.
JOURNAL OF MICROMECHANICS AND MICROENGINEERING. Volume: 25 (2015) - 2015 • 3429
On the widths of the hysteresis of mechanically and thermally induced martensitic transformations in Ni-Ti-based shape memory alloys
Jaeger, S. and Maaß, B. and Frenzel, J. and Schmidt, M. and Ullrich, J. and Seelecke, S. and Schütze, A. and Kastner, O. and Eggeler, G.
INTERNATIONAL JOURNAL OF MATERIALS RESEARCH. Volume: 106 (2015) - 2015 • 3428
2D stepping drive for hyperspectral systems
Endrödy, C. and Mehner, H. and Grewe, A. and Sinzinger, S. and Hoffmann, M.
JOURNAL OF MICROMECHANICS AND MICROENGINEERING. Volume: 25 (2015) - 2015 • 3427
Modeling thermally induced martensitic transformations in nickel titanium shape memory alloys
Jaeger, S. and Eggeler, G. and Kastner, O.
CONTINUUM MECHANICS AND THERMODYNAMICS. Volume: 27 (2015) - 2015 • 3426
Dislocation multiplication mechanisms - Glissile junctions and their role on the plastic deformation at the microscale
Stricker, M. and Weygand, D.
ACTA MATERIALIA. Volume: 99 (2015) - 2015 • 3425
Metal-halide Nanoparticle Formation: Electrolytic and Chemical Synthesis of Mercury(I) Chloride Nanoparticles
Bartlett, T.R. and Batchelor-Mcauley, C. and Tschulik, K. and Jurkschat, K. and Compton, R.G.
CHEMELECTROCHEM. Volume: 2 (2015) - 2015 • 3424
Codeposited Poly(benzoxazine) and Os-Complex Modified Polymethacrylate Layers as Immobilization Matrix for Glucose Biosensors
Barwe, S. and Andronescu, C. and Pöller, S. and Schuhmann, W.
ELECTROANALYSIS. Volume: 27 (2015) - 2015 • 3423
Synergistic stabilization of metastable Fe23B6 and γ -Fe in undercooled Fe83B17
Quirinale, D.G. and Rustan, G.E. and Kreyssig, A. and Goldman, A.I.
APPLIED PHYSICS LETTERS. Volume: 106 (2015) - 2015 • 3422
Large magnetocaloric effects in magnetic intermetallics: First-principles and Monte Carlo studies
Entel, P. and Gruner, M.E. and Ogura, M. and Sokolovskiy, V.V. and Buchelnikov, V.D. and Grünebohm, A. and Arróyave, R. and Uebayashi, K. and Singh, N. and Talapatra, A. and Duong, T. and Acet, M. and Çakir, A.
MATEC WEB OF CONFERENCES. Volume: 33 (2015) - 2015 • 3421
High-Quality Solution-Processed Silicon Oxide Gate Dielectric Applied on Indium Oxide Based Thin-Film Transistors
Jaehnike, F. and Pham, D.V. and Anselmann, R. and Bock, C. and Kunze, U.
ACS APPLIED MATERIALS AND INTERFACES. Volume: 7 (2015) - 2015 • 3420
Classification of the pre-settlement behaviour of barnacle cyprids
Maleschlijski, S. and Bauer, S. and Aldred, N. and Clare, A.S. and Rosenhahn, A.
JOURNAL OF THE ROYAL SOCIETY INTERFACE. Volume: 12 (2015) - 2015 • 3419
Photonic crystal cavities with metallic Schottky contacts
Quiring, W. and Al-Hmoud, M. and Rai, A. and Reuter, D. and Wieck, A.D. and Zrenner, A.
APPLIED PHYSICS LETTERS. Volume: 107 (2015) - 2015 • 3418
Effects of various chair-side surface treatment methods on dental restorative materials with respect to contact angles and surface roughness
Sturz, C.R.C. and Faber, F.-J. and Scheer, M. and Rothamel, D. and Neugebauer, J.
DENTAL MATERIALS JOURNAL. Volume: 34 (2015) - 2015 • 3417
In situ nanoparticle sizing with zeptomole sensitivity
Batchelor-Mcauley, C. and Ellison, J. and Tschulik, K. and Hurst, P.L. and Boldt, R. and Compton, R.G.
ANALYST. Volume: 140 (2015) - 2015 • 3416
The metamagnetic behavior and giant inverse magnetocaloric effect in Ni-Co-Mn-(Ga, In, Sn) Heusler alloys
Entel, P. and Sokolovskiy, V.V. and Buchelnikov, V.D. and Ogura, M. and Gruner, M.E. and Grünebohm, A. and Comtesse, D. and Akai, H.
JOURNAL OF MAGNETISM AND MAGNETIC MATERIALS. Volume: 385 (2015) - 2015 • 3415
Modeling the flow in diffuse interface methods of solidification
Subhedar, A. and Steinbach, I. and Varnik, F.
PHYSICAL REVIEW E - STATISTICAL, NONLINEAR, AND SOFT MATTER PHYSICS. Volume: 92 (2015) - 2015 • 3414
A first principles investigation of zinc induced embrittlement at grain boundaries in bcc iron
Bauer, K.-D. and Todorova, M. and Hingerl, K. and Neugebauer, J.
ACTA MATERIALIA. Volume: 90 (2015) - 2015 • 3413
A new hybrid scheme for simulations of highly collisional RF-driven plasmas
Eremin, D. and Hemke, T. and Mussenbrock, T.
PLASMA SOURCES SCIENCE AND TECHNOLOGY. Volume: 25 (2015) - 2015 • 3412
Infrared Study of the Spin Reorientation Transition and Its Reversal in the Superconducting State in Underdoped Ba1-x KxFe2As2
Mallett, B.P.P. and Marsik, P. and Yazdi-Rizi, M. and Wolf, Th. and Böhmer, A.E. and Hardy, F. and Meingast, C. and Munzar, D. and Bernhard, C.
PHYSICAL REVIEW LETTERS. Volume: 115 (2015) - 2015 • 3411
Convective drying of agitated silica gel and beech wood particle beds—experiments and transient DEM-CFD simulations
Sudbrock, F. and Kruggel-Emden, H. and Wirtz, S. and Scherer, V.
DRYING TECHNOLOGY. Volume: 33 (2015) - 2015 • 3410
Nonlocal behavior of the excitation rate in highly collisional RF discharges
Eremin, D. and Hemke, T. and Mussenbrock, T.
PLASMA SOURCES SCIENCE AND TECHNOLOGY. Volume: 24 (2015) - 2015 • 3409
Potential-Assisted DNA Immobilization as a Prerequisite for Fast and Controlled Formation of DNA Monolayers
Jambrec, D. and Gebala, M. and La Mantia, F. and Schuhmann, W.
ANGEWANDTE CHEMIE - INTERNATIONAL EDITION. Volume: 54 (2015) - 2015 • 3408
Monitoring Local Strain in a Thermal Barrier Coating System Under Thermal Mechanical Gas Turbine Operating Conditions
Manero, A., II and Sofronsky, S. and Knipe, K. and Meid, C. and Wischek, J. and Okasinski, J. and Almer, J. and Karlsson, A.M. and Raghavan, S. and Bartsch, M.
JOM. Volume: 67 (2015) - 2015 • 3407
Atom probe tomography reveals options for microstructural design of steels and titanium alloys by segregation engineering
Raabe, D. and Herbig, M. and Kuzmina, M. and Sandlöbes, S. and Tarzimoghadam, Z. and Ponge, D.
MATEC WEB OF CONFERENCES. Volume: 33 (2015) - 2015 • 3406
One-Pot Synthesis of Carbon-Coated Nanostructured Iron Oxide on Few-Layer Graphene for Lithium-Ion Batteries
Sun, Z. and Madej, E. and Wiktor, C. and Sinev, I. and Fischer, R.A. and Van Tendeloo, G. and Muhler, M. and Schuhmann, W. and Ventosa, E.
CHEMISTRY - A EUROPEAN JOURNAL. Volume: 21 (2015) - 2015 • 3405
From high-entropy alloys to high-entropy steels
Raabe, D. and Tasan, C.C. and Springer, H. and Bausch, M.
STEEL RESEARCH INTERNATIONAL. Volume: 86 (2015) - 2015 • 3404
High-quality functionalized few-layer graphene: Facile fabrication and doping with nitrogen as a metal-free catalyst for the oxygen reduction reaction
Sun, Z. and Masa, J. and Weide, P. and Fairclough, S.M. and Robertson, A.W. and Ebbinghaus, P. and Warner, J.H. and Tsang, S.C.E. and Muhler, M. and Schuhmann, W.
JOURNAL OF MATERIALS CHEMISTRY A. Volume: 3 (2015) - 2015 • 3403
Comparison of thermal barrier coating stresses via high energy X-rays and piezospectroscopy
Manero, A., II and Sofronsky, S., II and Knipe, K. and Lacdao, C. and Smith, M. and Meid, C. and Wischek, J. and Okasinksi, J. and Almer, J. and Karlsson, A.M. and Bartsch, M. and Raghavan, S.
53RD AIAA AEROSPACE SCIENCES MEETING. Volume: (2015) - 2015 • 3402
Equivalent plastic strain gradient plasticity with grain boundary hardening and comparison to discrete dislocation dynamics
Bayerschen, E. and Stricker, M. and Wulfinghoff, S. and Weygand, D. and Böhlke, T.
PROCEEDINGS OF THE ROYAL SOCIETY A: MATHEMATICAL, PHYSICAL AND ENGINEERING SCIENCES. Volume: 471 (2015) - 2015 • 3401
Mechanisms and kinetics of the migration of grain boundaries containing extended defects
Race, C.P. and Hadian, R. and Von Pezold, J. and Grabowski, B. and Neugebauer, J.
PHYSICAL REVIEW B - CONDENSED MATTER AND MATERIALS PHYSICS. Volume: 92 (2015) - 2015 • 3400
Robust population inversion by polarization selective pulsed excitation
Mantei, D. and Förstner, J. and Gordon, S. and Leier, Y.A. and Rai, A.K. and Reuter, D. and Wieck, A.D. and Zrenner, A.
SCIENTIFIC REPORTS. Volume: 5 (2015) - 2015 • 3399
Mechanism of a molecular photo-switch adsorbed on Si(100)
Bazarnik, M. and Jurczyszyn, L. and Czajka, R. and Morgenstern, K.
PHYSICAL CHEMISTRY CHEMICAL PHYSICS. Volume: 17 (2015) - 2015 • 3398
Note: Ion-induced secondary electron emission from oxidized metal surfaces measured in a particle beam reactor
Marcak, A. and Corbella, C. and de los Arcos, T. and von Keudell, A.
REVIEW OF SCIENTIFIC INSTRUMENTS. Volume: 86 (2015) - 2015 • 3397
Rabi oscillations in an InAs quantum dot ensemble observed in pre-pulse 2D coherent spectroscopy
Suzuki, T. and Singh, R. and Akimov, I.A. and Bayer, M. and Reuter, D. and Wieck, A.D. and Cundiff, S.T.
SPRINGER PROCEEDINGS IN PHYSICS. Volume: 162 (2015) - 2015 • 3396
Transition from shear to stress-assisted diffusion of copper-chromium nanolayered thin films at elevated temperatures
Raghavan, R. and Wheeler, J.M. and Harzer, T.P. and Chawla, V. and Djaziri, S. and Thomas, K. and Philippi, B. and Kirchlechner, C. and Jaya, B.N. and Wehrs, J. and Michler, J. and Dehm, G.
ACTA MATERIALIA. Volume: 100 (2015) - 2015 • 3395
Doping Level of Boron-Doped Diamond Electrodes Controls the Grafting Density of Functional Groups for DNA Assays
Švorc, L. and Jambrec, D. and Vojs, M. and Barwe, S. and Clausmeyer, J. and Michniak, P. and Marton, M. and Schuhmann, W.
ACS APPLIED MATERIALS AND INTERFACES. Volume: 7 (2015) - 2015 • 3394
Near conductive cooling rates in the upper-plutonic section of crust formed at the East Pacific Rise
Faak, K. and Coogan, L.A. and Chakraborty, S.
EARTH AND PLANETARY SCIENCE LETTERS. Volume: 423 (2015) - 2015 • 3393
Numerical modelling of the normal adhesive elastic-plastic interaction of a bacterium
Jasevičius, R. and Baronas, R. and Kruggel-Emden, H.
ADVANCED POWDER TECHNOLOGY. Volume: 26 (2015) - 2015 • 3392
Quantitative chemical-structure evaluation using atom probe tomography: Short-range order analysis of Fe-Al
Marceau, R.K.W. and Ceguerra, A.V. and Breen, A.J. and Raabe, D. and Ringer, S.P.
ULTRAMICROSCOPY. Volume: 157 (2015) - 2015 • 3391
Comparing small scale plasticity of copper-chromium nanolayered and alloyed thin films at elevated temperatures
Raghavan, R. and Harzer, T.P. and Chawla, V. and Djaziri, S. and Phillipi, B. and Wehrs, J. and Wheeler, J.M. and Michler, J. and Dehm, G.
ACTA MATERIALIA. Volume: 93 (2015) - 2015 • 3390
Modelling of the normal elastic dissipative interaction of a S. Aureus Bacterium
Jasevičius, R. and Baronas, R. and Kačianauskas, R. and Kruggel-Emden, H.
AIP CONFERENCE PROCEEDINGS. Volume: 1648 (2015) - 2015 • 3389
Atom probe tomography investigation of heterogeneous short-range ordering in the 'komplex' phase state (K-state) of Fe-18Al (at.%)
Marceau, R.K.W. and Ceguerra, A.V. and Breen, A.J. and Palm, M. and Stein, F. and Ringer, S.P. and Raabe, D.
INTERMETALLICS. Volume: 64 (2015) - 2015 • 3388
Can microscale fracture tests provide reliable fracture toughness values? A case study in silicon
Jaya, B.N. and Kirchlechner, C. and Dehm, G.
JOURNAL OF MATERIALS RESEARCH. Volume: 30 (2015) - 2015 • 3387
Adaptive dynamic load-balancing with irregular domain decomposition for particle simulations
Begau, C. and Sutmann, G.
COMPUTER PHYSICS COMMUNICATIONS. Volume: 190 (2015) - 2015 • 3386
Pressure-induced collapsed-tetragonal phase in SrCo2As2
Jayasekara, W.T. and Kaluarachchi, U.S. and Ueland, B.G. and Pandey, A. and Lee, Y.B. and Taufour, V. and Sapkota, A. and Kothapalli, K. and Sangeetha, N.S. and Fabbris, G. and Veiga, L.S.I. and Feng, Y. and Dos Santos, A.M. and Bud'Ko, S.L. and Harmon, B.N. and Canfield, P.C. and Johnston, D.C. and Kreyssig, A. and Goldman, A.I.
PHYSICAL REVIEW B - CONDENSED MATTER AND MATERIALS PHYSICS. Volume: 92 (2015) - 2015 • 3385
Geometrically nonlinear transient analysis of delaminated composite and sandwich plates using a layerwise displacement model with contact conditions
Marjanović, M. and Vuksanović, D. and Meschke, G.
COMPOSITE STRUCTURES. Volume: 122 (2015) - 2015 • 3384
Vegetable oil-based polybenzoxazine derivatives coatings on Zn-Mg-Al alloy coated steel
Raicopol, M. and Bălănucă, B. and Sliozberg, K. and Schlüter, B. and Gârea, S.A. and Chira, N. and Schuhmann, W. and Andronescu, C.
CORROSION SCIENCE. Volume: 100 (2015) - 2015 • 3383
Constructing high-dimensional neural network potentials: A tutorial review
Behler, J.
INTERNATIONAL JOURNAL OF QUANTUM CHEMISTRY. Volume: 115 (2015) - 2015 • 3382
Formation of dislocation networks in a coherent Cu Σ3(1 1 1) twin boundary
Jeon, J.B. and Dehm, G.
SCRIPTA MATERIALIA. Volume: 102 (2015) - 2015 • 3381
Double-pulse injection seeding of a terahertz quantum cascade laser
Markmann, S. and Nong, H. and Pal, S. and Hekmat, N. and Scholz, S. and Kukharchyk, N. and Ludwig, Ar. and Dhillon, S. and Tignon, J. and Marcadet, X. and Bock, C. and Kunze, U. and Wieck, A.D. and Jukam, N.
IRMMW-THZ 2015 - 40TH INTERNATIONAL CONFERENCE ON INFRARED, MILLIMETER, AND TERAHERTZ WAVES. Volume: (2015) - 2015 • 3380
Spectral modification of the laser emission of a terahertz quantum cascade laser induced by broad-band double pulse injection seeding
Markmann, S. and Nong, H. and Pal, S. and Hekmat, N. and Scholz, S. and Kukharchyk, N. and Ludwig, Ar. and Dhillon, S. and Tignon, J. and Marcadet, X. and Bock, C. and Kunze, U. and Wieck, A.D. and Jukam, N.
APPLIED PHYSICS LETTERS. Volume: 107 (2015) - 2015 • 3379
Error analysis of the crystal orientations and disorientations obtained by the classical electron backscatter diffraction technique
Ram, F. and Zaefferer, S. and Jäpel, T. and Raabe, D.
JOURNAL OF APPLIED CRYSTALLOGRAPHY. Volume: 48 (2015) - 2015 • 3378
Observation of time-resolved gain dynamics in a terahertz quantum cascade laser
Markmann, S. and Nong, H. and Pal, S. and Hekmat, N. and Mohandas, R.A. and Dean, P. and Li, L. and Linfield, E.H. and Davies, A.G. and Wieck, A.D. and Jukam, N.
IRMMW-THZ 2015 - 40TH INTERNATIONAL CONFERENCE ON INFRARED, MILLIMETER, AND TERAHERTZ WAVES. Volume: (2015) - 2015 • 3377
Dependence of alloying and island composition on terrace width: Growth of Cu on Ag(100)
Beichert, A. and Zaum, C. and Morgenstern, K.
PHYSICAL REVIEW B - CONDENSED MATTER AND MATERIALS PHYSICS. Volume: 92 (2015) - 2015 • 3376
New approaches to nanoparticle sample fabrication for atom probe tomography
Felfer, P. and Li, T. and Eder, K. and Galinski, H. and Magyar, A.P. and Bell, D.C. and Smith, G.D.W. and Kruse, N. and Ringer, S.P. and Cairney, J.M.
ULTRAMICROSCOPY. Volume: 159 (2015) - 2015 • 3375
Investigation of time-resolved gain dynamics in an injection seeded terahertz quantum cascade laser
Markmann, S. and Nong, H. and Pal, S. and Hekmat, N. and Dean, P. and Mohandas, R.A. and Li, L. and Linfield, E.H. and Giles Davis, A. and Wieck, A.D. and Jukam, N.
CLEO: SCIENCE AND INNOVATIONS, CLEO-SI 2015. Volume: (2015) - 2015 • 3374
Fluctuating multicomponent lattice Boltzmann model
Belardinelli, D. and Sbragaglia, M. and Biferale, L. and Gross, M. and Varnik, F.
PHYSICAL REVIEW E - STATISTICAL, NONLINEAR, AND SOFT MATTER PHYSICS. Volume: 91 (2015) - 2015 • 3373
The subtleties of the reversible hydrogen evolution reaction arising from the nonunity stoichiometry
Jiao, X. and Batchelor-Mcauley, C. and Kätelhön, E. and Ellison, J. and Tschulik, K. and Compton, R.G.
JOURNAL OF PHYSICAL CHEMISTRY C. Volume: 119 (2015) - 2015 • 3372
Numerical modeling of damage detection in concrete beams using piezoelectric patches
Markovic, N. and Nestorovic, T. and Stojic, D.
MECHANICS RESEARCH COMMUNICATIONS. Volume: 64 (2015) - 2015 • 3371
Measurement of the transmission phase of an electron in a quantum two-path interferometer
Takada, S. and Yamamoto, M. and Bäuerle, C. and Watanabe, K. and Ludwig, Ar. and Wieck, A.D. and Tarucha, S.
APPLIED PHYSICS LETTERS. Volume: 107 (2015) - 2015 • 3370
Multiphase microstructures via confined precipitation and dissolution of vessel phases: Example of austenite in martensitic steel
Belde, M. and Springer, H. and Inden, G. and Raabe, D.
ACTA MATERIALIA. Volume: 86 (2015) - 2015 • 3369
Crystal structure analyses of two TMA silicates with ordered defects: RUB-20, a layered zeolite precursor, and RUB-22, a microporous framework silicate
Marler, B. and Gies, H.
ZEITSCHRIFT FUR KRISTALLOGRAPHIE - CRYSTALLINE MATERIALS. Volume: 230 (2015) - 2015 • 3368
A highly accurate 1st- and 2nd-order differentiation scheme for hyperelastic material models based on hyper-dual numbers
Tanaka, M. and Sasagawa, T. and Omote, R. and Fujikawa, M. and Balzani, D. and Schröder, J.
COMPUTER METHODS IN APPLIED MECHANICS AND ENGINEERING. Volume: 283 (2015) - 2015 • 3367
Nanoparticle Capping Agent Dynamics and Electron Transfer: Polymer-Gated Oxidation of Silver Nanoparticles
Tanner, E.E.L. and Tschulik, K. and Tahany, R. and Jurkschat, K. and Batchelor-McAuley, C. and Compton, R.G.
JOURNAL OF PHYSICAL CHEMISTRY C. Volume: 119 (2015) - 2015 • 3366
Reaction Network of Methanol Synthesis over Cu/ZnO Nanocatalysts
Martinez-Suarez, L. and Siemer, N. and Frenzel, J. and Marx, D.
ACS CATALYSIS. Volume: 5 (2015) - 2015 • 3365
Microstructure design and mechanical properties in a near-α Ti-4Mo alloy
Tarzimoghadam, Z. and Sandlöbes, S. and Pradeep, K.G. and Raabe, D.
ACTA MATERIALIA. Volume: 97 (2015) - 2015 • 3364
Variational prediction of the mechanical behavior of shape memory alloys based on thermal experiments
Junker, P. and Jaeger, S. and Kastner, O. and Eggeler, G. and Hackl, K.
JOURNAL OF THE MECHANICS AND PHYSICS OF SOLIDS. Volume: 80 (2015) - 2015 • 3363
An Overview of Dual-Phase Steels: Advances in Microstructure-Oriented Processing and Micromechanically Guided Design
Tasan, C.C. and Diehl, M. and Yan, D. and Bechtold, M. and Roters, F. and Schemmann, L. and Zheng, C. and Peranio, N. and Ponge, D. and Koyama, M. and Tsuzaki, K. and Raabe, D.
ANNUAL REVIEW OF MATERIALS RESEARCH. Volume: 45 (2015) - 2015 • 3362
A variational growth approach to topology optimization
Junker, P. and Hackl, K.
STRUCTURAL AND MULTIDISCIPLINARY OPTIMIZATION. Volume: 52 (2015) - 2015 • 3361
The influence of a brittle Cr interlayer on the deformation behavior of thin Cu films on flexible substrates: Experiment and model
Marx, V.M. and Toth, F. and Wiesinger, A. and Berger, J. and Kirchlechner, C. and Cordill, M.J. and Fischer, F.D. and Rammerstorfer, F.G. and Dehm, G.
ACTA MATERIALIA. Volume: 89 (2015) - 2015 • 3360
Adhesion measurement of a buried Cr interlayer on polyimide
Marx, V.M. and Kirchlechner, C. and Zizak, I. and Cordill, M.J. and Dehm, G.
PHILOSOPHICAL MAGAZINE. Volume: 95 (2015) - 2015 • 3359
Nanoindentation studies of the mechanical properties of the μ phase in a creep deformed Re containing nickel-based superalloy
Rehman, H.U. and Durst, K. and Neumeier, S. and Parsa, A.B. and Kostka, A. and Eggeler, G. and Göken, M.
MATERIALS SCIENCE AND ENGINEERING A. Volume: 634 (2015) - 2015 • 3358
Extending the spectral range of CdSe/ZnSe quantum wells by strain engineering
Finke, A. and Ruth, M. and Scholz, S. and Ludwig, Ar. and Wieck, A.D. and Reuter, D. and Pawlis, A.
PHYSICAL REVIEW B - CONDENSED MATTER AND MATERIALS PHYSICS. Volume: 91 (2015) - 2015 • 3357
Correlations between microstructure and room temperature tensile behavior of a duplex TNB alloy for systematically heat treated samples
Kabir, M.R. and Bartsch, M. and Chernova, L. and Kelm, K. and Wischek, J.
MATERIALS SCIENCE AND ENGINEERING A. Volume: 635 (2015) - 2015 • 3356
On the Role of Metals in Nitrogen-Doped Carbon Electrocatalysts for Oxygen Reduction
Masa, J. and Xia, W. and Muhler, M. and Schuhmann, W.
ANGEWANDTE CHEMIE - INTERNATIONAL EDITION. Volume: 54 (2015) - 2015 • 3355
Experimental investigations of electron heating dynamics and ion energy distributions in capacitive discharges driven by customized voltage waveforms
Berger, B. and Brandt, S. and Franek, J. and Schüngel, E. and Koepke, M. and Mussenbrock, T. and Schulze, J.
JOURNAL OF APPLIED PHYSICS. Volume: 118 (2015) - 2015 • 3354
Critical assessment of visco-elastic damping models used in DEM simulations
Kačianauskas, R. and Kruggel-Emden, H. and Markauskas, D. and Zdancevičius, E.
PROCEDIA ENGINEERING. Volume: 102 (2015) - 2015 • 3353
Self-Assembled Monolayers: Star-Shaped Crystallographic Cracking of Localized Nanoporous Defects (Adv. Mater. 33/2015)
Renner, F.U. and Ankah, G.N. and Bashir, A. and Ma, D. and Biedermann, P.U. and Shrestha, B.R. and Nellessen, M. and Khorashadizadeh, A. and Losada-Pérez, P. and Duarte, M.J. and Raabe, D. and Valtiner, M.
ADVANCED MATERIALS (DEERFIELD BEACH, FLA.). Volume: 27 (2015) - 2015 • 3352
Investigating antennas as ignition aid for automotive HID lamps
Bergner, A. and Engelhardt, M. and Bienholz, S. and Ruhrmann, C. and Hoebing, T. and Groeger, S. and Mentel, J. and Awakowicz, P.
JOURNAL OF PHYSICS D: APPLIED PHYSICS. Volume: 48 (2015) - 2015 • 3351
Radio frequency microelectromechanical system-platform based on silicon-ceramic composite substrates
Fischer, M. and Gropp, S. and Nowak, J. and Capraro, B. and Sommer, R. and Hoffmann, M. and Mülle, J.
JOURNAL OF MICROELECTRONICS AND ELECTRONIC PACKAGING. Volume: 12 (2015) - 2015 • 3350
Star-Shaped Crystallographic Cracking of Localized Nanoporous Defects
Renner, F.U. and Ankah, G.N. and Bashir, A. and Ma, D. and Biedermann, P.U. and Shrestha, B.R. and Nellessen, M. and Khorashadizadeh, A. and Losada-Pérez, P. and Duarte, M.J. and Raabe, D. and Valtiner, M.
ADVANCED MATERIALS. Volume: 27 (2015) - 2015 • 3349
A numerical model of the combustion of single lithium particles with CO2
Fischer, P. and Schiemann, M. and Scherer, V. and Maas, P. and Schmid, G. and Taroata, D.
FUEL. Volume: 160 (2015) - 2015 • 3348
Effect of ruthenium on the precipitation of topologically close packed phases in Ni-based superalloys of 3rd and 4th generation
Matuszewski, K. and Rettig, R. and Matysiak, H. and Peng, Z. and Povstugar, I. and Choi, P. and Müller, J. and Raabe, D. and Spiecker, E. and Kurzydłowski, K.J. and Singer, R.F.
ACTA MATERIALIA. Volume: 95 (2015) - 2015 • 3347
Interplay of Electron and Nuclear Spin Noise in n -Type GaAs
Berski, F. and Hübner, J. and Oestreich, M. and Ludwig, Ar. and Wieck, A.D. and Glazov, M.
PHYSICAL REVIEW LETTERS. Volume: 115 (2015) - 2015 • 3346
Experimental characterization of the combustion of single lithium particles with CO2
Fischer, P. and Schiemann, M. and Scherer, V. and Maas, P. and Schmid, G. and Taroata, D.
FUEL. Volume: 153 (2015) - 2015 • 3345
Assembling Paramagnetic Ceruloplasmin at Electrode Surfaces Covered with Ferromagnetic Nanoparticles. Scanning Electrochemical Microscopy in the Presence of a Magnetic Field
Matysiak, E. and Botz, A.J.R. and Clausmeyer, J. and Wagner, B. and Schuhmann, W. and Stojek, Z. and Nowicka, A.M.
LANGMUIR. Volume: 31 (2015) - 2015 • 3344
Quantum Manipulation of Two-Electron Spin States in Isolated Double Quantum Dots
Bertrand, B. and Flentje, H. and Takada, S. and Yamamoto, M. and Tarucha, S. and Ludwig, Ar. and Wieck, A.D. and Bäuerle, C. and Meunier, T.
PHYSICAL REVIEW LETTERS. Volume: 115 (2015) - 2015 • 3343
Effects of Feedstock Decomposition and Evaporation on the Composition of Suspension Plasma-Sprayed Coatings
Mauer, G. and Schlegel, N. and Guignard, A. and Vaßen, R. and Guillon, O.
JOURNAL OF THERMAL SPRAY TECHNOLOGY. Volume: 24 (2015) - 2015 • 3342
Metal-metal terahertz quantum cascade laser with hybrid mode section
Fobbe, T. and Nong, H. and Schott, R. and Pal, S. and Markmann, S. and Hekmat, N. and Zhu, J. and Han, Y. and Li, L. and Dean, P. and Linfield, E.H. and Davies, A.G. and Wieck, A.D. and Jukam, N.
IRMMW-THZ 2015 - 40TH INTERNATIONAL CONFERENCE ON INFRARED, MILLIMETER, AND TERAHERTZ WAVES. Volume: (2015) - 2015 • 3341
Novel opportunities for thermal spray by PS-PVD
Mauer, G. and Jarligo, M.O. and Rezanka, S. and Hospach, A. and Vaßen, R.
SURFACE AND COATINGS TECHNOLOGY. Volume: 268 (2015) - 2015 • 3340
Pressure-induced antiferromagnetic transition and phase diagram in FeSe
Terashima, T. and Kikugawa, N. and Kasahara, S. and Watashige, T. and Shibauchi, T. and Matsuda, Y. and Wolf, T. and Böhmer, A.E. and Hardy, F. and Meingast, C. and Löhneysen, H.V. and Uji, S.
JOURNAL OF THE PHYSICAL SOCIETY OF JAPAN. Volume: 84 (2015) - 2015 • 3339
Non-collinear magnetism with analytic Bond-Order Potentials
Ford, M.E. and Pettifor, D.G. and Drautz, R.
JOURNAL OF PHYSICS CONDENSED MATTER. Volume: 27 (2015) - 2015 • 3338
Graphene-intercalated Fe2O3/TiO2 heterojunctions for efficient photoelectrolysis of water
Kaouk, A. and Ruoko, T.-P. and Gönüllü, Y. and Kaunisto, K. and Mettenbörger, A. and Gurevich, E. and Lemmetyinen, H. and Ostendorf, A. and Mathur, S.
RSC ADVANCES. Volume: 5 (2015) - 2015 • 3337
Isothermal aging of a γ'-strengthened Co-Al-W alloy coated with vacuum plasma-sprayed MCrAlY bond coats
Terberger, P.J. and Sebold, D. and Webler, R. and Ziener, M. and Neumeier, S. and Klein, L. and Virtanen, S. and Göken, M. and Vaßen, R.
SURFACE AND COATINGS TECHNOLOGY. Volume: 276 (2015) - 2015 • 3336
Mechanism of protection of catalysts supported in redox hydrogel films
Fourmond, V. and Stapf, S. and Li, H. and Buesen, D. and Birrell, J. and Rüdiger, O. and Lubitz, W. and Schuhmann, W. and Plumeré, N. and Léger, C.
JOURNAL OF THE AMERICAN CHEMICAL SOCIETY. Volume: 137 (2015) - 2015 • 3335
Importance of dislocation pile-ups on the mechanical properties and the Bauschinger effect in microcantilevers
Kapp, M.W. and Kirchlechner, C. and Pippan, R. and Dehm, G.
JOURNAL OF MATERIALS RESEARCH. Volume: 30 (2015) - 2015 • 3334
A new zeolite formed from interlayer expansion of the precursor COK-5
Bian, C. and Wu, Q. and Zhang, J. and Chen, F. and Pan, S. and Wang, L. and Meng, X. and Müller, U. and Feyen, M. and Yilmaz, B. and Gies, H. and Zhang, W. and Bao, X. and De Vos, D. and Yokoi, T. and Tatsumi, T. and Xiao, F.-S.
MICROPOROUS AND MESOPOROUS MATERIALS. Volume: 214 (2015) - 2015 • 3333
Mode-selected heat flow through a one-dimensional waveguide network
Riha, C. and Miechowski, P. and Buchholz, S.S. and Chiatti, O. and Wieck, A.D. and Reuter, D. and Fischer, S.F.
APPLIED PHYSICS LETTERS. Volume: 106 (2015) - 2015 • 3332
Metal-organic CVD of Y2O3 Thin Films using Yttrium tris-amidinates
Karle, S. and Dang, V.-S. and Prenzel, M. and Rogalla, D. and Becker, H.-W. and Devi, A.
CHEMICAL VAPOR DEPOSITION. Volume: 21 (2015) - 2015 • 3331
Using cavity microelectrodes for electrochemical noise studies of oxygen-evolving catalysts
Rincón, R.A. and Battistel, A. and Ventosa, E. and Chen, X. and Nebel, M. and Schuhmann, W.
CHEMSUSCHEM. Volume: 8 (2015) - 2015 • 3330
Hybrid surrogate modelling for mechanised tunnelling simulations with uncertain data
Freitag, S. and Cao, B.T. and Ninić, J. and Meschke, G.
INTERNATIONAL JOURNAL OF RELIABILITY AND SAFETY. Volume: 9 (2015) - 2015 • 3329
Nanostructure of wet-chemically prepared, polymer-stabilized silver-gold nanoalloys (6 nm) over the entire composition range
Ristig, S. and Prymak, O. and Loza, K. and Gocyla, M. and Meyer-Zaika, W. and Heggen, M. and Raabe, D. and Epple, M.
JOURNAL OF MATERIALS CHEMISTRY B. Volume: 3 (2015) - 2015 • 3328
On the effect of alloy composition on martensite start temperatures and latent heats in Ni-Ti-based shape memory alloys
Frenzel, J. and Wieczorek, A. and Opahle, I. and Maaß, B. and Drautz, R. and Eggeler, G.
ACTA MATERIALIA. Volume: 90 (2015) - 2015 • 3327
A passive microsystem for detecting multiple acceleration events beyond a threshold
Mehner, H. and Weise, C. and Schwebke, S. and Hampl, S. and Hoffmann, M.
MICROELECTRONIC ENGINEERING. Volume: 145 (2015) - 2015 • 3326
Synergy of atom-probe structural data and quantum-mechanical calculations in a theory-guided design of extreme-stiffness superlattices containing metastable phases
Friák, M. and Tytko, D. and Holec, D. and Choi, P.-P. and Eisenlohr, P. and Raabe, D. and Neugebauer, J.
NEW JOURNAL OF PHYSICS. Volume: 17 (2015) - 2015 • 3325
Unraveling the interactions between cold atmospheric plasma and skin-components with vibrational microspectroscopy
Kartaschew, K. and Mischo, M. and Baldus, S. and Bründermann, E. and Awakowicz, P. and Havenith, M.
BIOINTERPHASES. Volume: 10 (2015) - 2015 • 3324
Influence of Post-Implantation Annealing Parameters on the Focused Ion Beam Directed Nucleation of InAs Quantum Dots
Mehta, M. and Reuter, D. and Kamruddin, M. and Tyagi, A.K. and Wieck, A.D.
NANO. Volume: 10 (2015) - 2015 • 3323
Crystalline TiO2: A Generic and Effective Electron-Conducting Protection Layer for Photoanodes and -cathodes
Mei, B. and Pedersen, T. and Malacrida, P. and Bae, D. and Frydendal, R. and Hansen, O. and Vesborg, P.C.K. and Seger, B. and Chorkendorff, I.
JOURNAL OF PHYSICAL CHEMISTRY C. Volume: 119 (2015) - 2015 • 3322
Mechanocatalytic depolymerization of lignocellulose performed on hectogram and kilogram scales
Kaufman Rechulski, M.D. and Käldström, M. and Richter, U. and Schüth, F. and Rinaldi, R.
INDUSTRIAL AND ENGINEERING CHEMISTRY RESEARCH. Volume: 54 (2015) - 2015 • 3321
Pairing symmetry of the one-band Hubbard model in the paramagnetic weak-coupling limit: A numerical RPA study
Rømer, A.T. and Kreisel, A. and Eremin, I. and Malakhov, M.A. and Maier, T.A. and Hirschfeld, P.J. and Andersen, B.M.
PHYSICAL REVIEW B - CONDENSED MATTER AND MATERIALS PHYSICS. Volume: 92 (2015) - 2015 • 3320
Boron-alloyed Fe-Cr-C-B tool steels - Thermodynamic calculations and experimental validation
Röttger, A. and Lentz, J. and Theisen, W.
MATERIALS AND DESIGN. Volume: 88 (2015) - 2015 • 3319
Nanocatalysis: Size- and shape-dependent chemisorption and catalytic reactivity
Roldan Cuenya, B. and Behafarid, F.
SURFACE SCIENCE REPORTS. Volume: 70 (2015) - 2015 • 3318
A Generalized Finite Element Method for hydro-mechanically coupled analysis of hydraulic fracturing problems using space-time variant enrichment functions
Meschke, G. and Leonhart, D.
COMPUTER METHODS IN APPLIED MECHANICS AND ENGINEERING. Volume: 290 (2015) - 2015 • 3317
Water window ptychographic imaging with characterized coherent X-rays
Rose, M. and Skopintsev, P. and Dzhigaev, D. and Gorobtsov, O. and Senkbeil, T. and Von Gundlach, A. and Gorniak, T. and Shabalin, A. and Viefhaus, J. and Rosenhahn, A. and Vartanyants, I.
JOURNAL OF SYNCHROTRON RADIATION. Volume: 22 (2015) - 2015 • 3316
Microscopic origin of resistance drift in the amorphous state of the phase-change compound GeTe
Gabardi, S. and Caravati, S. and Sosso, G.C. and Behler, J. and Bernasconi, M.
PHYSICAL REVIEW B - CONDENSED MATTER AND MATERIALS PHYSICS. Volume: 92 (2015) - 2015 • 3315
Constraining the conditions of Barrovian metamorphism in Sikkim, India: P-T-t paths of garnet crystallization in the Lesser Himalayan Belt
Gaidies, F. and Petley-Ragan, A. and Chakraborty, S. and Dasgupta, S. and Jones, P.
JOURNAL OF METAMORPHIC GEOLOGY. Volume: 33 (2015) - 2015 • 3314
High-throughput screening of thin-film semiconductor material libraries II: Characterization of Fe-W-O libraries
Meyer, R. and Sliozberg, K. and Khare, C. and Schuhmann, W. and Ludwig, Al.
CHEMSUSCHEM. Volume: 8 (2015) - 2015 • 3313
Doping asymmetry of superconductivity coexisting with antiferromagnetism in spin fluctuation theory
Rowe, W. and Eremin, I. and Rømer, A.T. and Andersen, B.M. and Hirschfeld, P.J.
NEW JOURNAL OF PHYSICS. Volume: 17 (2015) - 2015 • 3312
Direct photonic coupling of a semiconductor quantum dot and a trapped ion
Meyer, H.M. and Stockill, R. and Steiner, M. and Le Gall, C. and Matthiesen, C. and Clarke, E. and Ludwig, Ar. and Reichel, J. and Atatüre, M. and Köhl, M.
PHYSICAL REVIEW LETTERS. Volume: 114 (2015) - 2015 • 3311
New edge magnetoplasmon interference like photovoltage oscillations and their amplitude enhancement in the presence of an antidot lattice
Bisotto, I. and Portal, J. C. and Brown, D. and Wieck, A. D.
AIP ADVANCES. Volume: 5 (2015) - 2015 • 3310
Scalability and feasibility of photoelectrochemical H2 evolution: the ultimate limit of Pt nanoparticle as an HER catalyst
Kemppainen, E. and Bodin, A. and Sebok, B. and Pedersen, T. and Seger, B. and Mei, B. and Bae, D. and Vesborg, P.C.K. and Halme, J. and Hansen, O. and Lund, P.D. and Chorkendorff, I.
ENERGY AND ENVIRONMENTAL SCIENCE. Volume: 8 (2015) - 2015 • 3309
Cavitation-resistant NiTi coatings produced by low-pressure plasma spraying (LPPS)
Bitzer, M. and Rauhut, N. and Mauer, G. and Bram, M. and Vaßen, R. and Buchkremer, H.-P. and Stöver, D. and Pohl, M.
WEAR. Volume: 328-329 (2015) - 2015 • 3308
Global modeling of hiPIMS systems: Transition from homogeneous to self organized discharges
Gallian, S. and Trieschmann, J. and Mussenbrock, T. and Hitchon, W.N.G. and Brinkmann, R.P.
ICOPS/BEAMS 2014 - 41ST IEEE INTERNATIONAL CONFERENCE ON PLASMA SCIENCE AND THE 20TH INTERNATIONAL CONFERENCE ON HIGH-POWER PARTICLE BEAMS. Volume: (2015) - 2015 • 3307
The gas phase emitter effect of lanthanum within ceramic metal halide lamps and its dependence on the la vapor pressure and operating frequency
Ruhrmann, C. and Hoebing, T. and Bergner, A. and Groeger, S. and Denissen, C. and Suijker, J. and Awakowicz, P. and Mentel, J.
JOURNAL OF APPLIED PHYSICS. Volume: 118 (2015) - 2015 • 3306
Analytic model of the energy distribution function for highly energetic electrons in magnetron plasmas
Gallian, S. and Trieschmann, J. and Mussenbrock, T. and Brinkmann, R.P. and Hitchon, W.N.G.
JOURNAL OF APPLIED PHYSICS. Volume: 117 (2015) - 2015 • 3305
General Method for the Synthesis of Hollow Mesoporous Carbon Spheres with Tunable Textural Properties
Mezzavilla, S. and Baldizzone, C. and Mayrhofer, K.J.J. and Schüth, F.
ACS APPLIED MATERIALS AND INTERFACES. Volume: 7 (2015) - 2015 • 3304
Optical properties of strain-compensated CdSe/ZnSe/(Zn,Mg)Se quantum well microdisks
Ruth, M. and Finke, A. and Schmidt, G. and Reuter, D. and Scholz, S. and Ludwig, Ar. and Wieck, A.D. and Pawlis, A.
OPTICS EXPRESS. Volume: 23 (2015) - 2015 • 3303
International high manganese steel conference
Bleck, W. and Raabe, D. and Dong, H.
STEEL RESEARCH INTERNATIONAL. Volume: 86 (2015) - 2015 • 3302
Modeling precursor diffusion and reaction of atomic layer deposition in porous structures
Keuter, T. and Menzler, N.H. and Mauer, G. and Vondahlen, F. and Vaßen, R. and Buchkremer, H.P.
JOURNAL OF VACUUM SCIENCE AND TECHNOLOGY A: VACUUM, SURFACES AND FILMS. Volume: 33 (2015) - 2015 • 3301
Redox-stable high-performance thin-film solid oxide fuel cell
Keuter, T. and Roehrens, D. and Menzler, N.H. and Vaßen, R.
ECS TRANSACTIONS. Volume: 68 (2015) - 2015 • 3300
Computational Modeling of Concrete Degradation Due to Alkali Silica Reaction
Timothy, J.J. and Nguyen, M.N. and Meschke, G.
CONCREEP 2015: MECHANICS AND PHYSICS OF CREEP, SHRINKAGE, AND DURABILITY OF CONCRETE AND CONCRETE STRUCTURES - PROCEEDINGS OF THE 10TH INTERNATIONAL CONFERENCE ON MECHANICS AND PHYSICS OF CREEP, SHRINKAGE, AND DURABILITY OF CONCRETE AND CONCRETE STRUCTURES. Volume: (2015) - 2015 • 3299
In vitro comparison of the sagittal split osteotomy with and without inferior border osteotomy
Böckmann, R. and Schön, P. and Neuking, K. and Meyns, J. and Kessler, P. and Eggeler, G.
JOURNAL OF ORAL AND MAXILLOFACIAL SURGERY. Volume: 73 (2015) - 2015 • 3298
Non-covalent interactions in water electrolysis: Influence on the activity of Pt(111) and iridium oxide catalysts in acidic media
Ganassin, A. and Colic, V. and Tymoczko, J. and Bandarenka, A.S. and Schuhmann, W.
PHYSICAL CHEMISTRY CHEMICAL PHYSICS. Volume: 17 (2015) - 2015 • 3297
Fabrication of Gold/Titania Photocatalyst for CO2 Reduction Based on Pyrolytic Conversion of the Metal-Organic Framework NH2-MIL-125(Ti) Loaded with Gold Nanoparticles
Khaletskaya, K. and Pougin, A. and Medishetty, R. and Rösler, C. and Wiktor, C. and Strunk, J. and Fischer, R.A.
CHEMISTRY OF MATERIALS. Volume: 27 (2015) - 2015 • 3296
Microstructural evolution in a Ti-Ta hightemperature shape memory alloy during creep
Rynko, R. and Marquardt, A. and Paulsen, A. and Frenzel, J. and Somsen, C. and Eggeler, G.
INTERNATIONAL JOURNAL OF MATERIALS RESEARCH. Volume: 106 (2015) - 2015 • 3295
Formulation of nonlocal damage models based on spectral methods for application to complex microstructures
Boeff, M. and Gutknecht, F. and Engels, P.S. and Ma, A. and Hartmaier, A.
ENGINEERING FRACTURE MECHANICS. Volume: 147 (2015) - 2015 • 3294
Identification of bulk oxide defects in an electrochemical environment
Todorova, M. and Neugebauer, J.
FARADAY DISCUSSIONS. Volume: 180 (2015) - 2015 • 3293
Superconductivity-induced re-entrance of the orthorhombic distortion in Ba1-xKxFe2As2
Böhmer, A.E. and Hardy, F. and Wang, L. and Wolf, T. and Schweiss, P. and Meingast, C.
NATURE COMMUNICATIONS. Volume: 6 (2015) - 2015 • 3292
Primary combination of phase-field and discrete dislocation dynamics methods for investigating athermal plastic deformation in various realistic Ni-base single crystal superalloy microstructures
Gao, S. and Kumar Rajendran, M. and Fivel, M. and Ma, A. and Shchyglo, O. and Hartmaier, A. and Steinbach, I.
MODELLING AND SIMULATION IN MATERIALS SCIENCE AND ENGINEERING. Volume: 23 (2015) - 2015 • 3291
Spectroscopic and Microscopic Investigations of Degradation Processes in Polymer Surface-Near Regions during the Deposition of SiOx Films
Mitschker, F. and Dietrich, J. and Ozkaya, B., Dr. and De los Arcos, T., Dr. and Giner, I., Dr. and Awakowicz, P., Prof. and Grundmeier, G., Prof.
PLASMA PROCESSES AND POLYMERS. Volume: 12 (2015) - 2015 • 3290
Connecting semiconductor defect chemistry with electrochemistry: Impact of the electrolyte on the formation and concentration of point defects in ZnO
Todorova, M. and Neugebauer, J.
SURFACE SCIENCE. Volume: 631 (2015) - 2015 • 3289
Origin of the tetragonal-to-orthorhombic phase transition in FeSe: A combined thermodynamic and NMR study of nematicity
Böhmer, A.E. and Arai, T. and Hardy, F. and Hattori, T. and Iye, T. and Wolf, T. and Löhneysen, H.V. and Ishida, K. and Meingast, C.
PHYSICAL REVIEW LETTERS. Volume: 114 (2015) - 2015 • 3288
Influence of misfit stresses on dislocation glide in single crystal superalloys: A three-dimensional discrete dislocation dynamics study
Gao, S. and Fivel, M. and Ma, A. and Hartmaier, A.
JOURNAL OF THE MECHANICS AND PHYSICS OF SOLIDS. Volume: 76 (2015) - 2015 • 3287
Carbon partitioning during quenching and partitioning heat treatment accompanied by carbide precipitation
Toji, Y. and Miyamoto, G. and Raabe, D.
ACTA MATERIALIA. Volume: 86 (2015) - 2015 • 3286
Array sensor: Plasmonic improved optical resonance methods and instrument for biomedical diagnostics
Saetchnikov, V.A. and Tcherniavskaia, E.A. and Saetchnikov, A.V. and Schweiger, G. and Ostendorf, A.
PROGRESS IN BIOMEDICAL OPTICS AND IMAGING - PROCEEDINGS OF SPIE. Volume: 9540 (2015) - 2015 • 3285
From wetting to melting along grain boundaries using phase field and sharp interface methods
Sai Pavan Kumar Bhogireddy, V. and Hüter, C. and Neugebauer, J. and Shchyglo, O. and Steinbach, I. and Spatschek, R.
COMPUTATIONAL MATERIALS SCIENCE. Volume: 108 (2015) - 2015 • 3284
Characteristics of flexibility in metal-organic framework solid solutions of composition [Zn2(BME-bdc)x(DB-bdc)2-xdabco]n: In situ powder X-ray diffraction, in situ NMR spectroscopy, and molecular dynamics simulations
Bon, V. and Pallmann, J. and Eisbein, E. and Hoffmann, H.C. and Senkovska, I. and Schwedler, I. and Schneemann, A. and Henke, S. and Wallacher, D. and Fischer, R.A. and Seifert, G. and Brunner, E. and Kaskel, S.
MICROPOROUS AND MESOPOROUS MATERIALS. Volume: 216 (2015) - 2015 • 3283
Reduced tight-binding models for elemental Si and N, and ordered binary Si-N systems
Gehrmann, J. and Pettifor, D.G. and Kolmogorov, A.N. and Reese, M. and Mrovec, M. and Elsässer, C. and Drautz, R.
PHYSICAL REVIEW B - CONDENSED MATTER AND MATERIALS PHYSICS. Volume: 91 (2015) - 2015 • 3282
Transport of sputtered particles in capacitive sputter sources
Trieschmann, J. and Mussenbrock, T.
JOURNAL OF APPLIED PHYSICS. Volume: 118 (2015) - 2015 • 3281
Adsorption of a Switchable Industrial Dye on Au(111) and Ag(111)
Boom, K. and Müller, M. and Stein, F. and Ernst, S. and Morgenstern, K.
JOURNAL OF PHYSICAL CHEMISTRY C. Volume: 119 (2015) - 2015 • 3280
Pressure-induced electronic phase separation of magnetism and superconductivity in CrAs
Khasanov, R. and Guguchia, Z. and Eremin, I. and Luetkens, H. and Amato, A. and Biswas, P.K. and Rüegg, C. and Susner, M.A. and Sefat, A.S. and Zhigadlo, N.D. and Morenzoni, E.
SCIENTIFIC REPORTS. Volume: 5 (2015) - 2015 • 3279
Structuring zeolite bodies for enhanced heat-transfer properties
Borchardt, L. and Michels, N.-L. and Nowak, T. and Mitchell, S. and Pérez-Ramírez, J.
MICROPOROUS AND MESOPOROUS MATERIALS. Volume: 208 (2015) - 2015 • 3278
The fluorite/water interfaces: Structure and spectroscopy from first principles simulations
Khatib, R. and Sulpizi, M.
HIGH PERFORMANCE COMPUTING IN SCIENCE AND ENGINEERING '14: TRANSACTIONS OF THE HIGH PERFORMANCE COMPUTING CENTER, STUTTGART (HLRS) 2014. Volume: (2015) - 2015 • 3277
Simulations of the Eutectic Transformations in the Platinum–Carbon System
Monas, A. and Bloembergen, P. and Dong, W. and Shchyglo, O. and Steinbach, I.
INTERNATIONAL JOURNAL OF THERMOPHYSICS. Volume: 36 (2015) - 2015 • 3276
Butadiene from acetylene-ethylene cross-metathesis
Trotuş, I.-T. and Zimmermann, T. and Duyckaerts, N. and Geboers, J. and Schüth, F.
CHEMICAL COMMUNICATIONS. Volume: 51 (2015) - 2015 • 3275
Metal-support interactions in surface-modified Cu-Co catalysts applied in higher alcohol synthesis
Bordoloi, A. and Anton, J. and Ruland, H. and Muhler, M. and Kaluza, S.
CATALYSIS SCIENCE AND TECHNOLOGY. Volume: 5 (2015) - 2015 • 3274
Divorced Eutectic Solidification of Mg-Al Alloys
Monas, A. and Shchyglo, O. and Kim, S.-J. and Yim, C.D. and Höche, D. and Steinbach, I.
JOM. Volume: 67 (2015) - 2015 • 3273
Combinatorial synthesis and high-throughput characterization of the thin film materials system Co-Mn-Ge: Composition, structure, and magnetic properties
Salomon, S. and Hamann, S. and Decker, P. and Savan, A. and Meshi, L. and Ludwig, Al.
PHYSICA STATUS SOLIDI (A) APPLICATIONS AND MATERIALS SCIENCE. Volume: 212 (2015) - 2015 • 3272
Large deformation framework for phase-field simulations at the mesoscale
Borukhovich, E. and Engels, P.S. and Mosler, J. and Shchyglo, O. and Steinbach, I.
COMPUTATIONAL MATERIALS SCIENCE. Volume: 108 (2015) - 2015 • 3271
Diffusion limited aggregation in low temperature growth of sodium chloride
Gerß, B. and Osterloh, N. and Heidorn, S.-C. and Morgenstern, K.
CRYSTAL GROWTH AND DESIGN. Volume: 15 (2015) - 2015 • 3270
Dual-scale phase-field simulation of Mg-Al alloy solidification
Monas, A. and Shchyglo, O. and Höche, D. and Tegeler, M. and Steinbach, I.
IOP CONFERENCE SERIES: MATERIALS SCIENCE AND ENGINEERING. Volume: 84 (2015) - 2015 • 3269
Core-Shell Nanoparticles: Characterizing Multifunctional Materials beyond Imaging - Distinguishing and Quantifying Perfect and Broken Shells
Tschulik, K. and Ngamchuea, K. and Ziegler, C. and Beier, M.G. and Damm, C. and Eychmueller, A. and Compton, R.G.
ADVANCED FUNCTIONAL MATERIALS. Volume: 25 (2015) - 2015 • 3268
Non-Invasive Probing of Nanoparticle Electrostatics
Tschulik, K. and Cheng, W. and Batchelor-Mcauley, C. and Murphy, S. and Omanović, D. and Compton, R.G.
CHEMELECTROCHEM. Volume: 2 (2015) - 2015 • 3267
Onset potential determination at gas-evolving catalysts by means of constant-distance mode positioning of nanoelectrodes
Botz, A.J.R. and Nebel, M. and Rincón, R.A. and Ventosa, E. and Schuhmann, W.
ELECTROCHIMICA ACTA. Volume: 179 (2015) - 2015 • 3266
Elementary surface processes during reactive magnetron sputtering of chromium
Monje, S. and Corbella, C. and von Keudell, A.
JOURNAL OF APPLIED PHYSICS. Volume: 118 (2015) - 2015 • 3265
Ammonia decomposition over iron phthalocyanine-based materials
Tüysüz, H. and Schüth, F. and Zhi, L. and Müllen, K. and Comotti, M.
CHEMCATCHEM. Volume: 7 (2015) - 2015 • 3264
Propanoate grafting on (H,OH)-Si(0 0 1)-2×1
Bournel, F. and Gallet, J.-J. and Köhler, U. and Ellakhmissi, B.B. and Kubsky, S. and Carniato, S. and Rochet, F.
JOURNAL OF PHYSICS CONDENSED MATTER. Volume: 27 (2015) - 2015 • 3263
3D structural and atomic-scale analysis of lath martensite: Effect of the transformation sequence
Morsdorf, L. and Tasan, C.C. and Ponge, D. and Raabe, D.
ACTA MATERIALIA. Volume: 95 (2015) - 2015 • 3262
Evaluation of the Electrochemical Stability of Model Cu-Pt(111) Near-Surface Alloy Catalysts
Tymoczko, J. and Calle-Vallejo, F. and Čolić, V. and Schuhmann, W. and Bandarenka, A.S.
ELECTROCHIMICA ACTA. Volume: 179 (2015) - 2015 • 3261
MOCVD of TiO2 thin films from a modified titanium alkoxide precursor
Kim, S.J. and Dang, V.-S. and Xu, K. and Barreca, D. and Maccato, C. and Carraro, G. and Bhakta, R.K. and Winter, M. and Becker, H.-W. and Rogalla, D. and Sada, C. and Fischer, R.A. and Devi, A.
PHYSICA STATUS SOLIDI (A) APPLICATIONS AND MATERIALS SCIENCE. Volume: 212 (2015) - 2015 • 3260
Microstructure, Shape Memory Effect and Functional Stability of Ti67 Ta33 Thin Films
Motemani, Y. and Kadletz, P.M. and Maier, B. and Rynko, R. and Somsen, C. and Paulsen, A. and Frenzel, J. and Schmahl, W.W. and Eggeler, G. and Ludwig, Al.
ADVANCED ENGINEERING MATERIALS. Volume: 17 (2015) - 2015 • 3259
Influence of the alkali metal cations on the activity of Pt(1 1 1) towards model electrocatalytic reactions in acidic sulfuric media
Tymoczko, J. and Colic, V. and Ganassin, A. and Schuhmann, W. and Bandarenka, A.S.
CATALYSIS TODAY. Volume: 244 (2015) - 2015 • 3258
Increasing variety of our building materials: How can complex interactions in new concretes be controlled?
Breitenbücher, R.
BETON- UND STAHLBETONBAU. Volume: 110 (2015) - 2015 • 3257
On the room temperature deformation mechanisms of a Mg-Y-Zn alloy with long-period-stacking-ordered structures
Kim, J.-K. and Sandlöbes, S. and Raabe, D.
ACTA MATERIALIA. Volume: 82 (2015) - 2015 • 3256
Detection of 2D phase transitions at the electrode/electrolyte interface using electrochemical impedance spectroscopy
Tymoczko, J. and Colic, V. and Bandarenka, A.S. and Schuhmann, W.
SURFACE SCIENCE. Volume: 631 (2015) - 2015 • 3255
The role of grain boundaries in the initial oxidation behavior of austenitic stainless steel containing alloyed Cu at 700°C for advanced thermal power plant applications
Kim, J.-H. and Kim, B.K. and Kim, D.-I. and Choi, P.-P. and Raabe, D. and Yi, K.-W.
CORROSION SCIENCE. Volume: 96 (2015) - 2015 • 3254
Thermal dissolution mechanisms of AlN/CrN hard coating superlattices studied by atom probe tomography and transmission electron microscopy
Tytko, D. and Choi, P.-P. and Raabe, D.
ACTA MATERIALIA. Volume: 85 (2015) - 2015 • 3253
Nanotribology in austenite: Plastic plowing and crack formation
Brinckmann, S. and Dehm, G.
WEAR. Volume: 338-339 (2015) - 2015 • 3252
Spin dynamics near a putative antiferromagnetic quantum critical point in Cu-substituted BaFe2As2 and its relation to high-temperature superconductivity
Kim, M.G. and Wang, M. and Tucker, G.S. and Valdivia, P.N. and Abernathy, D.L. and Chi, S. and Christianson, A.D. and Aczel, A.A. and Hong, T. and Heitmann, T.W. and Ran, S. and Canfield, P.C. and Bourret-Courchesne, E.D. and Kreyssig, A. and Lee, D.H. and Goldman, A.I. and McQueeney, R.J. and Birgeneau, R.J.
PHYSICAL REVIEW B - CONDENSED MATTER AND MATERIALS PHYSICS. Volume: 92 (2015) - 2015 • 3251
Itinerant ferromagnetism in the as 4p conduction band of Ba0.6 K0.4Mn2As2 identified by X-Ray magnetic circular dichroism
Ueland, B.G. and Pandey, A. and Lee, Y. and Sapkota, A. and Choi, Y. and Haskel, D. and Rosenberg, R.A. and Lang, J.C. and Harmon, B.N. and Johnston, D.C. and Kreyssig, A. and Goldman, A.I.
PHYSICAL REVIEW LETTERS. Volume: 114 (2015) - 2015 • 3250
Nanotribology in austenite: Normal force dependence
Brinckmann, S. and Fink, C.A.C. and Dehm, G.
WEAR. Volume: 338-339 (2015) - 2015 • 3249
High-resolution x-ray diffraction study of the heavy-fermion compound YbBiPt
Ueland, B.G. and Saunders, S.M. and Bud'Ko, S.L. and Schmiedeshoff, G.M. and Canfield, P.C. and Kreyssig, A. and Goldman, A.I.
PHYSICAL REVIEW B - CONDENSED MATTER AND MATERIALS PHYSICS. Volume: 92 (2015) - 2015 • 3248
Physics of the Advanced Plasma Source: A review of recent experimental and modeling approaches
Brinkmann, R.P. and Harhausen, J. and Schröder, B. and Lapke, M. and Storch, R. and Styrnoll, T. and Awakowicz, P. and Foest, R. and Hannemann, M. and Loffhagen, D. and Ohl, A.
PLASMA PHYSICS AND CONTROLLED FUSION. Volume: 58 (2015) - 2015 • 3247
The crystallographic template effect assisting the formation of stable α-Al2O3 during low temperature oxidation of Fe-Al alloys
Brito, P. and Pinto, H. and Kostka, A.
CORROSION SCIENCE. Volume: (2015) - 2015 • 3246
On the identification of superdislocations in the γ′-phase of single-crystal Ni-base superalloys - An application of the LACBED method to complex microstructures
Müller, J. and Eggeler, G. and Spiecker, E.
ACTA MATERIALIA. Volume: 87 (2015) - 2015 • 3245
Strain dependent electron spin dynamics in bulk cubic GaN
Schaefer, A. and Buß, J.H. and Schupp, T. and Zado, A. and As, D.J. and Hägele, D. and Rudolph, J.
JOURNAL OF APPLIED PHYSICS. Volume: 117 (2015) - 2015 • 3244
Visibility of two-dimensional layered materials on various substrates
Müller, M.R. and Gumprich, A. and Ecik, E. and Kallis, K.T. and Winkler, F. and Kardynal, B. and Petrov, I. and Kunze, U. and Knoch, J.
JOURNAL OF APPLIED PHYSICS. Volume: 118 (2015) - 2015 • 3243
Understanding anharmonicity in fcc materials: From its origin to ab initio strategies beyond the quasiharmonic approximation
Glensk, A. and Grabowski, B. and Hickel, T. and Neugebauer, J.
PHYSICAL REVIEW LETTERS. Volume: 114 (2015) - 2015 • 3242
Processing, Microstructure and Mechanical Properties of the CrMnFeCoNi High-Entropy Alloy
Gludovatz, B. and George, E.P. and Ritchie, R.O.
JOM. Volume: 67 (2015) - 2015 • 3241
Effect of heat treatment on phase structure and thermal conductivity of a copper-infiltrated steel
Klein, S. and Weber, S. and Theisen, W.
JOURNAL OF MATERIALS SCIENCE. Volume: 50 (2015) - 2015 • 3240
How Atomic Steps Modify Diffusion and Inter-adsorbate Forces: Empirical Evidence from Hopping Dynamics in Na/Cu(115)
Godsi, O. and Corem, G. and Kravchuk, T. and Bertram, C. and Morgenstern, K. and Hedgeland, H. and Jardine, A.P. and Allison, W. and Ellis, J. and Alexandrowicz, G.
JOURNAL OF PHYSICAL CHEMISTRY LETTERS. Volume: 6 (2015) - 2015 • 3239
A mechanical model for dissolution-precipitation creep based on the minimum principle of the dissipation potential
Klinge, S. and Hackl, K. and Renner, J.
PROCEEDINGS OF THE ROYAL SOCIETY A: MATHEMATICAL, PHYSICAL AND ENGINEERING SCIENCES. Volume: 471 (2015) - 2015 • 3238
Ab Initio Liquid Water Dynamics in Aqueous TMAO Solution
Usui, K. and Hunger, J. and Sulpizi, M. and Ohto, T. and Bonn, M. and Nagata, Y.
JOURNAL OF PHYSICAL CHEMISTRY B. Volume: 119 (2015) - 2015 • 3237
On the complexity reduction of laser fault injection campaigns using OBIC measurements
Schellenberg, F. and Finkeldey, M. and Richter, B. and Schapers, M. and Gerhardt, N. and Hofmann, M. and Paar, C.
PROCEEDINGS - 2015 WORKSHOP ON FAULT DIAGNOSIS AND TOLERANCE IN CRYPTOGRAPHY, FDTC 2015. Volume: (2015) - 2015 • 3236
Mid-infrared spectroscopic characterisation of an ultra-broadband tunable EC-QCL system intended for biomedical applications
Vahlsing, T. and Moser, H. and Grafen, M. and Nalpantidis, K. and Brandstetter, M. and Heise, H.M. and Lendl, B. and Leonhardt, S. and Ihrig, D. and Ostendorf, A.
PROGRESS IN BIOMEDICAL OPTICS AND IMAGING - PROCEEDINGS OF SPIE. Volume: 9537 (2015) - 2015 • 3235
Gamma-channel stabilization mechanism in Ni-base superalloys
Goerler, J.V. and Brinckmann, S. and Shchyglo, O. and Steinbach, I.
PHILOSOPHICAL MAGAZINE LETTERS. Volume: 95 (2015) - 2015 • 3234
Alloying effects on microstructure formation of dual phase steels
Schemmann, L. and Zaefferer, S. and Raabe, D. and Friedel, F. and Mattissen, D.
ACTA MATERIALIA. Volume: 95 (2015) - 2015 • 3233
Design and characterization of a resonant triaxial microprobe
Goj, B. and Dressler, L. and Hoffmann, M.
JOURNAL OF MICROMECHANICS AND MICROENGINEERING. Volume: 25 (2015) - 2015 • 3232
Synchrotron X-Ray Diffraction Measurements Mapping Internal Strains of Thermal Barrier Coatings During Thermal Gradient Mechanical Fatigue Loading
Knipe, K. and Manero, A. C. and Sofronsky, S. and Okasinski, J. and Almer, J. and Wischek, J. and Meid, C. and Karlsson, A. and Bartsch, M. and Raghavan, S.
JOURNAL OF ENGINEERING FOR GAS TURBINES AND POWER-TRANSACTIONS OF THE ASME. Volume: 137 (2015) - 2015 • 3231
Design of 3D statistically similar Representative Volume Elements based on Minkowski functionals
Scheunemann, L. and Balzani, D. and Brands, D. and Schröder, J.
MECHANICS OF MATERIALS. Volume: 90 (2015) - 2015 • 3230
Evolution of the Laves Phase in Ferritic Heat-Resistant Steels During Long-term Annealing and its Influence on the High-Temperature Strength
Nabiran, N. and Klein, S. and Weber, S. and Theisen, W.
METALLURGICAL AND MATERIALS TRANSACTIONS A: PHYSICAL METALLURGY AND MATERIALS SCIENCE. Volume: 46 (2015) - 2015 • 3229
Construction of statistically similar RVEs
Scheunemann, L. and Balzani, D. and Brands, D. and Schröder, J.
LECTURE NOTES IN APPLIED AND COMPUTATIONAL MECHANICS. Volume: 78 (2015) - 2015 • 3228
QuickFF: A program for a quick and easy derivation of force fields for metal-organic frameworks from ab initio input
Vanduyfhuys, L. and Vandenbrande, S. and Verstraelen, T. and Schmid, R. and Waroquier, M. and Van Speybroeck, V.
JOURNAL OF COMPUTATIONAL CHEMISTRY. Volume: 36 (2015) - 2015 • 3227
Scale Formation of Alloy 602 CA During Isothermal Oxidation at 800-1100 A degrees C in Different Types of Water Vapor Containing Atmospheres
Schiek, M. and Niewolak, L. and Nowak, W. and Meier, G. H. and Vassen, R. and Quadakkers, W. J.
OXIDATION OF METALS. Volume: 84 (2015) - 2015 • 3226
Resistivity and magnetoresistance of FeSe single crystals under helium-gas pressure
Knöner, S. and Zielke, D. and Köhler, S. and Wolf, B. and Wolf, Th. and Wang, L. and Böhmer, A. and Meingast, C. and Lang, M.
PHYSICAL REVIEW B - CONDENSED MATTER AND MATERIALS PHYSICS. Volume: 91 (2015) - 2015 • 3225
Drying of iron chloride solutions: Laser heating of levitated single particles
Schiemann, M. and Baer, S. and Esen, C. and Ostendorf, A.
CHEMICAL ENGINEERING AND TECHNOLOGY. Volume: 38 (2015) - 2015 • 3224
Holographic microscopy provides new insights into the settlement of zoospores of the green alga Ulva linza on cationic oligopeptide surfaces
Vater, S.M. and Finlay, J. and Callow, M.E. and Callow, J.A. and Ederth, T. and Liedberg, B. and Grunze, M. and Rosenhahn, A.
BIOFOULING. Volume: 31 (2015) - 2015 • 3223
Stereoscopic pyrometer for char combustion characterization
Schiemann, M. and Vorobiev, N. and Scherer, V.
APPLIED OPTICS. Volume: 54 (2015) - 2015 • 3222
Development and application of a Ni-Ti interatomic potential with high predictive accuracy of the martensitic phase transition
Ko, W.-S. and Grabowski, B. and Neugebauer, J.
PHYSICAL REVIEW B - CONDENSED MATTER AND MATERIALS PHYSICS. Volume: 92 (2015) - 2015 • 3221
Utilization of the array factor for narrowband direction of arrival estimation
Nalobin, A. and Rolfes, I.
IEEE ANTENNAS AND PROPAGATION SOCIETY, AP-S INTERNATIONAL SYMPOSIUM (DIGEST). Volume: 2015-October (2015) - 2015 • 3220
The Interaction of Formic Acid with Zinc Oxide: A Combined Experimental and Theoretical Study on Single Crystal and Powder Samples
Buchholz, M. and Li, Q. and Noei, H. and Nefedov, A. and Wang, Y. and Muhler, M. and Fink, K. and Wöll, C.
TOPICS IN CATALYSIS. Volume: 58 (2015) - 2015 • 3219
Development and analysis of a modified Saleh-Valenzuela channel model for the UHF band
Nalobin, A. and Dortmund, S. and Sczyslo, S. and Barowski, J. and Meiners, B. and Rolfes, I.
2015 GERMAN MICROWAVE CONFERENCE, GEMIC 2015. Volume: (2015) - 2015 • 3218
A quantitative metallographic assessment of the evolution of porosity during processing and creep in single crystal Ni-base super alloys
Buck, H. and Wollgramm, P. and Parsa, A.B. and Eggeler, G.
MATERIALWISSENSCHAFT UND WERKSTOFFTECHNIK. Volume: 46 (2015) - 2015 • 3217
Antibacterial activity of microstructured Ag/Au sacrificial anode thin films
Köller, M. and Sengstock, C. and Motemani, Y. and Khare, C. and Buenconsejo, P.J.S. and Geukes, J. and Schildhauer, T.A. and Ludwig, Al.
MATERIALS SCIENCE AND ENGINEERING C. Volume: 46 (2015) - 2015 • 3216
Solid electrolyte interphase in semi-solid flow batteries: A Wolf in sheep's clothing
Ventosa, E. and Zampardi, G. and Flox, C. and La Mantia, F. and Schuhmann, W. and Morante, J.R.
CHEMICAL COMMUNICATIONS. Volume: 51 (2015) - 2015 • 3215
New Au-Cu-Al thin film shape memory alloys with tunable functional properties and high thermal stability
Buenconsejo, P.J.S. and Ludwig, Al.
ACTA MATERIALIA. Volume: 85 (2015) - 2015 • 3214
Exploration of ternary subsystems of superalloys by high-throughput thin film experimentation: Optical and electrical data of the Co-Al-W system
Naujoks, D. and Koenig, D. and Ludwig, Al.
MATERIALS RESEARCH SOCIETY SYMPOSIUM PROCEEDINGS. Volume: 1760 (2015) - 2015 • 3213
Non-aqueous semi-solid flow battery based on Na-ion chemistry. P2-type Nax Ni0.22 Co0.11 Mn0.66 O2 -NaTi2 (PO4 )3
Ventosa, E. and Buchholz, D. and Klink, S. and Flox, C. and Chagas, L.G. and Vaalma, C. and Schuhmann, W. and Passerini, S. and Morante, J.R.
CHEMICAL COMMUNICATIONS. Volume: 51 (2015) - 2015 • 3212
X-ray photoelectron spectroscopy investigations of the surface reaction layer and its effects on the transformation properties of nanoscale Ti51 Ni38 Cu11 shape memory thin films
König, D. and Naujoks, D. and De Los Arcos, T. and Grosse-Kreul, S. and Ludwig, Al.
ADVANCED ENGINEERING MATERIALS. Volume: 17 (2015) - 2015 • 3211
Cycling Performance of a Columnar-Structured Complex Perovskite in a Temperature Gradient Test
Schlegel, N. and Sebold, D. and Sohn, Y.J. and Mauer, G. and Vaßen, R.
JOURNAL OF THERMAL SPRAY TECHNOLOGY. Volume: 24 (2015) - 2015 • 3210
Scanning electrochemical microscopy of Li-ion batteries
Ventosa, E. and Schuhmann, W.
PHYSICAL CHEMISTRY CHEMICAL PHYSICS. Volume: 17 (2015) - 2015 • 3209
Three-Dimensional, Fibrous Lithium Iron Phosphate Structures Deposited by Magnetron Sputtering
Bünting, A. and Uhlenbruck, S. and Sebold, D. and Buchkremer, H.P. and Vaßen, R.
ACS APPLIED MATERIALS AND INTERFACES. Volume: 7 (2015) - 2015 • 3208
A sterically stabilized FeI-FeI semi-rotated conformation of [FeFe] hydrogenase subsite model
Goy, R. and Bertini, L. and Elleouet, C. and Görls, H. and Zampella, G. and Talarmin, J. and De Gioia, L. and Schollhammer, P. and Apfel, U.-P. and Weigand, W.
DALTON TRANSACTIONS. Volume: 44 (2015) - 2015 • 3207
High-Throughput Investigation of the Oxidation and Phase Constitution of Thin-Film Ni-Al-Cr Materials Libraries
König, D. and Eberling, C. and Kieschnick, M. and Virtanen, S. and Ludwig, Al.
ADVANCED ENGINEERING MATERIALS. Volume: 17 (2015) - 2015 • 3206
Influence of titanium nitride interlayer on the morphology, structure and electrochemical performance of magnetron-sputtered lithium iron phosphate thin films
Bünting, A. and Uhlenbruck, S. and Dellen, C. and Finsterbusch, M. and Tsai, C.-L. and Sebold, D. and Buchkremer, H.P. and Vaßen, R.
JOURNAL OF POWER SOURCES. Volume: 281 (2015) - 2015 • 3205
Random phase approximation up to the melting point: Impact of anharmonicity and nonlocal many-body effects on the thermodynamics of Au
Grabowski, B. and Wippermann, S. and Glensk, A. and Hickel, T. and Neugebauer, J.
PHYSICAL REVIEW B - CONDENSED MATTER AND MATERIALS PHYSICS. Volume: 91 (2015) - 2015 • 3204
Nitrogen-doped carbon cloth as a stable self-supported cathode catalyst for air/H2 -breathing alkaline fuel cells
Vivekananthan, J. and Masa, J. and Chen, P. and Xie, K. and Muhler, M. and Schuhmann, W.
ELECTROCHIMICA ACTA. Volume: 182 (2015) - 2015 • 3203
Isoreticular isomerism in 4,4-connected paddle-wheel metal-organic frameworks: Structural prediction by the reverse topological approach
Bureekaew, S. and Balwani, V. and Amirjalayer, S. and Schmid, R.
CRYSTENGCOMM. Volume: 17 (2015) - 2015 • 3202
Polar Kerr effect from a time-reversal symmetry breaking unidirectional charge density wave
Gradhand, M. and Eremin, I. and Knolle, J.
PHYSICAL REVIEW B - CONDENSED MATTER AND MATERIALS PHYSICS. Volume: 91 (2015) - 2015 • 3201
Magnetostatic nearest neighbor interactions in a Co48Fe52 nanowire array probed by in-field magnetic force microscopy
Vock, S. and Tschulik, K. and Uhlemann, M. and Hengst, C. and Fähler, S. and Schultz, L. and Neu, V.
JOURNAL OF APPLIED PHYSICS. Volume: 118 (2015) - 2015 • 3200
Characterization of single coal particle combustion within oxygen-enriched environments using high-speed OH-PLIF
Köser, J. and Becker, L.G. and Vorobiev, N. and Schiemann, M. and Scherer, V. and Böhm, B. and Dreizler, A.
APPLIED PHYSICS B: LASERS AND OPTICS. Volume: 121 (2015) - 2015 • 3199
Downscaling metal-dielectric interface fracture experiments to sub-micron dimensions: A feasibility study using TEM
Völker, B. and Heinz, W. and Roth, R. and Batke, J.M. and Cordill, M.J. and Dehm, G.
SURFACE AND COATINGS TECHNOLOGY. Volume: 270 (2015) - 2015 • 3198
Following crack path selection in multifilm structures with weak and strong interfaces by in situ 4-point-bending
Völker, B. and Venkatesan, S. and Heinz, W. and Matoy, K. and Roth, R. and Batke, J.-M. and Cordill, M.J. and Dehm, G.
JOURNAL OF MATERIALS RESEARCH. Volume: 30 (2015) - 2015 • 3197
Elastocaloric cooling with ni-Ti based alloys - material characterization and process variation
Schmidt, M. and Ullrich, J. and Wieczorek, A. and Frenzel, J. and Schötze, A. and Eggeler, G. and Seelecke, S.
ASME 2015 CONFERENCE ON SMART MATERIALS, ADAPTIVE STRUCTURES AND INTELLIGENT SYSTEMS, SMASIS 2015. Volume: 1 (2015) - 2015 • 3196
Interface fracture and chemistry of a tungsten-based metallization on borophosphosilicate glass
Völker, B. and Heinz, W. and Matoy, K. and Roth, R. and Batke, J.M. and Schöberl, T. and Scheu, C. and Dehm, G.
PHILOSOPHICAL MAGAZINE. Volume: 95 (2015) - 2015 • 3195
Temperature dependence of the electron Landé g -factor in cubic GaN
Buß, J.H. and Schupp, T. and As, D.J. and Hägele, D. and Rudolph, J.
JOURNAL OF APPLIED PHYSICS. Volume: 118 (2015) - 2015 • 3194
Heat transfer in indirect heated rotary drums filled with monodisperse spheres: Comparison of experiments with DEM simulations
Komossa, H. and Wirtz, S. and Scherer, V. and Herz, F. and Specht, E.
POWDER TECHNOLOGY. Volume: 286 (2015) - 2015 • 3193
Mechanical and chemical investigation of the interface between tungsten-based metallizations and annealed borophosphosilicate glass
Völker, B. and Heinz, W. and Matoy, K. and Roth, R. and Batke, J.M. and Schöberl, T. and Cordill, M.J. and Dehm, G.
THIN SOLID FILMS. Volume: 583 (2015) - 2015 • 3192
Cocatalyst Designing: A Regenerable Molybdenum-Containing Ternary Cocatalyst System for Efficient Photocatalytic Water Splitting
Busser, G.W. and Mei, B. and Weide, P. and Vesborg, P.C.K. and Stührenberg, K. and Bauer, M. and Huang, X. and Willinger, M.-G. and Chorkendorff, I. and Schlögl, R. and Muhler, M.
ACS CATALYSIS. Volume: 5 (2015) - 2015 • 3191
Multivariate Characterization of a Continuous Soot Monitoring System Based on Raman Spectroscopy
Grafen, M. and Nalpantidis, K. and Platte, F. and Monz, C. and Ostendorf, A.
AEROSOL SCIENCE AND TECHNOLOGY. Volume: 49 (2015) - 2015 • 3190
Representing the potential-energy surface of protonated water clusters by high-dimensional neural network potentials
Kondati Natarajan, S. and Morawietz, T. and Behler, J.
PHYSICAL CHEMISTRY CHEMICAL PHYSICS. Volume: 17 (2015) - 2015 • 3189
Influence of isotropic and anisotropic material models on the mechanical response in arterial walls as a result of supra-physiological loadings
Schmidt, T. and Pandya, D. and Balzani, D.
MECHANICS RESEARCH COMMUNICATIONS. Volume: 64 (2015) - 2015 • 3188
Fe-Mg diffusion in spinel: New experimental data and a point defect model
Vogt, K. and Dohmen, R. and Chakraborty, S.
AMERICAN MINERALOGIST. Volume: 100 (2015) - 2015 • 3187
Phenolated Oleic Acid Based Polybenzoxazine Derivatives as Corrosion Protection Layers
Bələnucə, B. and Raicopol, M. and Maljusch, A. and Garea, S. and Hanganu, A. and Schuhmann, W. and Andronescu, C.
CHEMPLUSCHEM. Volume: 80 (2015) - 2015 • 3186
Quantile Correlations: Uncovering Temporal Dependencies in Financial Time Series
Schmitt, T. A. and Schafer, R. and Dette, H. and Guhr, T.
INTERNATIONAL JOURNAL OF THEORETICAL AND APPLIED FINANCE. Volume: 18 (2015) - 2015 • 3185
Detecting gradual changes in locally stationary processes
Vogt, M. and Dette, H.
ANNALS OF STATISTICS. Volume: 43 (2015) - 2015 • 3184
Effects of strain amplitude, cycle number and orientation on low cycle fatigue microstructures in austenitic stainless steel studied by electron channelling contrast imaging
Nellessen, J. and Sandlöbes, S. and Raabe, D.
ACTA MATERIALIA. Volume: 87 (2015) - 2015 • 3183
An 80 GHz radar level measurement system with dielectric lens antenna
Vogt, M. and Schulz, C. and Dahl, C. and Rolfes, I. and Gerding, M.
PROCEEDINGS INTERNATIONAL RADAR SYMPOSIUM. Volume: 2015-August (2015) - 2015 • 3182
Epitaxial lift-off for solid-state cavity quantum electrodynamics
Greuter, L. and Najer, D. and Kuhlmann, A.V. and Valentin, S.R. and Ludwig, Ar. and Wieck, A.D. and Starosielec, S. and Warburton, R.J.
JOURNAL OF APPLIED PHYSICS. Volume: 118 (2015) - 2015 • 3181
Tutorial: Laser in material nanoprocessing
König, K. and Ostendorf, A.
OPTICALLY INDUCED NANOSTRUCTURES: BIOMEDICAL AND TECHNICAL APPLICATIONS. Volume: (2015) - 2015 • 3180
Assessment of geometrically necessary dislocation levels derived by 3D EBSD
Konijnenberg, P.J. and Zaefferer, S. and Raabe, D.
ACTA MATERIALIA. Volume: 99 (2015) - 2015 • 3179
Influence of Solvent-Like Sidechains on the Adsorption of Light Hydrocarbons in Metal-Organic Frameworks
Schneemann, A. and Bloch, E.D. and Henke, S. and Llewellyn, P.L. and Long, J.R. and Fischer, R.A.
CHEMISTRY - A EUROPEAN JOURNAL. Volume: 21 (2015) - 2015 • 3178
Pressure drop investigations in packings of arbitrary shaped particles
Vollmari, K. and Oschmann, T. and Wirtz, S. and Kruggel-Emden, H.
POWDER TECHNOLOGY. Volume: 271 (2015) - 2015 • 3177
Finding optimal surface sites on heterogeneous catalysts by counting nearest neighbors
Calle-Vallejo, F. and Tymoczko, J. and Colic, V. and Vu, Q.H. and Pohl, M.D. and Morgenstern, K. and Loffreda, D. and Sautet, P. and Schuhmann, W. and Bandarenka, A.S.
SCIENCE. Volume: 350 (2015) - 2015 • 3176
Locally optimal designs for errors-in-variables models
Konstantinou, M. and Dette, H.
BIOMETRIKA. Volume: 102 (2015) - 2015 • 3175
Optimal placement of piezoelectric actuators and sensors on a smart beam and a smart plate using multi-objective genetic algorithm
Nestorović, T. and Trajkov, M. and Garmabi, S.
SMART STRUCTURES AND SYSTEMS. Volume: 15 (2015) - 2015 • 3174
Mullite: Crystal Structure and Related Properties
Schneider, H. and Fischer, R.X. and Schreuer, J.
JOURNAL OF THE AMERICAN CERAMIC SOCIETY. Volume: 98 (2015) - 2015 • 3173
Cd and impurity redistribution at the p-n junction of CIGS based solar cells resolved by atom-probe tomography
Koprek, A. and Cojocaru-Miredin, O. and Wuerz, R. and Freysoldt, C. and Raabe, D.
2015 IEEE 42ND PHOTOVOLTAIC SPECIALIST CONFERENCE, PVSC 2015. Volume: (2015) - 2015 • 3172
Numerical and experimental investigation of the pressure drop in packings of spherical and non-spherical particles
Vollmari, K. and Khan, M.S. and Oschmann, T. and Kruggel-Emden, H.
AIP CONFERENCE PROCEEDINGS. Volume: 1648 (2015) - 2015 • 3171
Electron-phonon interaction and thermal boundary resistance at the crystal-amorphous interface of the phase change compound GeTe
Campi, D. and Donadio, D. and Sosso, G.C. and Behler, J. and Bernasconi, M.
JOURNAL OF APPLIED PHYSICS. Volume: 117 (2015) - 2015 • 3170
Damage evolution in pseudoelastic polycrystalline Co-Ni-Ga high-temperature shape memory alloys
Vollmer, M. and Krooß, P. and Segel, C. and Weidner, A. and Paulsen, A. and Frenzel, J. and Schaper, M. and Eggeler, G. and Maier, H.J. and Niendorf, T.
JOURNAL OF ALLOYS AND COMPOUNDS. Volume: 633 (2015) - 2015 • 3169
Electrochemical sensor for nitric oxide using layered films composed of a polycationic dendrimer and nickel(II) phthalocyaninetetrasulfonate deposited on a carbon fiber electrode
Cancino, J. and Borgmann, S. and Machado, S.A.S. and Zucolotto, V. and Schuhmann, W. and Masa, J.
MICROCHIMICA ACTA. Volume: 182 (2015) - 2015 • 3168
Domain Structure and Magnetoresistance in Co2MnGe Zigzag Structures
Gross, K. and Westerholt, K. and Gómez, M.E. and Zabel, H.
PHYSICS PROCEDIA. Volume: 75 (2015) - 2015 • 3167
The influence of low level pre- and perinatal exposure to PCDD/Fs, PCBs, and lead on attention performance and attention-related behavior among German school-aged children: Results from the Duisburg Birth Cohort Study
Neugebauer, J. and Wittsiepe, J. and Kasper-Sonnenberg, M. and Schoneck, N. and Scholmerich, A. and Wilhelm, M.
INTERNATIONAL JOURNAL OF HYGIENE AND ENVIRONMENTAL HEALTH. Volume: 218 (2015) - 2015 • 3166
Mass spectrometry of atmospheric pressure plasmas
Grosse-Kreul, S. and Hubner, S. and Schneider, S. and Ellerweg, D. and von Keudell, A. and Matejcik, S. and Benedikt, J.
PLASMA SOURCES SCIENCE & TECHNOLOGY. Volume: 24 (2015) - 2015 • 3165
Microsegregation and precipitates of an as-cast Co-based superalloy—microstructural characterization and phase stability modelling
Koßmann, J. and Zenk, C.H. and Lopez-Galilea, I. and Neumeier, S. and Kostka, A. and Huth, S. and Theisen, W. and Göken, M. and Drautz, R. and Hammerschmidt, T.
JOURNAL OF MATERIALS SCIENCE. Volume: 50 (2015) - 2015 • 3164
Effect of process parameters on the formation of laser-induced nanoparticles during material processing with continuous solid-state lasers
Scholz, T. and Dickmann, K. and Ostendorf, A. and Uphoff, H. and Michalewicz, M.
JOURNAL OF LASER APPLICATIONS. Volume: 27 (2015) - 2015 • 3163
Solubility and ordering of Ti, Ta, Mo and W on the Al sublattice in L12 -Co3 Al
Koßmann, J. and Hammerschmidt, T. and Maisel, S. and Müller, S. and Drautz, R.
INTERMETALLICS. Volume: 64 (2015) - 2015 • 3162
Comparison of design and torque measurements of various manual wrenches
Neugebauer, J. and Petermöller, S. and Scheer, M. and Happe, A. and Faber, F.-J. and Zoeller, J.E.
INTERNATIONAL JOURNAL OF ORAL AND MAXILLOFACIAL IMPLANTS. Volume: 30 (2015) - 2015 • 3161
Semi-artificial photosynthetic z-scheme for hydrogen production from water
Kothe, T. and Schuhmann, W. and Rögner, M. and Plumeré, N.
BIOHYDROGEN. Volume: (2015) - 2015 • 3160
Special issue: Crystal physics in Germany - The lifework of siegfried haussühl (∗25th November 1927, †07th January 2014)
Schreuer, J.
ZEITSCHRIFT FUR KRISTALLOGRAPHIE - CRYSTALLINE MATERIALS. Volume: 230 (2015) - 2015 • 3159
Ab initio phase diagram of BaTiO3 under epitaxial strain revisited
Grünebohm, A. and Marathe, M. and Ederer, C.
APPLIED PHYSICS LETTERS. Volume: 107 (2015) - 2015 • 3158
Spatially and kinetically resolved mapping of hydrogen in a twinning-induced plasticity steel by use of Scanning Kelvin Probe Force Microscopy
Koyama, M. and Bashir, A. and Rohwerder, M. and Merzlikin, S.V. and Akiyama, E. and Tsuzaki, K. and Raabe, D.
JOURNAL OF THE ELECTROCHEMICAL SOCIETY. Volume: 162 (2015) - 2015 • 3157
Influence of the Bi 6s2 lone electron pair on elastic properties of monoclinic Bi4B2O9
Schreuer, J. and Mühlberg, M. and Burianek, M. and Wallrafen, F.
ZEITSCHRIFT FUR KRISTALLOGRAPHIE - CRYSTALLINE MATERIALS. Volume: 230 (2015) - 2015 • 3156
Linking atomistic, kinetic Monte Carlo and crystal plasticity simulations of single-crystal tungsten strength
Cereceda, D. and Diehl, M. and Roters, F. and Shanthraj, P. and Raabe, D. and Perlado, J.M. and Marian, J.
GAMM MITTEILUNGEN. Volume: 38 (2015) - 2015 • 3155
Fundamentals and Applications of Reflection FTIR Spectroscopy for the Analysis of Plasma Processes at Materials Interfaces
Grundmeier, G. and von Keudell, A. and de los Arcos, T.
PLASMA PROCESSES AND POLYMERS. Volume: 12 (2015) - 2015 • 3154
Selective enzymatic removal of elastin and collagen from human abdominal aortas: Uniaxial mechanical response and constitutive modeling
Schriefl, A.J. and Schmidt, T. and Balzani, D. and Sommer, G. and Holzapfel, G.A.
ACTA BIOMATERIALIA. Volume: 17 (2015) - 2015 • 3153
Homogeneity and composition of AlInGaN: A multiprobe nanostructure study
Krause, F.F. and Ahl, J.-P. and Tytko, D. and Choi, P.-P. and Egoavil, R. and Schowalter, M. and Mehrtens, T. and Müller-Caspary, K. and Verbeeck, J. and Raabe, D. and Hertkorn, J. and Engl, K. and Rosenauer, A.
ULTRAMICROSCOPY. Volume: 156 (2015) - 2015 • 3152
Magnetic control: Switchable ultrahigh magnetic gradients at Fe3 O4 nanoparticles to enhance solution-phase mass transport
Ngamchuea, K. and Tschulik, K. and Compton, R.G.
NANO RESEARCH. Volume: 8 (2015) - 2015 • 3151
Martensitic transformation between competing phases in Ti-Ta alloys: A solid-state nudged elastic band study
Chakraborty, T. and Rogal, J. and Drautz, R.
JOURNAL OF PHYSICS CONDENSED MATTER. Volume: 27 (2015) - 2015 • 3150
Element-resolved thermodynamics of magnetocaloric lafe13-xsix
Gruner, M.E. and Keune, W. and Roldan Cuenya, B. and Weis, C. and Landers, J. and Makarov, S.I. and Klar, D. and Hu, M.Y. and Alp, E.E. and Zhao, J. and Krautz, M. and Gutfleisch, O. and Wende, H.
PHYSICAL REVIEW LETTERS. Volume: 114 (2015) - 2015 • 3149
Coupled three dimensional DEM-CFD simulation of a lime shaft kiln-Calcination, particle movement and gas phase flow field
Krause, B. and Liedmann, B. and Wiese, J. and Wirtz, S. and Scherer, V.
CHEMICAL ENGINEERING SCIENCE. Volume: 134 (2015) - 2015 • 3148
In Situ Detection of Particle Aggregation on Electrode Surfaces
Ngamchuea, K. and Tschulik, K. and Eloul, S. and Compton, R.G.
CHEMPHYSCHEM. Volume: 16 (2015) - 2015 • 3147
Large scale Molecular Dynamics simulation of microstructure formation during thermal spraying of pure copper
Wang, T. and Begau, C. and Sutmann, G. and Hartmaier, A.
SURFACE AND COATINGS TECHNOLOGY. Volume: 280 (2015) - 2015 • 3146
Advancing from Rules of Thumb: Quantifying the Effects of Small Density Changes in Mass Transport to Electrodes. Understanding Natural Convection
Ngamchuea, K. and Eloul, S. and Tschulik, K. and Compton, R.G.
ANALYTICAL CHEMISTRY. Volume: 87 (2015) - 2015 • 3145
Development and application of a thermal lattice Boltzmann scheme
Kravets, B. and Khan, M.S. and Kruggel-Emden, H.
AIP CONFERENCE PROCEEDINGS. Volume: 1648 (2015) - 2015 • 3144
New insight into calcium tantalate nanocomposite photocatalysts for overall water splitting and reforming of alcohols and biomass derivatives
Wang, P. and Weide, P. and Muhler, M. and Marschall, R. and Wark, M.
APL MATERIALS. Volume: 3 (2015) - 2015 • 3143
Highly Ordered Mesoporous Cobalt-Containing Oxides: Structure, Catalytic Properties, and Active Sites in Oxidation of Carbon Monoxide
Gu, D. and Jia, C.-J. and Weidenthaler, C. and Bongard, H.-J. and Spliethoff, B. and Schmidt, W. and Schüth, F.
JOURNAL OF THE AMERICAN CHEMICAL SOCIETY. Volume: 137 (2015) - 2015 • 3142
Magnetoconductance of a magnetic double barrier in a quantum wire
Schüler, B. and Cerchez, M. and Xu, H. and Heinzel, T. and Reuter, D. and Wieck, A.D.
SUPERLATTICES AND MICROSTRUCTURES. Volume: 79 (2015) - 2015 • 3141
Enhancing Hydrogen Embrittlement Resistance of Lath Martensite by Introducing Nano-Films of Interlath Austenite
Wang, M. and Tasan, C.C. and Koyama, M. and Ponge, D. and Raabe, D.
METALLURGICAL AND MATERIALS TRANSACTIONS A: PHYSICAL METALLURGY AND MATERIALS SCIENCE. Volume: 46 (2015) - 2015 • 3140
Controllable Synthesis of Mesoporous Peapod-like Co3 O4 @Carbon Nanotube Arrays for High-Performance Lithium-Ion Batteries
Gu, D. and Li, W. and Wang, F. and Bongard, H. and Spliethoff, B. and Schmidt, W. and Weidenthaler, C. and Xia, Y. and Zhao, D. and Schüth, F.
ANGEWANDTE CHEMIE - INTERNATIONAL EDITION. Volume: 54 (2015) - 2015 • 3139
Nanolaminate transformation-induced plasticity-twinning-induced plasticity steel with dynamic strain partitioning and enhanced damage resistance
Wang, M.-M. and Tasan, C.C. and Ponge, D. and Dippel, A.-Ch. and Raabe, D.
ACTA MATERIALIA. Volume: 85 (2015) - 2015 • 3138
Interaction between nitrogen and sulfur in co-doped graphene and synergetic effect in supercapacitor
Wang, T. and Wang, L.-X. and Wu, D.-L. and Xia, W. and Jia, D.-Z.
SCIENTIFIC REPORTS. Volume: 5 (2015) - 2015 • 3137
CNT-TiO2-δ composites for improved co-catalyst dispersion and stabilized photocatalytic hydrogen production
Chen, P. and Wang, L. and Wang, P. and Kostka, A. and Wark, M. and Muhler, M. and Beranek, R.
CATALYSTS. Volume: 5 (2015) - 2015 • 3136
Mechanical properties, microstructure and thermal stability of a nanocrystalline CoCrFeMnNi high-entropy alloy after severe plastic deformation
Schuh, B. and Mendez-Martin, F. and Völker, B. and George, E.P. and Clemens, H. and Pippan, R. and Hohenwarter, A.
ACTA MATERIALIA. Volume: 96 (2015) - 2015 • 3135
Fabrication and characterisation of gallium arsenide ambipolar quantum point contacts
Chen, J.C.H. and Klochan, O. and Micolich, A.P. and Das Gupta, K. and Sfigakis, F. and Ritchie, D.A. and Trunov, K. and Reuter, D. and Wieck, A.D. and Hamilton, A.R.
APPLIED PHYSICS LETTERS. Volume: 106 (2015) - 2015 • 3134
Rate-independent versus viscous evolution of laminate microstructures in finite crystal plasticity
Günther, C. and Kochmann, D.M. and Hackl, K.
LECTURE NOTES IN APPLIED AND COMPUTATIONAL MECHANICS. Volume: 78 (2015) - 2015 • 3133
Evolution of microstructure and mechanical properties of coated Co-base superalloys during heat treatment and thermal exposure
Webler, R. and Ziener, M. and Neumeier, S. and Terberger, P.J. and Vaßen, R. and Göken, M.
MATERIALS SCIENCE AND ENGINEERING A. Volume: 628 (2015) - 2015 • 3132
A variational viscosity-limit approach to the evolution of microstructures in finite crystal plasticity
Günther, C. and Junker, P. and Hackl, K.
PROCEEDINGS OF THE ROYAL SOCIETY A: MATHEMATICAL, PHYSICAL AND ENGINEERING SCIENCES. Volume: 471 (2015) - 2015 • 3131
Measurement of drag coefficients of non-spherical particles with a camera-based method
Krueger, B. and Wirtz, S. and Scherer, V.
POWDER TECHNOLOGY. Volume: 278 (2015) - 2015 • 3130
ICME for Crashworthiness of TWIP Steels: From Ab Initio to the Crash Performance
Güvenç, O. and Roters, F. and Hickel, T. and Bambach, M.
JOM. Volume: 67 (2015) - 2015 • 3129
Numerical investigation of a continuous screening process by the discrete element method
Kruggel-Emden, H. and Elskamp, F.
AIP CONFERENCE PROCEEDINGS. Volume: 1648 (2015) - 2015 • 3128
The Planar Multipole Resonance Probe: Challenges and Prospects of a Planar Plasma Sensor
Schulz, C. and Styrnoll, T. and Awakowicz, P. and Rolfes, I.
IEEE TRANSACTIONS ON INSTRUMENTATION AND MEASUREMENT. Volume: 64 (2015) - 2015 • 3127
Laser-diode-based photoacoustic setup to analyze Grüneisen relaxation-effect induced signal enhancement
Cherkashin, M. and Brenner, C. and Göring, L. and Döpke, B. and Gerhardt, N.C. and Hofmann, M.R.
PROGRESS IN BIOMEDICAL OPTICS AND IMAGING - PROCEEDINGS OF SPIE. Volume: 9539 (2015) - 2015 • 3126
Deformation induced alloying in crystalline - metallic glass nano-composites
Guo, W. and Yao, J. and Jägle, E.A. and Choi, P.-P. and Herbig, M. and Schneider, J.M. and Raabe, D.
MATERIALS SCIENCE AND ENGINEERING A. Volume: 628 (2015) - 2015 • 3125
Electron density determination for plasma assisted sterilization processes
Schulz, C. and Runkel, J. and Rolfes, I.
2015 IEEE MTT-S INTERNATIONAL MICROWAVE WORKSHOP SERIES ON RF AND WIRELESS TECHNOLOGIES FOR BIOMEDICAL AND HEALTHCARE APPLICATIONS, IMWS-BIO 2015 - PROCEEDINGS. Volume: (2015) - 2015 • 3124
Uniform 2 nm gold nanoparticles supported on iron oxides as active catalysts for CO oxidation reaction: Structure-activity relationship
Guo, Y. and Gu, D. and Jin, Z. and Du, P.-P. and Si, R. and Tao, J. and Xu, W.-Q. and Huang, Y.-Y. and Senanayake, S. and Song, Q.-S. and Jia, C.-J. and Schüth, F.
NANOSCALE. Volume: 7 (2015) - 2015 • 3123
Martensite aging - Avenue to new high temperature shape memory alloys
Niendorf, T. and Krooß, P. and Somsen, C. and Eggeler, G. and Chumlyakov, Y.I. and Maier, H.J.
ACTA MATERIALIA. Volume: 89 (2015) - 2015 • 3122
Parallelization concept for spatially resolved in-situ plasma measurements
Schulz, C. and Runkel, J. and Rolfes, I.
2015 IEEE MTT-S INTERNATIONAL MICROWAVE SYMPOSIUM, IMS 2015. Volume: (2015) - 2015 • 3121
Oxygen-plasma-functionalized carbon nanotubes as supports for platinum-ruthenium catalysts applied in electrochemical methanol oxidation
Chetty, R. and Maniam, K.K. and Schuhmann, W. and Muhler, M.
CHEMPLUSCHEM. Volume: 80 (2015) - 2015 • 3120
Investigating the influence of crystal orientation on bending size effect of single crystal beams
Gupta, S. and Ma, A. and Hartmaier, A.
COMPUTATIONAL MATERIALS SCIENCE. Volume: 101 (2015) - 2015 • 3119
Cyclic degradation of titanium-tantalum high-temperature shape memory alloys - The role of dislocation activity and chemical decomposition
Niendorf, T. and Krooß, P. and Somsen, C. and Rynko, R. and Paulsen, A. and Batyrshina, E. and Frenzel, J. and Eggeler, G. and Maier, H.J.
FUNCTIONAL MATERIALS LETTERS. Volume: 8 (2015) - 2015 • 3118
Investigation of a circular TE11 -TE01 -mode converter in stepped waveguide technique
Schulz, C. and Baer, C. and Musch, T. and Rolfes, I. and Will, B.
INTERNATIONAL JOURNAL OF MICROWAVE AND WIRELESS TECHNOLOGIES. Volume: 7 (2015) - 2015 • 3117
Shear-Induced Detachment of Polystyrene Beads from SAM-Coated Surfaces
Cho, K.L. and Rosenhahn, A. and Thelen, R. and Grunze, M. and Lobban, M. and Karahka, M.L. and Kreuzer, H.J.
LANGMUIR. Volume: 31 (2015) - 2015 • 3116
Nitrogen uptake of nickel free austenitic stainless steel powder during heat treatment-an XPS study
Weddeling, A. and Lefor, K. and Hryha, E. and Huth, S. and Nyborg, L. and Weber, S. and Theisen, W.
SURFACE AND INTERFACE ANALYSIS. Volume: 47 (2015) - 2015 • 3115
Size and orientation effects in partial dislocation-mediated deformation of twinning-induced plasticity steel micro-pillars
Choi, W.S. and De Cooman, B.C. and Sandlöbes, S. and Raabe, D.
ACTA MATERIALIA. Volume: 98 (2015) - 2015 • 3114
Model update and real-time steering of tunnel boring machines using simulation-based meta models
Ninić, J. and Meschke, G.
TUNNELLING AND UNDERGROUND SPACE TECHNOLOGY. Volume: 45 (2015) - 2015 • 3113
Spin reorientation driven by the interplay between spin-orbit coupling and Hund's rule coupling in iron pnictides
Christensen, M.H. and Kang, J. and Andersen, B.M. and Eremin, I. and Fernandes, R.M.
PHYSICAL REVIEW B - CONDENSED MATTER AND MATERIALS PHYSICS. Volume: 92 (2015) - 2015 • 3112
Efficient Deposition of Semiconductor Powders for Photoelectrocatalysis by Airbrush Spraying
Gutkowski, R. and Schäfer, D. and Nagaiah, T.C. and Heras, J.E.Y. and Busser, W. and Muhler, M. and Schuhmann, W.
ELECTROANALYSIS. Volume: 27 (2015) - 2015 • 3111
Thermo-physical properties of heat-treatable steels in the temperature range relevant for hot-stamping applications
Kuepferle, J. and Wilzer, J. and Weber, S. and Theisen, W.
JOURNAL OF MATERIALS SCIENCE. Volume: 50 (2015) - 2015 • 3110
Transform-limited single photons from a single quantum dot
Kuhlmann, A.V. and Prechtel, J.H. and Houel, J. and Ludwig, Ar. and Reuter, D. and Wieck, A.D. and Warburton, R.J.
NATURE COMMUNICATIONS. Volume: 6 (2015) - 2015 • 3109
The effect of cast microstructure and crystallography on rafting, dislocation plasticity and creep anisotropy of single crystal Ni-base superalloys
Nörtershäuser, P. and Frenzel, J. and Ludwig, Al. and Neuking, K. and Eggeler, G.
MATERIALS SCIENCE AND ENGINEERING A. Volume: 626 (2015) - 2015 • 3108
Optical and microwave properties of focused ion beam implanted Erbium ions in Y2SiO5 crystals
Kukharchyk, N. and Probst, S. and Pal, S. and Xia, K. and Kolesov, R. and Ludwig, Ar. and Ustinov, A.V. and Bushev, P. and Wieck, A.D.
CONFERENCE ON LASERS AND ELECTRO-OPTICS EUROPE - TECHNICAL DIGEST. Volume: 2015-August (2015) - 2015 • 3107
From hard to rubber-like: mechanical properties of resorcinol–formaldehyde aerogels
Schwan, M. and Naikade, M. and Raabe, D. and Ratke, L.
JOURNAL OF MATERIALS SCIENCE. Volume: 50 (2015) - 2015 • 3106
Higgs mechanism, phase transitions, and anomalous Hall effect in three-dimensional topological superconductors
Nogueira, F.S. and Sudbø, A. and Eremin, I.
PHYSICAL REVIEW B - CONDENSED MATTER AND MATERIALS PHYSICS. Volume: 92 (2015) - 2015 • 3105
Self-sufficient sensor for oxygen detection in packaging via radio-frequency identification
Weigel, C. and Schneider, M. and Schmitt, J. and Hoffmann, M. and Kahl, S. and Jurisch, R.
JOURNAL OF SENSORS AND SENSOR SYSTEMS. Volume: 4 (2015) - 2015 • 3104
Selection of longitudinal modes in a terahertz quantum cascade laser via narrow-band injection seeding
Nong, H. and Pal, S. and Markmann, S. and Hekmat, N. and Mohandas, R.A. and Dean, P. and Li, L. and Linfield, E.H. and Giles Davis, A. and Wieck, A.D. and Jukam, N.
CLEO: SCIENCE AND INNOVATIONS, CLEO-SI 2015. Volume: (2015) - 2015 • 3103
Detection of Cu2Zn5SnSe8 and Cu2Zn6SnSe9 phases in co-evaporated Cu2ZnSnSe4 thin-films
Schwarz, T. and Marques, M.A.L. and Botti, S. and Mousel, M. and Redinger, A. and Siebentritt, S. and Cojocaru-Mirédin, O. and Raabe, D. and Choi, P.-P.
APPLIED PHYSICS LETTERS. Volume: 107 (2015) - 2015 • 3102
Temperature-Induced Modulation of the Sample Position in Scanning Electrochemical Microscopy
Clausmeyer, J. and Schäfer, D. and Nebel, M. and Schuhmann, W.
CHEMELECTROCHEM. Volume: 2 (2015) - 2015 • 3101
The effect of charged quantum dots on the mobility of a two-dimensional electron gas: How important is the Coulomb scattering?
Kurzmann, A. and Beckel, A. and Ludwig, Ar. and Wieck, A.D. and Lorke, A. and Geller, M.
JOURNAL OF APPLIED PHYSICS. Volume: 117 (2015) - 2015 • 3100
Atom probe tomography study of internal interfaces in Cu2 ZnSnSe4 thin-films
Schwarz, T. and Cojocaru-Mirédin, O. and Choi, P. and Mousel, M. and Redinger, A. and Siebentritt, S. and Raabe, D.
JOURNAL OF APPLIED PHYSICS. Volume: 118 (2015) - 2015 • 3099
Conformal mm-wave antennas for catheter embedded atherosclerotic plaque sensors
Notzon, G. and Baer, C. and Musch, T. and Dahl, C. and Rolfes, I.
EUROPEAN MICROWAVE WEEK 2015: "FREEDOM THROUGH MICROWAVES", EUMW 2015 - CONFERENCE PROCEEDINGS; 2015 45TH EUROPEAN MICROWAVE CONFERENCE PROCEEDINGS, EUMC. Volume: (2015) - 2015 • 3098
Relationship Between Damping Capacity and Variations of Vacancies Concentration and Segregation of Carbon Atom in an Fe-Mn Alloy
Wen, Y. and Xiao, H. and Peng, H. and Li, N. and Raabe, D.
METALLURGICAL AND MATERIALS TRANSACTIONS A: PHYSICAL METALLURGY AND MATERIALS SCIENCE. Volume: 46 (2015) - 2015 • 3097
Interface engineering and characterization at the atomic-scale of pure and mixed ion layer gas reaction buffer layers in chalcopyrite thin-film solar cells
Cojocaru-Mirédin, O. and Fu, Y. and Kostka, A. and Sáez-Araoz, R. and Beyer, A. and Knaub, N. and Volz, K. and Fischer, C.-H. and Raabe, D.
PROGRESS IN PHOTOVOLTAICS: RESEARCH AND APPLICATIONS. Volume: 23 (2015) - 2015 • 3096
Linear complexions: Confined chemical and structural states at dislocations
Kuzmina, M. and Herbig, M. and Ponge, D. and Sandlöbes, S. and Raabe, D.
SCIENCE. Volume: 349 (2015) - 2015 • 3095
Experimental Aspects in Benchmarking of the Electrocatalytic Activity
Čolić, V. and Tymoczko, J. and Maljusch, A. and Ganassin, A. and Schuhmann, W., Prof. and Bandarenka, A.S.
CHEMELECTROCHEM. Volume: 2 (2015) - 2015 • 3094
Grain boundary segregation engineering and austenite reversion turn embrittlement into toughness: Example of a 9 wt.% medium Mn steel
Kuzmina, M. and Ponge, D. and Raabe, D.
ACTA MATERIALIA. Volume: 86 (2015) - 2015 • 3093
Influence of Temperature and Tempering Conditions on Thermal Conductivity of Hot Work Tool Steels for Hot Stamping Applications
Hafenstein, S. and Werner, E. and Wilzer, J. and Theisen, W. and Weber, S. and Sunderkötter, C. and Bachmann, M.
STEEL RESEARCH INTERNATIONAL. Volume: 86 (2015) - 2015 • 3092
Wet Nanoindentation of the Solid Electrolyte Interphase on Thin Film Si Electrodes
Kuznetsov, V. and Zinn, A.-H. and Zampardi, G. and Borhani-Haghighi, S. and La Mantia, F. and Ludwig, Al. and Schuhmann, W. and Ventosa, E.
ACS APPLIED MATERIALS AND INTERFACES. Volume: 7 (2015) - 2015 • 3091
Controlling the charge of pH-responsive redox hydrogels by means of redox-silent biocatalytic processes. A biocatalytic off/on switch
Contin, A. and Plumeré, N. and Schuhmann, W.
ELECTROCHEMISTRY COMMUNICATIONS. Volume: 51 (2015) - 2015 • 3090
Characterization of dislocation structures and deformation mechanisms in as-grown and deformed directionally solidified NiAl-Mo composites
Kwon, J. and Bowers, M.L. and Brandes, M.C. and McCreary, V. and Robertson, I.M. and Phani, P.S. and Bei, H. and Gao, Y.F. and Pharr, G.M. and George, E.P. and Mills, M.J.
ACTA MATERIALIA. Volume: 89 (2015) - 2015 • 3089
Lipid Carbonyl Groups Terminate the Hydrogen Bond Network of Membrane-Bound Water
Ohto, T. and Backus, E.H.G. and Hsieh, C.-S. and Sulpizi, M. and Bonn, M. and Nagata, Y.
JOURNAL OF PHYSICAL CHEMISTRY LETTERS. Volume: 6 (2015) - 2015 • 3088
A pH Responsive Redox Hydrogel for Electrochemical Detection of Redox Silent Biocatalytic Processes. Control of Hydrogel Solvation
Contin, A. and Frasca, S. and Vivekananthan, J. and Leimkühler, S. and Wollenberger, U. and Plumeré, N. and Schuhmann, W.
ELECTROANALYSIS. Volume: 27 (2015) - 2015 • 3087
Polycrystalline elastic moduli of a high-entropy alloy at cryogenic temperatures
Haglund, A. and Koehler, M. and Catoor, D. and George, E.P. and Keppens, V.
INTERMETALLICS. Volume: 58 (2015) - 2015 • 3086
A dielectric barrier discharge terminally inactivates RNase A by oxidizing sulfur-containing amino acids and breaking structural disulfide bonds
Lackmann, J.-W. and Baldus, S. and Steinborn, E. and Edengeiser, E. and Kogelheide, F. and Langklotz, S. and Schneider, S. and Leichert, L.I.O. and Benedikt, J. and Awakowicz, P. and Bandow, J.E.
JOURNAL OF PHYSICS D: APPLIED PHYSICS. Volume: 48 (2015) - 2015 • 3085
Synthesis and electrochromic properties of conducting polymers based on highly planar 2,7-disubstituted xanthene derivatives
Olech, K. and Gutkowski, R. and Kuznetsov, V., Dr. and Roszak, S., Prof. Dr. and Sołoducho, J. and Schuhmann, W.
CHEMPLUSCHEM. Volume: 80 (2015) - 2015 • 3084
Exploring the Structure of the Modified Top Layer of Polypropylene During Plasma Treatment
Corbella, C. and Grosse-Kreul, S. and von Keudell, A.
PLASMA PROCESSES AND POLYMERS. Volume: 12 (2015) - 2015 • 3083
Structural stability of Fe-based topologically close-packed phases
Ladines, A.N. and Hammerschmidt, T. and Drautz, R.
INTERMETALLICS. Volume: 59 (2015) - 2015 • 3082
Multiscale Simulation of Plasticity in bcc Metals
Weygand, D. and Mrovec, M. and Hochrainer, T. and Gumbsch, P.
ANNUAL REVIEW OF MATERIALS RESEARCH. Volume: 45 (2015) - 2015 • 3081
Guided mass spectrum labelling in atom probe tomography
Haley, D. and Choi, P. and Raabe, D.
ULTRAMICROSCOPY. Volume: 159 (2015) - 2015 • 3080
Deformation mechanism of ω-enriched Ti-Nb-based gum metal: Dislocation channeling and deformation induced ω-β transformation
Lai, M.J. and Tasan, C.C. and Raabe, D.
ACTA MATERIALIA. Volume: 100 (2015) - 2015 • 3079
Role of biaxial strain and microscopic ordering for structural and electronic properties of InxGa1-x N
Cui, Y. and Lee, S. and Freysoldt, C. and Neugebauer, J.
PHYSICAL REVIEW B - CONDENSED MATTER AND MATERIALS PHYSICS. Volume: 92 (2015) - 2015 • 3078
Origin of shear induced β to ω transition in Ti-Nb-based alloys
Lai, M.J. and Tasan, C.C. and Zhang, J. and Grabowski, B. and Huang, L.F. and Raabe, D.
ACTA MATERIALIA. Volume: 92 (2015) - 2015 • 3077
Peeling by Nanomechanical Forces: A Route to Selective Creation of Surface Structures
Seema, P. and Behler, J. and Marx, D.
PHYSICAL REVIEW LETTERS. Volume: 115 (2015) - 2015 • 3076
Hydrogen diffusion and segregation in α iron ∑ 3 (111) grain boundaries
Hamza, M. and Hatem, T.M. and Raabe, D. and El-Awady, J.A.
ASME INTERNATIONAL MECHANICAL ENGINEERING CONGRESS AND EXPOSITION, PROCEEDINGS (IMECE). Volume: 9-2015 (2015) - 2015 • 3075
Emulsion soft templating of carbide-derived carbon nanospheres with controllable porosity for capacitive electrochemical energy storage
Oschatz, M. and Zeiger, M. and Jäckel, N. and Strubel, P. and Borchardt, L. and Reinhold, R. and Nickel, W. and Eckert, J. and Presser, V. and Kaskel, S.
JOURNAL OF MATERIALS CHEMISTRY A. Volume: 3 (2015) - 2015 • 3074
Comparison of virtual arrays for MIMO radar applications based on hexagonal configurations
Dahl, C. and Rolfes, I. and Vogt, M.
2015 EUROPEAN RADAR CONFERENCE, EURAD 2015 - PROCEEDINGS. Volume: (2015) - 2015 • 3073
A double barrier memristive device
Hansen, M. and Ziegler, M. and Kolberg, L. and Soni, R. and Dirkmann, S. and Mussenbrock, T. and Kohlstedt, H.
SCIENTIFIC REPORTS. Volume: 5 (2015) - 2015 • 3072
Nanoporous Carbide-Derived Carbons as Electrode Materials in Electrochemical Double-Layer Capacitors
Oschatz, M. and Borchardt, L. and Hao, G. and Kaskel, S.
NANOCARBONS FOR ADVANCED ENERGY STORAGE. Volume: 1 (2015) - 2015 • 3071
A two-dimensional radar simulator for level measurement of bulk material in silos
Dahl, C. and Rolfes, I. and Vogt, M.
2015 GERMAN MICROWAVE CONFERENCE, GEMIC 2015. Volume: (2015) - 2015 • 3070
Numerical investigation of the mixing of non-spherical particles in fluidized beds and during pneumatic conveying
Oschmann, T. and Vollmari, K. and Kruggel-Emden, H. and Wirtz, S.
PROCEDIA ENGINEERING. Volume: 102 (2015) - 2015 • 3069
Atomistically enabled nonsingular anisotropic elastic representation of near-core dislocation stress fields in α -iron
Seif, D. and Po, G. and Mrovec, M. and Lazar, M. and Elsässer, C. and Gumbsch, P.
PHYSICAL REVIEW B - CONDENSED MATTER AND MATERIALS PHYSICS. Volume: 91 (2015) - 2015 • 3068
Nanostructure and mechanical behavior of metastable Cu-Cr thin films grown by molecular beam epitaxy
Harzer, T.P. and Djaziri, S. and Raghavan, R. and Dehm, G.
ACTA MATERIALIA. Volume: 83 (2015) - 2015 • 3067
The effect of the driving frequency on the confinement of beam electrons and plasma density in low-pressure capacitive discharges
Wilczek, S. and Trieschmann, J. and Schulze, J. and Schuengel, E. and Brinkmann, R.P. and Derzsi, A. and Korolov, I. and Donkó, Z. and Mussenbrock, T.
PLASMA SOURCES SCIENCE AND TECHNOLOGY. Volume: 24 (2015) - 2015 • 3066
Texture evolution in deformed AZ31 magnesium sheets: Experiments and phase-field study
Darvishi Kamachali, R. and Kim, S.-J. and Steinbach, I.
COMPUTATIONAL MATERIALS SCIENCE. Volume: 104 (2015) - 2015 • 3065
Electrochemical communication between electrodes and rhodobacter capsulatus grown in different metabolic modes
Hasan, K. and Reddy, K.V.R. and Eßmann, V. and Górecki, K. and Conghaile, P.O. and Schuhmann, W. and Leech, D. and Hägerhäll, C. and Gorton, L.
ELECTROANALYSIS. Volume: 27 (2015) - 2015 • 3064
Electrochemical detection of single E. coli bacteria labeled with silver nanoparticles
Sepunaru, L. and Tschulik, K. and Batchelor-McAuley, C. and Gavish, R. and Compton, R.G.
BIOMATERIALS SCIENCE. Volume: 3 (2015) - 2015 • 3063
Geometrical grounds of mean field solutions for normal grain growth
Darvishi Kamachali, R. and Abbondandolo, A. and Siburg, K.F. and Steinbach, I.
ACTA MATERIALIA. Volume: 90 (2015) - 2015 • 3062
Processing of NiTi shape memory sheets - Microstructural heterogeneity and evolution of texture
Laplanche, G. and Kazuch, A. and Eggeler, G.
JOURNAL OF ALLOYS AND COMPOUNDS. Volume: 651 (2015) - 2015 • 3061
Influence of Alloying Elements, Heat Treatment, and Temperature on the Thermal Conductivity of Heat Treatable Steels
Wilzer, J. and Küpferle, J. and Weber, S. and Theisen, W.
STEEL RESEARCH INTERNATIONAL. Volume: 86 (2015) - 2015 • 3060
Bimetallic Zn and Hf on silica catalysts for the conversion of ethanol to 1,3-butadiene
De Baerdemaeker, T. and Feyen, M. and Müller, U. and Yilmaz, B. and Xiao, F.-S. and Zhang, W. and Yokoi, T. and Bao, X. and Gies, H. and De Vos, D.E.
ACS CATALYSIS. Volume: 5 (2015) - 2015 • 3059
Electromagnetic characterization of fluid vortices by means of three-dimensional field simulations
Hattenhorst, B. and Baer, C. and Musch, T. and Schulz, C. and Rolfes, I.
EUROPEAN MICROWAVE WEEK 2015: "FREEDOM THROUGH MICROWAVES", EUMW 2015 - CONFERENCE PROCEEDINGS; 2015 45TH EUROPEAN MICROWAVE CONFERENCE PROCEEDINGS, EUMC. Volume: (2015) - 2015 • 3058
Microstructural evolution of a CoCrFeMnNi high-entropy alloy after swaging and annealing
Laplanche, G. and Horst, O. and Otto, F. and Eggeler, G. and George, E.P.
JOURNAL OF ALLOYS AND COMPOUNDS. Volume: 647 (2015) - 2015 • 3057
Fast probe of local electronic states in nanostructures utilizing a single-lead quantum dot
Otsuka, T. and Amaha, S. and Nakajima, T. and Delbecq, M.R. and Yoneda, J. and Takeda, K. and Sugawara, R. and Allison, G. and Ludwig, Ar. and Wieck, A.D. and Tarucha, S.
SCIENTIFIC REPORTS. Volume: 5 (2015) - 2015 • 3056
Thermal conductivity of advanced TiC reinforced metal matrix composites for polymer processing applications
Wilzer, J. and Windmann, M. and Weber, S. and Hill, H. and Bennekom, A.V. and Theisen, W.
JOURNAL OF COMPOSITE MATERIALS. Volume: 49 (2015) - 2015 • 3055
From layered zeolite precursors to zeolites with a three-dimensional porosity: Textural and structural modifications through alkaline treatment
De Baerdemaeker, T. and Feyen, M. and Vanbergen, T. and Müller, U. and Yilmaz, B. and Xiao, F.-S. and Zhang, W. and Yokoi, T. and Bao, X. and De Vos, D.E. and Gies, H.
CHEMISTRY OF MATERIALS. Volume: 27 (2015) - 2015 • 3054
Microwave sensor concept for the detection of gas inclusions inside microfluidic channels
Hattenhorst, B. and Theissen, H. and Schulz, C. and Rolfes, I. and Baer, C. and Musch, T.
2015 IEEE MTT-S INTERNATIONAL MICROWAVE WORKSHOP SERIES ON RF AND WIRELESS TECHNOLOGIES FOR BIOMEDICAL AND HEALTHCARE APPLICATIONS, IMWS-BIO 2015 - PROCEEDINGS. Volume: (2015) - 2015 • 3053
Micro-tension study of miniaturized cu lines at variable temperatures
Wimmer, A. and Heinz, W. and Leitner, A. and Detzel, T. and Robl, W. and Kirchlechner, C. and Dehm, G.
ACTA MATERIALIA. Volume: 92 (2015) - 2015 • 3052
Nuclear spin polarization in the electron spin-flip Raman scattering of singly charged (In,Ga)As/GaAs quantum dots
Debus, J. and Kudlacik, D. and Sapega, V.F. and Dunker, D. and Bohn, P. and Paßmann, F. and Braukmann, D. and Rautert, J. and Yakovlev, D.R. and Reuter, D. and Wieck, A.D. and Bayer, M.
PHYSICAL REVIEW B - CONDENSED MATTER AND MATERIALS PHYSICS. Volume: 92 (2015) - 2015 • 3051
Spoke transitions in HiPIMS discharges
Hecimovic, A. and Schulz-von der Gathen, V. and Boke, M. and von Keudell, A. and Winter, J.
PLASMA SOURCES SCIENCE & TECHNOLOGY. Volume: 24 (2015) - 2015 • 3050
Suppression of twinning and phase transformation in an ultrafine grained 2 GPa strong metastable austenitic steel: Experiment and simulation
Shen, Y.F. and Jia, N. and Wang, Y.D. and Sun, X. and Zuo, L. and Raabe, D.
ACTA MATERIALIA. Volume: 97 (2015) - 2015 • 3049
Cyclic bending experiments on free-standing Cu micron lines observed by electron backscatter diffraction
Wimmer, A. and Heinz, W. and Detzel, T. and Robl, W. and Nellessen, M. and Kirchlechner, C. and Dehm, G.
ACTA MATERIALIA. Volume: 83 (2015) - 2015 • 3048
Effects of retained austenite volume fraction, morphology, and carbon content on strength and ductility of nanostructured TRIP-assisted steels
Shen, Y.F. and Qiu, L.N. and Sun, X. and Zuo, L. and Liaw, P.K. and Raabe, D.
MATERIALS SCIENCE AND ENGINEERING A. Volume: 636 (2015) - 2015 • 3047
Consecutive mechanism in the diffusion of D2 O on a NaCl(100) bilayer
Heidorn, S.-C. and Bertram, C. and Cabrera-Sanfelix, P. and Morgenstern, K.
ACS NANO. Volume: 9 (2015) - 2015 • 3046
Preparation and sintering behaviour of La5.4WO12-δ asymmetric membranes with optimised microstructure for hydrogen separation
Deibert, W. and Ivanova, M. E. and Meulenberg, W. A. and Vassen, R. and Guillon, O.
JOURNAL OF MEMBRANE SCIENCE. Volume: 492 (2015) - 2015 • 3045
A Redox Hydrogel Protects the O2-Sensitive [FeFe]-Hydrogenase from Chlamydomonas reinhardtii from Oxidative Damage
Oughli, A.A. and Conzuelo, F. and Winkler, M. and Happe, T. and Lubitz, W. and Schuhmann, W. and Rüdiger, O. and Plumeré, N.
ANGEWANDTE CHEMIE - INTERNATIONAL EDITION. Volume: 54 (2015) - 2015 • 3044
Multiferroic Vacancies at Ferroelectric PbTiO3 Surfaces
Shimada, T. and Wang, J. and Araki, Y. and Mrovec, M. and Elsässer, C. and Kitamura, T.
PHYSICAL REVIEW LETTERS. Volume: 115 (2015) - 2015 • 3043
Dynamic strain aging studied at the atomic scale
Aboulfadl, H. and Deges, J. and Choi, P. and Raabe, D.
ACTA MATERIALIA. Volume: 86 (2015) - 2015 • 3042
Multiferroic grain boundaries in oxygen-deficient ferroelectric lead titanate
Shimada, T. and Wang, J. and Ueda, T. and Uratani, Y. and Arisue, K. and Mrovec, M. and Elsä Sser, C. and Kitamura, T.
NANO LETTERS. Volume: 15 (2015) - 2015 • 3041
Influence of microstructure on macroscopic elastic properties and thermal expansion of nickel-base superalloys ERBO/1 and LEK94
Demtröder, K. and Eggeler, G. and Schreuer, J.
MATERIALWISSENSCHAFT UND WERKSTOFFTECHNIK. Volume: 46 (2015) - 2015 • 3040
Aging in amorphous solids: A study of the first-passage time and persistence time distributions
Siboni, N.H. and Raabe, D. and Varnik, F.
EPL. Volume: 111 (2015) - 2015 • 3039
Trimodal hierarchical carbide-derived carbon monoliths from steam- and CO2-activated wood templates for high rate lithium sulfur batteries
Adam, M. and Strubel, P. and Borchardt, L. and Althues, H. and Dörfler, S. and Kaskel, S.
JOURNAL OF MATERIALS CHEMISTRY A. Volume: 3 (2015) - 2015 • 3038
Inhibition of interfacial oxidative degradation during SiOx plasma polymer barrier film deposition on model organic substrates
Ozkaya, B. and Mitschker, F. and Ozcan, O. and Awakowicz, P. and Grundmeier, G.
PLASMA PROCESSES AND POLYMERS. Volume: 12 (2015) - 2015 • 3037
Quantitative phase imaging by wide field lensless digital holographic microscope
Adinda-Ougba, A. and Koukourakis, N. and Essaidi, A. and Gerhardt, N.C. and Hofmann, M.R.
PROCEEDINGS OF SPIE - THE INTERNATIONAL SOCIETY FOR OPTICAL ENGINEERING. Volume: 9529 (2015) - 2015 • 3036
Design of a twinning-induced plasticity high entropy alloy
Deng, Y. and Tasan, C.C. and Pradeep, K.G. and Springer, H. and Kostka, A. and Raabe, D.
ACTA MATERIALIA. Volume: 94 (2015) - 2015 • 3035
Influence of initial microstructure on thermomechanical fatigue behavior of Cu films on substrates
Heinz, W. and Robl, W. and Dehm, G.
MICROELECTRONIC ENGINEERING. Volume: 137 (2015) - 2015 • 3034
Compact low-cost lensless digital holographic microscope for topographic measurements of microstructures in reflection geometry
Adinda-Ougba, A. and Kabir, B. and Koukourakis, N. and Mitschker, F. and Gerhardt, N.C. and Hofmann, M.R.
PROCEEDINGS OF SPIE - THE INTERNATIONAL SOCIETY FOR OPTICAL ENGINEERING. Volume: 9628 (2015) - 2015 • 3033
Pseudomorphic Generation of Supported Catalysts for Glycerol Oxidation
Deng, X. and Dodekatos, G. and Pupovac, K. and Weidenthaler, C. and Schmidt, W. and Schüth, F. and Tüysüz, H.
CHEMCATCHEM. Volume: 7 (2015) - 2015 • 3032
Ultrawide electrical tuning of light matter interaction in a high electron mobility transistor structure
Pal, S. and Nong, H. and Markmann, S. and Kukharchyk, N. and Valentin, S.R. and Scholz, S. and Ludwig, Ar. and Bock, C. and Kunze, U. and Wieck, A.D. and Jukam, N.
SCIENTIFIC REPORTS. Volume: 5 (2015) - 2015 • 3031
Strong coupling of intersubband resonance in a single triangular well to a THz metamaterial
Pal, S. and Nongt, H. and Markmann, S. and Kukharchyk, N. and Valentin, S.R. and Scholz, S. and Ludwig, Ar. and Bock, C. and Kunze, U. and Wieck, A.D. and Jukam, N.
IRMMW-THZ 2015 - 40TH INTERNATIONAL CONFERENCE ON INFRARED, MILLIMETER, AND TERAHERTZ WAVES. Volume: (2015) - 2015 • 3030
Designing dose-finding studies with an active control for exponential families
Dette, H. and Kettelhake, K. and Bretz, F.
BIOMETRIKA. Volume: 102 (2015) - 2015 • 3029
Solidification and phase formation of alloys in the hypoeutectic region of the Fe-C-B system
Lentz, J. and Röttger, A. and Theisen, W.
ACTA MATERIALIA. Volume: 99 (2015) - 2015 • 3028
Density-chopped far-infrared transmission spectroscopy to probe subband-Landau splittings and tune intersubband transitions
Pal, S. and Nong, H. and Valentin, S.R. and Kukharchyk, N. and Ludwig, Ar. and Jukam, N. and Wieck, A.D.
CONFERENCE ON LASERS AND ELECTRO-OPTICS EUROPE - TECHNICAL DIGEST. Volume: 2015-August (2015) - 2015 • 3027
On the role of Re in the stress and temperature dependence of creep of Ni-base single crystal superalloys
Wollgramm, P. and Buck, H. and Neuking, K. and Parsa, A.B. and Schuwalow, S. and Rogal, J. and Drautz, R. and Eggeler, G.
MATERIALS SCIENCE AND ENGINEERING A. Volume: 628 (2015) - 2015 • 3026
Dose response signal detection under model uncertainty
Dette, H. and Titoff, S. and Volgushev, S. and Bretz, F.
BIOMETRICS. Volume: 71 (2015) - 2015 • 3025
Spectral domain optical coherence tomography for ex vivo brain tumor analysis
Lenz, M. and Krug, R. and Jaedicke, V. and Stroop, R. and Schmieder, K. and Hofmann, M.R.
PROGRESS IN BIOMEDICAL OPTICS AND IMAGING - PROCEEDINGS OF SPIE. Volume: 9541 (2015) - 2015 • 3024
Toward economical purification of styrene monomers: Eggshell Mo2C for front-end hydrogenation of phenylacetylene
Pang, M. and Shao, Z. F. and Wang, X. K. and Liang, C. H. and Xia, W.
AICHE JOURNAL. Volume: 61 (2015) - 2015 • 3023
Testing for additivity in nonparametric quantile regression
Dette, H. and Guhlich, M. and Neumeyer, N.
ANNALS OF THE INSTITUTE OF STATISTICAL MATHEMATICS. Volume: 67 (2015) - 2015 • 3022
Interplanar potential for tension-shear coupling at grain boundaries derived from ab initio calculations
Pang, X.Y. and Janisch, R. and Hartmaier, A.
MODELLING AND SIMULATION IN MATERIALS SCIENCE AND ENGINEERING. Volume: 24 (2015) - 2015 • 3021
Deformation-induced spatiotemporal fluctuation, evolution and localization of strain fields in a bulk metallic glass
Wu, Y. and Bei, H. and Wang, Y.L. and Lu, Z.P. and George, E.P. and Gao, Y.F.
INTERNATIONAL JOURNAL OF PLASTICITY. Volume: 71 (2015) - 2015 • 3020
Shape-Selection of Thermodynamically Stabilized Colloidal Pd and Pt Nanoparticles Controlled via Support Effects
Ahmadi, M. and Behafarid, F. and Holse, C. and Nielsen, J.H. and Roldan Cuenya, B.
JOURNAL OF PHYSICAL CHEMISTRY C. Volume: 119 (2015) - 2015 • 3019
Optimal designs for rational regression models
Dette, H. and Kiss, C.
JOURNAL OF STATISTICAL THEORY AND PRACTICE. Volume: 9 (2015) - 2015 • 3018
Ledges and grooves at γ/γ′ interfaces of single crystal superalloys
Parsa, A.B. and Wollgramm, P. and Buck, H. and Kostka, A. and Somsen, C. and Dlouhy, A. and Eggeler, G.
ACTA MATERIALIA. Volume: 90 (2015) - 2015 • 3017
A molecular switch based on the manipulation of 1,3-dichlorobenzene on Ge(001) between two adsorption sites by inelastic tunneling electrons
Wykrota, A. and Bazarnik, M. and Czajka, R. and Morgenstern, K.
PHYSICAL CHEMISTRY CHEMICAL PHYSICS. Volume: 17 (2015) - 2015 • 3016
Carbon Monoxide-Induced Stability and Atomic Segregation Phenomena in Shape-Selected Octahedral PtNi Nanoparticles
Ahmadi, M. and Cui, C. and Mistry, H. and Strasser, P. and Roldan Cuenya, B.
ACS NANO. Volume: 9 (2015) - 2015 • 3015
Of copulas, quantiles, ranks and spectra: An L1 -approach to spectral analysis
Dette, H. and Hallin, M. and Kley, T. and Volgushev, S.
BERNOULLI. Volume: 21 (2015) - 2015 • 3014
Advanced scale bridging microstructure analysis of single crystal Ni-base superalloys
Parsa, A.B. and Wollgramm, P. and Buck, H. and Somsen, C. and Kostka, A. and Povstugar, I. and Choi, P.-P. and Raabe, D. and Dlouhy, A. and Müller, J. and Spiecker, E. and Demtroder, K. and Schreuer, J. and Neuking, K. and Eggeler, G.
ADVANCED ENGINEERING MATERIALS. Volume: 17 (2015) - 2015 • 3013
All-Optical Preparation of Coherent Dark States of a Single Rare Earth Ion Spin in a Crystal
Xia, K. and Kolesov, R. and Wang, Y. and Siyushev, P. and Reuter, R. and Kornher, T. and Kukharchyk, N. and Wieck, A.D. and Villa, B. and Yang, S. and Wrachtrup, J.
PHYSICAL REVIEW LETTERS. Volume: 115 (2015) - 2014 • 3012
Enhanced Quantum Confined Stark Effect in a mesoporous hybrid multifunctional system
Gogoi, M. and Deb, P. and Sen, D. and Mazumder, S. and Kostka, A.
SOLID STATE COMMUNICATIONS. Volume: 187 (2014) - 2014 • 3011
Crossover from spin waves to diffusive spin excitations in underdoped Ba(Fe1-xCox)2As2
Tucker, G.S. and Fernandes, R.M. and Pratt, D.K. and Thaler, A. and Ni, N. and Marty, K. and Christianson, A.D. and Lumsden, M.D. and Sales, B.C. and Sefat, A.S. and Bud'Ko, S.L. and Canfield, P.C. and Kreyssig, A. and Goldman, A.I. and McQueeney, R.J.
PHYSICAL REVIEW B - CONDENSED MATTER AND MATERIALS PHYSICS. Volume: 89 (2014) - 2014 • 3010
Semi-contact measurements of three-dimensional surfaces utilizing a resonant uniaxial microprobe
Goj, B. and Dressler, L. and Hoffmann, M.
MEASUREMENT SCIENCE AND TECHNOLOGY. Volume: 25 (2014) - 2014 • 3009
Single photoelectron detection after selective excitation of electron heavy-hole and electron light-hole pairs in double quantum dots
Morimoto, K. and Fujita, T. and Allison, G. and Teraoka, S. and Larsson, M. and Kiyama, H. and Haffouz, S. and Austing, D.G. and Ludwig, Ar. and Wieck, A.D. and Oiwa, A. and Tarucha, S.
PHYSICAL REVIEW B - CONDENSED MATTER AND MATERIALS PHYSICS. Volume: 90 (2014) - 2014 • 3008
A novel homogenization method for phase field approaches based on partial rank-one relaxation
Mosler, J. and Shchyglo, O. and Montazer Hojjat, H.
JOURNAL OF THE MECHANICS AND PHYSICS OF SOLIDS. Volume: 68 (2014) - 2014 • 3007
Oxygen reduction at a Cu-modified Pt(111) model electrocatalyst in contact with nafion polymer
Tymoczko, J. and Calle-Vallejo, F. and Colic, V. and Koper, M.T.M. and Schuhmann, W. and Bandarenka, A.S.
ACS CATALYSIS. Volume: 4 (2014) - 2014 • 3006
Adherence of human mesenchymal stem cells on Ti and TiO2 nano-columnar surfaces fabricated by glancing angle sputter deposition
Motemani, Y. and Greulich, C. and Khare, C. and Lopian, M. and Buenconsejo, P.J.S. and Schildhauer, T.A. and Ludwig, Al. and Köller, M.
APPLIED SURFACE SCIENCE. Volume: 292 (2014) - 2014 • 3005
Position of Cu atoms at the Pt(111) electrode surfaces controls electrosorption of (H)SO4 (2)- from H2SO4 electrolytes
Tymoczko, J. and Schuhmann, W. and Bandarenka, A.S.
CHEMELECTROCHEM. Volume: 1 (2014) - 2014 • 3004
Determining the stability and activation energy of Si acceptors in AlGaAs using quantum interference in an open hole quantum dot
Carrad, D.J. and Burke, A.M. and Klochan, O. and See, A.M. and Hamilton, A.R. and Rai, A. and Reuter, D. and Wieck, A.D. and Micolich, A.P.
PHYSICAL REVIEW B - CONDENSED MATTER AND MATERIALS PHYSICS. Volume: 89 (2014) - 2014 • 3003
Ptychographic X-ray Microscopy with the vacuum imaging apparatus HORST
Gorniak, T. and Rosenhahn, A.
ZEITSCHRIFT FUR PHYSIKALISCHE CHEMIE. Volume: 228 (2014) - 2014 • 3002
High-Temperature Shape Memory Effect in Ti-Ta Thin Films Sputter Deposited at Room Temperature
Motemani, Y. and Buenconsejo, P.J.S. and Craciunescu, C. and Ludwig, Al.
ADVANCED MATERIALS INTERFACES. Volume: 1 (2014) - 2014 • 3001
Electrical potential-assisted DNA hybridization. How to mitigate electrostatics for surface DNA hybridization
Tymoczko, J. and Schuhmann, W. and Gebala, M.
ACS APPLIED MATERIALS AND INTERFACES. Volume: 6 (2014) - 2014 • 3000
Nano-scale morphology of melanosomes revealed by small-angle X-ray scattering
Gorniak, T. and Haraszti, T. and Garamus, V.M. and Buck, A.R. and Senkbeil, T. and Priebe, M. and Hedberg-Buenz, A. and Koehn, D. and Salditt, T. and Grunze, M. and Anderson, M.G. and Rosenhahn, A.
PLOS ONE. Volume: 9 (2014) - 2014 • 2999
First approach for thermodynamic modelling of the high temperature oxidation behaviour of ternary γ'-strengthened Co-Al-W superalloys
Klein, L. and Zendegani, A. and Palumbo, M. and Fries, S.G. and Virtanen, S.
CORROSION SCIENCE. Volume: 89 (2014) - 2014 • 2998
Support and challenges to the melanosomal casing model based on nanoscale distribution of metals within iris melanosomes detected by X-ray fluorescence analysis
Gorniak, T. and Haraszti, T. and Suhonen, H. and Yang, Y. and Hedberg-Buenz, A. and Koehn, D. and Heine, R. and Grunze, M. and Rosenhahn, A. and Anderson, M.G.
PIGMENT CELL AND MELANOMA RESEARCH. Volume: 27 (2014) - 2014 • 2997
Fragile antiferromagnetism in the heavy-fermion compound YbBiPt
Ueland, B.G. and Kreyssig, A. and Prokeš, K. and Lynn, J.W. and Harriger, L.W. and Pratt, D.K. and Singh, D.K. and Heitmann, T.W. and Sauerbrei, S. and Saunders, S.M. and Mun, E.D. and Bud'Ko, S.L. and McQueeney, R.J. and Canfield, P.C. and Goldman, A.I.
PHYSICAL REVIEW B - CONDENSED MATTER AND MATERIALS PHYSICS. Volume: 89 (2014) - 2014 • 2996
Influence of formation and coarsening of the laves phase on the mechanical properties of heat-resistant ferritic steels
Klein, S. and Nabiran, N. and Weber, S. and Theisen, W.
STEEL RESEARCH INTERNATIONAL. Volume: 85 (2014) - 2014 • 2995
Generation of NiTi nanoparticles by femtosecond laser ablation in liquid
Chakif, M. and Essaidi, A. and Gurevich, E. and Ostendorf, A. and Prymak, O. and Epple, M.
JOURNAL OF MATERIALS ENGINEERING AND PERFORMANCE. Volume: 23 (2014) - 2014 • 2994
Immobilization of proteins in their physiological active state at functionalized thiol monolayers on ATR-germanium crystals
Schartner, J. and Gavriljuk, K. and Nabers, A. and Weide, P. and Muhler, M. and Gerwert, K. and Kötting, C.
CHEMBIOCHEM. Volume: 15 (2014) - 2014 • 2993
Impact of solvent mixture on iron nanoparticles generated by laser ablation
Chakif, M. and Prymak, O. and Slota, M. and Heintze, E. and Gurevich, E.L. and Esen, C. and Bogani, L. and Epple, M. and Ostendorf, A.
PROGRESS IN BIOMEDICAL OPTICS AND IMAGING - PROCEEDINGS OF SPIE. Volume: 8955 (2014) - 2014 • 2992
High-precision green densities of thick films and their correlation with powder, ink, and film properties
Mücke, R. and Büchler, O. and Menzler, N.H. and Lindl, B. and Vaßen, R. and Buchkremer, H.P.
JOURNAL OF THE EUROPEAN CERAMIC SOCIETY. Volume: 34 (2014) - 2014 • 2991
A molecular iron catalyst for the acceptorless dehydrogenation and hydrogenation of N-heterocycles
Chakraborty, S. and Brennessel, W.W. and Jones, W.D.
JOURNAL OF THE AMERICAN CHEMICAL SOCIETY. Volume: 136 (2014) - 2014 • 2990
New insights into SEI formation in lithium ion batteries: Inhomogeneous distribution of irreversible charge losses across graphite electrodes
Klink, S. and Weide, P. and Ventosa, E. and Muhler, M. and Schuhmann, W. and La Mantia, F.
ECS TRANSACTIONS. Volume: 62 (2014) - 2014 • 2989
Chemically induced fracturing in alkali feldspar
Scheidl, K.S. and Schaeffer, A.-K. and Petrishcheva, E. and Habler, G. and Fischer, F.D. and Schreuer, J. and Abart, R.
PHYSICS AND CHEMISTRY OF MINERALS. Volume: 41 (2014) - 2014 • 2988
Experimental investigation and numerical simulation of the mechanical and thermal behavior of a superelastic shape memory alloy beam during bending
Ullrich, J. and Schmidt, M. and Schütze, A. and Wieczorek, A. and Frenzel, J. and Eggeler, G. and Seelecke, S.
ASME 2014 CONFERENCE ON SMART MATERIALS, ADAPTIVE STRUCTURES AND INTELLIGENT SYSTEMS, SMASIS 2014. Volume: 2 (2014) - 2014 • 2987
Iron-based catalysts for the hydrogenation of esters to alcohols
Chakraborty, S. and Dai, H. and Bhattacharya, P. and Fairweather, N.T. and Gibson, M.S. and Krause, J.A. and Guan, H.
JOURNAL OF THE AMERICAN CHEMICAL SOCIETY. Volume: 136 (2014) - 2014 • 2986
Vertical distribution of overpotentials and irreversible charge losses in lithium ion battery electrodes
Klink, S. and Schuhmann, W. and La Mantia, F.
CHEMSUSCHEM. Volume: 7 (2014) - 2014 • 2985
Fabrication of a Mo based high temperature TZM alloy by non-consumable arc melting technique
Chakraborty, S.P. and Krishnamurthy, N.
PROCEEDINGS - INTERNATIONAL SYMPOSIUM ON DISCHARGES AND ELECTRICAL INSULATION IN VACUUM, ISDEIV. Volume: (2014) - 2014 • 2984
Incommensurate modulations of relaxor ferroelectric Ca 0.24Ba0.76Nb2O6 (CBN24) and Ca 0.31Ba0.69Nb2O6 (CBN31)
Graetsch, H.A. and Pandey, C.S. and Schreuer, J. and Burianek, M. and Mühlberg, M.
ACTA CRYSTALLOGRAPHICA SECTION B: STRUCTURAL SCIENCE, CRYSTAL ENGINEERING AND MATERIALS. Volume: 70 (2014) - 2014 • 2983
Strain response of thermal barrier coatings captured under extreme engine environments through synchrotron X-ray diffraction
Knipe, K. and Manero II, A. and Siddiqui, S.F. and Meid, C. and Wischek, J. and Okasinski, J. and Almer, J. and Karlsson, A.M. and Bartsch, M. and Raghavan, S.
NATURE COMMUNICATIONS. Volume: 5 (2014) - 2014 • 2982
Construction of statistically similar representative volume elements - Comparative study regarding different statistical descriptors
Scheunemann, L. and Schröder, J. and Balzani, D. and Brands, D.
PROCEDIA ENGINEERING. Volume: 81 (2014) - 2014 • 2981
Synchrotron XRD measurements mapping internal strains of thermal barrier coatings during thermal gradient mechanical fatigue loading
Knipe, K. and Manero, A.C., II and Sofronsky, S. and Okasinski, J. and Almer, J. and Wischek, J. and Meid, C. and Karlsson, A. and Bartsch, M. and Raghavan, S., Prof.
PROCEEDINGS OF THE ASME TURBO EXPO. Volume: 6 (2014) - 2014 • 2980
Model of nonadiabatic-to-adiabatic dynamical quantum phase transition in photoexcited systems
Chang, J. and Eremin, I. and Zhao, J.
PHYSICAL REVIEW B - CONDENSED MATTER AND MATERIALS PHYSICS. Volume: 90 (2014) - 2014 • 2979
The modeling scheme to evaluate the influence of microstructure features on microcrack formation of DP-steel: The artificial microstructure model and its application to predict the strain hardening behavior
Vajragupta, N. and Wechsuwanmanee, P. and Lian, J. and Sharaf, M. and Münstermann, S. and Ma, A. and Hartmaier, A. and Bleck, W.
COMPUTATIONAL MATERIALS SCIENCE. Volume: 94 (2014) - 2014 • 2978
Combustion of lithium particles: Optical measurement methodology and initial results
Schiemann, M. and Fischer, P. and Scherer, V. and Schmid, G. and Taroata, D.
CHEMICAL ENGINEERING AND TECHNOLOGY. Volume: 37 (2014) - 2014 • 2977
Influence of chemical composition and physical structure on normal radiant emittance characteristics of ash deposits
Greffrath, F. and Gorewoda, J. and Schiemann, M. and Scherer, V.
FUEL. Volume: 134 (2014) - 2014 • 2976
Infrared emitting nanostructures for highly efficient microhotplates
Müller, L. and Käpplinger, I. and Biermann, S. and Brode, W. and Hoffmann, M.
JOURNAL OF MICROMECHANICS AND MICROENGINEERING. Volume: 24 (2014) - 2014 • 2975
Determination of char combustion kinetics parameters: Comparison of point detector and imaging-based particle-sizing pyrometry
Schiemann, M. and Geier, M. and Shaddix, C.R. and Vorobiev, N. and Scherer, V.
REVIEW OF SCIENTIFIC INSTRUMENTS. Volume: 85 (2014) - 2014 • 2974
Ceramic materials for H2 transport membranes applicable for gas separation under coal-gasification-related conditions
van Holt, D. and Forster, E. and Ivanova, M.E. and Meulenberg, W.A. and Müller, M. and Baumann, S. and Vaßen, R.
JOURNAL OF THE EUROPEAN CERAMIC SOCIETY. Volume: 34 (2014) - 2014 • 2973
Manipulation of the nuclear spin ensemble in a quantum dot with chirped magnetic resonance pulses
Munsch, M. and Wüst, G. and Kuhlmann, A.V. and Xue, F. and Ludwig, Ar. and Reuter, D. and Wieck, A.D. and Poggio, M. and Warburton, R.J.
NATURE NANOTECHNOLOGY. Volume: 9 (2014) - 2014 • 2972
A small mode volume tunable microcavity: Development and characterization
Greuter, L. and Starosielec, S. and Najer, D. and Ludwig, Ar. and Duempelmann, L. and Rohner, D. and Warburton, R.J.
APPLIED PHYSICS LETTERS. Volume: 105 (2014) - 2014 • 2971
Correlations of plasticity in sheared glasses
Varnik, F. and Mandal, S. and Chikkadi, V. and Denisov, D. and Olsson, P. and Vågberg, D. and Raabe, D. and Schall, P.
PHYSICAL REVIEW E - STATISTICAL, NONLINEAR, AND SOFT MATTER PHYSICS. Volume: 89 (2014) - 2014 • 2970
Columnar-Structured Mg-Al-Spinel Thermal Barrier Coatings (TBCs) by Suspension Plasma Spraying (SPS)
Schlegel, N. and Ebert, S. and Mauer, G. and Vaßen, R.
JOURNAL OF THERMAL SPRAY TECHNOLOGY. Volume: 24 (2014) - 2014 • 2969
Generation of microfluidic flow using an optically assembled and magnetically driven microrotor
Köhler, J. and Ghadiri, R. and Ksouri, S.I. and Guo, Q. and Gurevich, E.L. and Ostendorf, A.
JOURNAL OF PHYSICS D: APPLIED PHYSICS. Volume: 47 (2014) - 2014 • 2968
The contact heat transfer in rotary drums in dependence on the particle size ratio
Nafsun, A.I. and Herz, F. and Specht, E. and Scherer, V. and Wirtz, S. and Komossa, H.
PROCEEDINGS OF THE 15TH INTERNATIONAL HEAT TRANSFER CONFERENCE, IHTC 2014. Volume: (2014) - 2014 • 2967
All-optical implementation of a dynamic decoupling protocol for hole spins in (In,Ga)As quantum dots
Varwig, S. and Evers, E. and Greilich, A. and Yakovlev, D.R. and Reuter, D. and Wieck, A.D. and Bayer, M.
PHYSICAL REVIEW B - CONDENSED MATTER AND MATERIALS PHYSICS. Volume: 90 (2014) - 2014 • 2966
Multi-component nanoporous platinum-ruthenium-copper-osmium-iridium alloy with enhanced electrocatalytic activity towards methanol oxidation and oxygen reduction
Chen, X. and Si, C. and Gao, Y. and Frenzel, J. and Sun, J. and Eggeler, G. and Zhang, Z.
JOURNAL OF POWER SOURCES. Volume: 273 (2014) - 2014 • 2965
Optical tweezers as manufacturing and characterization tool in microfluidics
Köhler, J. and Ghadiri, R. and Ksouri, S.I. and Gurevich, E.L. and Ostendorf, A.
PROCEEDINGS OF SPIE - THE INTERNATIONAL SOCIETY FOR OPTICAL ENGINEERING. Volume: 9164 (2014) - 2014 • 2964
High-temperature creep and oxidation behavior of Mo-Si-B alloys with high Ti contents
Schliephake, D. and Azim, M. and Von Klinski-Wetzel, K. and Gorr, B. and Christ, H.-J. and Bei, H. and George, E.P. and Heilmaier, M.
METALLURGICAL AND MATERIALS TRANSACTIONS A: PHYSICAL METALLURGY AND MATERIALS SCIENCE. Volume: 45 (2014) - 2014 • 2963
Excitation of complex spin dynamics patterns in a quantum-dot electron spin ensemble
Varwig, S. and Yugova, I.A. and René, A. and Kazimierczuk, T. and Greilich, A. and Yakovlev, D.R. and Reuter, D. and Wieck, A.D. and Bayer, M.
PHYSICAL REVIEW B - CONDENSED MATTER AND MATERIALS PHYSICS. Volume: 90 (2014) - 2014 • 2962
Investigation of ternary subsystems of superalloys by thin-film combinatorial synthesis and high-throughput analysis
König, D. and Pfetzing-Micklich, J. and Frenzel, J. and Ludwig, Al.
MATEC WEB OF CONFERENCES. Volume: 14 (2014) - 2014 • 2961
All-optical tomography of electron spins in (In,Ga)As quantum dots
Varwig, S. and René, A. and Economou, S.E. and Greilich, A. and Yakovlev, D.R. and Reuter, D. and Wieck, A.D. and Reinecke, T.L. and Bayer, M.
PHYSICAL REVIEW B - CONDENSED MATTER AND MATERIALS PHYSICS. Volume: 89 (2014) - 2014 • 2960
High-throughput fabrication of Au-Cu nanoparticle libraries by combinatorial sputtering in ionic liquids
König, D. and Richter, K. and Siegel, A. and Mudring, A.-V. and Ludwig, Al.
ADVANCED FUNCTIONAL MATERIALS. Volume: 24 (2014) - 2014 • 2959
Temperature dependent magnon-phonon coupling in bcc Fe from theory and experiment
Körmann, F. and Grabowski, B. and Dutta, B. and Hickel, T. and Mauger, L. and Fultz, B. and Neugebauer, J.
PHYSICAL REVIEW LETTERS. Volume: 113 (2014) - 2014 • 2958
Swimming behavior of Pseudomonas aeruginosa studied by holographic 3D tracking
Vater, S.M. and Weiße, S. and Maleschlijski, S. and Lotz, C. and Koschitzki, F. and Schwartz, T. and Obst, U. and Rosenhahn, A.
PLOS ONE. Volume: 9 (2014) - 2014 • 2957
Local visualization of catalytic activity at gas evolving electrodes using frequency-dependent scanning electrochemical microscopy
Chen, X. and Maljusch, A. and Rincón, R.A. and Battistel, A. and Bandarenka, A.S. and Schuhmann, W.
CHEMICAL COMMUNICATIONS. Volume: 50 (2014) - 2014 • 2956
Fluctuations and diffusion in sheared athermal suspensions of deformable particles
Gross, M. and Krüger, T. and Varnik, F.
EPL. Volume: 108 (2014) - 2014 • 2955
Lambda transitions in materials science: Recent advances in CALPHAD and first-principles modelling
Körmann, F. and Breidi, A.A.H. and Dudarev, S.L. and Dupin, N. and Ghosh, G. and Hickel, T. and Korzhavyi, P. and Muñoz, J.A. and Ohnuma, I.
PHYSICA STATUS SOLIDI (B) BASIC RESEARCH. Volume: 251 (2014) - 2014 • 2954
Narrow-band indoor localization in cognitive radio networks using compressed sensing
Nalobin, A. and Rolfes, I.
IEEE ANTENNAS AND PROPAGATION SOCIETY, AP-S INTERNATIONAL SYMPOSIUM (DIGEST). Volume: (2014) - 2014 • 2953
Rheology of dense suspensions of elastic capsules: Normal stresses, yield stress, jamming and confinement effects
Gross, M. and Krüger, T. and Varnik, F.
SOFT MATTER. Volume: 10 (2014) - 2014 • 2952
Narrowband direction-of-arrival estimation of near-field sources using compressed sensing
Nalobin, A. and Rolfes, I.
EUROPEAN MICROWAVE WEEK 2014: CONNECTING THE FUTURE, EUMW 2014 - CONFERENCE PROCEEDINGS; EUMC 2014: 44TH EUROPEAN MICROWAVE CONFERENCE. Volume: (2014) - 2014 • 2951
PQQ-sGDH bioelectrodes based on os-complex modified electrodeposition polymers and carbon nanotubes
Chen, X. and Shao, M. and Pöller, S. and Guschin, D. and Pinyou, P. and Schuhmann, W.
JOURNAL OF THE ELECTROCHEMICAL SOCIETY. Volume: 161 (2014) - 2014 • 2950
Spreading dynamics of nanodrops:A lattice boltzmann study
Gross, M. and Varnik, F.
INTERNATIONAL JOURNAL OF MODERN PHYSICS C. Volume: 25 (2014) - 2014 • 2949
Statistical approach for a continuum description of damage evolution in soft collagenous tissues
Schmidt, T. and Balzani, D. and Holzapfel, G.A.
COMPUTER METHODS IN APPLIED MECHANICS AND ENGINEERING. Volume: 278 (2014) - 2014 • 2948
Interaction of cobalt nanoparticles with oxygen- and nitrogen- functionalized carbon nanotubes and impact on nitrobenzene hydrogenation catalysis
Chen, P. and Yang, F. and Kostka, A. and Xia, W.
ACS CATALYSIS. Volume: 4 (2014) - 2014 • 2947
Modeling supra-physiological loading of human arterial walls-damage, anisotropy and component-specific behavior
Schmidt, T. and Schriefl, A.J. and Balzani, D. and Holzapfel, G.A.
BIOMEDIZINISCHE TECHNIK. Volume: 59 (2014) - 2014 • 2946
Low temperature Hydrogen Reduction of High Surface Area Anatase and Anatase/β-TiO2 for High-Charging-Rate Batteries
Ventosa, E. and Tymoczko, A. and Xie, K. and Xia, W. and Muhler, M. and Schuhmann, W.
CHEMSUSCHEM. Volume: 7 (2014) - 2014 • 2945
Optimizing the magnetocaloric effect in Ni-Mn-Sn by substitution: A first-principles study
Grünebohm, A. and Comtesse, D. and Hucht, A. and Gruner, M.E. and Maslovskaya, A. and Entel, P.
IEEE TRANSACTIONS ON MAGNETICS. Volume: 50 (2014) - 2014 • 2944
Transversal bed motion in rotating drums using spherical particles: Comparison of experiments with DEM simulations
Komossa, H. and Wirtz, S. and Scherer, V. and Herz, F. and Specht, E.
POWDER TECHNOLOGY. Volume: 264 (2014) - 2014 • 2943
Negatively charged ions on Mg(0001) surfaces: Appearance and origin of attractive adsorbate-adsorbate interactions
Cheng, S.-T. and Todorova, M. and Freysoldt, C. and Neugebauer, J.
PHYSICAL REVIEW LETTERS. Volume: 113 (2014) - 2014 • 2942
Low-temperature oxidation of carbon monoxide with gold(III) ions supported on titanium oxide
Grünert, W. and Großmann, D. and Noei, H. and Pohl, M.-M. and Sinev, I. and De Toni, A. and Wang, Y. and Muhler, M.
ANGEWANDTE CHEMIE - INTERNATIONAL EDITION. Volume: 53 (2014) - 2014 • 2941
Ab initio study of H-vacancy interactions in fcc metals: Implications for the formation of superabundant vacancies
Nazarov, R. and Hickel, T. and Neugebauer, J.
PHYSICAL REVIEW B - CONDENSED MATTER AND MATERIALS PHYSICS. Volume: 89 (2014) - 2014 • 2940
Redox potentials and acidity constants from density functional theory based molecular dynamics
Cheng, J. and Liu, X. and VandeVondele, J. and Sulpizi, M. and Sprik, M.
ACCOUNTS OF CHEMICAL RESEARCH. Volume: 47 (2014) - 2014 • 2939
How different characterization techniques elucidate the nature of the gold species in a polycrystalline Au/TiO2 catalyst
Grünert, W. and Großmann, D. and Noei, H. and Pohl, M.-M. and Sinev, I. and De Toni, A. and Wang, Y. and Muhler, M.
CHEMIE-INGENIEUR-TECHNIK. Volume: 86 (2014) - 2014 • 2938
Magnetic and transport properties of i-R-Cd icosahedral quasicrystals (R=Y,Gd-Tm)
Kong, T. and Bud'Ko, S.L. and Jesche, A. and McArthur, J. and Kreyssig, A. and Goldman, A.I. and Canfield, P.C.
PHYSICAL REVIEW B - CONDENSED MATTER AND MATERIALS PHYSICS. Volume: 90 (2014) - 2014 • 2937
Constant-distance mode SECM as a tool to Visualize local electrocatalytic activity of oxygen reduction catalysts
Nebel, M. and Erichsen, T. and Schuhmann, W.
BEILSTEIN JOURNAL OF NANOTECHNOLOGY. Volume: 5 (2014) - 2014 • 2936
Effect of nitrogen doping on the reducibility, activity and selectivity of carbon nanotube-supported iron catalysts applied in CO2 hydrogenation
Chew, L.M. and Kangvansura, P. and Ruland, H. and Schulte, H.J. and Somsen, C. and Xia, W. and Eggeler, G. and Worayingyong, A. and Muhler, M.
APPLIED CATALYSIS A: GENERAL. Volume: 482 (2014) - 2014 • 2935
Microelectrochemical visualization of oxygen consumption of single living cells
Nebel, M. and Grützke, S. and Diab, N. and Schulte, A. and Schuhmann, W.
FARADAY DISCUSSIONS. Volume: 164 (2014) - 2014 • 2934
CO 2 hydrogenation to hydrocarbons over iron nanoparticles supported on oxygen-functionalized carbon nanotubes
Chew, L.M. and Ruland, H. and Schulte, H.J. and Xia, W. and Muhler, M.
JOURNAL OF CHEMICAL SCIENCES. Volume: 126 (2014) - 2014 • 2933
Biofuel-Cell Cathodes Based on Bilirubin Oxidase Immobilized through Organic Linkers on 3D Hierarchically Structured Carbon Electrodes
Vivekananthan, J. and Rincón, R.A. and Kuznetsov, V. and Pöller, S. and Schuhmann, W.
CHEMELECTROCHEM. Volume: 1 (2014) - 2014 • 2932
Targeted manipulation of metal-organic frameworks to direct sorption properties
Schneemann, A. and Henke, S. and Schwedler, I. and Fischer, R.A.
CHEMPHYSCHEM. Volume: 15 (2014) - 2014 • 2931
Magnetic vortex observation in FeCo nanowires by quantitative magnetic force microscopy
Vock, S. and Hengst, C. and Wolf, M. and Tschulik, K. and Uhlemann, M. and Sasvári, Z. and Makarov, D. and Schmidt, O.G. and Schultz, L. and Neu, V.
APPLIED PHYSICS LETTERS. Volume: 105 (2014) - 2014 • 2930
Electron waveguide interferometers for spin-dependent transport experiments
Chiatti, O. and Buchholz, S. and Kunze, U. and Reuter, D. and Wieck, A. and Fischer, S.
PHYSICA STATUS SOLIDI (B) BASIC RESEARCH. Volume: 251 (2014) - 2014 • 2929
Ordered mesoporous Cu-Ce-O catalysts for CO preferential oxidation in H2-rich gases: Influence of copper content and pretreatment conditions
Gu, D. and Jia, C.-J. and Bongard, H. and Spliethoff, B. and Weidenthaler, C. and Schmidt, W. and Schüth, F.
APPLIED CATALYSIS B: ENVIRONMENTAL. Volume: 152-153 (2014) - 2014 • 2928
Engineered electron-transfer chain in photosystem 1 based photocathodes outperforms electron-transfer rates in natural photosynthesis
Kothe, T. and Pöller, S. and Zhao, F. and Fortgang, P. and Rögner, M. and Schuhmann, W. and Plumeré, N.
CHEMISTRY (WEINHEIM AN DER BERGSTRASSE, GERMANY). Volume: 20 (2014) - 2014 • 2927
The influence of kinetics, mass transfer and catalyst deactivation on the growth rate of multiwalled carbon nanotubes from ethene on a cobalt-based catalyst
Voelskow, K. and Becker, M.J. and Xia, W. and Muhler, M. and Turek, T.
CHEMICAL ENGINEERING JOURNAL. Volume: 244 (2014) - 2014 • 2926
Coupled atomistic-continuum study of the effects of C atoms at α-Fe dislocation cores
Chockalingam, K. and Janisch, R. and Hartmaier, A.
MODELLING AND SIMULATION IN MATERIALS SCIENCE AND ENGINEERING. Volume: 22 (2014) - 2014 • 2925
Synthesis of non-siliceous mesoporous oxides
Gu, D. and Schüth, F.
CHEMICAL SOCIETY REVIEWS. Volume: 43 (2014) - 2014 • 2924
Large-scale synthesis and catalytic activity of nanoporous Cu-O system towards CO oxidation
Kou, T. and Si, C. and Gao, Y. and Frenzel, J. and Wang, H. and Yan, X. and Bai, Q. and Eggeler, G. and Zhang, Z.
RSC ADVANCES. Volume: 4 (2014) - 2014 • 2923
Micromechanical investigations and modelling of a Copper-Antimony-Alloy under creep conditions
Vöse, M. and Otto, F. and Fedelich, B. and Eggeler, G.
MECHANICS OF MATERIALS. Volume: 69 (2014) - 2014 • 2922
Comparative studies on the accumulation of strain and recovery ratio of Veriflex®, a shape-memory polymer for a high strain (∈m = 210%): Atomic force microscopic experiments
Chowdhury, A.M.S. and Schmidt, C. and Neuking, K. and Eggeler, G.
HIGH PERFORMANCE POLYMERS. Volume: 26 (2014) - 2014 • 2921
Anisotropic softening of magnetic excitations along the nodal direction in superconducting cuprates
Guarise, M. and Dalla Piazza, B. and Berger, H. and Giannini, E. and Schmitt, T. and Rønnow, H.M. and Sawatzky, G.A. and Van Den Brink, J. and Altenfeld, D. and Eremin, I. and Grioni, M.
NATURE COMMUNICATIONS. Volume: 5 (2014) - 2014 • 2920
Axial scanning in confocal microscopy employing adaptive lenses (CAL)
Koukourakis, N. and Finkeldey, M. and Stürmer, M. and Leithold, C. and Gerhardt, N.C. and Hofmann, M.R. and Wallrabe, U. and Czarske, J.W. and Fischer, A.
OPTICS EXPRESS. Volume: 22 (2014) - 2014 • 2919
Effects of axial scanning in confocal microscopy employing adaptive lenses (CAL)
Koukourakis, N. and Finkeldey, M. and Stürmer, M. and Gerhardt, N.C. and Wallrabe, U. and Hofmann, M.R. and Czarske, J.W. and Fischer, A.
PROCEEDINGS OF SPIE - THE INTERNATIONAL SOCIETY FOR OPTICAL ENGINEERING. Volume: 9132 (2014) - 2014 • 2918
Hydrogen-assisted decohesion and localized plasticity in dual-phase steel
Koyama, M. and Tasan, C.C. and Akiyama, E. and Tsuzaki, K. and Raabe, D.
ACTA MATERIALIA. Volume: 70 (2014) - 2014 • 2917
Local determination of the amount of integration of an atom into a crystal surface
Volgmann, K. and Gawronski, H. and Zaum, C. and Rusina, G.G. and Borisova, S.D. and Chulkov, E.V. and Morgenstern, K.
NATURE COMMUNICATIONS. Volume: 5 (2014) - 2014 • 2916
Intrinsic acidity of surface sites in calcium silicate hydrates and its implication to their electrokinetic properties
Churakov, S.V. and Labbez, C. and Pegado, L. and Sulpizi, M.
JOURNAL OF PHYSICAL CHEMISTRY C. Volume: 118 (2014) - 2014 • 2915
Hydrogen embrittlement associated with strain localization in a precipitation-hardened Fe-Mn-Al-C light weight austenitic steel
Koyama, M. and Springer, H. and Merzlikin, S.V. and Tsuzaki, K. and Akiyama, E. and Raabe, D.
INTERNATIONAL JOURNAL OF HYDROGEN ENERGY. Volume: 39 (2014) - 2014 • 2914
Timescales of disequilibrium melting in the crust: Constraints from modelling the distribution of multiple trace elements and a case study from the Lesser Himalayan rocks of Sikkim
Neogi, S. and Bolton, E.W. and Chakraborty, S.
CONTRIBUTIONS TO MINERALOGY AND PETROLOGY. Volume: 168 (2014) - 2014 • 2913
Censored quantile regression processes under dependence and penalization
Volgushev, S. and Wagener, J. and Dette, H.
ELECTRONIC JOURNAL OF STATISTICS. Volume: 8 (2014) - 2014 • 2912
Multifunctional, defect-engineered metal-organic frameworks with ruthenium centers: Sorption and catalytic properties
Kozachuk, O. and Luz, I. and Llabrés I Xamena, F.X. and Noei, H. and Kauer, M. and Albada, H.B. and Bloch, E.D. and Marler, B. and Wang, Y. and Muhler, M. and Fischer, R.A.
ANGEWANDTE CHEMIE - INTERNATIONAL EDITION. Volume: 53 (2014) - 2014 • 2911
User defined finite element for modeling and analysis of active piezoelectric shell structures
Nestorović, T. and Marinković, D. and Shabadi, S. and Trajkov, M.
MECCANICA. Volume: 49 (2014) - 2014 • 2910
Impact of Mn on the solution enthalpy of hydrogen in austenitic Fe-Mn alloys: A first-principles study
Von Appen, J. and Dronskowski, R. and Chakrabarty, A. and Hickel, T. and Spatschek, R. and Neugebauer, J.
JOURNAL OF COMPUTATIONAL CHEMISTRY. Volume: 35 (2014) - 2014 • 2909
The amorphous silica-liquid water interface studied by ab initio molecular dynamics (AIMD): Local organization in global disorder
Cimas, Á. and Tielens, F. and Sulpizi, M. and Gaigeot, M.-P. and Costa, D.
JOURNAL OF PHYSICS CONDENSED MATTER. Volume: 26 (2014) - 2014 • 2908
Ab initio structure determination of interlayer expanded zeolites by single crystal rotation electron diffraction
Guo, P. and Liu, L. and Yun, Y. and Su, J. and Wan, W. and Gies, H. and Zhang, H. and Xiao, F.-S. and Zou, X.
DALTON TRANSACTIONS. Volume: 43 (2014) - 2014 • 2907
Identification of parameters in nonlinear geotechnical models using extenden Kalman filter
Nestorović, T. and Nguyen, L.T. and Trajkov, M.
MATEC WEB OF CONFERENCES. Volume: 16 (2014) - 2014 • 2906
Comparison of in situ and ex situ methods for synthesis of two-photon polymerization polymer nanocomposites
Guo, Q. and Ghadiri, R. and Weigel, T. and Aumann, A. and Gurevich, E.L. and Esen, C. and Medenbach, O. and Cheng, W. and Chichkov, B. and Ostendorf, A.
POLYMERS. Volume: 6 (2014) - 2014 • 2905
Enhanced photocatalytic degradation rates at rutile TiO2 photocatalysts modified with redox co-catalysts
Neubert, S. and Pulisova, P. and Wiktor, C. and Weide, P. and Mei, B. and Guschin, D.A. and Fischer, R.A. and Muhler, M. and Beranek, R.
CATALYSIS TODAY. Volume: 230 (2014) - 2014 • 2904
Ex-situ preparation of high-conductive polymer/SWNTs nanocomposites for structure fabrication
Guo, Q. and Ghadiri, R. and Weigel, T. and Aumann, A. and Gurevich, E.L. and Esen, C. and Li, Y. and Cheng, W. and Chichkov, B. and Ostendorf, A.
PROCEEDINGS OF SPIE - THE INTERNATIONAL SOCIETY FOR OPTICAL ENGINEERING. Volume: 9277 (2014) - 2014 • 2903
Surface-modified TiO2 photocatalysts prepared by a photosynthetic route: Mechanism, enhancement, and limits
Neubert, S. and Ramakrishnan, A. and Strunk, J. and Shi, H. and Mei, B. and Wang, L. and Bledowski, M. and A. Guschin, D. and Kauer, M. and Wang, Y. and Muhler, M. and Beranek, R.
CHEMPLUSCHEM. Volume: 79 (2014) - 2014 • 2902
Shear-induced mixing governs codeformation of crystalline-amorphous nanolaminates
Guo, W. and Jägle, E.A. and Choi, P.-P. and Yao, J. and Kostka, A. and Schneider, J.M. and Raabe, D.
PHYSICAL REVIEW LETTERS. Volume: 113 (2014) - 2014 • 2901
Impact of process parameters on the laser-induced nanoparticle formation during keyhole welding under remote conditions
Scholz, T. and Dickmann, K. and Ostendorf, A.
PHYSICS PROCEDIA. Volume: 56 (2014) - 2014 • 2900
Intrinsic and extrinsic size effects in the deformation of amorphous CuZr/nanocrystalline Cu nanolaminates
Guo, W. and Jägle, E. and Yao, J. and Maier, V. and Korte-Kerzel, S. and Schneider, J.M. and Raabe, D.
ACTA MATERIALIA. Volume: 80 (2014) - 2014 • 2899
Dynamical behavior of laser-induced nanoparticles during remote processing
Scholz, T. and Dickmann, K. and Ostendorf, A.
PROCEEDINGS OF SPIE - THE INTERNATIONAL SOCIETY FOR OPTICAL ENGINEERING. Volume: 8963 (2014) - 2014 • 2898
The surface energy of single nanoparticles probed via anodic stripping voltammetry
Neumann, C.C.M. and Batchelor-McAuley, C. and Tschulik, K. and Toh, H.S. and Shumbul, P. and Pillay, J. and Tshikhudo, R. and Compton, R.G.
CHEMELECTROCHEM. Volume: 1 (2014) - 2014 • 2897
Nanosensors for the detection of hydrogen peroxide
Clausmeyer, J. and Actis, P. and López Córdoba, A. and Korchev, Y. and Schuhmann, W.
ELECTROCHEMISTRY COMMUNICATIONS. Volume: 40 (2014) - 2014 • 2896
High strength and ductile low density austenitic FeMnAlC steels: Simplex and alloys strengthened by nanoscale ordered carbides
Gutierrez-Urrutia, I. and Raabe, D.
MATERIALS SCIENCE AND TECHNOLOGY (UNITED KINGDOM). Volume: 30 (2014) - 2014 • 2895
Planar diffusion to macro disc electrodes—what electrode size is required for the Cottrell and Randles-Sevcik equations to apply quantitatively?
Ngamchuea, K. and Eloul, S. and Tschulik, K. and Compton, R.G.
JOURNAL OF SOLID STATE ELECTROCHEMISTRY. Volume: 18 (2014) - 2014 • 2894
Electrochemical patterning as a tool for fabricating biomolecule microarrays
Clausmeyer, J. and Schuhmann, W. and Plumeré, N.
TRAC - TRENDS IN ANALYTICAL CHEMISTRY. Volume: 58 (2014) - 2014 • 2893
Microstructure-magnetic property relations in grain-oriented electrical steels: Quantitative analysis of the sharpness of the Goss orientation
Gutierrez-Urrutia, I. and Böttcher, A. and Lahn, L. and Raabe, D.
JOURNAL OF MATERIALS SCIENCE. Volume: 49 (2014) - 2014 • 2892
Two-scale modeling of DP steel incorporating distributed properties inside micro-constituents
Schröder, J. and Gandhi, A. and Balzani, D.
PROCEDIA ENGINEERING. Volume: 81 (2014) - 2014 • 2891
Scanning droplet cell for chemoselective patterning through local electroactivation of protected quinone monolayers
Clausmeyer, J. and Henig, J. and Schuhmann, W. and Plumeré, N.
CHEMPHYSCHEM. Volume: 15 (2014) - 2014 • 2890
Cyclic degradation mechanisms in aged FeNiCoAlTa shape memory single crystals
Krooß, P. and Somsen, C. and Niendorf, T. and Schaper, M. and Karaman, I. and Chumlyakov, Y. and Eggeler, G. and Maier, H.J.
ACTA MATERIALIA. Volume: 79 (2014) - 2014 • 2889
Thermal cycling behavior of an aged FeNiCoAlTa single-crystal shape memory alloy
Krooß, P. and Holzweissig, M.J. and Niendorf, T. and Somsen, C. and Schaper, M. and Chumlyakov, Y.I. and Maier, H.J.
SCRIPTA MATERIALIA. Volume: 81 (2014) - 2014 • 2888
Atomistic study of the influence of lattice defects on the thermal conductivity of silicon
Wang, T. and Madsen, G.K.H. and Hartmaier, A.
MODELLING AND SIMULATION IN MATERIALS SCIENCE AND ENGINEERING. Volume: 22 (2014) - 2014 • 2887
Observation of quantum states without a semiclassical equivalence bound by a magnetic field gradient
Schüler, B. and Cerchez, M. and Xu, H. and Schluck, J. and Heinzel, T. and Reuter, D. and Wieck, A.D.
PHYSICAL REVIEW B - CONDENSED MATTER AND MATERIALS PHYSICS. Volume: 90 (2014) - 2014 • 2886
The effect of notches on the fatigue behavior in NiTi shape memory alloys
Wang, X.M. and Cao, W. and Deng, C.H. and Liu, H. and Pfetzing-Micklich, J. and Yue, Z.F.
MATERIALS SCIENCE AND ENGINEERING A. Volume: 610 (2014) - 2014 • 2885
Mechanocatalytic depolymerization of cellulose and raw biomass and downstream processing of the products
Schüth, F. and Rinaldi, R. and Meine, N. and Käldström, M. and Hilgert, J. and Rechulski, M.D.K.
CATALYSIS TODAY. Volume: 234 (2014) - 2014 • 2884
Smaller is less stable: Size effects on twinning vs. transformation of reverted austenite in TRIP-maraging steels
Wang, M.-M. and Tasan, C.C. and Ponge, D. and Kostka, A. and Raabe, D.
ACTA MATERIALIA. Volume: 79 (2014) - 2014 • 2883
First-principles calculation of the instability leading to giant inverse magnetocaloric effects
Comtesse, D. and Gruner, M.E. and Ogura, M. and Sokolovskiy, V.V. and Buchelnikov, V.D. and Grünebohm, A. and Arróyave, R. and Singh, N. and Gottschall, T. and Gutfleisch, O. and Chernenko, V.A. and Albertini, F. and Fähler, S. and Entel, P.
PHYSICAL REVIEW B - CONDENSED MATTER AND MATERIALS PHYSICS. Volume: 89 (2014) - 2014 • 2882
Numerical study of rope formation and dispersion of non-spherical particles during pneumatic conveying in a pipe bend
Kruggel-Emden, H. and Oschmann, T.
POWDER TECHNOLOGY. Volume: 268 (2014) - 2014 • 2881
Oxygen transport through supported Ba0.5Sr0.5Co 0.8Fe0.2O3-δ membranes
Niehoff, P. and Baumann, S. and Schulze-Küppers, F. and Bradley, R.S. and Shapiro, I. and Meulenberg, W.A. and Withers, P.J. and Vaßen, R.
SEPARATION AND PURIFICATION TECHNOLOGY. Volume: 121 (2014) - 2014 • 2880
Control of solid catalysts down to the atomic scale: Where is the limit?
Schüth, F.
ANGEWANDTE CHEMIE - INTERNATIONAL EDITION. Volume: 53 (2014) - 2014 • 2879
In situ observation of collective grain-scale mechanics in Mg and Mg-rare earth alloys
Wang, F. and Sandlöbes, S. and Diehl, M. and Sharma, L. and Roters, F. and Raabe, D.
ACTA MATERIALIA. Volume: 80 (2014) - 2014 • 2878
On the development of high quality NiTi shape memory and pseudoelastic parts by additive manufacturing
Haberland, C. and Elahinia, M. and Walker, J.M. and Meier, H. and Frenzel, J.
SMART MATERIALS AND STRUCTURES. Volume: 23 (2014) - 2014 • 2877
Analysis of Residence Time Distributions of Different Solid Biomass Fuels by using Radio Frequency Identification (RFID)
Kruggel-Emden, H. and Wirtz, S.
ENERGY TECHNOLOGY. Volume: 2 (2014) - 2014 • 2876
Encapsulation strategies in energy conversion materials
Schüth, F.
CHEMISTRY OF MATERIALS. Volume: 26 (2014) - 2014 • 2875
Immunologically Controlled Biofuel Cell as a Self-Powered Biosensor for Antibiotic Residue Determination
Conzuelo, F. and Vivekananthan, J. and Pöller, S. and Pingarrón, J.M. and Schuhmann, W.
CHEMELECTROCHEM. Volume: 1 (2014) - 2014 • 2874
Modeling of screening processes with the discrete element method involving non-spherical particles
Kruggel-Emden, H. and Elskamp, F.
CHEMICAL ENGINEERING AND TECHNOLOGY. Volume: 37 (2014) - 2014 • 2873
Functional and structural fatigue of titanium tantalum high temperature shape memory alloys (HT SMAs)
Niendorf, T. and Krooß, P. and Batyrsina, E. and Paulsen, A. and Motemani, Y. and Ludwig, Al. and Buenconsejo, P. and Frenzel, J. and Eggeler, G. and Maier, H.J.
MATERIALS SCIENCE AND ENGINEERING A. Volume: 620 (2014) - 2014 • 2872
Detection and Quantification of Sulfonamide Antibiotic Residues in Milk Using Scanning Electrochemical Microscopy
Conzuelo, F. and Stratmann, L. and Grützke, S. and Pingarrón, J.M. and Schuhmann, W.
ELECTROANALYSIS. Volume: 26 (2014) - 2014 • 2871
On the functional degradation of binary titanium-tantalum high-temperature shape memory alloys - A new concept for fatigue life extension
Niendorf, T. and Krooß, P. and Batyrsina, E. and Paulsen, A. and Frenzel, J. and Eggeler, G. and Maier, H.J.
FUNCTIONAL MATERIALS LETTERS. Volume: 7 (2014) - 2014 • 2870
A polyphenylene support for pd catalysts with exceptional catalytic activity
Wang, F. and Mielby, J. and Richter, F.H. and Wang, G. and Prieto, G. and Kasama, T. and Weidenthaler, C. and Bongard, H.-J. and Kegnæs, S. and Fürstner, A. and Schüth, F.
ANGEWANDTE CHEMIE - INTERNATIONAL EDITION. Volume: 53 (2014) - 2014 • 2869
Influence of the dislocation core on the glide of the 〈1 1 〉{1 1 0} edge dislocation in bcc-iron: An embedded atom method study
Hafez Haghighat, S.M. and Von Pezold, J. and Race, C.P. and Körmann, F. and Friák, M. and Neugebauer, J. and Raabe, D.
COMPUTATIONAL MATERIALS SCIENCE. Volume: 87 (2014) - 2014 • 2868
Atomistic simulation of the a0 〈1 0 0〉 binary junction formation and its unzipping in body-centered cubic iron
Hafez Haghighat, S.M. and Schäublin, R. and Raabe, D.
ACTA MATERIALIA. Volume: 64 (2014) - 2014 • 2867
Transient and persistent current induced conductivity changes in GaAs/AlGaAs high-electron-mobility transistors
Schulte-Braucks, C. and Valentin, S.R. and Ludwig, Ar. and Wieck, A.D.
APPLIED PHYSICS LETTERS. Volume: 104 (2014) - 2014 • 2866
Platinum-cobalt bimetallic nanoparticles in hollow carbon nanospheres for hydrogenolysis of 5-hydroxymethylfurfural
Wang, G.-H. and Hilgert, J. and Richter, F.H. and Wang, F. and Bongard, H.-J. and Spliethoff, B. and Weidenthaler, C. and Schüth, F.
NATURE MATERIALS. Volume: 13 (2014) - 2014 • 2865
Holographic optical tweezers: Microassembling of shape-complementary 2PP building blocks
Ksouri, S.I. and Mattern, M. and Köhler, J. and Aumann, A. and Zyla, G. and Ostendorf, A.
PROCEEDINGS OF SPIE - THE INTERNATIONAL SOCIETY FOR OPTICAL ENGINEERING. Volume: 9164 (2014) - 2014 • 2864
Beam-solid contact formulation for finite element analysis of pile-soil interaction with arbitrary discretization
Ninić, J. and Stascheit, J. and Meschke, G.
INTERNATIONAL JOURNAL FOR NUMERICAL AND ANALYTICAL METHODS IN GEOMECHANICS. Volume: 38 (2014) - 2014 • 2863
The strong catalytic effect of Pb(II) on the oxygen reduction reaction on 5 nm gold nanoparticles
Wang, Y. and Laborda, E. and Plowman, B.J. and Tschulik, K. and Ward, K.R. and Palgrave, R.G. and Damm, C. and Compton, R.G.
PHYSICAL CHEMISTRY CHEMICAL PHYSICS. Volume: 16 (2014) - 2014 • 2862
Carbon monoxide-assisted size confinement of bimetallic alloy nanoparticles
Cui, C. and Gan, L. and Neumann, M. and Heggen, M. and Roldan Cuenya, B. and Strasser, P.
JOURNAL OF THE AMERICAN CHEMICAL SOCIETY. Volume: 136 (2014) - 2014 • 2861
Thermal screening at finite chemical potential on a topological surface and its interplay with proximity-induced ferromagnetism
Nogueira, F.S. and Eremin, I.
PHYSICAL REVIEW B - CONDENSED MATTER AND MATERIALS PHYSICS. Volume: 90 (2014) - 2014 • 2860
The multipole resonance probe: Progression and evaluation of a process compatible plasma sensor
Schulz, C. and Styrnoll, T. and Storch, R. and Awakowicz, P. and Musch, T. and Rolfes, I.
IEEE SENSORS JOURNAL. Volume: 14 (2014) - 2014 • 2859
Strong negative nanocatalysis: Oxygen reduction and hydrogen evolution at very small (2 nm) gold nanoparticles
Wang, Y. and Laborda, E. and Tschulik, K. and Damm, C. and Molina, A. and Compton, R.G.
NANOSCALE. Volume: 6 (2014) - 2014 • 2858
Selective laser patterning in organic solar cells
Abreu Fernandes, S. and Maragkaki, S. and Ostendorf, A.
PROCEEDINGS OF SPIE - THE INTERNATIONAL SOCIETY FOR OPTICAL ENGINEERING. Volume: 9180 (2014) - 2014 • 2857
Atom probe tomography observation of hydrogen in high-Mn steel and silver charged via an electrolytic route
Haley, D. and Merzlikin, S.V. and Choi, P. and Raabe, D.
INTERNATIONAL JOURNAL OF HYDROGEN ENERGY. Volume: 39 (2014) - 2014 • 2856
Narrow-band injection seeding of a terahertz frequency quantum cascade laser: Selection and suppression of longitudinal modes
Nong, H. and Pal, S. and Markmann, S. and Hekmat, N. and Mohandas, R.A. and Dean, P. and Li, L. and Linfield, E.H. and Giles Davies, A. and Wieck, A.D. and Jukam, N.
APPLIED PHYSICS LETTERS. Volume: 105 (2014) - 2014 • 2855
Separating strain from composition in unit cell parameter maps obtained from aberration corrected high resolution transmission electron microscopy imaging
Schulz, T. and Duff, A. and Remmele, T. and Korytov, M. and Markurt, T. and Albrecht, M. and Lymperakis, L. and Neugebauer, J. and Cheze, C. and Skierbiszewski, C.
JOURNAL OF APPLIED PHYSICS. Volume: 115 (2014) - 2014 • 2854
Strategies to design efficient silica-supported photocatalysts for reduction of CO2
Hamdy, M.S. and Amrollahi, R. and Sinev, I. and Mei, B. and Mul, G.
JOURNAL OF THE AMERICAN CHEMICAL SOCIETY. Volume: 136 (2014) - 2014 • 2853
Narrow bandwidth injection seeding of a THz quantum cascade laser
Nong, H. and Pal, S. and Markmann, S. and Hekmat, N. and Mohandas, R.A. and Dean, P. and Li, L. and Linfield, E.H. and Davies, A.G. and Wieck, A.D. and Jukam, N.
INTERNATIONAL CONFERENCE ON INFRARED, MILLIMETER, AND TERAHERTZ WAVES, IRMMW-THZ. Volume: (2014) - 2014 • 2852
A new approach on advanced compact plasma sensors for industrial plasma applications
Schulz, C. and Rolfes, I.
2014 IEEE SENSORS APPLICATIONS SYMPOSIUM, SAS 2014 - PROCEEDINGS. Volume: (2014) - 2014 • 2851
Hydrothermal synthesis of nitrogen-doped graphene hydrogels using amino acids with different acidities as doping agents
Wang, T. and Wang, L. and Wu, D. and Xia, W. and Zhao, H. and Jia, D.
JOURNAL OF MATERIALS CHEMISTRY A. Volume: 2 (2014) - 2014 • 2850
Electrochemical nanoprobes for single-cell analysis
Actis, P. and Tokar, S. and Clausmeyer, J. and Babakinejad, B. and Mikhaleva, S. and Cornut, R. and Takahashi, Y. and López Córdoba, A. and Novak, P. and Shevchuck, A.I. and Dougan, J.A. and Kazarian, S.G. and Gorelkin, P.V. and Erofeev, A.S. and Yaminsky, I.V. and Unwin, P.R. and Schuhmann, W. and Klenerman, D. and Rusakov, D.A. and Sviderskaya, E.V. and Korchev, Y.E.
ACS NANO. Volume: 8 (2014) - 2014 • 2849
Effects of thermal cycling parameters on residual stresses in alumina scales of CoNiCrAlY and NiCoCrAlY bond coats
Nordhorn, C. and Mücke, R. and Unocic, K.A. and Lance, M.J. and Pint, B.A. and Vaßen, R.
SURFACE AND COATINGS TECHNOLOGY. Volume: 258 (2014) - 2014 • 2848
A stacked sensor concept for industry compatible plasma diagnostics
Schulz, C. and Will, B. and Rolfes, I.
PROCEEDINGS - 2014 INTERNATIONAL CONFERENCE ON ELECTROMAGNETICS IN ADVANCED APPLICATIONS, ICEAA 2014. Volume: (2014) - 2014 • 2847
Improved morphology and performance of solution-processed metal-oxide thin-film transistors due to a polymer based interface modifier
Weber, C. and Oberberg, M. and Weber, D. and Bock, C. and Pham, D.V. and Kunze, U.
ADVANCED MATERIALS INTERFACES. Volume: 1 (2014) - 2014 • 2846
Including the effects of pressure and stress in thermodynamic functions
Hammerschmidt, T. and Abrikosov, I.A. and Alfè, D. and Fries, S.G. and Höglund, L. and Jacobs, M.H.G. and Koßmann, J. and Lu, X.-G. and Paul, G.
PHYSICA STATUS SOLIDI (B) BASIC RESEARCH. Volume: 251 (2014) - 2014 • 2845
Simulation of the effect of realistic surface textures on thermally induced topcoat stress fields by two-dimensional interface functions
Nordhorn, C. and Mücke, R. and Vaßen, R.
SURFACE AND COATINGS TECHNOLOGY. Volume: 258 (2014) - 2014 • 2844
A broadband circular TE11 - to TE01 -mode converter using stepped waveguide technique
Schulz, C. and Rolfes, I. and Will, B.
EUROPEAN MICROWAVE WEEK 2014: CONNECTING THE FUTURE, EUMW 2014 - CONFERENCE PROCEEDINGS; EUMC 2014: 44TH EUROPEAN MICROWAVE CONFERENCE. Volume: (2014) - 2014 • 2843
Investigation of Optical, Electrical, and Mechanical Properties of MOCVD-grown ZrO2 Films
Dang, V.-S. and Banerjee, M. and Zhu, H. and Srinivasan, N.B. and Parala, H. and Pfetzing-Micklich, J. and Wieck, A.D. and Devi, A.
CHEMICAL VAPOR DEPOSITION. Volume: 20 (2014) - 2014 • 2842
Next generation interatomic potentials for condensed systems
Handley, C.M. and Behler, J.
EUROPEAN PHYSICAL JOURNAL B. Volume: 87 (2014) - 2014 • 2841
Effect of processing parameters on MCrAlY bondcoat roughness and lifetime of APS-TBC systems
Nowak, W. and Naumenko, D. and Mor, G. and Mor, F. and Mack, D.E. and Vassen, R. and Singheiser, L. and Quadakkers, W.J.
SURFACE AND COATINGS TECHNOLOGY. Volume: 260 (2014) - 2014 • 2840
Complex electron heating in capacitive multi-frequency plasmas
Schulze, J. and Schungel, E. and Derzsi, A. and Korolov, I. and Mussenbrock, T. and Donko, Z.
IEEE TRANSACTIONS ON PLASMA SCIENCE. Volume: 42 (2014) - 2014 • 2839
Electrical and optical properties of TiO2 thin films prepared by plasma-enhanced atomic layer deposition
Dang, V.-S. and Parala, H. and Kim, J.H. and Xu, K. and Srinivasan, N.B. and Edengeiser, E. and Havenith, M. and Wieck, A.D. and De Los Arcos, T. and Fischer, R.A. and Devi, A.
PHYSICA STATUS SOLIDI (A) APPLICATIONS AND MATERIALS SCIENCE. Volume: 211 (2014) - 2014 • 2838
Differences between healthy hematopoietic progenitors and leukemia cells with respect to CD44 mediated rolling versus adherence behavior on hyaluronic acid coated surfaces
Hanke, M. and Hoffmann, I. and Christophis, C. and Schubert, M. and Hoang, V.T. and Zepeda-Moreno, A. and Baran, N. and Eckstein, V. and Wuchter, P. and Rosenhahn, A. and Ho, A.D.
BIOMATERIALS. Volume: 35 (2014) - 2014 • 2837
Photoluminescence of focused ion beam implanted Er3+: Y2 SiO5 crystals
Kukharchyk, N. and Pal, S. and Rödiger, J. and Ludwig, Ar. and Probst, S. and Ustinov, A.V. and Bushev, P. and Wieck, A.D.
PHYSICA STATUS SOLIDI - RAPID RESEARCH LETTERS. Volume: 8 (2014) - 2014 • 2836
Phonons and electron-phonon coupling in YNi 2 B 2 C
Weber, F. and Pintschovius, L. and Reichardt, W. and Heid, R. and Bohnen, K.-P. and Kreyssig, A. and Reznik, D. and Hradil, K.
PHYSICAL REVIEW B - CONDENSED MATTER AND MATERIALS PHYSICS. Volume: 89 (2014) - 2014 • 2835
On the nature of γ′ phase cutting and its effect on high temperature and low stress creep anisotropy of Ni-base single crystal superalloys
Agudo Jácome, L. and Nörtershäuser, P. and Somsen, C. and Dlouhý, A. and Eggeler, G.
ACTA MATERIALIA. Volume: 69 (2014) - 2014 • 2834
Coupled computational simulation of excavation and soil transport in earth-pressure balance shield tunneling machines using a viscous two-phase fluid model for soil-foam mixtures
Dang, T.S. and Meschke, G. and Wessels, N. and Hackl, K.
GEOTECHNICAL SPECIAL PUBLICATION. Volume: (2014) - 2014 • 2833
An ALE-PFEM method for the numerical simulation of two-phase mixture flow
Dang, T.S. and Meschke, G.
COMPUTER METHODS IN APPLIED MECHANICS AND ENGINEERING. Volume: 278 (2014) - 2014 • 2832
Potential-resolved dissolution of Pt-Cu: A thin-film material library study
Schuppert, A.K. and Savan, A. and Ludwig, Al. and Mayrhofer, K.J.J.
ELECTROCHIMICA ACTA. Volume: 144 (2014) - 2014 • 2831
PVP-coated, negatively charged silver nanoparticles: A multi-center study of their physicochemical characteristics, cell culture and in vivo experiments
Ahlberg, S. and Antonopulos, A. and Diendorf, J. and Dringen, R. and Epple, M. and Flöck, R. and Goedecke, W. and Graf, C. and Haberl, N. and Helmlinger, J. and Herzog, F. and Heuer, F. and Hirn, S. and Johannes, C. and Kittler, S. and Köller, M. and Korn, K. and Kreyling, W.G. and Krombach, F. and Lademann, J. and Loza, K. and Luther, E.M. and Malissek, M. and Meinke, M.C. and Nordmeyer, D. and Pailliart, A. and Raabe, J. and Rancan, F. and Rothen-Rutishauser, B.-R. and Rühl, E. and Schleh, C. and Seibel, A. and Sengstock, C. and Treuel, L. and Vogt, A. and Weber, K. and Zellner, R.
BEILSTEIN JOURNAL OF NANOTECHNOLOGY. Volume: 5 (2014) - 2014 • 2830
Multiband superconductivity in KFe2As2: Evidence for one isotropic and several lilliputian energy gaps
Hardy, F. and Ederl, R. and Jackson, M. and Aoki, D. and Paulsen, C. and Wolf, T. and Burger, P. and Böhmer, A. and Schweiss, P. and Adelmann, P. and Fisher, R.A. and Meingast, C.
JOURNAL OF THE PHYSICAL SOCIETY OF JAPAN. Volume: 83 (2014) - 2014 • 2829
Composition-Dependent Oxygen Reduction Activity and Stability of Pt-Cu Thin Films
Schuppert, A.K. and Topalov, A.A. and Savan, A. and Ludwig, Al. and Mayrhofer, K.J.J.
CHEMELECTROCHEM. Volume: 1 (2014) - 2014 • 2828
Shape-selective organic-inorganic zeolitic catalysts prepared via interlayer expansion
De Baerdemaeker, T. and Vandebroeck, W. and Gies, H. and Yilmaz, B. and Müller, U. and Feyen, M. and De Vos, D.
CATALYSIS TODAY. Volume: 235 (2014) - 2014 • 2827
Possibilities to improve the antioxidative capacity of beer by optimized hopping regimes
Kunz, T. and Frenzel, J. and Wietstock, P.C. and Methner, F.-J.
JOURNAL OF THE INSTITUTE OF BREWING. Volume: 120 (2014) - 2014 • 2826
Annulated and bridged tetrahydrofurans from alkenoxyl radical cyclization
Schur, C. and Kelm, H. and Gottwald, T. and Ludwig, Ar. and Kneuer, R. and Hartung, J.
ORGANIC AND BIOMOLECULAR CHEMISTRY. Volume: 12 (2014) - 2014 • 2825
Superconductivity from repulsion in LiFeAs: Novel s -wave symmetry and potential time-reversal symmetry breaking
Ahn, F. and Eremin, I. and Knolle, J. and Zabolotnyy, V.B. and Borisenko, S.V. and Büchner, B. and Chubukov, A.V.
PHYSICAL REVIEW B - CONDENSED MATTER AND MATERIALS PHYSICS. Volume: 89 (2014) - 2014 • 2824
A new class of solid Lewis acid catalysts based on interlayer expansion of layered silicates of the RUB-36 type with heteroatoms
De Baerdemaeker, T. and Gies, H. and Yilmaz, B. and Müller, U. and Feyen, M. and Xiao, F.-S. and Zhang, W. and Yokoi, T. and Bao, X. and De Vos, D.E.
JOURNAL OF MATERIALS CHEMISTRY A. Volume: 2 (2014) - 2014 • 2823
Redox hydrogels with adjusted redox potential for improved efficiency in Z-scheme inspired biophotovoltaic cells
Hartmann, V. and Kothe, T. and Pöller, S. and El-Mohsnawy, E. and Nowaczyk, M.M. and Plumeré, N. and Schuhmann, W. and Rögner, M.
PHYSICAL CHEMISTRY CHEMICAL PHYSICS. Volume: 16 (2014) - 2014 • 2822
Recent advances using guanidinate ligands for Chemical Vapour Deposition (CVD) and Atomic Layer Deposition (ALD) applications
Kurek, A. and Gordon, P.G. and Karle, S. and Devi, A. and Barry, S.T.
AUSTRALIAN JOURNAL OF CHEMISTRY. Volume: 67 (2014) - 2014 • 2821
Vacancy mobility and interaction with transition metal solutes in Ni
Schuwalow, S. and Rogal, J. and Drautz, R.
JOURNAL OF PHYSICS CONDENSED MATTER. Volume: 26 (2014) - 2014 • 2820
Ferrocene in the metal-organic framework MOF-5 studied by homo- and heteronuclear correlation NMR and MD simulation
Wehring, M. and Magusin, P.C.M.M. and Amirjalayer, S. and Schmid, R. and Stallmach, F.
MICROPOROUS AND MESOPOROUS MATERIALS. Volume: 186 (2014) - 2014 • 2819
Plastic anisotropy of electro-deposited pure α-iron with sharp crystallographic <1 1 1>// texture in normal direction: Analysis by an explicitly dislocation-based crystal plasticity model
Alankar, A. and Field, D.P. and Raabe, D.
INTERNATIONAL JOURNAL OF PLASTICITY. Volume: 52 (2014) - 2014 • 2818
Spin-flip Raman scattering of the resident electron in singly charged (In,Ga)As/GaAs quantum dot ensembles
Debus, J. and Sapega, V.F. and Dunker, D. and Yakovlev, D.R. and Reuter, D. and Wieck, A.D. and Bayer, M.
PHYSICAL REVIEW B - CONDENSED MATTER AND MATERIALS PHYSICS. Volume: 90 (2014) - 2014 • 2817
Spherical optical microresonator array as a multi-purpose measuring device
Weigel, T. and Dobbelstein, H. and Esen, C. and Schweiger, G. and Ostendorf, A.
PROCEEDINGS OF SPIE - THE INTERNATIONAL SOCIETY FOR OPTICAL ENGINEERING. Volume: 8960 (2014) - 2014 • 2816
Vacancy strengthening in Fe3Al iron aluminides
Hasemann, G. and Schneibel, J.H. and Krüger, M. and George, E.P.
INTERMETALLICS. Volume: 54 (2014) - 2014 • 2815
Influence of multiple particles in optical tweezers on the trapping efficiency
Weigel, T. and Ghadiri, R. and Esen, C. and Schweiger, G. and Ostendorf, A.
PROCEEDINGS OF SPIE - THE INTERNATIONAL SOCIETY FOR OPTICAL ENGINEERING. Volume: 8999 (2014) - 2014 • 2814
Parameterized electronic description of carbon cohesion in iron grain boundaries
Hatcher, N. and Madsen, G.K.H. and Drautz, R.
JOURNAL OF PHYSICS CONDENSED MATTER. Volume: 26 (2014) - 2014 • 2813
Origin of the unusually strong luminescence of a -type screw dislocations in GaN
Albrecht, M. and Lymperakis, L. and Neugebauer, J.
PHYSICAL REVIEW B - CONDENSED MATTER AND MATERIALS PHYSICS. Volume: 90 (2014) - 2014 • 2812
Structure-property relations of orthorhombic [(CH 3) 3 NCH 2COO ] 2(CuCl 2) 3·2 H 2O
Haussühl, E. and Schreuer, J. and Wiehl, L. and Paulsen, N.
JOURNAL OF SOLID STATE CHEMISTRY. Volume: 212 (2014) - 2014 • 2811
Characterisation of localised corrosion processes using scanning electrochemical impedance microscopy
Kuznetsov, V. and Maljusch, A. and Souto, R.M. and Bandarenka, A.S. and Schuhmann, W.
ELECTROCHEMISTRY COMMUNICATIONS. Volume: 44 (2014) - 2014 • 2810
Deposition and oxidation of oxide-dispersed CoNiCrAlY bondcoats
Okada, M. and Vassen, R. and Karger, M. and Sebold, D. and Mack, D. and Jarligo, M.O. and Bozza, F.
JOURNAL OF THERMAL SPRAY TECHNOLOGY. Volume: 23 (2014) - 2014 • 2809
Nano-scale characterization of thin-film solar cells
Schwarz, T. and Cojocaru-Mirédin, O. and Choi, P.-P. and Lämmle, A. and Würz, R. and Mousel, M. and Redinger, A. and Siebentritt, S. and Botti, S. and Raabe, D.
MICROSCOPY AND MICROANALYSIS. Volume: 20 (2014) - 2014 • 2808
Quasi-static micromirror with enlarged deflection based on aluminum nitride thin film springs
Weinberger, S. and Nguyen, T.T. and Ament, C. and Hoffmann, M.
SENSORS AND ACTUATORS, A: PHYSICAL. Volume: 210 (2014) - 2014 • 2807
Multi-layer thin-film electrolytes for metal supported solid oxide fuel cells
Haydn, M. and Ortner, K. and Franco, T. and Uhlenbruck, S. and Menzler, N.H. and Stöver, D. and Bräuer, G. and Venskutonis, A. and Sigl, L.S. and Buchkremer, H.-P. and Vaßen, R.
JOURNAL OF POWER SOURCES. Volume: 256 (2014) - 2014 • 2806
Nuclear magnetic resonances in (In,Ga)As/GaAs quantum dots studied by resonant optical pumping
Kuznetsova, M.S. and Flisinski, K. and Gerlovin, I.Y. and Petrov, M.Y. and Ignatiev, I.V. and Verbin, S.Y. and Yakovlev, D.R. and Reuter, D. and Wieck, A.D. and Bayer, M.
PHYSICAL REVIEW B - CONDENSED MATTER AND MATERIALS PHYSICS. Volume: 89 (2014) - 2014 • 2805
Effect of retained beta layer on slip transmission in Ti-6Al-2Zr-1Mo-1V near alpha titanium alloy during tensile deformation at room temperature
He, D. and Zhu, J. and Zaefferer, S. and Raabe, D.
MATERIALS AND DESIGN. Volume: 56 (2014) - 2014 • 2804
Direct quantitative electrical measurement of many-body interactions in exciton complexes in InAs quantum dots
Labud, P.A. and Ludwig, Ar. and Wieck, A.D. and Bester, G. and Reuter, D.
PHYSICAL REVIEW LETTERS. Volume: 112 (2014) - 2014 • 2803
Oxidative coupling of methane: Catalytic behaviour assessment via comprehensive microkinetic modelling
Alexiadis, V.I. and Thybaut, J.W. and Kechagiopoulos, P.N. and Chaar, M. and Van Veen, A.C. and Muhler, M. and Marin, G.B.
APPLIED CATALYSIS B: ENVIRONMENTAL. Volume: 150-151 (2014) - 2014 • 2802
Various Shapes of Plasma Spokes Observed in HiPIMS
Hecimovic, A. and von Keudell, A. and Schulz-von der Gathen, V. and Winter, J.
IEEE TRANSACTIONS ON PLASMA SCIENCE. Volume: 42 (2014) - 2014 • 2801
A novel high manganese austenitic steel with higher work hardening capacity and much lower impact deformation than Hadfield manganese steel
Wen, Y.H. and Peng, H.B. and Si, H.T. and Xiong, R.L. and Raabe, D.
MATERIALS AND DESIGN. Volume: 55 (2014) - 2014 • 2800
Evolution of porosity in carbide-derived carbon aerogels
Oschatz, M. and Nickel, W. and Thommes, M. and Cychosz, K.A. and Leistner, M. and Adam, M. and Mondin, G. and Strubel, P. and Borchardt, L. and Kaskel, S.
JOURNAL OF MATERIALS CHEMISTRY A. Volume: 2 (2014) - 2014 • 2799
Large recovery strain in Fe-Mn-Si-based shape memory steels obtained by engineering annealing twin boundaries
Wen, Y.H. and Peng, H.B. and Raabe, D. and Gutierrez-Urrutia, I. and Chen, J. and Du, Y.Y.
NATURE COMMUNICATIONS. Volume: 5 (2014) - 2014 • 2798
Tuning the electrically evaluated electron Landé g factor in GaAs quantum dots and quantum wells of different well widths
Allison, G. and Fujita, T. and Morimoto, K. and Teraoka, S. and Larsson, M. and Kiyama, H. and Oiwa, A. and Haffouz, S. and Austing, D.G. and Ludwig, Ar. and Wieck, A.D. and Tarucha, S.
PHYSICAL REVIEW B - CONDENSED MATTER AND MATERIALS PHYSICS. Volume: 90 (2014) - 2014 • 2797
Size dependence of the dispersion relation for the interface state between NaCl(100) and Ag(111)
Heidorn, S.-C. and Sabellek, A. and Morgenstern, K.
NANO LETTERS. Volume: 14 (2014) - 2014 • 2796
Micro- and mesoporous carbide-derived carbon prepared by a sacrificial template method in high performance lithium sulfur battery cathodes
Oschatz, M. and Lee, J.T. and Kim, H. and Nickel, W. and Borchardt, L. and Cho, W.I. and Ziegler, C. and Kaskel, S. and Yushin, G.
JOURNAL OF MATERIALS CHEMISTRY A. Volume: 2 (2014) - 2014 • 2795
Controlling the growth of palladium aerogels with high-performance toward bioelectrocatalytic oxidation of glucose
Wen, D. and Herrmann, A.-K. and Borchardt, L. and Simon, F. and Liu, W. and Kaskel, S. and Eychmüller, A.
JOURNAL OF THE AMERICAN CHEMICAL SOCIETY. Volume: 136 (2014) - 2014 • 2794
Adaptive computational simulation of TBM-soil interactions during machine-driven tunnel construction in saturated soft soils
Alsahly, A. and Stascheit, J. and Meschke, G.
GEOTECHNICAL SPECIAL PUBLICATION. Volume: (2014) - 2014 • 2793
Structural characterization of micro- and mesoporous carbon materials using in situ high pressure 129Xe NMR spectroscopy
Oschatz, M. and Hoffmann, H.C. and Pallmann, J. and Schaber, J. and Borchardt, L. and Nickel, W. and Senkovska, I. and Rico-Francés, S. and Silvestre-Albero, J. and Kaskel, S. and Brunner, E.
CHEMISTRY OF MATERIALS. Volume: 26 (2014) - 2014 • 2792
Nanoscale understanding of bond formation during cold welding of aluminum and steel
Altin, A. and Wohletz, S. and Krieger, W. and Kostka, A. and Groche, P. and Erbe, A.
ADVANCED MATERIALS RESEARCH. Volume: 966-967 (2014) - 2014 • 2791
Kroll-carbons based on silica and alumina templates as high-rate electrode materials in electrochemical double-layer capacitors
Oschatz, M. and Boukhalfa, S. and Nickel, W. and Lee, J.T. and Klosz, S. and Borchardt, L. and Eychmüller, A. and Yushin, G. and Kaskel, S.
JOURNAL OF MATERIALS CHEMISTRY A. Volume: 2 (2014) - 2014 • 2790
Hierarchical carbide-derived carbon foams with advanced mesostructure as a versatile electrochemical energy-storage material
Oschatz, M. and Borchardt, L. and Pinkert, K. and Thieme, S. and Lohe, M.R. and Hoffmann, C. and Benusch, M. and Wisser, F.M. and Ziegler, C. and Giebeler, L. and Rümmeli, M.H. and Eckert, J. and Eychmüller, A. and Kaskel, S.
ADVANCED ENERGY MATERIALS. Volume: 4 (2014) - 2014 • 2789
Numerical investigation of mixing and orientation of non-spherical particles in a model type fluidized bed
Oschmann, T. and Hold, J. and Kruggel-Emden, H.
POWDER TECHNOLOGY. Volume: 258 (2014) - 2014 • 2788
A miniaturized soil moisture sensor based on time domain transmissometry
Will, B. and Rolfes, I.
2014 IEEE SENSORS APPLICATIONS SYMPOSIUM, SAS 2014 - PROCEEDINGS. Volume: (2014) - 2014 • 2787
Surface termination of the metal-organic framework HKUST-1: A theoretical investigation
Amirjalayer, S. and Tafipolsky, M. and Schmid, R.
JOURNAL OF PHYSICAL CHEMISTRY LETTERS. Volume: 5 (2014) - 2014 • 2786
Sigma phase evolution in Co-Re-Cr-based alloys at 1100 C
Depka, T. and Somsen, C. and Eggeler, G. and Mukherji, D. and Rösler, J.
INTERMETALLICS. Volume: 48 (2014) - 2014 • 2785
Crystallography and physical properties of BaCo2 As2, Ba0.94 K0.06 Co2 As2, and Ba0.78 K0.22 Co2 As2
Anand, V.K. and Quirinale, D.G. and Lee, Y. and Harmon, B.N. and Furukawa, Y. and Ogloblichev, V.V. and Huq, A. and Abernathy, D.L. and Stephens, P.W. and McQueeney, R.J. and Kreyssig, A. and Goldman, A.I. and Johnston, D.C.
PHYSICAL REVIEW B - CONDENSED MATTER AND MATERIALS PHYSICS. Volume: 90 (2014) - 2014 • 2784
Some Comments on Copula-Based Regression
Dette, H. and Van Hecke, R. and Volgushev, S.
JOURNAL OF THE AMERICAN STATISTICAL ASSOCIATION. Volume: 109 (2014) - 2014 • 2783
Temperature dependencies of the elastic moduli and thermal expansion coefficient of an equiatomic, single-phase CoCrFeMnNi high-entropy alloy
Laplanche, G. and Gadaud, P. and Horst, O. and Otto, F. and Eggeler, G. and George, E.P.
JOURNAL OF ALLOYS AND COMPOUNDS. Volume: 623 (2014) - 2014 • 2782
Selective Ablation Of Thin Films By Pulsed Laser
Ostendorf, A. and Gurevich, E.L. and Shizhou, X.
SPRINGER SERIES IN MATERIALS SCIENCE. Volume: 195 (2014) - 2014 • 2781
Timing, duration and inversion of prograde Barrovian metamorphism constrained by high resolution Lu-Hf garnet dating: A case study from the Sikkim Himalaya, NE India
Anczkiewicz, R. and Chakraborty, S. and Dasgupta, S. and Mukhopadhyay, D. and Kołtonik, K.
EARTH AND PLANETARY SCIENCE LETTERS. Volume: 407 (2014) - 2014 • 2780
'Nearly' universally optimal designs for models with correlated observations
Dette, H. and Pepelyshev, A. and Zhigljavsky, A.
COMPUTATIONAL STATISTICS AND DATA ANALYSIS. Volume: 71 (2014) - 2014 • 2779
Sudden stress-induced transformation events during nanoindentation of NiTi shape memory alloys
Laplanche, G. and Pfetzing-Micklich, J. and Eggeler, G.
ACTA MATERIALIA. Volume: 78 (2014) - 2014 • 2778
Material requirements for hot stamping tools using the example of tool steels X38CrMoV5-3, 30MoW33-7, and 60MoCrW28-8-4
Wilzer, J. and Weber, S. and Escher, C. and Theisen, W.
HTM - JOURNAL OF HEAT TREATMENT AND MATERIALS. Volume: 69 (2014) - 2014 • 2777
Optimal designs for the Michaelis–Menten model with correlated observations
Dette, H. and Kunert, J.
STATISTICS. Volume: 48 (2014) - 2014 • 2776
Orientation dependence of stress-induced martensite formation during nanoindentation in NiTi shape memory alloys
Laplanche, G. and Pfetzing-Micklich, J. and Eggeler, G.
ACTA MATERIALIA. Volume: 68 (2014) - 2014 • 2775
Effect of silver nanoparticles on human mesenchymal stem cell differentiation
Sengstock, C. and Diendorf, J. and Epple, M. and Schildhauer, T.A. and Köller, M.
BEILSTEIN JOURNAL OF NANOTECHNOLOGY. Volume: 5 (2014) - 2014 • 2774
Temperature-dependent thermal conductivities of non-alloyed and high-alloyed heat-treatable steels in the temperature range between 20 and 500 °c
Wilzer, J. and Küpferle, J. and Weber, S. and Theisen, W.
JOURNAL OF MATERIALS SCIENCE. Volume: 49 (2014) - 2014 • 2773
Electrochemically induced deposition of poly(benzoxazine) precursors as immobilization matrix for enzymes
Andronescu, C. and Pöller, S. and Schuhmann, W.
ELECTROCHEMISTRY COMMUNICATIONS. Volume: 41 (2014) - 2014 • 2772
E-optimal designs for second-order response surface models
Dette, H. and Grigoriev, Y.
ANNALS OF STATISTICS. Volume: 42 (2014) - 2014 • 2771
Mechanical properties of Al-Cu-Fe quasicrystalline and crystalline phases: An analogy
Laplanche, G. and Bonneville, J. and Joulain, A. and Gauthier-Brunet, V. and Dubois, S.
INTERMETALLICS. Volume: 50 (2014) - 2014 • 2770
Temperature dependent transition of intragranular plastic to intergranular brittle failure in electrodeposited Cu micro-tensile samples
Wimmer, A. and Smolka, M. and Heinz, W. and Detzel, T. and Robl, W. and Motz, C. and Eyert, V. and Wimmer, E. and Jahnel, F. and Treichler, R. and Dehm, G.
MATERIALS SCIENCE AND ENGINEERING A. Volume: 618 (2014) - 2014 • 2769
Optimal designs for dose finding studies with an active control
Dette, H. and Kiss, C. and Benda, N. and Bretz, F.
JOURNAL OF THE ROYAL STATISTICAL SOCIETY. SERIES B: STATISTICAL METHODOLOGY. Volume: 76 (2014) - 2014 • 2768
Structure-related antibacterial activity of a titanium nanostructured surface fabricated by glancing angle sputter deposition
Sengstock, C. and Lopian, M. and Motemani, Y. and Borgmann, A. and Khare, C. and Buenconsejo, P.J.S. and Schildhauer, T.A. and Ludwig, Al. and Köller, M.
NANOTECHNOLOGY. Volume: 25 (2014) - 2014 • 2767
Damage evolution during cyclic tension-tension loading of micron-sized Cu lines
Wimmer, A. and Leitner, A. and Detzel, T. and Robl, W. and Heinz, W. and Pippan, R. and Dehm, G.
ACTA MATERIALIA. Volume: 67 (2014) - 2014 • 2766
Stabilizing CuPc coordination networks on Ag(100) by Ag atoms
Antczak, G. and Kamiński, W. and Morgenstern, K.
JOURNAL OF PHYSICAL CHEMISTRY C. Volume: 119 (2014) - 2014 • 2765
Distributions on matrix moment spaces
Dette, H. and Guhlich, M. and Nagel, J.
JOURNAL OF MULTIVARIATE ANALYSIS. Volume: 131 (2014) - 2014 • 2764
Microstructural evolution after thermomechanical processing in an equiatomic, single-phase CoCrFeMnNi high-entropy alloy with special focus on twin boundaries
Otto, F. and Hanold, N.L. and George, E.P.
INTERMETALLICS. Volume: 54 (2014) - 2014 • 2763
Cellobiose dehydrogenase entrapped within specifically designed Os-complex modified electrodeposition polymers as potential anodes for biofuel cells
Shao, M. and Guschin, D.A. and Kawah, Z. and Beyl, Y. and Stoica, L. and Ludwig, R. and Schuhmann, W. and Chen, X.
ELECTROCHIMICA ACTA. Volume: 128 (2014) - 2014 • 2762
Modeling the microstructure influence on fatigue life variability in structural steels
Sharaf, M. and Kucharczyk, P. and Vajragupta, N. and Münstermann, S. and Hartmaier, A. and Bleck, W.
COMPUTATIONAL MATERIALS SCIENCE. Volume: 94 (2014) - 2014 • 2761
Multiscale Approaches to Hydrogen-Assisted Degradation of Metals
Winzer, N. and Mrovec, M.
JOM. Volume: 66 (2014) - 2014 • 2760
[FeFe] Hydrogenase Models: An Overview
Apfel, U. and Pétillon, F.Y. and Schollhammer, P. and Talarmin, J. and Weigand, W.
BIOINSPIRED CATALYSIS: METAL-SULFUR COMPLEXES. Volume: (2014) - 2014 • 2759
Site occupation of Nb atoms in ternary Ni-Ti-Nb shape memory alloys
Shi, H. and Frenzel, J. and Martinez, G.T. and Van Rompaey, S. and Bakulin, A. and Kulkova, S. and Van Aert, S. and Schryvers, D.
ACTA MATERIALIA. Volume: 74 (2014) - 2014 • 2758
Dielectric tube antennas for industrial radar level gauging
Armbrecht, G. and Zietz, C. and Denicke, E. and Rolfes, I.
IEEE TRANSACTIONS ON ANTENNAS AND PROPAGATION. Volume: 60 (2014) - 2014 • 2757
Combined In Situ XPS and UHV- Chemical Force Microscopy ( CFM) Studies of the Plasma Induced Surface Oxidation of Polypropylene
Ozkaya, B. and Grosse-Kreul, S. and Corbella, C. and von Keudell, A. and Grundmeier, G.
PLASMA PROCESSES AND POLYMERS. Volume: 11 (2014) - 2014 • 2756
Energy Carriers Made from Hydrogen
Djinović, P. and Schüth, F.
ELECTROCHEMICAL ENERGY STORAGE FOR RENEWABLE SOURCES AND GRID BALANCING. Volume: (2014) - 2014 • 2755
Time and spatially resolved electron spin detection in semiconductor heterostructures by magneto-optical Kerr microscopy
Henn, T. and Kießling, T. and Molenkamp, L.W. and Reuter, D. and Wieck, A.D. and Biermann, K. and Santos, P.V. and Ossau, W.
PHYSICA STATUS SOLIDI (B) BASIC RESEARCH. Volume: 251 (2014) - 2014 • 2754
Infrared transmission spectroscopy of charge carriers in self-assembled InAs quantum dots under surface electric fields
Pal, S. and Valentin, S.R. and Kukharchyk, N. and Nong, H. and Parsa, A.B. and Eggeler, G. and Ludwig, Ar. and Jukam, N. and Wieck, A.D.
JOURNAL OF PHYSICS CONDENSED MATTER. Volume: 26 (2014) - 2014 • 2753
Almost enclosed buckyball joints: Synthesis, complex formation, and computational simulations of pentypticene-extended tribenzotriquinacene
Henne, S. and Bredenkötter, B. and Alaghemandi, M. and Bureekaew, S. and Schmid, R. and Volkmer, D.
CHEMPHYSCHEM. Volume: 15 (2014) - 2014 • 2752
First-principles-based phase diagrams and thermodynamic properties of TCP phases in Re-X systems (X = Ta, V, W)
Palumbo, M. and Fries, S.G. and Hammerschmidt, T. and Abe, T. and Crivello, J.-C. and Breidi, A.A.H. and Joubert, J.-M. and Drautz, R.
COMPUTATIONAL MATERIALS SCIENCE. Volume: 81 (2014) - 2014 • 2751
Direct observation and analysis of york-shell materials using low-voltage high-resolution scanning electron microscopy: Nanometal-particles encapsulated in metal-oxide, carbon, and polymer
Asahina, S. and Suga, M. and Takahashi, H. and Young Jeong, H. and Galeano, C. and Schüth, F. and Terasaki, O.
APL MATERIALS. Volume: 2 (2014) - 2014 • 2750
Reliability evaluation of thermophysical properties from first-principles calculations
Palumbo, M. and Fries, S.G. and Corso, A.D. and Körmann, F. and Hickel, T. and Neugebauer, J.
JOURNAL OF PHYSICS CONDENSED MATTER. Volume: 26 (2014) - 2014 • 2749
Thermogravimetric analysis of activated carbons, ordered mesoporous carbide-derived carbons, and their deactivation kinetics of catalytic methane decomposition
Shilapuram, V. and Ozalp, N. and Oschatz, M. and Borchardt, L. and Kaskel, S. and Lachance, R.
INDUSTRIAL AND ENGINEERING CHEMISTRY RESEARCH. Volume: 53 (2014) - 2014 • 2748
Recent achievements of electron beam deceleration method for FE-SEM enhanced elemental analysis including soft X-ray Emission Spectroscopy
Asahina, S. and Sakuda, Y. and Murano, T. and Takahashi, H. and Kikuchi, N. and Kawauchi, K. and Nokuo, T. and Schüth, F. and Terasaki, O.
MICROSCOPY AND MICROANALYSIS. Volume: 20 (2014) - 2014 • 2747
In-situ calibration of spatial light modulators in femtosecond pulse shapers
Döpke, B. and Balzer, J.C. and Hofmann, M.R.
PROCEEDINGS OF SPIE - THE INTERNATIONAL SOCIETY FOR OPTICAL ENGINEERING. Volume: 9004 (2014) - 2014 • 2746
Anharmonicity, mechanical instability, and thermodynamic properties of the Cr-Re σ-phase
Palumbo, M. and Fries, S.G. and Pasturel, A. and Alfè, D.
JOURNAL OF CHEMICAL PHYSICS. Volume: 140 (2014) - 2014 • 2745
Hydrogen production from catalytic decomposition of methane over ordered mesoporous carbons (CMK-3) and carbide-derived carbon (DUT-19)
Shilapuram, V. and Ozalp, N. and Oschatz, M. and Borchardt, L. and Kaskel, S.
CARBON. Volume: 67 (2014) - 2014 • 2744
Lifting of xz / yz orbital degeneracy at the structural transition in detwinned FeSe
Shimojima, T. and Suzuki, Y. and Sonobe, T. and Nakamura, A. and Sakano, M. and Omachi, J. and Yoshioka, K. and Kuwata-Gonokami, M. and Ono, K. and Kumigashira, H. and Böhmer, A.E. and Hardy, F. and Wolf, T. and Meingast, C. and Löhneysen, H.V. and Ikeda, H. and Ishizaka, K.
PHYSICAL REVIEW B - CONDENSED MATTER AND MATERIALS PHYSICS. Volume: 90 (2014) - 2014 • 2743
Using the first steps of hydration for the determination of molecular conformation of a single molecule
Henzl, J. and Boom, K. and Morgenstern, K.
JOURNAL OF THE AMERICAN CHEMICAL SOCIETY. Volume: 136 (2014) - 2014 • 2742
Relaxor behavior of pure and cerium doped CaxBa 1-xNb2O6
Pandey, C.S. and Schreuer, J. and Burianek, M. and Muehlberg, M.
FERROELECTRICS. Volume: 464 (2014) - 2014 • 2741
Magnetic properties of Gd-doped GaN
Shvarkov, S. and Ludwig, As. and Wieck, A.D. and Cordier, Y. and Ney, A. and Hardtdegen, H. and Haab, A. and Trampert, A. and Ranchal, R. and Herfort, J. and Becker, H.-W. and Rogalla, D. and Reuter, D.
PHYSICA STATUS SOLIDI (B) BASIC RESEARCH. Volume: 251 (2014) - 2014 • 2740
Use of the capping agent for the electrochemical detection and quantification of nanoparticles: CdSe quantum dots
Hepburn, W.G. and Batchelor-Mcauley, C. and Tschulik, K. and Kachoosangi, R.T. and Ness, D. and Compton, R.G.
SENSORS AND ACTUATORS, B: CHEMICAL. Volume: 204 (2014) - 2014 • 2739
Diffusional transport to and through thin-layer nanoparticle film modified electrodes: Capped CdSe nanoparticle modified electrodes
Hepburn, W.G. and Batchelor-Mcauley, C. and Tschulik, K. and Barnes, E.O. and Kachoosangi, R.T. and Compton, R.G.
PHYSICAL CHEMISTRY CHEMICAL PHYSICS. Volume: 16 (2014) - 2014 • 2738
Ordering phenomena and formation of nanostructures in Inx Ga1-x N layers coherently grown on GaN(0001)
Lee, S. and Freysoldt, C. and Neugebauer, J.
PHYSICAL REVIEW B - CONDENSED MATTER AND MATERIALS PHYSICS. Volume: 90 (2014) - 2014 • 2737
Atomic-scale quantification of grain boundary segregation in nanocrystalline material
Herbig, M. and Raabe, D. and Li, Y.J. and Choi, P. and Zaefferer, S. and Goto, S.
PHYSICAL REVIEW LETTERS. Volume: 112 (2014) - 2014 • 2736
Electrochemical detection of glutathione using a poly(caffeic acid) nanocarbon composite modified electrode
Lee, P.T. and Ward, K.R. and Tschulik, K. and Chapman, G. and Compton, R.G.
ELECTROANALYSIS. Volume: 26 (2014) - 2014 • 2735
Multimetallic aerogels by template-free self-assembly of Au, Ag, Pt, and Pd nanoparticles
Herrmann, A.-K. and Formanek, P. and Borchardt, L. and Klose, M. and Giebeler, L. and Eckert, J. and Kaskel, S. and Gaponik, N. and Eychmüller, A.
CHEMISTRY OF MATERIALS. Volume: 26 (2014) - 2014 • 2734
Influence of the PM-Processing Route and Nitrogen Content on the Properties of Ni-Free Austenitic Stainless Steel
Lefor, K. and Walter, M. and Weddeling, A. and Hryha, E. and Huth, S. and Weber, S. and Nyborg, L. and Theisen, W.
METALLURGICAL AND MATERIALS TRANSACTIONS A: PHYSICAL METALLURGY AND MATERIALS SCIENCE. Volume: 46 (2014) - 2014 • 2733
On the OES line-ratio technique in argon and argon-containing plasmas
Siepa, S. and Danko, S. and Tsankov, T.V. and Mussenbrock, T. and Czarnetzki, U.
JOURNAL OF PHYSICS D: APPLIED PHYSICS. Volume: 47 (2014) - 2014 • 2732
Recovery, recrystallization, grain growth and phase stability of a family of FCC-structured multi-component equiatomic solid solution alloys
Wu, Z. and Bei, H. and Otto, F. and Pharr, G.M. and George, E.P.
INTERMETALLICS. Volume: 46 (2014) - 2014 • 2731
Temperature dependence of the mechanical properties of equiatomic solid solution alloys with face-centered cubic crystal structures
Wu, Z. and Bei, H. and Pharr, G.M. and George, E.P.
ACTA MATERIALIA. Volume: 81 (2014) - 2014 • 2730
Unexpected magnetism, Griffiths phase, and exchange bias in the mixed lanthanide Pr0.6 Er0.4 Al2
Pathak, A.K. and Paudyal, D. and Jayasekara, W.T. and Calder, S. and Kreyssig, A. and Goldman, A.I. and Gschneidner, K.A. and Pecharsky, V.K.
PHYSICAL REVIEW B - CONDENSED MATTER AND MATERIALS PHYSICS. Volume: 89 (2014) - 2014 • 2729
Plerixafor induces the rapid and transient release of stromal cell-derived factor-1 alpha from human mesenchymal stromal cells and influences the migration behavior of human hematopoietic progenitor cells
Wuchter, P. and Leinweber, C. and Saffrich, R. and Hanke, M. and Eckstein, V. and Ho, A.D. and Grunze, M. and Rosenhahn, A.
CELL AND TISSUE RESEARCH. Volume: 355 (2014) - 2014 • 2728
Resolution and aspect ratio in two-photon lithography of positive photoresist
Aumann, A. and Isabelle Ksouri, S. and Guo, Q. and Sure, C. and Gurevich, E.L. and Ostendorf, A.
JOURNAL OF LASER APPLICATIONS. Volume: 26 (2014) - 2014 • 2727
Magnetic analytic bond-order potential for modeling the different phases of Mn at zero Kelvin
Drain, J.F. and Drautz, R. and Pettifor, D.G.
PHYSICAL REVIEW B - CONDENSED MATTER AND MATERIALS PHYSICS. Volume: 89 (2014) - 2014 • 2726
Magnetically driven suppression of nematic order in an iron-based superconductor
Avci, S. and Chmaissem, O. and Allred, J.M. and Rosenkranz, S. and Eremin, I. and Chubukov, A.V. and Bugaris, D.E. and Chung, D.Y. and Kanatzidis, M.G. and Castellan, J.-P. and Schlueter, J.A. and Claus, H. and Khalyavin, D.D. and Manuel, P. and Daoud-Aladine, A. and Osborn, R.
NATURE COMMUNICATIONS. Volume: 5 (2014) - 2014 • 2725
Applications of scale-bridging to computational materials design
Drautz, R. and Steinbach, I.
MODELLING AND SIMULATION IN MATERIALS SCIENCE AND ENGINEERING. Volume: 22 (2014) - 2014 • 2724
Spin-resolved conductance quantization in InAs
Lehmann, H. and Benter, T. and Von Ahnen, I. and Jacob, J. and Matsuyama, T. and Merkt, U. and Kunze, U. and Wieck, A.D. and Reuter, D. and Heyn, C. and Hansen, W.
SEMICONDUCTOR SCIENCE AND TECHNOLOGY. Volume: 29 (2014) - 2014 • 2723
Polyethylene wear particles induce TLR 2 upregulation in the synovial layer of mice
Paulus, A.C. and Frenzel, J. and Ficklscherer, A. and Roßbach, B.P. and Melcher, C. and Jansson, V. and Utzschneider, S.
JOURNAL OF MATERIALS SCIENCE: MATERIALS IN MEDICINE. Volume: 25 (2014) - 2014 • 2722
Synthesis, characterization, and nanoindentation response of single crystal Fe-Cr-Ni alloys with FCC and BCC structures
Xia, Y.Z. and Bei, H. and Gao, Y.F. and Catoor, D. and George, E.P.
MATERIALS SCIENCE AND ENGINEERING A. Volume: 611 (2014) - 2014 • 2721
A linear relationship between the Hall carrier concentration and the effective absorption coefficient measured by means of photothermal radiometry in IR semi-transparent n-type CdMgSe mixed crystals
Pawlak, M. and Maliński, M. and Firszt, F. and Pelzl, J. and Ludwig, Ar. and Marasek, A.
MEASUREMENT SCIENCE AND TECHNOLOGY. Volume: 25 (2014) - 2014 • 2720
Solid-state dimer method for calculating solid-solid phase transitions
Xiao, P. and Sheppard, D. and Rogal, J. and Henkelman, G.
JOURNAL OF CHEMICAL PHYSICS. Volume: 140 (2014) - 2014 • 2719
Investigation of carrier scattering mechanisms in n-Cd1- xMgxSe single crystals using Fourier Transform Infrared Spectroscopy
Pawlak, M. and Maliński, M. and Firszt, F. and Łȩgowski, S. and Mȩczyńska, H. and Ollesch, J. and Ludwig, Ar. and Marasek, A. and Schulte-Braucks, C.
INFRARED PHYSICS AND TECHNOLOGY. Volume: 64 (2014) - 2014 • 2718
Microshear deformation of gold single crystals
Heyer, J.-K. and Brinckmann, S. and Pfetzing-Micklich, J. and Eggeler, G.
ACTA MATERIALIA. Volume: 62 (2014) - 2014 • 2717
Tailoring iron(III) oxide nanomorphology by chemical vapor deposition: Growth and characterization
Peeters, D. and Carraro, G. and Maccato, C. and Parala, H. and Gasparotto, A. and Barreca, D. and Sada, C. and Kartaschew, K. and Havenith, M. and Rogalla, D. and Becker, H.-W. and Devi, A.
PHYSICA STATUS SOLIDI (A) APPLICATIONS AND MATERIALS SCIENCE. Volume: 211 (2014) - 2014 • 2716
Transition of effective hydraulic properties from low to high Reynolds number flow in porous media
Sivanesapillai, R. and Steeb, H. and Hartmaier, A.
GEOPHYSICAL RESEARCH LETTERS. Volume: 41 (2014) - 2014 • 2715
Nanostructured Er2O3 thin films grown by metalorganic chemical vapour deposition
Xu, K. and Dang, V.-S. and Ney, A. and De Los Arcos, T. and Devi, A.
JOURNAL OF NANOSCIENCE AND NANOTECHNOLOGY. Volume: 14 (2014) - 2014 • 2714
Phase equilibrium measurements of acoustically levitated squalane-CO 2 mixtures by Raman spectroscopy
Baer, S. and Esen, C. and Ostendorf, A.
JOURNAL OF RAMAN SPECTROSCOPY. Volume: 45 (2014) - 2014 • 2713
Crystallization, phase evolution and corrosion of Fe-based metallic glasses: An atomic-scale structural and chemical characterization study
Duarte, M.J. and Kostka, A. and Jimenez, J.A. and Choi, P. and Klemm, J. and Crespo, D. and Raabe, D. and Renner, F.U.
ACTA MATERIALIA. Volume: 71 (2014) - 2014 • 2712
Computational Thermodynamics: Recent developments and future potential and prospects
Hickel, T. and Kattner, U.R. and Fries, S.G.
PHYSICA STATUS SOLIDI (B) BASIC RESEARCH. Volume: 251 (2014) - 2014 • 2711
Coherent properties of single rare-earth spin qubits
Siyushev, P. and Xia, K. and Reuter, R. and Jamali, M. and Zhao, N. and Yang, N. and Duan, C. and Kukharchyk, N. and Wieck, A.D. and Kolesov, R. and Wrachtrup, J.
NATURE COMMUNICATIONS. Volume: 5 (2014) - 2014 • 2710
Enhancement of low-temperature activity over Cu-exchanged zeolite beta from organotemplate-free synthesis for the selective catalytic reduction of NOx with NH3 in exhaust gas streams
Xu, L. and Shi, C. and Zhang, Z. and Gies, H. and Xiao, F.-S. and De Vos, D. and Yokoi, T. and Bao, X. and Feyen, M. and Maurer, S. and Yilmaz, B. and Müller, U. and Zhang, W.
MICROPOROUS AND MESOPOROUS MATERIALS. Volume: 200 (2014) - 2014 • 2709
A robust dielectric feeding concept for harsh environmental TLPR antennas
Baer, C. and Musch, T. and Schulz, C. and Rolfes, I.
EUROPEAN MICROWAVE WEEK 2014: "CONNECTING THE FUTURE", EUMW 2014 - CONFERENCE PROCEEDINGS; EURAD 2014: 11TH EUROPEAN RADAR CONFERENCE. Volume: (2014) - 2014 • 2708
The role of carbonaceous deposits in the activity and stability of Ni-based catalysts applied in the dry reforming of methane
Düdder, H. and Kähler, K. and Krause, B. and Mette, K. and Kühl, S. and Behrens, M. and Scherer, V. and Muhler, M.
CATALYSIS SCIENCE AND TECHNOLOGY. Volume: 4 (2014) - 2014 • 2707
Impact of nanodiffusion on the stacking fault energy in high-strength steels
Hickel, T. and Sandlöbes, S. and Marceau, R.K.W. and Dick, A. and Bleskov, I. and Neugebauer, J. and Raabe, D.
ACTA MATERIALIA. Volume: 75 (2014) - 2014 • 2706
Understanding and controlling indium incorporation and surface segregation on in x Ga 1 - X N surfaces: An ab initio approach
Duff, A.I. and Lymperakis, L. and Neugebauer, J.
PHYSICAL REVIEW B - CONDENSED MATTER AND MATERIALS PHYSICS. Volume: 89 (2014) - 2014 • 2705
Ab initio based understanding of the segregation and diffusion mechanisms of hydrogen in steels
Hickel, T. and Nazarov, R. and McEniry, E.J. and Leyson, G. and Grabowski, B. and Neugebauer, J.
JOM. Volume: 66 (2014) - 2014 • 2704
D-lactate-selective amperometric biosensor based on the cell debris of the recombinant yeast Hansenula polymorpha
Smutok, O.V. and Dmytruk, K.V. and Karkovska, M.I. and Schuhmann, W. and Gonchar, M.V. and Sibirny, A.A.
TALANTA. Volume: 125 (2014) - 2014 • 2703
Deformation mechanisms in an austenitic single-phase duplex microstructured steel with nanotwinned grains
Yan, F.K. and Tao, N.R. and Archie, F. and Gutierrez-Urrutia, I. and Raabe, D. and Lu, K.
ACTA MATERIALIA. Volume: 81 (2014) - 2014 • 2702
CrN/AlN nanolaminate coatings deposited via high power pulsed and middle frequency pulsed magnetron sputtering
Bagcivan, N. and Bobzin, K. and Ludwig, Al. and Grochla, D. and Brugnara, R.H.
THIN SOLID FILMS. Volume: 572 (2014) - 2014 • 2701
Structural and functional characterization of enamel pigmentation in shrews
Dumont, M. and Tütken, T. and Kostka, A. and Duarte, M.J. and Borodin, S.
JOURNAL OF STRUCTURAL BIOLOGY. Volume: 186 (2014) - 2014 • 2700
Ultrafast modulation of the chemical potential in BaFe2As2 by coherent phonons
Yang, L.X. and Rohde, G. and Rohwer, T. and Stange, A. and Hanff, K. and Sohrt, C. and Rettig, L. and Cortés, R. and Chen, F. and Feng, D.L. and Wolf, T. and Kamble, B. and Eremin, I. and Popmintchev, T. and Murnane, M.M. and Kapteyn, H.C. and Kipp, L. and Fink, J. and Bauer, M. and Bovensiepen, U. and Rossnagel, K.
PHYSICAL REVIEW LETTERS. Volume: 112 (2014) - 2014 • 2699
Ab initio and Monte Carlo approaches for the magnetocaloric effect in Co- and in-doped Ni-Mn-Ga Heusler alloys
Sokolovskiy, V. and Grünebohm, A. and Buchelnikov, V. and Entel, P.
ENTROPY. Volume: 16 (2014) - 2014 • 2698
Additive inverse regression models with convolution-type operators
Hildebrandt, T. and Bissantz, N. and Dette, H.
ELECTRONIC JOURNAL OF STATISTICS. Volume: 8 (2014) - 2014 • 2697
Dynamic High-temperature Testing of an Iridium Alloy in Compression at High-strain Rates
Song, B. and Nelson, K. and Lipinski, R. and Bignell, J. and Ulrich, G. and George, E.P.
STRAIN. Volume: 50 (2014) - 2014 • 2696
Two-colour spin noise spectroscopy and fluctuation correlations reveal homogeneous linewidths within quantum-dot ensembles
Yang, L. and Glasenapp, P. and Greilich, A. and Reuter, D. and Wieck, A.D. and Yakovlev, D.R. and Bayer, M. and Crooker, S.A.
NATURE COMMUNICATIONS. Volume: 5 (2014) - 2014 • 2695
Direct microstructural evidence for the stress induced formation of martensite during nanonindentation of NiTi
Pfetzing-Micklich, J. and Wieczorek, N. and Simon, T. and Maaß, B. and Eggeler, G.
MATERIALS SCIENCE AND ENGINEERING A. Volume: 591 (2014) - 2014 • 2694
On the spheroidized carbide dissolution and elemental partitioning in high carbon bearing steel 100Cr6
Song, W. and Choi, P.-P. and Inden, G. and Prahl, U. and Raabe, D. and Bleck, W.
METALLURGICAL AND MATERIALS TRANSACTIONS A: PHYSICAL METALLURGY AND MATERIALS SCIENCE. Volume: 45 (2014) - 2014 • 2693
Ab Initio Predicted Impact of Pt on Phase Stabilities in Ni-Mn-Ga Heusler Alloys
Dutta, B. and Hickel, T. and Entel, P. and Neugebauer, J.
JOURNAL OF PHASE EQUILIBRIA AND DIFFUSION. Volume: 35 (2014) - 2014 • 2692
Load-bearing behavior of steel fiber-reinforced concrete for precast tunnel lining segments under partial-area loading
Song, F. and Breitenbücher, R.
GEOTECHNICAL SPECIAL PUBLICATION. Volume: (2014) - 2014 • 2691
Magnetic separation of paramagnetic ions from initially homogeneous solutions
Yang, X. and Tschulik, K. and Uhlemann, M. and Odenbach, S. and Eckert, K.
IEEE TRANSACTIONS ON MAGNETICS. Volume: 50 (2014) - 2014 • 2690
Influence of layer type and order on barrier properties of multilayer PECVD barrier coatings
Bahroun, K. and Behm, H. and Mitschker, F. and Awakowicz, P. and Dahlmann, R. and Hopmann, C.
JOURNAL OF PHYSICS D: APPLIED PHYSICS. Volume: 47 (2014) - 2014 • 2689
Spectroelectrochemical and morphological studies of the ageing of silver nanoparticles embedded in ultra-thin perfluorinated sputter deposited films
Ebbert, C. and Alissawi, N. and Somsen, C. and Eggeler, G. and Strunskus, T. and Faupel, F. and Grundmeier, G.
THIN SOLID FILMS. Volume: 571 (2014) - 2014 • 2688
Extraction of ACE-inhibiting dipeptides from protein hydrolysates using porous carbon materials
Hippauf, F. and Lunow, D. and Borchardt, L. and Henle, T. and Kaskel, S.
CARBON. Volume: 77 (2014) - 2014 • 2687
Intermetallic phase selection during homogenization for AA6082 alloy
Li, J.H. and Wimmer, A. and Dehm, G. and Schumacher, P.
PHILOSOPHICAL MAGAZINE. Volume: 94 (2014) - 2014 • 2686
A novel, single phase, non-equiatomic FeMnNiCoCr high-entropy alloy with exceptional phase stability and tensile ductility
Yao, M.J. and Pradeep, K.G. and Tasan, C.C. and Raabe, D.
SCRIPTA MATERIALIA. Volume: 72-73 (2014) - 2014 • 2685
High-temperature cooling histories of migmatites from the High Himalayan Crystallines in Sikkim, India: Rapid cooling unrelated to exhumation?
Sorcar, N. and Hoppe, U. and Dasgupta, S. and Chakraborty, S.
CONTRIBUTIONS TO MINERALOGY AND PETROLOGY. Volume: 167 (2014) - 2014 • 2684
Gadolinium zirconate/YSZ thermal barrier coatings: Plasma spraying, microstructure, and thermal cycling behavior
Bakan, E. and Mack, D.E. and Mauer, G. and Vaßen, R.
JOURNAL OF THE AMERICAN CERAMIC SOCIETY. Volume: 97 (2014) - 2014 • 2683
Framework stability and Brønsted acidity of isomorphously substituted interlayer-expanded zeolite COE-4: A density functional theory study
Li, H. and Zhou, D. and Tian, D. and Shi, C. and Müller, U. and Feyen, M. and Yilmaz, B. and Gies, H. and Xiao, F.-S. and De Vos, D. and Yokoi, T. and Tatsumi, T. and Bao, X. and Zhang, W.
CHEMPHYSCHEM. Volume: 15 (2014) - 2014 • 2682
Dynamical heterogeneity in the supercooled liquid state of the phase change material GeTe
Sosso, G.C. and Colombo, J. and Behler, J. and Del Gado, E. and Bernasconi, M.
JOURNAL OF PHYSICAL CHEMISTRY B. Volume: 118 (2014) - 2014 • 2681
Confined-space alloying of nanoparticles for the synthesis of efficient PtNi fuel-cell catalysts
Baldizzone, C. and Mezzavilla, S. and Carvalho, H.W.P. and Meier, J.C. and Schuppert, A.K. and Heggen, M. and Galeano, C. and Grunwaldt, J.-D. and Schüth, F. and Mayrhofer, K.J.J.
ANGEWANDTE CHEMIE - INTERNATIONAL EDITION. Volume: 53 (2014) - 2014 • 2680
Precipitation of the α-phase in an ultrafine grained beta-titanium alloy processed by severe plastic deformation
Li, T. and Kent, D. and Sha, G. and Dargusch, M.S. and Cairney, J.M.
MATERIALS SCIENCE AND ENGINEERING A. Volume: 605 (2014) - 2014 • 2679
The influence of manganese content on the stacking fault and austenite/ε-martensite interfacial energies in Fe-Mn-(Al-Si) steels investigated by experiment and theory
Pierce, D.T. and Jiménez, J.A. and Bentley, J. and Raabe, D. and Oskay, C. and Wittig, J.E.
ACTA MATERIALIA. Volume: 68 (2014) - 2014 • 2678
Construction of two- and three-dimensional statistically similar RVEs for coupled micro-macro simulations
Balzani, D. and Scheunemann, L. and Brands, D. and Schröder, J.
COMPUTATIONAL MECHANICS. Volume: 54 (2014) - 2014 • 2677
The effect of active antennas on the hot-restrike of high intensity discharge lamps
Hoebing, T. and Bergner, A. and Koch, B. and Manders, F. and Ruhrmann, C. and Mentel, J. and Awakowicz, P.
JOURNAL OF PHYSICS D: APPLIED PHYSICS. Volume: 47 (2014) - 2014 • 2676
Atomic imaging of carbon-supported Pt, Pt/Co, and Ir@Pt nanocatalysts by atom-probe tomography
Li, T. and Bagot, P.A.J. and Christian, E. and Theobald, B.R.C. and Sharman, J.D.B. and Ozkaya, D. and Moody, M.P. and Tsang, S.C.E. and Smith, G.D.W.
ACS CATALYSIS. Volume: 4 (2014) - 2014 • 2675
Benzaldehyde on water-saturated Si(001): Reaction with isolated silicon dangling bonds versus concerted hydrosilylation
Pierucci, D. and Naitabdi, A. and Bournel, F. and Gallet, J.-J. and Tissot, H. and Carniato, S. and Rochet, F. and Köhler, U. and Laumann, D. and Kubsky, S. and Silly, M.G. and Sirotti, F.
JOURNAL OF PHYSICAL CHEMISTRY C. Volume: 118 (2014) - 2014 • 2674
Influencing parameters on the dewatering behavior of annular gap grouts
Youn, B.-Y. and Breitenbücher, R.
GEOTECHNICAL SPECIAL PUBLICATION. Volume: (2014) - 2014 • 2673
A study on the influence of particle shape and shape approximation on particle mechanics in a rotating drum using the discrete element method
Höhner, D. and Wirtz, S. and Scherer, V.
POWDER TECHNOLOGY. Volume: 253 (2014) - 2014 • 2672
Segregation stabilizes nanocrystalline bulk steel with near theoretical strength
Li, Y. and Raabe, D. and Herbig, M. and Choi, P.-P. and Goto, S. and Kostka, A. and Yarita, H. and Borchers, C. and Kirchheim, R.
PHYSICAL REVIEW LETTERS. Volume: 113 (2014) - 2014 • 2671
Influencing parameters of the grout mix on the properties of annular gap grouts in mechanized tunneling
Youn, B.-Y. and Breitenbücher, R.
TUNNELLING AND UNDERGROUND SPACE TECHNOLOGY. Volume: 43 (2014) - 2014 • 2670
Femtosecond semiconductor laser system with arbitrary intracavity phase and amplitude manipulation
Balzer, J.C. and Döpke, B. and Klehr, A. and Erbert, G. and Hofmann, M.R.
PROCEEDINGS OF SPIE - THE INTERNATIONAL SOCIETY FOR OPTICAL ENGINEERING. Volume: 9002 (2014) - 2014 • 2669
Phase-specific deformation behavior of a relatively tough NiAl-Cr(Mo) lamellar composite
Yu, D. and Bei, H. and Chen, Y. and George, E.P. and An, K.
SCRIPTA MATERIALIA. Volume: 84-85 (2014) - 2014 • 2668
Mode-locked semiconductor laser system with intracavity spatial light modulator for linear and nonlinear dispersion management
Balzer, J.C. and Döpke, B. and Brenner, C. and Klehr, A. and Erbert, G. and Tränkle, G. and Hofmann, M.R.
OPTICS EXPRESS. Volume: 22 (2014) - 2014 • 2667
Role of surface functional groups in ordered mesoporous carbide-derived carbon/ionic liquid electrolyte double-layer capacitor interfaces
Pinkert, K. and Oschatz, M. and Borchardt, L. and Klose, M. and Zier, M. and Nickel, W. and Giebeler, L. and Oswald, S. and Kaskel, S. and Eckert, J.
ACS APPLIED MATERIALS AND INTERFACES. Volume: 6 (2014) - 2014 • 2666
Localized impedance measurements for electrochemical surface science
Bandarenka, A.S. and Maljusch, A. and Kuznetsov, V. and Eckhard, K. and Schuhmann, W.
JOURNAL OF PHYSICAL CHEMISTRY C. Volume: 118 (2014) - 2014 • 2665
A redox hydrogel protects hydrogenase from high-potential deactivation and oxygen damage
Plumeré, N. and Rüdiger, O. and Oughli, A.A. and Williams, R. and Vivekananthan, J. and Pöller, S. and Schuhmann, W. and Lubitz, W.
NATURE CHEMISTRY. Volume: 6 (2014) - 2014 • 2664
Techniques and methodologies in modern electrocatalysis: Evaluation of activity, selectivity and stability of catalytic materials
Bandarenka, A.S. and Ventosa, E. and Maljusch, A. and Masa, J. and Schuhmann, W.
ANALYST. Volume: 139 (2014) - 2014 • 2663
Controlled switching of ultrafast circular polarization oscillations in spin-polarized vertical-cavity surface-emitting lasers
Höpfner, H. and Lindemann, M. and Gerhardt, N.C. and Hofmann, M.R.
APPLIED PHYSICS LETTERS. Volume: 104 (2014) - 2014 • 2662
Fabrication of a Ni-Cu thin film material library using pulsed electrodeposition
Srinivas, P. and Hamann, S. and Wambach, M. and Ludwig, Al. and Dey, S.R.
JOURNAL OF THE ELECTROCHEMICAL SOCIETY. Volume: 161 (2014) - 2014 • 2661
Novel β-ketoiminato complexes of zirconium: Synthesis, characterization and evaluation for solution based processing of ZrO2 thin films
Banerjee, M. and Seidel, R.W. and Winter, M. and Becker, H.-W. and Rogalla, D. and Devi, A.
DALTON TRANSACTIONS. Volume: 43 (2014) - 2014 • 2660
Coherent switching of polarization oscillations in vertical-cavity surface-emitting lasers
Höpfner, H. and Lindemann, M. and Gerhardt, N.C. and Hofmann, M.R.
PROCEEDINGS OF SPIE - THE INTERNATIONAL SOCIETY FOR OPTICAL ENGINEERING. Volume: 9001 (2014) - 2014 • 2659
A miniaturized voltammetric pH sensor based on optimized redox polymers
Pöller, S. and Schuhmann, W.
ELECTROCHIMICA ACTA. Volume: 140 (2014) - 2014 • 2658
MOCVD of tungsten nitride thin films: Comparison of precursor performance and film characteristics
Srinivasan, N.B. and Thiede, T.B. and De Los Arcos, T. and Rogalla, D. and Becker, H.-W. and Devi, A. and Fischer, R.A.
PHYSICA STATUS SOLIDI (A) APPLICATIONS AND MATERIALS SCIENCE. Volume: 211 (2014) - 2014 • 2657
Quantitative determination of a nano-object's atom density without atomic resolution
Zaum, C. and Meyer, J. and Reuter, K. and Morgenstern, K.
PHYSICAL REVIEW B - CONDENSED MATTER AND MATERIALS PHYSICS. Volume: 90 (2014) - 2014 • 2656
MOCVD of TiO2 thin films using a heteroleptic titanium complex: Precursor evaluation and investigation of optical, photoelectrochemical and electrical properties
Banerjee, M. and Dang, V.-S. and Bledowski, M. and Beranek, R. and Becker, H.-W. and Rogalla, D. and Edengeiser, E. and Havenith, M. and Wieck, A.D. and Devi, A.
CHEMICAL VAPOR DEPOSITION. Volume: 20 (2014) - 2014 • 2655
Spin-controlled ultrafast vertical-cavity surface-emitting lasers
Höpfner, H. and Lindemann, M. and Gerhardt, N.C. and Hofmann, M.R.
PROCEEDINGS OF SPIE - THE INTERNATIONAL SOCIETY FOR OPTICAL ENGINEERING. Volume: 9134 (2014) - 2014 • 2654
Nanojunction-mediated photocatalytic enhancement in heterostructured CdS/ZnO, CdSe/ZnO, and CdTe/ZnO nanocrystals
Eley, C. and Li, T. and Liao, F. and Fairclough, S.M. and Smith, J.M. and Smith, G. and Tsang, S.C.E.
ANGEWANDTE CHEMIE - INTERNATIONAL EDITION. Volume: 53 (2014) - 2014 • 2653
On the faradaic selectivity and the role of surface inhomogeneity during the chlorine evolution reaction on ternary Ti-Ru-Ir mixed metal oxide electrocatalysts
Zeradjanin, A.R. and Menzel, N. and Schuhmann, W. and Strasser, P.
PHYSICAL CHEMISTRY CHEMICAL PHYSICS. Volume: 16 (2014) - 2014 • 2652
A system simulator including channel and frontend models for cognitive professional wireless microphones
Barowski, J. and Meiners, B. and Nalobin, A. and Dortmund, S. and Sczyslo, S. and Rolfes, I.
2014 1ST INTERNATIONAL WORKSHOP ON COGNITIVE CELLULAR SYSTEMS, CCS 2014. Volume: (2014) - 2014 • 2651
The use of cylindrical micro-wire electrodes for nano-impact experiments; Facilitating the sub-picomolar detection of single nanoparticles
Ellison, J. and Batchelor-Mcauley, C. and Tschulik, K. and Compton, R.G.
SENSORS AND ACTUATORS, B: CHEMICAL. Volume: 200 (2014) - 2014 • 2650
Plasmoids for etching and deposition
Pothiraja, R. and Bibinov, N. and Awakowicz, P.
JOURNAL OF PHYSICS D: APPLIED PHYSICS. Volume: 47 (2014) - 2014 • 2649
Rational design of the electrode morphology for oxygen evolution-enhancing the performance for catalytic water oxidation
Zeradjanin, A.R. and Topalov, A.A. and Van Overmeere, Q. and Cherevko, S. and Chen, X. and Ventosa, E. and Schuhmann, W. and Mayrhofer, K.J.J.
RSC ADVANCES. Volume: 4 (2014) - 2014 • 2648
Surface decoration of ε-Fe2O3 nanorods by CuO via a two-step CVD/sputtering approach
Barreca, D. and Carraro, G. and Peeters, D. and Gasparotto, A. and Maccato, C. and Kessels, W.M.M. and Longo, V. and Rossi, F. and Bontempi, E. and Sada, C. and Devi, A.
CHEMICAL VAPOR DEPOSITION. Volume: 20 (2014) - 2014 • 2647
Macroscopic elastic properties of textured ZrN-AlN polycrystalline aggregates: From ab initio calculations to grain-scale interactions
Holec, D. and Tasnádi, F. and Wagner, P. and Friák, M. and Neugebauer, J. and Mayrhofer, P.H. and Keckes, J.
PHYSICAL REVIEW B - CONDENSED MATTER AND MATERIALS PHYSICS. Volume: 90 (2014) - 2014 • 2646
Carbon-based micro-ball and micro-crystal deposition using filamentary pulsed atmospheric pressure plasma
Pothiraja, R. and Bibinov, N. and Awakowicz, P.
JOURNAL OF PHYSICS D: APPLIED PHYSICS. Volume: 47 (2014) - 2014 • 2645
Ab initio study of point defects in NiTi-based alloys
Holec, D. and Friák, M. and Dlouhý, A. and Neugebauer, J.
PHYSICAL REVIEW B - CONDENSED MATTER AND MATERIALS PHYSICS. Volume: 89 (2014) - 2014 • 2644
A new method for development of bond-order potentials for transition bcc metals
Lin, Y.-S. and Mrovec, M. and Vitek, V.
MODELLING AND SIMULATION IN MATERIALS SCIENCE AND ENGINEERING. Volume: 22 (2014) - 2014 • 2643
A H2 very high frequency capacitively coupled plasma inactivates glyceraldehyde 3-phosphate dehydrogenase(GapDH) more efficiently than UV photons and heat combined
Stapelmann, K. and Lackmann, J.-W. and Buerger, I. and Bandow, J.E. and Awakowicz, P.
JOURNAL OF PHYSICS D: APPLIED PHYSICS. Volume: 47 (2014) - 2014 • 2642
Analytical model for the pullout behavior of straight and hooked-end steel fibers
Zhan, Y. and Meschke, G.
JOURNAL OF ENGINEERING MECHANICS. Volume: 140 (2014) - 2014 • 2641
Role and evolution of nanoparticle structure and chemical state during the oxidation of NO over size- and shape-controlled Pt/γ-Al2O 3 catalysts under operando conditions
Lira, E. and Merte, L.R. and Behafarid, F. and Ono, L.K. and Zhang, L. and Roldan Cuenya, B.
ACS CATALYSIS. Volume: 4 (2014) - 2014 • 2640
Elemental partitioning and mechanical properties of Ti- and Ta-containing Co-Al-W-base superalloys studied by atom probe tomography and nanoindentation
Povstugar, I. and Choi, P.-P. and Neumeier, S. and Bauer, A. and Zenk, C.H. and Göken, M. and Raabe, D.
ACTA MATERIALIA. Volume: 78 (2014) - 2014 • 2639
Discrete-time windows with minimal RMS bandwidth for given RMS temporal width
Starosielec, S. and Hägele, D.
SIGNAL PROCESSING. Volume: 102 (2014) - 2014 • 2638
Ingot metallurgy and microstructural characterization of Ti-Ta alloys
Zhang, J. and Rynko, R. and Frenzel, J. and Somsen, C. and Eggeler, G.
INTERNATIONAL JOURNAL OF MATERIALS RESEARCH. Volume: 105 (2014) - 2014 • 2637
Ultrastructural organization and micromechanical properties of shark tooth enameloid
Enax, J. and Janus, A.M. and Raabe, D. and Epple, M. and Fabritius, H.-O.
ACTA BIOMATERIALIA. Volume: 10 (2014) - 2014 • 2636
Designing quadplex (four-phase) microstructures in an ultrahigh carbon steel
Zhang, H. and Ponge, D. and Raabe, D.
MATERIALS SCIENCE AND ENGINEERING A. Volume: 612 (2014) - 2014 • 2635
Characterization and Optimization Technique for Microwave-Driven High-Intensity Discharge Lamps Using Hot S-Parameters
Holtrup, S. and Sadeghfam, A. and Heuermann, H. and Awakowicz, P.
IEEE TRANSACTIONS ON MICROWAVE THEORY AND TECHNIQUES. Volume: 62 (2014) - 2014 • 2634
Mn2+ cation-directed ionothermal synthesis of an open-framework fluorinated aluminium phosphite-phosphate
Liu, H. and Tian, Z.-J. and Gies, H. and Wei, Y. and Marler, B. and Wang, L. and Wang, Y.-S. and Li, D.-W.
RSC ADVANCES. Volume: 4 (2014) - 2014 • 2633
Superplastic Mn-Si-Cr-C duplex and triplex steels: Interaction of microstructure and void formation
Zhang, H. and Ponge, D. and Raabe, D.
MATERIALS SCIENCE AND ENGINEERING A. Volume: 610 (2014) - 2014 • 2632
Simultaneous electrochemical and 3D optical imaging of silver nanoparticle oxidation
Batchelor-Mcauley, C. and Martinez-Marrades, A. and Tschulik, K. and Patel, A.N. and Combellas, C. and Kanoufi, F. and Tessier, G. and Compton, R.G.
CHEMICAL PHYSICS LETTERS. Volume: 597 (2014) - 2014 • 2631
Multiscale modeling of nanoindentation: From Atomistic to continuum models
Engels, P.S. and Begau, C. and Gupta, S. and Schmaling, B. and Ma, A. and Hartmaier, A.
SOLID MECHANICS AND ITS APPLICATIONS. Volume: 203 (2014) - 2014 • 2630
Gas-phase oxidation of ethanol over Au/TiO2catalysts to probe metal-support interactions
Holz, M.C. and Tölle, K. and Muhler, M.
CATALYSIS SCIENCE AND TECHNOLOGY. Volume: 4 (2014) - 2014 • 2629
Atom probe tomography study of ultrahigh nanocrystallization rates in FeSiNbBCu soft magnetic amorphous alloys on rapid annealing
Pradeep, K.G. and Herzer, G. and Choi, P. and Raabe, D.
ACTA MATERIALIA. Volume: 68 (2014) - 2014 • 2628
Enhanced superplasticity in an Al-alloyed multicomponent Mn-Si-Cr-C steel
Zhang, H. and Pradeep, K.G. and Mandal, S. and Ponge, D. and Choi, P. and Tasan, C.C. and Raabe, D.
ACTA MATERIALIA. Volume: 63 (2014) - 2014 • 2627
New insights into the austenitization process of low-alloyed hypereutectoid steels: Nucleation analysis of strain-induced austenite formation
Zhang, H. and Pradeep, K.G. and Mandal, S. and Ponge, D. and Raabe, D.
ACTA MATERIALIA. Volume: 80 (2014) - 2014 • 2626
Antiferromagnetism in iron-based superconductors: Selection of magnetic order and quasiparticle interference
Eremin, I. and Knolle, J. and Fernandes, R.M. and Schmalian, J. and Chubukov, A.V.
JOURNAL OF THE PHYSICAL SOCIETY OF JAPAN. Volume: 83 (2014) - 2014 • 2625
Interfacial dislocation motion and interactions in single-crystal superalloys
Liu, B. and Raabe, D. and Roters, F. and Arsenlis, A.
ACTA MATERIALIA. Volume: 79 (2014) - 2014 • 2624
Antiferromagnetism in iron-based superconductors: Magnetic order in the model of delocalized electrons
Eremin, I.M.
PHYSICS-USPEKHI. Volume: 57 (2014) - 2014 • 2623
Hybrid quantum circuit with implanted erbium ions
Probst, S. and Kukharchyk, N. and Rotzinger, H. and Tkalčec, A. and Wünsch, S. and Wieck, A.D. and Siegel, M. and Ustinov, A.V. and Bushev, P.A.
APPLIED PHYSICS LETTERS. Volume: 105 (2014) - 2014 • 2622
Development and evaluation of a device for simultaneous uniaxial compression and optical imaging of cartilage samples in vitro
Steinert, M. and Kratz, M. and Jaedicke, V. and Hofmann, M.R. and Jones, D.B.
REVIEW OF SCIENTIFIC INSTRUMENTS. Volume: 85 (2014) - 2014 • 2621
Influence of grain orientation on the local deformation mode induced by cavitation erosion in a CuSnNi alloy
Stella, J. and Pohl, M. and Bock, C. and Kunze, U.
WEAR. Volume: 316 (2014) - 2014 • 2620
Structural, optical, and magnetic properties of highly-resistive Sm-implanted GaN thin films
Lo, F.-Y. and Huang, C.-D. and Chou, K.-C. and Guo, J.-Y. and Liu, H.-L. and Ney, V. and Ney, A. and Shvarkov, S. and Pezzagna, S. and Reuter, D. and Chia, C.-T. and Chern, M.-Y. and Wieck, A.D. and Massies, J.
JOURNAL OF APPLIED PHYSICS. Volume: 116 (2014) - 2014 • 2619
Influence of zwitterionic SAMs on protein adsorption and the attachment of algal cells
Bauer, S. and Alles, M. and Finlay, J.A. and Callow, J.A. and Callow, M.E. and Rosenhahn, A.
JOURNAL OF BIOMATERIALS SCIENCE, POLYMER EDITION. Volume: 25 (2014) - 2014 • 2618
Evidences of defect contribution in magnetically ordered Sm-implanted GaN
Lo, F.-Y. and Guo, J.-Y. and Huang, C.-D. and Chou, K.-C. and Liu, H.-L. and Ney, V. and Ney, A. and Chern, M.-Y. and Shvarkov, S. and Reuter, D. and Wieck, A.D. and Pezzagna, S. and Massies, J.
CURRENT APPLIED PHYSICS. Volume: 14 (2014) - 2014 • 2617
Spinel Mn-Co oxide in N-doped carbon nanotubes as a bifunctional electrocatalyst synthesized by oxidative cutting
Zhao, A. and Masa, J. and Xia, W. and Maljusch, A. and Willinger, M.-G. and Clavel, G. and Xie, K. and Schlögl, R. and Schuhmann, W. and Muhler, M.
JOURNAL OF THE AMERICAN CHEMICAL SOCIETY. Volume: 136 (2014) - 2014 • 2616
Coal based activated carbon nanofibers prepared by electrospinning
Zhao, H. and Wang, L. and Jia, D. and Xia, W. and Li, J. and Guo, Z.
JOURNAL OF MATERIALS CHEMISTRY A. Volume: 2 (2014) - 2014 • 2615
On the superconductivity of graphite interfaces
Esquinazi, P. and Heikkilä, T.T. and Lysogorskiy, Y.V. and Tayurskii, D.A. and Volovik, G.E.
JETP LETTERS. Volume: 100 (2014) - 2014 • 2614
Reactivity of metal catalysts in glucose-fructose conversion
Loerbroks, C. and vanRijn, J. and Ruby, M.-P. and Tong, Q. and Schüth, F. and Thiel, W.
CHEMISTRY - A EUROPEAN JOURNAL. Volume: 20 (2014) - 2014 • 2613
Genome-wide association study identifies 25 known breast cancer susceptibility loci as risk factors for triple-negative breast cancer
Purrington, K. S. and Slager, S. and Eccles, D. and Yannoukakos, D. and Fasching, P. A. and Miron, P. and Carpenter, J. and Chang-Claude, J. and Martin, N. G. and Montgomery, G. W. and Kristensen, V. and Anton-Culver, H. and Goodfellow, P. and Tapper, W. J. and Rafiq, S. and Gerty, S. M. and Durcan, L. and Konstantopoulou, I. and Fostira, F. and Vratimos, A. and Apostolou, P. and Konstanta, I. and Kotoula, V. and Lakis, S. and Dimopoulos, M. A. and Skarlos, D. and Pectasides, D. and Fountzilas, G. and Beckmann, M. W. and Hein, A. and Ruebner, M. and Ekici, A. B. and Hartmann, A. and Schulz-Wendtland, R. and Renner, S. P. and Janni, W. and Rack, B. and Scholz, C. and Neugebauer, J. and Andergassen, U. and Lux, M. P. and Haeberle, L. and Clarke, C. and Pathmanathan, N. and Rudolph, A. and Flesch-Janys, D. and Nickels, S. and Olson, J. E. and Ingle, J. N. and Olswold, C. and Slettedahl, S. and Eckel-Passow, J. E. and Anderson, S. K. and Visscher, D. W. and Cafourek, V. L. and Sicotte, H. and Prodduturi, N. and Weiderpass, E. and Bernstein, L. and Ziogas, A. and Ivanovich, J. and Giles, G. G. and Baglietto, L. and Southey, M. and Kosma, V. M. and Fischer, H. P. and Reed, M. W. R. and Cross, S. S. and Deming-Halverson, S. and Shrubsole, M. and Cai, Q. Y. and Shu, X. O. and Daly, M. and Weaver, J. and Ross, E. and Klemp, J. and Sharma, P. and Torres, D. and Rudiger, T. and Wolfing, H. and Ulmer, H. U. and Forsti, A. and Khoury, T. and Kumar, S. and Pilarski, R. and Shapiro, C. L. and Greco, D. and Heikkila, P. and Aittomaki, K. and Blomqvist, C. and Irwanto, A. and Liu, J. J. and Pankratz, V. S. and Wang, X. S. and Severi, G. and Mannermaa, A. and Easton, D. and Hall, P. and Brauch, H. and Cox, A. and Zheng, W. and Godwin, A. K. and Hamann, U. and Ambrosone, C. and Toland, A. E. and Nevanlinna, H. and Vachon, C. M. and Fergus, J.
CARCINOGENESIS. Volume: 35 (2014) - 2014 • 2612
Point-by-point compositional analysis for atom probe tomography
Stephenson, L.T. and Ceguerra, A.V. and Li, T. and Rojhirunsakool, T. and Nag, S. and Banerjee, R. and Cairney, J.M. and Ringer, S.P.
METHODSX. Volume: 1 (2014) - 2014 • 2611
The role of hydrophobicity of Os-complex-modified polymers for photosystem 1 based photocathodes
Zhao, F. and Sliozberg, K. and Rögner, M. and Plumeré, N. and Schuhmann, W.
JOURNAL OF THE ELECTROCHEMICAL SOCIETY. Volume: 161 (2014) - 2014 • 2610
Highly microporous monodisperse silica spheres synthesized by the Stöber process
Bazuła, P.A. and Arnal, P.M. and Galeano, C. and Zibrowius, B. and Schmidt, W. and Schüth, F.
MICROPOROUS AND MESOPOROUS MATERIALS. Volume: 200 (2014) - 2014 • 2609
Linking glucose oxidation to luminol-based electrochemiluminescence using bipolar electrochemistry
Eßmann, V. and Jambrec, D. and Kuhn, A. and Schuhmann, W.
ELECTROCHEMISTRY COMMUNICATIONS. Volume: 50 (2014) - 2014 • 2608
Oxygen-deficient titania as alternative support for Pt catalysts for the oxygen reduction reaction
Zhao, A. and Masa, J. and Xia, W.
JOURNAL OF ENERGY CHEMISTRY. Volume: 23 (2014) - 2014 • 2607
Influence of short-range forces on melting along grain boundaries
Hüter, C. and Twiste, F. and Brener, E.A. and Neugebauer, J. and Spatschek, R.
PHYSICAL REVIEW B - CONDENSED MATTER AND MATERIALS PHYSICS. Volume: 89 (2014) - 2014 • 2606
High-pressure evolution of the specific heat of a strongly underdoped Ba(Fe 0.963 Co 0.037)As 2 iron-based superconductor
Zheng, Y. and Wang, Y. and Hardy, F. and Böhmer, A.E. and Wolf, T. and Meingast, C. and Lortz, R.
PHYSICAL REVIEW B - CONDENSED MATTER AND MATERIALS PHYSICS. Volume: 89 (2014) - 2014 • 2605
Time-resolved transconductance spectroscopy on self-assembled quantum dots: Spectral evolution from single- into many-particle states
Beckel, A. and Ludwig, Ar. and Wieck, A.D. and Lorke, A. and Geller, M. P.
PHYSICAL REVIEW B - CONDENSED MATTER AND MATERIALS PHYSICS. Volume: 89 (2014) - 2014 • 2604
Scale bridging between atomistic and mesoscale modelling: Applications of amplitude equation descriptions
Hüter, C. and Nguyen, C.-D. and Spatschek, R. and Neugebauer, J.
MODELLING AND SIMULATION IN MATERIALS SCIENCE AND ENGINEERING. Volume: 22 (2014) - 2014 • 2603
Asymmetry of charge relaxation times in quantum dots: The influence of degeneracy
Beckel, A. and Kurzmann, A. and Geller, M. P. and Ludwig, Ar. and Wieck, A.D. and König, J. and Lorke, A.
EPL. Volume: 106 (2014) - 2014 • 2602
Grain boundary characterization in multicrystalline silicon using joint EBSD, EBIC, and atom probe tomography
Stoffers, A. and Cojocaru-Miredin, O. and Breitenstein, O. and Seifert, W. and Zaefferer, S. and Raabe, D.
2014 IEEE 40TH PHOTOVOLTAIC SPECIALIST CONFERENCE (PVSC). Volume: (2014) - 2014 • 2601
Strength homogenization of matrix-inclusion composites using the linear comparison composite approach
Zhou, M.-M. and Meschke, G.
INTERNATIONAL JOURNAL OF SOLIDS AND STRUCTURES. Volume: 51 (2014) - 2014 • 2600
Zr-based conversion layer on Zn-Al-Mg alloy coated steel sheets: Insights into the formation mechanism
Lostak, T. and Maljusch, A. and Klink, B. and Krebs, S. and Kimpel, M. and Flock, J. and Schulz, S. and Schuhmann, W.
ELECTROCHIMICA ACTA. Volume: 137 (2014) - 2014 • 2599
Numerical modeling of artificial ground freezing: Multiphase modeling and strength upscaling
Zhou, M.-M. and Meschke, G.
GEOTECHNICAL SPECIAL PUBLICATION. Volume: (2014) - 2014 • 2598
A new Mg-in-plagioclase geospeedometer for the determination of cooling rates of mafic rocks
Faak, K. and Coogan, L.A. and Chakraborty, S.
GEOCHIMICA ET COSMOCHIMICA ACTA. Volume: 140 (2014) - 2014 • 2597
Yield strength dependence on strain rate of molybdenum- Alloy nanofibers
Loya, P.E. and Xia, Y.Z. and Peng, C. and Bei, H. and Zhang, P. and Zhang, J. and George, E.P. and Gao, Y.F. and Lou, J.
APPLIED PHYSICS LETTERS. Volume: 104 (2014) - 2014 • 2596
Structural stability and thermodynamics of CrN magnetic phases from ab initio calculations and experiment
Zhou, L. and Körmann, F. and Holec, D. and Bartosik, M. and Grabowski, B. and Neugebauer, J. and Mayrhofer, P.H.
PHYSICAL REVIEW B - CONDENSED MATTER AND MATERIALS PHYSICS. Volume: 90 (2014) - 2014 • 2595
An in situ transmission electron microscopy study of sintering and redispersion phenomena over size-selected metal nanoparticles: Environmental effects
Behafarid, F. and Pandey, S. and Diaz, R.E. and Stach, E.A. and Cuenya, B.R.
PHYSICAL CHEMISTRY CHEMICAL PHYSICS. Volume: 16 (2014) - 2014 • 2594
The dissolution and biological effects of silver nanoparticles in biological media
Loza, K. and Diendorf, J. and Sengstock, C. and Ruiz-Gonzalez, L. and Gonzalez-Calbet, J.M. and Vallet-Regi, M. and Köller, M. and Epple, M.
JOURNAL OF MATERIALS CHEMISTRY B. Volume: 2 (2014) - 2014 • 2593
Electrochemical detection and characterisation of polymer nanoparticles
Zhou, X.-F. and Cheng, W. and Batchelor-Mcauley, C. and Tschulik, K. and Compton, R.G.
ELECTROANALYSIS. Volume: 26 (2014) - 2014 • 2592
Structural and electronic properties of micellar Au nanoparticles: Size and ligand effects
Behafarid, F. and Matos, J. and Hong, S. and Zhang, L. and Rahman, T.S. and Roldan Cuenya, B.
ACS NANO. Volume: 8 (2014) - 2014 • 2591
Self-powered wireless carbohydrate/oxygen sensitive biodevice based on radio signal transmission
Falk, M. and Alcalde, M. and Bartlett, P.N. and De Lacey, A.L. and Gorton, L. and Gutierrez-Sanchez, C. and Haddad, R. and Kilburn, J. and Leech, D. and Ludwig, R. and Magner, E. and Mate, D.M. and Conghaile, P.Ó. and Ortiz, R. and Pita, M. and Pöller, S. and Ruzgas, T. and Salaj-Kosla, U. and Schuhmann, W. and Sebelius, F. and Shao, M. and Stoica, L. and Sygmund, C. and Tilly, J. and Toscano, M.D. and Vivekananthan, J. and Wright, E. and Shleev, S.
PLOS ONE. Volume: 9 (2014) - 2014 • 2590
The predominant species of ionic silver in biological media is colloidally dispersed nanoparticulate silver chloride
Loza, K. and Sengstock, C. and Chernousova, S. and Köller, M. and Epple, M.
RSC ADVANCES. Volume: 4 (2014) - 2014 • 2589
Ab initio based study of finite-temperature structural, elastic and thermodynamic properties of FeTi
Zhu, L.-F. and Friák, M. and Udyansky, A. and Ma, D. and Schlieter, A. and Kühn, U. and Eckert, J. and Neugebauer, J.
INTERMETALLICS. Volume: 45 (2014) - 2014 • 2588
Focussed model selection in quantile regression
Behl, P. and Claeskens, G. and Dette, H.
STATISTICA SINICA. Volume: 24 (2014) - 2014 • 2587
Characterization of Ta-Ti Thin Films by using a Scanning Droplet Cell in Combination with AC Linear Sweep Voltammetry
Fan, M. and Sliozberg, K. and La Mantia, F. and Miyashita, N. and Hagymási, M. and Schnitter, C. and Ludwig, Al. and Schuhmann, W.
CHEMELECTROCHEM. Volume: 1 (2014) - 2014 • 2586
Differences in deformation behavior of bicrystalline Cu micropillars containing a twin boundary or a large-angle grain boundary
Imrich, P.J. and Kirchlechner, C. and Motz, C. and Dehm, G.
ACTA MATERIALIA. Volume: 73 (2014) - 2014 • 2585
Recovery and Recrystallization: Phenomena, Physics, Models, Simulation
Raabe, D.
PHYSICAL METALLURGY: FIFTH EDITION. Volume: 1 (2014) - 2014 • 2584
Nanoscale sliding friction phenomena at the interface of diamond-like carbon and tungsten
Stoyanov, P. and Romero, P.A. and Merz, R. and Kopnarski, M. and Stricker, M. and Stemmer, P. and Dienwiebel, M. and Moseler, M.
ACTA MATERIALIA. Volume: 67 (2014) - 2014 • 2583
Modelling of dendritic growth during alloy solidification under natural convection
Zhu, M. and Sun, D. and Pan, S. and Zhang, Q. and Raabe, D.
MODELLING AND SIMULATION IN MATERIALS SCIENCE AND ENGINEERING. Volume: 22 (2014) - 2014 • 2582
Representing potential energy surfaces by high-dimensional neural network potentials
Behler, J.
JOURNAL OF PHYSICS CONDENSED MATTER. Volume: 26 (2014) - 2014 • 2581
Structural complexity in metal-organic frameworks: Simultaneous modification of open metal sites and hierarchical porosity by systematic doping with defective linkers
Fang, Z. and Dürholt, J.P. and Kauer, M. and Zhang, W. and Lochenie, C. and Jee, B. and Albada, B. and Metzler-Nolte, N. and Pöppl, A. and Weber, B. and Muhler, M. and Wang, Y. and Schmid, R. and Fischer, R.A.
JOURNAL OF THE AMERICAN CHEMICAL SOCIETY. Volume: 136 (2014) - 2014 • 2580
Sequential growth of zinc oxide nanorod arrays at room temperature via a corrosion process: Application in visible light photocatalysis
Iqbal, D. and Kostka, A. and Bashir, A. and Sarfraz, A. and Chen, Y. and Wieck, A.D. and Erbe, A.
ACS APPLIED MATERIALS AND INTERFACES. Volume: 6 (2014) - 2014 • 2579
Alloy Design, Combinatorial Synthesis, and Microstructure–Property Relations for Low-Density Fe-Mn-Al-C Austenitic Steels
Raabe, D. and Springer, H. and Gutierrez-Urrutia, I. and Roters, F. and Bausch, M. and Seol, J.-B. and Koyama, M. and Choi, P.-P. and Tsuzaki, K.
JOM. Volume: 66 (2014) - 2014 • 2578
Adhesion of thin CVD films on pulsed plasma pre-treated polypropylene
Behm, H. and Bahroun, K. and Bahre, H. and Kirchheim, D. and Mitschker, F. and Bibinov, N. and Böke, M. and Dahlmann, R. and Awakowicz, P. and Hopmann, C. and Winter, J.
PLASMA PROCESSES AND POLYMERS. Volume: 11 (2014) - 2014 • 2577
Polyelectrolytes polarization in nonuniform electric fields
Farahpour, F. and Ejtehadi, M.R. and Varnik, F.
INTERNATIONAL JOURNAL OF MODERN PHYSICS C. Volume: 25 (2014) - 2014 • 2576
On the nucleation of Laves phase particles during high-temperature exposure and creep of tempered martensite ferritic steels
Isik, M.I. and Kostka, A. and Eggeler, G.
ACTA MATERIALIA. Volume: 81 (2014) - 2014 • 2575
Grain boundary segregation engineering in metallic alloys: A pathway to the design of interfaces
Raabe, D. and Herbig, M. and Sandlöbes, S. and Li, Y. and Tytko, D. and Kuzmina, M. and Ponge, D. and Choi, P.-P.
CURRENT OPINION IN SOLID STATE AND MATERIALS SCIENCE. Volume: 18 (2014) - 2014 • 2574
Potential-induced degradation in solar cells: Electronic structure and diffusion mechanism of sodium in stacking faults of silicon
Ziebarth, B. and Mrovec, M. and Elsässer, C. and Gumbsch, P.
JOURNAL OF APPLIED PHYSICS. Volume: 116 (2014) - 2014 • 2573
Role of the mesoscale in migration kinetics of flat grain boundaries
Race, C.P. and Von Pezold, J. and Neugebauer, J.
PHYSICAL REVIEW B - CONDENSED MATTER AND MATERIALS PHYSICS. Volume: 89 (2014) - 2014 • 2572
Long-term microstructural stability of oxide-dispersion strengthened Eurofer steel annealed at 800 °c
Zilnyk, K.D. and Sandim, H.R.Z. and Bolmaro, R.E. and Lindau, R. and Möslang, A. and Kostka, A. and Raabe, D.
JOURNAL OF NUCLEAR MATERIALS. Volume: 448 (2014) - 2014 • 2571
A critical evaluation of the interpretation of electrocatalytic nanoimpacts
Ly, L.S.Y. and Batchelor-Mcauley, C. and Tschulik, K. and Kätelhön, E. and Compton, R.G.
JOURNAL OF PHYSICAL CHEMISTRY C. Volume: 118 (2014) - 2014 • 2570
On the influence of isotropic and kinematic hardening caused by strain gradients on the deformation behaviour of polycrystals
Ma, A. and Hartmaier, A.
PHILOSOPHICAL MAGAZINE. Volume: 94 (2014) - 2014 • 2569
Large scale 3-D phase-field simulation of coarsening in Ni-base superalloys
Rajendran, M.K. and Shchyglo, O. and Steinbach, I.
MATEC WEB OF CONFERENCES. Volume: 14 (2014) - 2014 • 2568
Gold electrodes from recordable CDs for the sensitive, semi-quantitative detection of commercial silver nanoparticles in seawater media
Stuart, E.J.E. and Tschulik, K. and Lowinsohn, D. and Cullen, J.T. and Compton, R.G.
SENSORS AND ACTUATORS, B: CHEMICAL. Volume: 195 (2014) - 2014 • 2567
Mechanical properties of SiLix thin films at different stages of electrochemical Li insertion
Zinn, A.-H. and Borhani-Haghighi, S. and Ventosa, E. and Pfetzing-Micklich, J. and Wieczorek, N. and Schuhmann, W. and Ludwig, Al.
PHYSICA STATUS SOLIDI (A) APPLICATIONS AND MATERIALS SCIENCE. Volume: 211 (2014) - 2014 • 2566
Multiwavelength phase unwrapping and aberration correction using depth filtered digital holography
Jaedicke, V. and Goebel, S. and Koukourakis, N. and Gerhardt, N.C. and Welp, H. and Hofmann, M.R.
OPTICS LETTERS. Volume: 39 (2014) - 2014 • 2565
Improving the rate of silver nanoparticle adhesion to 'sticky electrodes': Stick and strip experiments at a DMSA-modified gold electrode
Stuart, E.J.E. and Tschulik, K. and Ellison, J. and Compton, R.G.
ELECTROANALYSIS. Volume: 26 (2014) - 2014 • 2564
Interface reactions of Ag@TiO2 nanocomposite films
Zuo, J. and Rao, J. and Eggeler, G.
MATERIALS CHEMISTRY AND PHYSICS. Volume: 145 (2014) - 2014 • 2563
Performance comparison of different metrics for spectroscopic optical coherence tomography
Jaedicke, V. and Agcaer, S. and Robles, F.E. and Steinert, M. and Jones, D. and Goebel, S. and Gerhardt, N.C. and Welp, H. and Hofmann, M.R.
PROGRESS IN BIOMEDICAL OPTICS AND IMAGING - PROCEEDINGS OF SPIE. Volume: 8952 (2014) - 2014 • 2562
Electrochemical observation of single collision events: Fullerene nanoparticles
Stuart, E.J.E. and Tschulik, K. and Batchelor-Mcauley, C. and Compton, R.G.
ACS NANO. Volume: 8 (2014) - 2014 • 2561
Transmission electron microscopy and ferromagnetic resonance investigations of tunnel magnetic junctions using Co2MnGe Heusler alloys as magnetic electrodes
Belmeguenai, M. and Genevois, C. and Zighem, F. and Roussigné, Y. and Chérif, S.M. and Westerholt, K. and El Bahoui, A. and Fnidiki, A. and Moch, P.
THIN SOLID FILMS. Volume: 551 (2014) - 2014 • 2560
Kikuchi bandlet method for the accurate deconvolution and localization of Kikuchi bands in Kikuchi diffraction patterns
Ram, F. and Zaefferer, S. and Raabe, D.
JOURNAL OF APPLIED CRYSTALLOGRAPHY. Volume: 47 (2014) - 2014 • 2559
The maximum separation cluster analysis algorithm for atom-probe tomography: Parameter determination and accuracy
Jägle, E.A. and Choi, P.-P. and Raabe, D.
MICROSCOPY AND MICROANALYSIS. Volume: 20 (2014) - 2014 • 2558
Lignite fired igcc with ceramic membranes for CO2 separation
Maas, P. and Scherer, V.
ENERGY PROCEDIA. Volume: 63 (2014) - 2014 • 2557
Study on electrostatic and electromagnetic probes operated in ceramic and metallic depositing plasmas
Styrnoll, T. and Bienholz, S. and Lapke, M. and Awakowicz, P.
PLASMA SOURCES SCIENCE AND TECHNOLOGY. Volume: 23 (2014) - 2014 • 2556
Precipitation and austenite reversion behavior of a maraging steel produced by selective laser melting
Jägle, E.A. and Choi, P.-P. and Van Humbeeck, J. and Raabe, D.
JOURNAL OF MATERIALS RESEARCH. Volume: 29 (2014) - 2014 • 2555
Growth mechanism of Al2Cu precipitates during in situ TEM heating of a HPT deformed Al-3wt.%Cu alloy
Rashkova, B. and Faller, M. and Pippan, R. and Dehm, G.
JOURNAL OF ALLOYS AND COMPOUNDS. Volume: 600 (2014) - 2014 • 2554
Retarding the corrosion of iron by inhomogeneous magnetic fields
Sueptitz, R. and Tschulik, K. and Uhlemann, M. and Eckert, J. and Gebert, A.
MATERIALS AND CORROSION. Volume: 65 (2014) - 2014 • 2553
Flow characteristics of porous metal structures for specified permeability manufactured by laser beam melting technology
Benra, F.-K. and Dohmen, H.J. and Clauss, S. and Sehrt, J.T. and Witt, G.
ASME INTERNATIONAL MECHANICAL ENGINEERING CONGRESS AND EXPOSITION, PROCEEDINGS (IMECE). Volume: 2A (2014) - 2014 • 2552
Recent progress in scanning electron microscopy for the characterization of fine structural details of nano materials
Suga, M. and Asahina, S. and Sakuda, Y. and Kazumori, H. and Nishiyama, H. and Nokuo, T. and Alfredsson, V. and Kjellman, T. and Stevens, S.M. and Cho, H.S. and Cho, M. and Han, L. and Che, S. and Anderson, M.W. and Schüth, F. and Deng, H. and Yaghi, O.M. and Liu, Z. and Jeong, H.Y. and Stein, A. and Sakamoto, K. and Ryoo, R. and Terasaki, O.
PROGRESS IN SOLID STATE CHEMISTRY. Volume: 42 (2014) - 2014 • 2551
Reliable benchmark material for anatase TiO2 in Li-ion batteries: On the role of dehydration of commercial TiO2
Madej, E. and La Mantia, F. and Mei, B. and Klink, S. and Muhler, M. and Schuhmann, W. and Ventosa, E.
JOURNAL OF POWER SOURCES. Volume: 266 (2014) - 2014 • 2550
Optimization of primary printed batteries based on Zn/MnO2
Madej, E. and Espig, M. and Baumann, R.R. and Schuhmann, W. and La Mantia, F.
JOURNAL OF POWER SOURCES. Volume: 261 (2014) - 2014 • 2549
Hollow and yolk-shell iron oxide nanostructures on few-layer graphene in li-ion batteries
Sun, Z. and Xie, K. and Li, Z.A. and Sinev, I. and Ebbinghaus, P. and Erbe, A. and Farle, M. and Schuhmann, W. and Muhler, M. and Ventosa, E.
CHEMISTRY - A EUROPEAN JOURNAL. Volume: 20 (2014) - 2014 • 2548
Investigation of the thin-film phase diagram of the Cr-Ni-Re system by high-throughput experimentation
Janghorban, A. and Pfetzing-Micklich, J. and Frenzel, J. and Ludwig, Al.
ADVANCED ENGINEERING MATERIALS. Volume: 16 (2014) - 2014 • 2547
Aging effects of anatase TiO2 nanoparticles in Li-ion batteries
Madej, E. and Ventosa, E. and Klink, S. and Schuhmann, W. and La Mantia, F.
PHYSICAL CHEMISTRY CHEMICAL PHYSICS. Volume: 16 (2014) - 2014 • 2546
Impact of the specific surface area on the memory effect in Li-ion batteries: The case of anatase TiO2
Madej, E. and Mantia, F.L. and Schuhmann, W. and Ventosa, E.
ADVANCED ENERGY MATERIALS. Volume: 4 (2014) - 2014 • 2545
Amine-based solvents for exfoliating graphite to graphene outperform the dispersing capacity of N-methyl-pyrrolidone and surfactants
Sun, Z. and Huang, X. and Liu, F. and Yang, X. and Rösler, C. and Fischer, R.A. and Muhler, M. and Schuhmann, W.
CHEMICAL COMMUNICATIONS. Volume: 50 (2014) - 2014 • 2544
Simulation of the hot core mode of arc attachment at a thoriated tungsten cathode by an emitter spot model
Bergner, A. and Scharf, F.H. and Kühn, G. and Ruhrmann, C. and Hoebing, T. and Awakowicz, P. and Mentel, J.
PLASMA SOURCES SCIENCE AND TECHNOLOGY. Volume: 23 (2014) - 2014 • 2543
A carbon-coated TiO2(B) nanosheet composite for lithium ion batteries
Sun, Z. and Huang, X. and Muhler, M. and Schuhmann, W. and Ventosa, E.
CHEMICAL COMMUNICATIONS. Volume: 50 (2014) - 2014 • 2542
Investigating the outer-bulb discharge as ignition aid for automotive-HID lamps
Bergner, A. and Groeger, S. and Hoebing, T. and Ruhrmann, C. and Hechtfischer, U. and Tochadse, G. and Mentel, J. and Awakowicz, P.
JOURNAL OF PHYSICS D: APPLIED PHYSICS. Volume: 47 (2014) - 2014 • 2541
High-concentration graphene dispersions with minimal stabilizer: a scaffold for enzyme immobilization for glucose oxidation
Sun, Z. and Vivekananthan, J. and Guschin, D.A. and Huang, X. and Kuznetsov, V. and Ebbinghaus, P. and Sarfraz, A. and Muhler, M. and Schuhmann, W.
CHEMISTRY (WEINHEIM AN DER BERGSTRASSE, GERMANY). Volume: 20 (2014) - 2014 • 2540
Counting of oxygen defects versus metal surface sites in methanol synthesis catalysts by different probe molecules
Fichtl, M.B. and Schumann, J. and Kasatkin, I. and Jacobsen, N. and Behrens, M. and Schlögl, R. and Muhler, M. and Hinrichsen, O.
ANGEWANDTE CHEMIE - INTERNATIONAL EDITION. Volume: 53 (2014) - 2014 • 2539
Cyclic plasticity and lifetime of the nickel-based Alloy C-263: Experiments, models and component simulation
Maier, G. and Hübsch, O. and Riedel, H. and Somsen, C. and Klöwer, J. and Mohrmann, R.
MATEC WEB OF CONFERENCES. Volume: 14 (2014) - 2014 • 2538
Rediscovering zeolite mechanochemistry-A pathway beyond current synthesis and modification boundaries
Majano, G. and Borchardt, L. and Mitchell, S. and Valtchev, V. and Pérez-Ramírez, J.
MICROPOROUS AND MESOPOROUS MATERIALS. Volume: 194 (2014) - 2014 • 2537
Phase-field modeling of grain-boundary premelting using obstacle potentials
Bhogireddy, V.S.P.K. and Hüter, C. and Neugebauer, J. and Steinbach, I. and Karma, A. and Spatschek, R.
PHYSICAL REVIEW E - STATISTICAL, NONLINEAR, AND SOFT MATTER PHYSICS. Volume: 90 (2014) - 2014 • 2536
Plasma spray physical vapor deposition of La1-x Sr x Co y Fe1-y O3-δ Thin-film oxygen transport membrane on porous metallic supports
Jarligo, M.O. and Mauer, G. and Bram, M. and Baumann, S. and Vaßen, R.
JOURNAL OF THERMAL SPRAY TECHNOLOGY. Volume: 23 (2014) - 2014 • 2535
Versatile reactivity of a solvent-coordinated diiron(II) compound: Synthesis and dioxygen reactivity of a mixed-valent FeIIFe III species
Majumdar, A. and Apfel, U.-P. and Jiang, Y. and Moënne-Loccoz, P. and Lippard, S.J.
INORGANIC CHEMISTRY. Volume: 53 (2014) - 2014 • 2534
Complex magnetic ordering in CeGe1.76 studied by neutron diffraction
Jayasekara, W.T. and Tian, W. and Hodovanets, H. and Canfield, P.C. and Bud'Ko, S.L. and Kreyssig, A. and Goldman, A.I.
PHYSICAL REVIEW B - CONDENSED MATTER AND MATERIALS PHYSICS. Volume: 90 (2014) - 2014 • 2533
Barnacle cyprid motility and distribution in the water column as an indicator of the settlement-inhibiting potential of nontoxic antifouling chemistries
Maleschlijski, S. and Bauer, S. and Di Fino, A. and Sendra, G.H. and Clare, A.S. and Rosenhahn, A.
BIOFOULING. Volume: 30 (2014) - 2014 • 2532
Unusual band renormalization in the simplest iron-based superconductor FeSe1-x
Maletz, J. and Zabolotnyy, V.B. and Evtushinsky, D.V. and Thirupathaiah, S. and Wolter, A.U.B. and Harnagea, L. and Yaresko, A.N. and Vasiliev, A.N. and Chareev, D.A. and Böhmer, A.E. and Hardy, F. and Wolf, T. and Meingast, C. and Rienks, E.D.L. and Büchner, B. and Borisenko, S.V.
PHYSICAL REVIEW B - CONDENSED MATTER AND MATERIALS PHYSICS. Volume: 89 (2014) - 2014 • 2531
On the electrical asymmetry effect in large area multiple frequency capacitively coupled plasmas
Bienholz, S. and Styrnoll, T. and Awakowicz, P.
JOURNAL OF PHYSICS D: APPLIED PHYSICS. Volume: 47 (2014) - 2014 • 2530
Giant magnetic anisotropy and tunnelling of the magnetization in Li 2(Li1-xFex)N
Jesche, A. and McCallum, R.W. and Thimmaiah, S. and Jacobs, J.L. and Taufour, V. and Kreyssig, A. and Houk, R.S. and Bud'Ko, S.L. and Canfield, P.C.
NATURE COMMUNICATIONS. Volume: 5 (2014) - 2014 • 2529
Monitoring of amorfization of the oxygen implanted layers in silicon wafers using photothermal radiometry and modulated free carrier absorption methods
Maliński, M. and Pawlak, M. and Chrobak, Ł. and Pal, S. and Ludwig, Ar.
APPLIED PHYSICS A: MATERIALS SCIENCE AND PROCESSING. Volume: 118 (2014) - 2014 • 2528
Revealing onset potentials using electrochemical microscopy to assess the catalytic activity of gas-evolving electrodes
Maljusch, A. and Ventosa, E. and Rincón, R.A. and Bandarenka, A.S. and Schuhmann, W.
ELECTROCHEMISTRY COMMUNICATIONS. Volume: 38 (2014) - 2014 • 2527
Convergence of an analytic bond-order potential for collinear magnetism in Fe
Ford, M.E. and Drautz, R. and Hammerschmidt, T. and Pettifor, D.G.
MODELLING AND SIMULATION IN MATERIALS SCIENCE AND ENGINEERING. Volume: 22 (2014) - 2014 • 2526
Texture and microstructure evolution during non-crystallographic shear banding in a plane strain compressed Cu-Ag metal matrix composite
Jia, N. and Raabe, D. and Zhao, X.
ACTA MATERIALIA. Volume: 76 (2014) - 2014 • 2525
Characterisation of non-uniform functional surfaces: Towards linking basic surface properties with electrocatalytic activity
Maljusch, A. and Henry, J.B. and Tymoczko, J. and Bandarenka, A.S. and Schuhmann, W.
RSC ADVANCES. Volume: 4 (2014) - 2014 • 2524
Electrical conductivity of synthetic mullite single crystals
Malki, M. and Schreuer, J. and Schneider, H.
AMERICAN MINERALOGIST. Volume: 99 (2014) - 2014 • 2523
Confocal shift interferometry of coherent emission from trapped dipolar excitons
Repp, J. and Schinner, G.J. and Schubert, E. and Rai, A.K. and Reuter, D. and Wieck, A.D. and Wurstbauer, U. and Kotthaus, J.P. and Holleitner, A.W.
APPLIED PHYSICS LETTERS. Volume: 105 (2014) - 2014 • 2522
Charge order and its connection with fermi-liquid charge transport in a pristine high-Tc cuprate
Tabis, W. and Li, Y. and Le Tacon, M. and Braicovich, L. and Kreyssig, A. and Minola, M. and Dellea, G. and Weschke, E. and Veit, M.J. and Ramazanoglu, M. and Goldman, A.I. and Schmitt, T. and Ghiringhelli, G. and Barišić, N. and Chan, M.K. and Dorow, C.J. and Yu, G. and Zhao, X. and Keimer, B. and Greven, M.
NATURE COMMUNICATIONS. Volume: 5 (2014) - 2014 • 2521
Multiple reentrant glass transitions in confined hard-sphere glasses
Mandal, S. and Lang, S. and Gross, M. and Oettel, M. and Raabe, D. and Franosch, T. and Varnik, F.
NATURE COMMUNICATIONS. Volume: 5 (2014) - 2014 • 2520
Tertiary dendritic instability in late stage solidification of Ni-based superalloys
Franke, M.M. and Singer, R.F. and Steinbach, I.
MODELLING AND SIMULATION IN MATERIALS SCIENCE AND ENGINEERING. Volume: 22 (2014) - 2014 • 2519
A novel approach to measure grain boundary segregation in bulk polycrystalline materials in dependence of the boundaries' five rotational degrees of freedom
Mandal, S. and Pradeep, K.G. and Zaefferer, S. and Raabe, D.
SCRIPTA MATERIALIA. Volume: 81 (2014) - 2014 • 2518
Particle size effects in the catalytic electroreduction of CO2 on Cu nanoparticles
Reske, R. and Mistry, H. and Behafarid, F. and Roldan Cuenya, B. and Strasser, P.
JOURNAL OF THE AMERICAN CHEMICAL SOCIETY. Volume: 136 (2014) - 2014 • 2517
Economic evaluation of pre-combustion CO2-capture in IGCC power plants by porous ceramic membranes
Franz, J. and Maas, P. and Scherer, V.
APPLIED ENERGY. Volume: 130 (2014) - 2014 • 2516
Dislocation density distribution around an indent in single-crystalline nickel: Comparing nonlocal crystal plasticity finite-element predictions with experiments
Reuber, C. and Eisenlohr, P. and Roters, F. and Raabe, D.
ACTA MATERIALIA. Volume: 71 (2014) - 2014 • 2515
Hydrogen embrittlement of a carbon segregated σ5 (310) [001] symmetrical tilt grain boundary in α-Fe
Tahir, A.M. and Janisch, R. and Hartmaier, A.
MATERIALS SCIENCE AND ENGINEERING A. Volume: 612 (2014) - 2014 • 2514
Investigation on the effectiveness of chemically synthesized nano cement in controlling the physical and mechanical performances of concrete
Jo, B.W. and Chakraborty, S. and Kim, K.H.
CONSTRUCTION AND BUILDING MATERIALS. Volume: 70 (2014) - 2014 • 2513
Improved thermal cycling durability of thermal barrier coatings manufactured by PS-PVD
Rezanka, S. and Mauer, G. and Vaßen, R.
JOURNAL OF THERMAL SPRAY TECHNOLOGY. Volume: 23 (2014) - 2014 • 2512
Transmission phase in the Kondo regime revealed in a two-path interferometer
Takada, S. and Bäuerle, C. and Yamamoto, M. and Watanabe, K. and Hermelin, S. and Meunier, T. and Alex, A. and Weichselbaum, A. and Von Delft, J. and Ludwig, Ar. and Wieck, A.D. and Tarucha, S.
PHYSICAL REVIEW LETTERS. Volume: 113 (2014) - 2014 • 2511
Numerical Predictions of Surface Settlements in Mechanized Tunneling: Hybrid POD and ANN Surrogate Modeling for Reliability Analyses
Freitag, S. and Cao, B.T. and Meschke, G.
VULNERABILITY, UNCERTAINTY, AND RISK: QUANTIFICATION, MITIGATION, AND MANAGEMENT - PROCEEDINGS OF THE 2ND INTERNATIONAL CONFERENCE ON VULNERABILITY AND RISK ANALYSIS AND MANAGEMENT, ICVRAM 2014 AND THE 6TH INTERNATIONAL SYMPOSIUM ON UNCERTAINTY MODELING AND ANALYSIS, ISUMA 2014. Volume: (2014) - 2014 • 2510
A hypothetical model based on effectiveness of combined alkali and polymer latex modified jute fibre in controlling the setting and hydration behaviour of cement
Jo, B.-W. and Chakraborty, S. and Yoon, K.W.
CONSTRUCTION AND BUILDING MATERIALS. Volume: 68 (2014) - 2014 • 2509
Robust numerical calculation of tangent moduli at finite strains based on complex-step derivative approximation and its application to localization analysis
Tanaka, M. and Fujikawa, M. and Balzani, D. and Schröder, J.
COMPUTER METHODS IN APPLIED MECHANICS AND ENGINEERING. Volume: 269 (2014) - 2014 • 2508
Methanol synthesis on ZnO(000(1)over-bar). IV. Reaction mechanisms and electronic structure
Frenzel, J. and Marx, D.
JOURNAL OF CHEMICAL PHYSICS. Volume: 141 (2014) - 2014 • 2507
Effectiveness of the top-down nanotechnology in the production of ultrafine cement ( 220 nm)
Jo, B.-W. and Chakraborty, S. and Kim, K.H. and Lee, Y.S.
JOURNAL OF NANOMATERIALS. Volume: 2014 (2014) - 2014 • 2506
First description of Phanerozoic radiaxial fibrous dolomite
Richter, D.K. and Heinrich, F. and Geske, A. and Neuser, R.D. and Gies, H. and Immenhauser, A.
SEDIMENTARY GEOLOGY. Volume: 304 (2014) - 2014 • 2505
Synthesis of a cementitious material nanocement using bottom-up nanotechnology concept: An alternative approach to avoid COemission during production of cement
Jo, B.W. and Chakraborty, S. and Yoon, K.W.
JOURNAL OF NANOMATERIALS. Volume: 2014 (2014) - 2014 • 2504
Micro-patterning of self-assembled organic monolayers by using tunable ultrafast laser pulses
Maragkaki, S. and Aumann, A. and Schulz, F. and Schröter, A. and Schöps, B. and Franzka, S. and Hartmann, N. and Ostendorf, A.
PROCEEDINGS OF SPIE - THE INTERNATIONAL SOCIETY FOR OPTICAL ENGINEERING. Volume: 8972 (2014) - 2014 • 2503
First-principles calculations for point defects in solids
Freysoldt, C. and Grabowski, B. and Hickel, T. and Neugebauer, J. and Kresse, G. and Janotti, A. and Van De Walle, C.G.
REVIEWS OF MODERN PHYSICS. Volume: 86 (2014) - 2014 • 2502
Electrochemistry on binary valve metal combinatorial libraries: Niobium-tantalum thin films
Mardare, A.I. and Ludwig, Al. and Savan, A. and Hassel, A.W.
ELECTROCHIMICA ACTA. Volume: 140 (2014) - 2014 • 2501
Investigation of coking during dry reforming of methane by means of thermogravimetry
Tarasov, A. and Düdder, H. and Mette, K. and Kühl, S. and Kähler, K. and Schlögl, R. and Muhler, M. and Behrens, M.
CHEMIE-INGENIEUR-TECHNIK. Volume: 86 (2014) - 2014 • 2500
Quantum-mechanical study of single-crystalline and polycrystalline elastic properties of Mg-substituted calcite crystals
Friák, M. and Zhu, L.-F. and Lymperakis, L. and Titrian, H. and Aydin, U. and Janus, A.M. and Fabritius, H.-O. and Ziegler, A. and Nikolov, S. and Hemzalová, P. and Raabe, D. and Neugebauer, J.
KEY ENGINEERING MATERIALS. Volume: 592-593 (2014) - 2014 • 2499
Properties of anodic oxides grown on a hafnium-tantalum-titanium thin film library
Mardare, A.I. and Ludwig, Al. and Savan, A. and Hassel, A.W.
SCIENCE AND TECHNOLOGY OF ADVANCED MATERIALS. Volume: 15 (2014) - 2014 • 2498
Composition Dependence of Phase Stability, Deformation Mechanisms, and Mechanical Properties of the CoCrFeMnNi High-Entropy Alloy System
Tasan, C.C. and Deng, Y. and Pradeep, K.G. and Yao, M.J. and Springer, H. and Raabe, D.
JOM. Volume: 66 (2014) - 2014 • 2497
Adsorption and reactivity of nitrogen atoms on silica surface under plasma exposure
Marinov, D. and Guaitella, O. and de los Arcos, T. and von Keudell, A. and Rousseau, A.
JOURNAL OF PHYSICS D-APPLIED PHYSICS. Volume: 47 (2014) - 2014 • 2496
Physicochemical characteristics of drip waters: Influence on mineralogy and crystal morphology of recent cave carbonate precipitates
Riechelmann, S. and Schröder-Ritzrau, A. and Wassenburg, J.A. and Schreuer, J. and Richter, D.K. and Riechelmann, D.F.C. and Terente, M. and Constantin, S. and Mangini, A. and Immenhauser, A.
GEOCHIMICA ET COSMOCHIMICA ACTA. Volume: 145 (2014) - 2014 • 2495
Strain localization and damage in dual phase steels investigated by coupled in-situ deformation experiments and crystal plasticity simulations
Tasan, C.C. and Hoefnagels, J.P.M. and Diehl, M. and Yan, D. and Roters, F. and Raabe, D.
INTERNATIONAL JOURNAL OF PLASTICITY. Volume: 63 (2014) - 2014 • 2494
A thermo-mechanically coupled field model for shape memory alloys
Junker, P. and Hackl, K.
CONTINUUM MECHANICS AND THERMODYNAMICS. Volume: 26 (2014) - 2014 • 2493
Integrated experimental-simulation analysis of stress and strain partitioning in multiphase alloys
Tasan, C.C. and Diehl, M. and Yan, D. and Zambaldi, C. and Shanthraj, P. and Roters, F. and Raabe, D.
ACTA MATERIALIA. Volume: 81 (2014) - 2014 • 2492
The principle of the minimum of the dissipation potential for non-isothermal processes
Junker, P. and Makowski, J. and Hackl, K.
CONTINUUM MECHANICS AND THERMODYNAMICS. Volume: 26 (2014) - 2014 • 2491
Topotactic condensation of layer silicates with ferrierite-type layers forming porous tectosilicates
Marler, B. and Wang, Y. and Song, J. and Gies, H.
DALTON TRANSACTIONS. Volume: 43 (2014) - 2014 • 2490
A generalized plane-wave formulation of k · p formalism and continuum-elasticity approach to elastic and electronic properties of semiconductor nanostructures
Marquardt, O. and Boeck, S. and Freysoldt, C. and Hickel, T. and Schulz, S. and Neugebauer, J. and O'Reilly, E.P.
COMPUTATIONAL MATERIALS SCIENCE. Volume: 95 (2014) - 2014 • 2489
Activation of oxygen evolving perovskites for oxygen reduction by functionalization with Fe-Nx/C groups
Rincón, R.A. and Masa, J. and Mehrpour, S. and Tietz, F. and Schuhmann, W.
CHEMICAL COMMUNICATIONS. Volume: 50 (2014) - 2014 • 2488
Evaluation of Perovskites as Electrocatalysts for the Oxygen Evolution Reaction
Rincõn, R.A. and Ventosa, E. and Tietz, F. and Masa, J. and Seisel, S. and Kuznetsov, V. and Schuhmann, W.
CHEMPHYSCHEM. Volume: 15 (2014) - 2014 • 2487
Fractionation of 'water-soluble lignocellulose' into C5/C 6 sugars and sulfur-free lignins
Käldström, M. and Meine, N. and Farès, C. and Rinaldi, R. and Schüth, F.
GREEN CHEMISTRY. Volume: 16 (2014) - 2014 • 2486
Cu/ZnO nanocatalysts in response to environmental conditions: surface morphology, electronic structure, redox state and CO2 activation
Martinez-Suarez, L. and Frenzel, J. and Marx, D.
PHYSICAL CHEMISTRY CHEMICAL PHYSICS. Volume: 16 (2014) - 2014 • 2485
Deciphering 'water-soluble lignocellulose' obtained by mechanocatalysis: New insights into the chemical processes leading to deep depolymerization
Käldström, M. and Meine, N. and Farès, C. and Schüth, F. and Rinaldi, R.
GREEN CHEMISTRY. Volume: 16 (2014) - 2014 • 2484
Influence of solution nitriding of supersolidus-sintered cold work tool steels on their hardenability
Blüm, M. and Conrads, J. and Weber, S. and Theisen, W.
HTM - JOURNAL OF HEAT TREATMENT AND MATERIALS. Volume: 69 (2014) - 2014 • 2483
Hydrogen evolution from metal-surface hydroxyl interaction
Fujimori, Y. and Kaden, W.E. and Brown, M.A. and Roldan Cuenya, B. and Sterrer, M. and Freund, H.-J.
JOURNAL OF PHYSICAL CHEMISTRY C. Volume: 118 (2014) - 2014 • 2482
Nanoparticle-Impact Experiments are Highly Sensitive to the Presence of Adsorbed Species on Electrode Surfaces
Kätelhön, E. and Cheng, W. and Batchelor-Mcauley, C. and Tschulik, K. and Compton, R.G.
CHEMELECTROCHEM. Volume: 1 (2014) - 2014 • 2481
Perspectives on point defect thermodynamics
Rogal, J. and Divinski, S.V. and Finnis, M.W. and Glensk, A. and Neugebauer, J. and Perepezko, J.H. and Schuwalow, S. and Sluiter, M.H.F. and Sundman, B.
PHYSICA STATUS SOLIDI (B) BASIC RESEARCH. Volume: 251 (2014) - 2014 • 2480
Tailored beam shaping for laser spot joining of highly conductive thin foils
Funck, K. and Nett, R. and Ostendorf, A.
PHYSICS PROCEDIA. Volume: 56 (2014) - 2014 • 2479
Constraints on the nature and evolution of the magma plumbing system of Mt. Etna volcano (1991-2008) from a combined thermodynamic and kinetic modelling of the compositional record of minerals
Kahl, M. and Chakraborty, S. and Pompilio, M. and Costa, F.
JOURNAL OF PETROLOGY. Volume: 56 (2014) - 2014 • 2478
MnxOy/NC and CoxOy/NC nanoparticles embedded in a nitrogen-doped carbon matrix for high-performance bifunctional oxygen electrodes
Masa, J. and Xia, W. and Sinev, I. and Zhao, A. and Sun, Z. and Grützke, S. and Weide, P. and Muhler, M. and Schuhmann, W.
ANGEWANDTE CHEMIE - INTERNATIONAL EDITION. Volume: 53 (2014) - 2014 • 2477
Metal-free catalysts for oxygen reduction in alkaline electrolytes: Influence of the presence of Co, Fe, Mn and Ni inclusions
Masa, J. and Zhao, A. and Wei, X. and Muhler, M. and Schuhmann, W.
ELECTROCHIMICA ACTA. Volume: 128 (2014) - 2014 • 2476
Anomalous Fermi surface in FeSe seen by Shubnikov-de Haas oscillation measurements
Terashima, T. and Kikugawa, N. and Kiswandhi, A. and Choi, E.-S. and Brooks, J.S. and Kasahara, S. and Watashige, T. and Ikeda, H. and Shibauchi, T. and Matsuda, Y. and Wolf, T. and Böhmer, A.E. and Hardy, F. and Meingast, C. and Löhneysen, H.V. and Suzuki, M.-T. and Arita, R. and Uji, S.
PHYSICAL REVIEW B - CONDENSED MATTER AND MATERIALS PHYSICS. Volume: 90 (2014) - 2014 • 2475
Liquid-solid interfaces: Structure and dynamics from spectroscopy and simulations
Gaigeot, M.-P. and Sulpizi, M.
JOURNAL OF PHYSICS CONDENSED MATTER. Volume: 26 (2014) - 2014 • 2474
Interaction of charged amino-acid side chains with ions: An optimization strategy for classical force fields
Kahlen, J. and Salimi, L. and Sulpizi, M. and Peter, C. and Donadio, D.
JOURNAL OF PHYSICAL CHEMISTRY B. Volume: 118 (2014) - 2014 • 2473
Koutecky-Levich analysis applied to nanoparticle modified rotating disk electrodes: Electrocatalysis or misinterpretation
Masa, J. and Batchelor-McAuley, C. and Schuhmann, W. and Compton, R.G.
NANO RESEARCH. Volume: 7 (2014) - 2014 • 2472
Atomic layer deposition of TiO2 and ZrO2 thin films using heteroleptic guanidinate precursors
Kaipio, M. and Blanquart, T. and Banerjee, M. and Xu, K. and Niinistö, J. and Longo, V. and Mizohata, K. and Devi, A. and Ritala, M. and Leskelä, M.
CHEMICAL VAPOR DEPOSITION. Volume: 20 (2014) - 2014 • 2471
Fast Time Resolved Techniques as Key to the Understanding of Energy and Particle Transport in HPPMS-Plasmas
Maszl, C. and Breilmann, W. and Berscheid, L. and Benedikt, J. and von Keudell, A.
IEEE TRANSACTIONS ON PLASMA SCIENCE. Volume: 42 (2014) - 2014 • 2470
Coarse graining and localized plasticity between sliding nanocrystalline metals
Romero, P.A. and Järvi, T.T. and Beckmann, N. and Mrovec, M. and Moseler, M.
PHYSICAL REVIEW LETTERS. Volume: 113 (2014) - 2014 • 2469
Interplay between exchange interaction and magnetic field gradient in a double quantum dot with two individual electron spin qubits
Thalineau, R. and Valentin, S.R. and Wieck, A.D. and Bäuerle, C. and Meunier, T.
PHYSICAL REVIEW B - CONDENSED MATTER AND MATERIALS PHYSICS. Volume: 90 (2014) - 2014 • 2468
Nematic susceptibility of hole-doped and electron-doped Ba F e 2 As 2 iron-based superconductors from shear modulus measurements
Böhmer, A.E. and Burger, P. and Hardy, F. and Wolf, T. and Schweiss, P. and Fromknecht, R. and Reinecker, M. and Schranz, W. and Meingast, C.
PHYSICAL REVIEW LETTERS. Volume: 112 (2014) - 2014 • 2467
Origin of the energetic ions at the substrate generated during high power pulsed magnetron sputtering of titanium
Maszl, C. and Breilmann, W. and Benedikt, J. and von Keudell, A.
JOURNAL OF PHYSICS D-APPLIED PHYSICS. Volume: 47 (2014) - 2014 • 2466
Atomic-scale engineering of multifunctional nano-sized materials and films
Roozeboom, F. and Barreca, D. and Devi, A. and Parala, H. and Ritala, M.
PHYSICA STATUS SOLIDI (A) APPLICATIONS AND MATERIALS SCIENCE. Volume: 211 (2014) - 2014 • 2465
DFT-supported phase-field study on the effect of mechanically driven fluxes in Ni4Ti3 precipitation
Kamachali, R.D. and Borukhovich, E. and Hatcher, N. and Steinbach, I.
MODELLING AND SIMULATION IN MATERIALS SCIENCE AND ENGINEERING. Volume: 22 (2014) - 2014 • 2464
Rapid identification of areas of interest in thin film materials libraries by combining electrical, optical, X-ray diffraction, and mechanical high-throughput measurements: A case study for the system ni-al
Thienhaus, S. and Naujoks, D. and Pfetzing-Micklich, J. and König, D. and Ludwig, Al.
ACS COMBINATORIAL SCIENCE. Volume: 16 (2014) - 2014 • 2463
Nitrogen-doped hollow carbon spheres as a support for platinum-based electrocatalysts
Galeano, C. and Meier, J.C. and Soorholtz, M. and Bongard, H. and Baldizzone, C. and Mayrhofer, K.J.J. and Schüth, F.
ACS CATALYSIS. Volume: 4 (2014) - 2014 • 2462
Carbon-based yolk-shell materials for fuel cell applications
Galeano, C. and Baldizzone, C. and Bongard, H. and Spliethoff, B. and Weidenthaler, C. and Meier, J.C. and Mayrhofer, K.J.J. and Schüth, F.
ADVANCED FUNCTIONAL MATERIALS. Volume: 24 (2014) - 2014 • 2461
Interface properties in lamellar TiAl microstructures from density functional theory
Kanani, M. and Hartmaier, A. and Janisch, R.
INTERMETALLICS. Volume: 54 (2014) - 2014 • 2460
Structure of rapidly quenched (Cu0.5Zr0.5) 100-xAgx alloys (x = 0-40 at.%)
Mattern, N. and Han, J.H. and Pradeep, K.G. and Kim, K.C. and Park, E.M. and Kim, D.H. and Yokoyama, Y. and Raabe, D. and Eckert, J.
JOURNAL OF ALLOYS AND COMPOUNDS. Volume: 607 (2014) - 2014 • 2459
Electron spin dynamics in GaN
Rudolph, J. and Buß, J.H. and Hägele, D.
PHYSICA STATUS SOLIDI (B) BASIC RESEARCH. Volume: 251 (2014) - 2014 • 2458
Conditioning of self-assembled monolayers at two static immersion test sites along the east coast of Florida and its effect on early fouling development
Thome, I. and Bauer, S. and Vater, S. and Zargiel, K. and Finlay, J.A. and Arpa-Sancet, M.P. and Alles, M. and Callow, J.A. and Callow, M.E. and Swain, G.W. and Grunze, M. and Rosenhahn, A.
BIOFOULING. Volume: 30 (2014) - 2014 • 2457
Tailoring porosity in carbon materials for supercapacitor applications
Borchardt, L. and Oschatz, M. and Kaskel, S.
MATERIALS HORIZONS. Volume: 1 (2014) - 2014 • 2456
Mesostructural design and manufacturing of open-pore metal foams by investment casting
Matz, A.M. and Mocker, B.S. and Müller, D.W. and Jost, N. and Eggeler, G.
ADVANCES IN MATERIALS SCIENCE AND ENGINEERING. Volume: 2014 (2014) - 2014 • 2455
A hard-templating route towards ordered mesoporous tungsten carbide and carbide-derived carbons
Borchardt, L. and Oschatz, M. and Graetz, S. and Lohe, M.R. and Rümmeli, M.H. and Kaskel, S.
MICROPOROUS AND MESOPOROUS MATERIALS. Volume: 186 (2014) - 2014 • 2454
Effect of strain rate on twinning in a Zr alloy
Kapoor, R. and Sarkar, A. and Singh, J. and Samajdar, I. and Raabe, D.
SCRIPTA MATERIALIA. Volume: 74 (2014) - 2014 • 2453
The effect of surface reactions of O, O-3 and N on film properties during the growth of silica-like films
Rugner, K. and Reuter, R. and von Keudell, A. and Benedikt, J.
JOURNAL OF PHYSICS D-APPLIED PHYSICS. Volume: 47 (2014) - 2014 • 2452
MCrAlY bondcoats by high-velocity atmospheric plasma spraying
Mauer, G. and Sebold, D. and Vaßen, R.
JOURNAL OF THERMAL SPRAY TECHNOLOGY. Volume: 23 (2014) - 2014 • 2451
Gas Phase Emitter Effect of Thulium within Ceramic Metal Halide Lamps in Dependence on Frequency
Ruhrmann, C. and Depta, M. and Bergner, A. and Höbing, T. and Denissen, C. and Suijker, J. and Mentel, J. and Awakowicz, P.
CONTRIBUTIONS TO PLASMA PHYSICS. Volume: 54 (2014) - 2014 • 2450
Large strain elasto-plasticity for diffuse interface models
Borukhovich, E. and Engels, P.S. and Böhlke, T. and Shchyglo, O. and Steinbach, I.
MODELLING AND SIMULATION IN MATERIALS SCIENCE AND ENGINEERING. Volume: 22 (2014) - 2014 • 2449
Plasma Spraying of Ceramics with Particular Difficulties in Processing
Mauer, G. and Schlegel, N. and Guignard, A. and Jarligo, M.O. and Rezanka, S. and Hospach, A. and Vaßen, R.
JOURNAL OF THERMAL SPRAY TECHNOLOGY. Volume: 24 (2014) - 2014 • 2448
Effect of constant-rate reduction on the performance of a ternary Cu/ZnO/Al2O3 catalyst in methanol synthesis
Ruland, H. and Busser, W. and Otto, H. and Muhler, M.
CHEMIE-INGENIEUR-TECHNIK. Volume: 86 (2014) - 2014 • 2447
Magnetic field templated patterning of the soft magnetic alloy CoFe
Karnbach, F. and Uhlemann, M. and Gebert, A. and Eckert, J. and Tschulik, K.
ELECTROCHIMICA ACTA. Volume: 123 (2014) - 2014 • 2446
Interface effects in NaAlH4-carbon nanocomposites for hydrogen storage
Gao, J. and Ngene, P. and Herrich, M. and Xia, W. and Gutfleisch, O. and Muhler, M. and De Jong, K.P. and De Jongh, P.E.
INTERNATIONAL JOURNAL OF HYDROGEN ENERGY. Volume: 39 (2014) - 2014 • 2445
Molecular-Scale Imaging of Water Near Charged Surfaces
Mehlhorn, M. and Schnur, S. and Groß, A. and Morgenstern, K.
CHEMELECTROCHEM. Volume: 1 (2014) - 2014 • 2444
Iron-treated NiO as a highly transparent p-type protection layer for efficient Si-based photoanodes
Mei, B. and Permyakova, A.A. and Frydendal, R. and Bae, D. and Pedersen, T. and Malacrida, P. and Hansen, O. and Stephens, I.E.L. and Vesborg, P.C.K. and Seger, B. and Chorkendorff, I.
JOURNAL OF PHYSICAL CHEMISTRY LETTERS. Volume: 5 (2014) - 2014 • 2443
Effect of ternary element addition on the corrosion behaviour of NiTi shape memory alloys
Kassab, E. and Neelakantan, L. and Frotscher, M. and Swaminathan, S. and Maaß, B. and Rohwerder, M. and Gomes, J. and Eggeler, G.
MATERIALS AND CORROSION. Volume: 65 (2014) - 2014 • 2442
Protection of p+-n-Si photoanodes by sputter-deposited Ir/IrOx thin films
Mei, B. and Seger, B. and Pedersen, T. and Malizia, M. and Hansen, O. and Chorkendorff, I. and Vesborg, P.C.K.
JOURNAL OF PHYSICAL CHEMISTRY LETTERS. Volume: 5 (2014) - 2014 • 2441
On the physical nature of tribolayers and wear debris after sliding wear in a superalloy/steel tribosystem at 25 and 300°C
Rynio, C. and Hattendorf, H. and Klöwer, J. and Eggeler, G.
WEAR. Volume: 317 (2014) - 2014 • 2440
On the electropolishing of NiTi braided stents - Challenges and solutions
Kassab, E. and Marquardt, A. and Neelakantan, L. and Frotscher, M. and Schreiber, F. and Gries, T. and Jockenhoevel, S. and Gomes, J. and Eggeler, G.
MATERIALWISSENSCHAFT UND WERKSTOFFTECHNIK. Volume: 45 (2014) - 2014 • 2439
Design criteria for stable Pt/C fuel cell catalysts
Meier, J.C. and Galeano, C. and Katsounaros, I. and Witte, J. and Bongard, H.J. and Topalov, A.A. and Baldizzone, C. and Mezzavilla, S. and Schüth, F. and Mayrhofer, K.J.J.
BEILSTEIN JOURNAL OF NANOTECHNOLOGY. Volume: 5 (2014) - 2014 • 2438
The evolution of tribolayers during high temperature sliding wear
Rynio, C. and Hattendorf, H. and Klöwer, J. and Eggeler, G.
WEAR. Volume: 315 (2014) - 2014 • 2437
Physisorption versus chemisorption in inelastic electron tunneling spectroscopy: Mode position, intensity, and spatial distribution
Gawronski, H. and Morgenstern, K.
PHYSICAL REVIEW B - CONDENSED MATTER AND MATERIALS PHYSICS. Volume: 89 (2014) - 2014 • 2436
The Bain library: A Cu-Au buffer template for a continuous variation of lattice parameters in epitaxial films
Kauffmann-Weiss, S. and Hamann, S. and Reichel, L. and Siegel, A. and Alexandrakis, V. and Heller, R. and Schultz, L. and Ludwig, Al. and Fähler, S.
APL MATERIALS. Volume: 2 (2014) - 2014 • 2435
Tribenzotriquinacene receptors for C60 fullerene rotors: Towards C3 symmetrical chiral stators for unidirectionally operating nanoratchets
Bredenkötter, B. and Grzywa, M. and Alaghemandi, M. and Schmid, R. and Herrebout, W. and Bultinck, P. and Volkmer, D.
CHEMISTRY - A EUROPEAN JOURNAL. Volume: 20 (2014) - 2014 • 2434
Indium-tris-guanidinates: A promising class of precursors for water assisted atomic layer deposition of In2O3 thin films
Gebhard, M. and Hellwig, M. and Parala, H. and Xu, K. and Winter, M. and Devi, A.
DALTON TRANSACTIONS. Volume: 43 (2014) - 2014 • 2433
Biochemical component identification by plasmonic improved whispering gallery mode optical resonance based sensor
Saetchnikov, V.A. and Tcherniavskaia, E.A. and Saetchnikov, A.V. and Schweiger, G. and Ostendorf, A.
PROCEEDINGS OF SPIE - THE INTERNATIONAL SOCIETY FOR OPTICAL ENGINEERING. Volume: 9126 (2014) - 2014 • 2432
Experimental, analytical and numerical analysis of the pullout behaviour of steel fibres considering different fibre types, inclinations and concrete strengths
Breitenbücher, R. and Meschke, G. and Song, F. and Zhan, Y.
STRUCTURAL CONCRETE. Volume: 15 (2014) - 2014 • 2431
[FeFe]-hydrogenase models assembled into vesicular structures
Menzel, K. and Apfel, U.-P. and Wolter, N. and Rüger, R. and Alpermann, T. and Steiniger, F. and Gabel, D. and Förster, S. and Weigand, W. and Fahr, A.
JOURNAL OF LIPOSOME RESEARCH. Volume: 24 (2014) - 2014 • 2430
Biochemical component identification by light scattering techniques in whispering gallery mode optical resonance based sensor
Saetchnikov, V.A. and Tcherniavskaia, E.A. and Saetchnikov, A.V. and Schweiger, G. and Ostendorf, A.
PROGRESS IN BIOMEDICAL OPTICS AND IMAGING - PROCEEDINGS OF SPIE. Volume: 8952 (2014) - 2014 • 2429
Influence of casting thickness on the strength and durability of cementitious grouting concrete
Breitenbücher, R. and Benra, M.
BETON- UND STAHLBETONBAU. Volume: 109 (2014) - 2014 • 2428
Reaction products in the combustion of the high energy density storage material lithium with carbon dioxide and nitrogen
Kellermann, R. and Taroata, D. and Schiemann, M. and Eckert, H. and Fischer, P. and Scherer, V. and Hock, R. and Schmid, G.
PREHOSPITAL AND DISASTER MEDICINE. Volume: 1644 (2014) - 2014 • 2427
Plasmonic improvement of microcavity biomedical sensor spectroscopic characteristics
Saetchnikov, V.A. and Tcherniavskaia, E.A. and Saetchnikov, A.V. and Schweiger, G. and Ostendorf, A. and Ghadiri, R.
PROGRESS IN BIOMEDICAL OPTICS AND IMAGING - PROCEEDINGS OF SPIE. Volume: 8957 (2014) - 2014 • 2426
Experimental investigation of the pullout behavior of steel fibers in high-strength concrete
Breitenbücher, R. and Song, F.
BETON- UND STAHLBETONBAU. Volume: 109 (2014) - 2014 • 2425
Reaction products in the combustion of the high energy density storage material lithium with carbon dioxide and nitrogen
Kellermann, R. and Taroata, D. and Schiemann, M. and Eckert, H. and Fischer, P. and Scherer, V. and Hock, R. and Schmid, G.
MATERIALS RESEARCH SOCIETY SYMPOSIUM PROCEEDINGS. Volume: 1644 (2014) - 2014 • 2424
Crack deflection in multi-layered four-point bending samples
Brinckmann, S. and Völker, B. and Dehm, G.
INTERNATIONAL JOURNAL OF FRACTURE. Volume: 190 (2014) - 2014 • 2423
Polarization dynamics in spin-polarized vertical-cavity surface-emitting lasers
Gerhardt, N.C. and Höpfner, H. and Lindemann, M. and Hofmann, M.R.
PROCEEDINGS OF SPIE - THE INTERNATIONAL SOCIETY FOR OPTICAL ENGINEERING. Volume: 9167 (2014) - 2014 • 2422
Redox dynamics of Ni catalysts in CO2 reforming of methane
Mette, K. and Kühl, S. and Tarasov, A. and Düdder, H. and Kähler, K. and Muhler, M. and Schlögl, R. and Behrens, M.
CATALYSIS TODAY. Volume: (2014) - 2014 • 2421
Germany's Energiewende pushes for renewables
Saini, A. and Brick, S. and Schüth, F.
MRS BULLETIN. Volume: 39 (2014) - 2014 • 2420
Strong coupling of an Er3+ -doped YAlO3 crystal to a superconducting resonator
Tkalčec, A. and Probst, S. and Rieger, D. and Rotzinger, H. and Wünsch, S. and Kukharchyk, N. and Wieck, A.D. and Siegel, M. and Ustinov, A.V. and Bushev, P.
PHYSICAL REVIEW B - CONDENSED MATTER AND MATERIALS PHYSICS. Volume: 90 (2014) - 2014 • 2419
The thermodynamic assessment of the Au-In-Ga system
Ghasemi, M. and Sundman, B. and Fries, S.G. and Johansson, J.
JOURNAL OF ALLOYS AND COMPOUNDS. Volume: 600 (2014) - 2014 • 2418
Stable performance of Ni catalysts in the dry reforming of methane at high temperatures for the efficient conversion of CO2 into syngas
Mette, K. and Kühl, S. and Düdder, H. and Kähler, K. and Tarasov, A. and Muhler, M. and Behrens, M.
CHEMCATCHEM. Volume: 6 (2014) - 2014 • 2417
Self-directed localization of ZIF-8 thin film formation by conversion of ZnO nanolayers
Khaletskaya, K. and Turner, S. and Tu, M. and Wannapaiboon, S. and Schneemann, A. and Meyer, R. and Ludwig, Al. and Van Tendeloo, G. and Fischer, R.A.
ADVANCED FUNCTIONAL MATERIALS. Volume: 24 (2014) - 2014 • 2416
Extending the concept of defect chemistry from semiconductor physics to electrochemistry
Todorova, M. and Neugebauer, J.
PHYSICAL REVIEW APPLIED. Volume: 1 (2014) - 2014 • 2415
Electrochemical quantification of iodide ions in synthetic urine using silver nanoparticles: A proof-of-concept
Toh, H.S. and Tschulik, K. and Batchelor-Mcauley, C. and Compton, R.G.
ANALYST. Volume: 139 (2014) - 2014 • 2414
A proof-of-concept - Using pre-created nucleation centres to improve the limit of detection in anodic stripping voltammetry
Toh, H.S. and Batchelor-Mcauley, C. and Tschulik, K. and Damm, C. and Compton, R.G.
SENSORS AND ACTUATORS, B: CHEMICAL. Volume: 193 (2014) - 2014 • 2413
Composition-structure-function diagrams of Ti-Ni-Au thin film shape memory alloys
Buenconsejo, P.J.S. and Ludwig, Al.
ACS COMBINATORIAL SCIENCE. Volume: 16 (2014) - 2014 • 2412
Chemical interactions between silver nanoparticles and thiols: A comparison of mercaptohexanol against cysteine
Toh, H.S. and Batchelor-Mcauley, C. and Tschulik, K. and Compton, R.G.
SCIENCE CHINA CHEMISTRY. Volume: 57 (2014) - 2014 • 2411
On the mechanism that leads to vanishing thermal hysteresis of the B2-R phase transformation in multilayered (TiNi)/(W) shape memory alloy thin films
Buenconsejo, P.J.S. and Zarnetta, R. and Young, M. and Brunken, H. and Mehta, A. and Ludwig, Al.
THIN SOLID FILMS. Volume: 564 (2014) - 2014 • 2410
Designing Heusler nanoprecipitates by elastic misfit stabilization in Fe-Mn maraging steels
Millán, J. and Sandlöbes, S. and Al-Zubi, A. and Hickel, T. and Choi, P. and Neugebauer, J. and Ponge, D. and Raabe, D.
ACTA MATERIALIA. Volume: 76 (2014) - 2014 • 2409
Application of PEDOT-CNT Microelectrodes for Neurotransmitter Sensing
Samba, R. and Fuchsberger, K. and Matiychyn, I. and Epple, S. and Kiesel, L. and Stett, A. and Schuhmann, W. and Stelzle, M.
ELECTROANALYSIS. Volume: 26 (2014) - 2014 • 2408
Atomic-scale analysis of carbon partitioning between martensite and austenite by atom probe tomography and correlative transmission electron microscopy
Toji, Y. and Matsuda, H. and Herbig, M. and Choi, P.-P. and Raabe, D.
ACTA MATERIALIA. Volume: 65 (2014) - 2014 • 2407
Optimal designs for nonlinear regression models with respect to non-informative priors
Burghaus, I. and Dette, H.
JOURNAL OF STATISTICAL PLANNING AND INFERENCE. Volume: 154 (2014) - 2014 • 2406
Synthesis of WO3 nanoblades by the dealloying of glancing angle deposited W-Fe nanocolumnar thin films
Khare, C. and Stepanovich, A. and Buenconsejo, P.J.S. and Ludwig, Al.
NANOTECHNOLOGY. Volume: 25 (2014) - 2014 • 2405
Exceptional size-dependent activity enhancement in the electroreduction of CO2 over Au nanoparticles
Mistry, H. and Reske, R. and Zeng, Z. and Zhao, Z.-J. and Greeley, J. and Strasser, P. and Cuenya, B.R.
JOURNAL OF THE AMERICAN CHEMICAL SOCIETY. Volume: 136 (2014) - 2014 • 2404
Breakdown of the arrhenius law in describing vacancy formation energies: The importance of local anharmonicity revealed by Ab initio thermodynamics
Glensk, A. and Grabowski, B. and Hickel, T. and Neugebauer, J.
PHYSICAL REVIEW X. Volume: 4 (2014) - 2014 • 2403
Shape-dependent catalytic oxidation of 2-butanol over Pt nanoparticles supported on γ-Al2O3
Mistry, H. and Behafarid, F. and Zhou, E. and Ono, L.K. and Zhang, L. and Roldan Cuenya, B.
ACS CATALYSIS. Volume: 4 (2014) - 2014 • 2402
Texture evolution as determined by in situ neutron diffraction during annealing of iron deformed by equal channel angular pressing
Sandim, H.R.Z. and Bolmaro, R.E. and Renzetti, R.A. and Sandim, M.J.R. and Hartwig, K.T. and Vogel, S.C. and Raabe, D.
METALLURGICAL AND MATERIALS TRANSACTIONS A: PHYSICAL METALLURGY AND MATERIALS SCIENCE. Volume: 45 (2014) - 2014 • 2401
A fracture-resistant high-entropy alloy for cryogenic applications
Gludovatz, B. and Hohenwarter, A. and Catoor, D. and Chang, E.H. and George, E.P. and Ritchie, R.O.
SCIENCE. Volume: 345 (2014) - 2014 • 2400
Pressure-dependent effect of hydrogen adsorption on structural and electronic properties of Pt/γ-Al2O3 nanoparticles
Mistry, H. and Behafarid, F. and Bare, S.R. and Roldan Cuenya, B.
CHEMCATCHEM. Volume: 6 (2014) - 2014 • 2399
Ductility improvement of Mg alloys by solid solution: Ab initio modeling, synthesis and mechanical properties
Sandlöbes, S. and Pei, Z. and Friák, M. and Zhu, L.-F. and Wang, F. and Zaefferer, S. and Raabe, D. and Neugebauer, J.
ACTA MATERIALIA. Volume: 70 (2014) - 2014 • 2398
Phase-field modeling for 3D grain growth based on a grain boundary energy database
Kim, H.-K. and Kim, S.G. and Dong, W. and Steinbach, I. and Lee, B.-J.
MODELLING AND SIMULATION IN MATERIALS SCIENCE AND ENGINEERING. Volume: 22 (2014) - 2014 • 2397
A model for high temperature creep of single crystal superalloys based on nonlocal damage and viscoplastic material behavior
Trinh, B.T. and Hackl, K.
CONTINUUM MECHANICS AND THERMODYNAMICS. Volume: 26 (2014) - 2014 • 2396
Catalytic reactions of acetylene: A feedstock for the chemical industry revisited
Trotuş, I.-T. and Zimmermann, T. and Schüth, F.
CHEMICAL REVIEWS. Volume: 114 (2014) - 2014 • 2395
Lattice distortion and stripelike antiferromagnetic order in Ca10 (Pt3As8)(Fe2As2) 5
Sapkota, A. and Tucker, G.S. and Ramazanoglu, M. and Tian, W. and Ni, N. and Cava, R.J. and McQueeney, R.J. and Goldman, A.I. and Kreyssig, A.
PHYSICAL REVIEW B - CONDENSED MATTER AND MATERIALS PHYSICS. Volume: 90 (2014) - 2014 • 2394
High temperature electron spin dynamics in bulk cubic GaN: Nanosecond spin lifetimes far above room-temperature
Buß, J.H. and Schaefer, A. and Schupp, T. and As, D.J. and Hägele, D. and Rudolph, J.
APPLIED PHYSICS LETTERS. Volume: 105 (2014) - 2014 • 2393
Nanoparticle impacts reveal magnetic field induced agglomeration and reduced dissolution rates
Tschulik, K. and Compton, R.G.
PHYSICAL CHEMISTRY CHEMICAL PHYSICS. Volume: 16 (2014) - 2014 • 2392
Photodeposition of copper and chromia on gallium oxide: The role of co-catalysts in photocatalytic water splitting
Busser, G.W. and Mei, B. and Pougin, A. and Strunk, J. and Gutkowski, R. and Schuhmann, W. and Willinger, M.-G. and Schlögl, R. and Muhler, M.
CHEMSUSCHEM. Volume: 7 (2014) - 2014 • 2391
Electrochemical studies of silver nanoparticles: A guide for experimentalists and a perspective
Tschulik, K. and Batchelor-Mcauley, C. and Toh, H.-S. and Stuart, E.J.E. and Compton, R.G.
PHYSICAL CHEMISTRY CHEMICAL PHYSICS. Volume: 16 (2014) - 2014 • 2390
Analytic bond-order potentials for the bcc refractory metals Nb, Ta, Mo and W
Čák, M. and Hammerschmidt, T. and Rogal, J. and Vitek, V. and Drautz, R.
JOURNAL OF PHYSICS CONDENSED MATTER. Volume: 26 (2014) - 2013 • 2389
Ring-disk microelectrodes for simultaneous constant-distance and constant-current mode scanning electrochemical microscopy
Nebel, M. and Neugebauer, S. and Eckhard, K. and Schuhmann, W.
ELECTROCHEMISTRY COMMUNICATIONS. Volume: 27 (2013) - 2013 • 2388
Time-resolved characterization of a filamentary argon discharge at atmospheric pressure in a capillary using emission and absorption spectroscopy
Schröter, S. and Pothiraja, R. and Awakowicz, P. and Bibinov, N. and Böke, M. and Niermann, B. and Winter, J.
JOURNAL OF PHYSICS D: APPLIED PHYSICS. Volume: 46 (2013) - 2013 • 2387
Imine-linked polymer-derived nitrogen-doped microporous carbons with excellent CO2 capture properties
Wang, J. and Senkovska, I. and Oschatz, M. and Lohe, M.R. and Borchardt, L. and Heerwig, A. and Liu, Q. and Kaskel, S.
ACS APPLIED MATERIALS AND INTERFACES. Volume: 5 (2013) - 2013 • 2386
Towards the understanding of sintering phenomena at the nanoscale: Geometric and environmental effects
Behafarid, F. and Roldan Cuenya, B.
TOPICS IN CATALYSIS. Volume: 56 (2013) - 2013 • 2385
Correlation of electronic and magnetic properties of thin polymer layers with cobalt nanoparticles
Kharchenko, A. and Lukashevich, M. and Popok, V. and Khaibullin, R. and Valeev, V. and Bazarov, V. and Petracic, O. and Wieck, A. and Odzhaev, V.
PARTICLE AND PARTICLE SYSTEMS CHARACTERIZATION. Volume: 30 (2013) - 2013 • 2384
Nano- and microcrystal investigations of precipitates, interfaces and strain fields in Ni-Ti-Nb by various TEM techniques
Schryvers, D. and Shi, H. and Martinez, G.T. and Van Aert, S. and Frenzel, J. and Van Humbeeck, J.
MATERIALS SCIENCE FORUM. Volume: 738-739 (2013) - 2013 • 2383
Control of phase coexistence in calcium tantalate composite photocatalysts for highly efficient hydrogen production
Wang, P. and Chen, P. and Kostka, A. and Marschall, R. and Wark, M.
CHEMISTRY OF MATERIALS. Volume: 25 (2013) - 2013 • 2382
Energy substitution: When model selection depends on the focus
Behl, P. and Dette, H. and Frondel, M. and Tauchmann, H.
ENERGY ECONOMICS. Volume: 39 (2013) - 2013 • 2381
Layered WO3/TiO2 nanostructures with enhanced photocurrent densities
Khare, C. and Sliozberg, K. and Meyer, R. and Savan, A. and Schuhmann, W. and Ludwig, Al.
INTERNATIONAL JOURNAL OF HYDROGEN ENERGY. Volume: 38 (2013) - 2013 • 2380
Design and fabrication of a bending rotation fatigue test rig for in situ electrochemical analysis during fatigue testing of NiTi shape memory alloy wires
Neelakantan, L. and Zglinski, J.K. and Frotscher, M. and Eggeler, G.
REVIEW OF SCIENTIFIC INSTRUMENTS. Volume: 84 (2013) - 2013 • 2379
Colloidal deposition as method to study the influence of the support on the activity of gold catalysts in CO-oxidation
Schüth, F.
PHYSICA STATUS SOLIDI (B) BASIC RESEARCH. Volume: 250 (2013) - 2013 • 2378
Preparation of cubic ordered mesoporous silicon carbide monoliths by pressure assisted preceramic polymer nanocasting
Wang, J. and Oschatz, M. and Biemelt, T. and Lohe, M.R. and Borchardt, L. and Kaskel, S.
MICROPOROUS AND MESOPOROUS MATERIALS. Volume: 168 (2013) - 2013 • 2377
Thickness dependent exchange bias in martensitic epitaxial Ni-Mn-Sn thin films
Behler, A. and Teichert, N. and Dutta, B. and Waske, A. and Hickel, T. and Auge, A. and Hütten, A. and Eckert, J.
AIP ADVANCES. Volume: 3 (2013) - 2013 • 2376
A genetic strategy to identify targets for the development of drugs that prevent bacterial persistence
Kim, J.-H. and O'Brien, K.M. and Sharma, R. and Boshoff, H.I.M. and Rehren, G. and Chakraborty, S. and Wallach, J.B. and Monteleone, M. and Wilson, D.J. and Aldrich, C.C. and Barry, C.E. and Rhee, K.Y. and Ehrt, S. and Schnappinger, D.
PROCEEDINGS OF THE NATIONAL ACADEMY OF SCIENCES OF THE UNITED STATES OF AMERICA. Volume: 110 (2013) - 2013 • 2375
Intrinsic nitrogen-doped CVD-grown TiO2 thin films from all-N-coordinated Ti precursors for photoelectrochemical applications
Kim, S.J. and Xu, K. and Parala, H. and Beranek, R. and Bledowski, M. and Sliozberg, K. and Becker, H.-W. and Rogalla, D. and Barreca, D. and Maccato, C. and Sada, C. and Schuhmann, W. and Fischer, R.A. and Devi, A.
CHEMICAL VAPOR DEPOSITION. Volume: 19 (2013) - 2013 • 2374
Thermodynamics of carbon solubility in ferrite and vacancy formation in cementite in strained pearlite
Nematollahi, G.A. and Von Pezold, J. and Neugebauer, J. and Raabe, D.
ACTA MATERIALIA. Volume: 61 (2013) - 2013 • 2373
Functionally graded vacuum plasma sprayed and magnetron sputtered tungsten/EUROFER97 interlayers for joints in helium-cooled divertor components
Weber, T. and Stüber, M. and Ulrich, S. and Vaßen, R. and Basuki, W.W. and Lohmiller, J. and Sittel, W. and Aktaa, J.
JOURNAL OF NUCLEAR MATERIALS. Volume: 436 (2013) - 2013 • 2372
Spin polarization of Ru in superconducting Ba(Fe0.795Ru 0.205)2As2 studied by x-ray resonant magnetic scattering
Kim, M.G. and Soh, J. and Lang, J. and Dean, M.P.M. and Thaler, A. and Bud'Ko, S.L. and Canfield, P.C. and Bourret-Courchesne, E. and Kreyssig, A. and Goldman, A.I. and Birgeneau, R.J.
PHYSICAL REVIEW B - CONDENSED MATTER AND MATERIALS PHYSICS. Volume: 88 (2013) - 2013 • 2371
Supervision and control of medical sterilization processes utilizing the multipole resonance probe
Schulz, C. and Styrnoll, T. and Awakowicz, P. and Rolfes, I.
2013 IEEE MTT-S INTERNATIONAL MICROWAVE WORKSHOP SERIES ON RF AND WIRELESS TECHNOLOGIES FOR BIOMEDICAL AND HEALTHCARE APPLICATIONS, IMWS-BIO 2013 - PROCEEDINGS. Volume: (2013) - 2013 • 2370
The effect of Al-doping on ZnO nanoparticles applied as catalyst support
Behrens, M. and Lolli, G. and Muratova, N. and Kasatkin, I. and Hävecker, M. and D'Alnoncourt, R.N. and Storcheva, O. and Köhler, K. and Muhler, M. and Schlögl, R.
PHYSICAL CHEMISTRY CHEMICAL PHYSICS. Volume: 15 (2013) - 2013 • 2369
Magnonlike dispersion of spin resonance in Ni-doped BaFe2As 2
Kim, M.G. and Tucker, G.S. and Pratt, D.K. and Ran, S. and Thaler, A. and Christianson, A.D. and Marty, K. and Calder, S. and Podlesnyak, A. and Bud'Ko, S.L. and Canfield, P.C. and Kreyssig, A. and Goldman, A.I. and McQueeney, R.J.
PHYSICAL REVIEW LETTERS. Volume: 110 (2013) - 2013 • 2368
Optimal actuator and sensor placement based on balanced reduced models
Nestorović, T. and Trajkov, M.
MECHANICAL SYSTEMS AND SIGNAL PROCESSING. Volume: 36 (2013) - 2013 • 2367
The multipole resonance probe: Evolution of a plasma sensor
Schulz, C. and Rolfes, I. and Styrnoll, T. and Awakowicz, P. and Oberrath, J. and Mussenbrock, T. and Brinkmann, R.P. and Storch, R. and Musch, T.
PROCEEDINGS OF IEEE SENSORS. Volume: (2013) - 2013 • 2366
Compressive behavior of Ti3AlC2 and Ti 3Al0.8Sn0.2C2 MAX phases at room temperature
Bei, G.-P. and Laplanche, G. and Gauthier-Brunet, V. and Bonneville, J. and Dubois, S.
JOURNAL OF THE AMERICAN CERAMIC SOCIETY. Volume: 96 (2013) - 2013 • 2365
Density functional theory in materials science
Neugebauer, J. and Hickel, T.
WILEY INTERDISCIPLINARY REVIEWS: COMPUTATIONAL MOLECULAR SCIENCE. Volume: 3 (2013) - 2013 • 2364
Investigation of interactions between plasmas and RF-diagnostics: Challenges of complex 3D-electromagnetic field simulations
Schulz, C. and Rolfes, I.
IEEE ANTENNAS AND PROPAGATION SOCIETY, AP-S INTERNATIONAL SYMPOSIUM (DIGEST). Volume: (2013) - 2013 • 2363
Cu2MnAl thin films grown onto sapphire and MgO substrates: Exchange stiffness and magnetic anisotropy
Belmeguenai, M. and Tuzcuoglu, H. and Chérif, S.M. and Westerholt, K. and Chauveau, T. and Mazaleyrat, F. and Moch, P.
PHYSICA STATUS SOLIDI (A) APPLICATIONS AND MATERIALS SCIENCE. Volume: 210 (2013) - 2013 • 2362
Investigating the influence of sweep gas on CO2/N2 membranes for post-combustion capture
Franz, J. and Schiebahn, S. and Zhao, L. and Riensche, E. and Scherer, V. and Stolten, D.
INTERNATIONAL JOURNAL OF GREENHOUSE GAS CONTROL. Volume: 13 (2013) - 2013 • 2361
Performance of silver nanoparticles in the catalysis of the oxygen reduction reaction in neutral media: Efficiency limitation due to hydrogen peroxide escape
Neumann, C.C.M. and Laborda, E. and Tschulik, K. and Ward, K.R. and Compton, R.G.
NANO RESEARCH. Volume: 6 (2013) - 2013 • 2360
Ferromagnetic resonance, transverse bias initial inverse susceptibility and torque studies of magnetic properties of Co2MnSi thin films
Belmeguenai, M. and Tuzcuoglu, H. and Berling, D. and Chérif, S.M. and Roussigné, Y. and Devolder, T. and Westerholt, K.
EPJ WEB OF CONFERENCES. Volume: 40 (2013) - 2013 • 2359
Methanol synthesis on ZnO from molecular dynamics
Frenzel, J. and Kiss, J. and Nair, N. N. and Meyer, B. and Marx, D.
PHYSICA STATUS SOLIDI B-BASIC SOLID STATE PHYSICS. Volume: 250 (2013) - 2013 • 2358
A multi directional dielectric lens approach for antennas used in industrial RADAR applications
Schulz, C. and Baer, C. and Pohl, N. and Musch, T. and Will, B. and Rolfes, I.
2013 INTERNATIONAL WORKSHOP ON ANTENNA TECHNOLOGY, IWAT 2013. Volume: (2013) - 2013 • 2357
A study of electrode temperature lowering in Dy-containing ceramic metal halide lamps: II. An investigation of the converse effect of Tl and/or Na additives
Westermeier, M. and Ruhrmann, C. and Bergner, A. and Denissen, C. and Suijker, J. and Awakowicz, P. and Mentel, J.
JOURNAL OF PHYSICS D: APPLIED PHYSICS. Volume: 46 (2013) - 2013 • 2356
Magnetization dynamics in Co2MnGe/Al2O3/Co tunnel junctions grown on different substrates
Belmeguenai, M. and Tuzcuoglu, H. and Zighem, F. and Chérif, S.-M. and Roussigné, Y. and Westerholt, K. and Moch, P. and El Bahoui, A. and Genevois, C. and Fnidiki, A.
SENSOR LETTERS. Volume: 11 (2013) - 2013 • 2355
A study of electrode temperature lowering in Dy-containing ceramic metal halide lamps: I. the effect of mixtures of Dy, Tl and Na compared with pure Dy
Westermeier, M. and Ruhrmann, C. and Bergner, A. and Denissen, C. and Suijker, J. and Awakowicz, P. and Mentel, J.
JOURNAL OF PHYSICS D: APPLIED PHYSICS. Volume: 46 (2013) - 2013 • 2354
Ab Initio Guided Design of Materials
Friák, M. and Raabe, D. and Neugebauer, J.
STRUCTURAL MATERIALS AND PROCESSES IN TRANSPORTATION. Volume: (2013) - 2013 • 2353
Methanol synthesis on ZnO(000(1)over-bar). II. Structure, energetics, and vibrational signature of reaction intermediates
Kiss, J. and Frenzel, J. and Meyer, B. and Marx, D.
JOURNAL OF CHEMICAL PHYSICS. Volume: 139 (2013) - 2013 • 2352
Identification of a fault zone ahead of the tunnel excavation face using the extended Kalman filter
Nguyen, L.T. and Datcheva, M. and Nestorović, T.
MECHANICS RESEARCH COMMUNICATIONS. Volume: 53 (2013) - 2013 • 2351
Aharonov-Bohm rings with strong spin-orbit interaction: The role of sample-specific properties
Nichele, F. and Komijani, Y. and Hennel, S. and Gerl, C. and Wegscheider, W. and Reuter, D. and Wieck, A.D. and Ihn, T. and Ensslin, K.
NEW JOURNAL OF PHYSICS. Volume: 15 (2013) - 2013 • 2350
Pt-Cu alloys as catalysts for the oxygen reduction reaction - A thin-film study of activity and stability
Schuppert, A.K. and Topalov, A.A. and Savan, A. and Ludwig, Al. and Mayrhofer, K.J.J.
ECS TRANSACTIONS. Volume: 58 (2013) - 2013 • 2349
Influence of recombination center interaction on the photoluminescence of AlGaAs/GaAs heterostructures
Schuster, J. and Kim, T.Y. and Batke, E. and Reuter, D. and Wieck, A.D.
SEMICONDUCTOR SCIENCE AND TECHNOLOGY. Volume: 28 (2013) - 2013 • 2348
Photoluminescence of inversion electrons with carbon acceptors in a single modulation-doped AlxGa1-xAs/GaAs heterostructure
Schuster, J. and Kim, T.Y. and Batke, E. and Reuter, D. and Wieck, A.D.
PHYSICAL REVIEW B - CONDENSED MATTER AND MATERIALS PHYSICS. Volume: 87 (2013) - 2013 • 2347
A synthetic calibration technique for broadband applications using focusing antenna setups
Will, B. and Rolfes, I.
IEEE ANTENNAS AND PROPAGATION SOCIETY, AP-S INTERNATIONAL SYMPOSIUM (DIGEST). Volume: (2013) - 2013 • 2346
Nondestructive real-time measurement of charge and spin dynamics of photoelectrons in a double quantum dot
Fujita, T. and Kiyama, H. and Morimoto, K. and Teraoka, S. and Allison, G. and Ludwig, Ar. and Wieck, A.D. and Oiwa, A. and Tarucha, S.
PHYSICAL REVIEW LETTERS. Volume: 110 (2013) - 2013 • 2345
Comparative study of moisture measurements by time domain transmissometry
Will, B. and Rolfes, I.
PROCEEDINGS OF IEEE SENSORS. Volume: (2013) - 2013 • 2344
Investigation of the influence of reflection on the attenuation of cancellous bone
Klinge, S. and Hackl, K. and Gilbert, R.P.
BIOMECHANICS AND MODELING IN MECHANOBIOLOGY. Volume: 12 (2013) - 2013 • 2343
Atom probe study of Cu2ZnSnSe4 thin-films prepared by co-evaporation and post-deposition annealing
Schwarz, T. and Cojocaru-Mirédin, O. and Choi, P. and Mousel, M. and Redinger, A. and Siebentritt, S. and Raabe, D.
APPLIED PHYSICS LETTERS. Volume: 102 (2013) - 2013 • 2342
Target implantation and redeposition processes during high-power impulse magnetron sputtering of aluminum
Will, A. and de los Arcos, T. and Corbella, C. and Hecimovic, A. and Machura, P. D. and Winter, J. and von Keudell, A.
JOURNAL OF PHYSICS D-APPLIED PHYSICS. Volume: 46 (2013) - 2013 • 2341
High-power InP quantum dot based semiconductor disk laser exceeding 1.3 W
Schwarzback, T. and Bek, R. and Hargart, F. and Kessler, C. A. and Kahle, H. and Koroknay, E. and Jetter, M. and Michler, P.
APPLIED PHYSICS LETTERS. Volume: 102 (2013) - 2013 • 2340
Tensile properties of high- and medium-entropy alloys
Gali, A. and George, E.P.
INTERMETALLICS. Volume: 39 (2013) - 2013 • 2339
FEM modelling of a coaxial three-electrode test cell for electrochemical impedance spectroscopy in lithium ion batteries
Klink, S. and Höche, D. and La Mantia, F. and Schuhmann, W.
JOURNAL OF POWER SOURCES. Volume: 240 (2013) - 2013 • 2338
The influence of heat treatment and resulting microstructures on the thermophysical properties of martensitic steels
Wilzer, J. and Lüdtke, F. and Weber, S. and Theisen, W.
JOURNAL OF MATERIALS SCIENCE. Volume: 48 (2013) - 2013 • 2337
Ab initio study of boron in α-iron: Migration barriers and interaction with point defects
Bialon, A.F. and Hammerschmidt, T. and Drautz, R.
PHYSICAL REVIEW B - CONDENSED MATTER AND MATERIALS PHYSICS. Volume: 87 (2013) - 2013 • 2336
A phenomenological model for the description of rotating spokes in HiPIMS discharges
Gallian, S. and Hitchon, W.N.G. and Eremin, D. and Mussenbrock, T. and Brinkmann, R.P.
PLASMA SCIENCE AND TECHNOLOGY. Volume: 22 (2013) - 2013 • 2335
Scaling of the Kondo zero-bias peak in a hole quantum dot at finite temperatures
Klochan, O. and Micolich, A.P. and Hamilton, A.R. and Reuter, D. and Wieck, A.D. and Reininghaus, F. and Pletyukhov, M. and Schoeller, H.
PHYSICAL REVIEW B - CONDENSED MATTER AND MATERIALS PHYSICS. Volume: 87 (2013) - 2013 • 2334
Vibrational spectroscopic studies on pure and metal-covered metal oxide surfaces
Noei, H. and Jin, L. and Qiu, H. and Xu, M. and Gao, Y. and Zhao, J. and Kauer, M. and Wöll, C. and Muhler, M. and Wang, Y.
PHYSICA STATUS SOLIDI (B) BASIC RESEARCH. Volume: 250 (2013) - 2013 • 2333
Multiple frequency capacitively coupled plasmas as a new technology for sputter processes
Bienholz, S. and Bibinov, N. and Awakowicz, P.
JOURNAL OF PHYSICS D: APPLIED PHYSICS. Volume: 46 (2013) - 2013 • 2332
Observation of the Kondo effect in a spin-3/2 hole quantum dot
Klochan, O. and Micolich, A.P. and Hamilton, A.R. and Trunov, K. and Reuter, D. and Wieck, A.D.
AIP CONFERENCE PROCEEDINGS. Volume: 1566 (2013) - 2013 • 2331
CO adsorption on a mixed-valence ruthenium metal-organic framework studied by UHV-FTIR spectroscopy and DFT calculations
Noei, H. and Kozachuk, O. and Amirjalayer, S. and Bureekaew, S. and Kauer, M. and Schmid, R. and Marler, B. and Muhler, M. and Fischer, R.A. and Wang, Y.
JOURNAL OF PHYSICAL CHEMISTRY C. Volume: 117 (2013) - 2013 • 2330
Diffusive and massive phase transformations in Ti-Al-Nb alloys-Modelling and experiments
Gamsjäger, E. and Liu, Y. and Rester, M. and Puschnig, P. and Draxl, C. and Clemens, H. and Dehm, G. and Fischer, F.D.
INTERMETALLICS. Volume: 38 (2013) - 2013 • 2329
Semimetal-insulator transition on the surface of a topological insulator with in-plane magnetization
Nogueira, F.S. and Eremin, I.
PHYSICAL REVIEW B - CONDENSED MATTER AND MATERIALS PHYSICS. Volume: 88 (2013) - 2013 • 2328
H-chondrite parent asteroid: A multistage cooling, fragmentation and re-accretion history constrained by thermometric studies, diffusion kinetic modeling and geochronological data
Ganguly, J. and Tirone, M. and Chakraborty, S. and Domanik, K.
GEOCHIMICA ET COSMOCHIMICA ACTA. Volume: 105 (2013) - 2013 • 2327
Surface morphology and atomic structure of thin layers of Fe3Si on GaAs(001) and their magnetic properties
Noor, S. and Barsukov, I. and Özkan, M.S. and Elbers, L. and Melnichak, N. and Lindner, J. and Farle, M. and Köhler, U.
JOURNAL OF APPLIED PHYSICS. Volume: 113 (2013) - 2013 • 2326
Amperometric sensing - Bioelectroanalysis
Seeber, R. and Schuhmann, W. and Terzi, F. and Zanardi, C. and Plumere, N. and Gebala, M.
ANALYTICAL AND BIOANALYTICAL CHEMISTRY. Volume: 405 (2013) - 2013 • 2325
Force-induced mechanical response of molecule-metal interfaces: Molecular nanomechanics of propanethiolate self-assembled monolayers on Au(111)
Seema, P. and Behler, J. and Marx, D.
PHYSICAL CHEMISTRY CHEMICAL PHYSICS. Volume: 15 (2013) - 2013 • 2324
Kinetic and thermodynamic hysteresis imposed by intercalation of proflavine in ferrocene-modified double-stranded DNA
Gebala, M. and La Mantia, F. and Schuhmann, W.
CHEMPHYSCHEM. Volume: 14 (2013) - 2013 • 2323
Asymmetric superconducting spin valves based on the Fe/V layer system grown on MgO(100)
Nowak, G. and Westerholt, K. and Zabel, H.
SUPERCONDUCTOR SCIENCE AND TECHNOLOGY. Volume: 26 (2013) - 2013 • 2322
Adsorption of methanethiolate and atomic sulfur at the Cu(111) surface: A computational study
Seema, P. and Behler, J. and Marx, D.
JOURNAL OF PHYSICAL CHEMISTRY C. Volume: 117 (2013) - 2013 • 2321
Influence of fiber alignment on creep in directionally solidified NiAl-10Mo in-situ composites
Seemüller, C. and Heilmaier, M. and Haenschke, T. and Bei, H. and Dlouhy, A. and George, E.P.
INTERMETALLICS. Volume: 35 (2013) - 2013 • 2320
Ultrafast spin-polarized vertical-cavity surface-emitting lasers
Gerhardt, N.C. and Höpfner, H. and Lindemann, M. and Li, M. and Jähme, H. and Ackemann, T. and Hofmann, M.R.
PROCEEDINGS OF SPIE - THE INTERNATIONAL SOCIETY FOR OPTICAL ENGINEERING. Volume: 8813 (2013) - 2013 • 2319
The thermally induced interaction of Cu and Au with ZnO single crystal surfaces
Köhler, U. and Kroll, M. and Löber, T. and Birkner, A. and Schott, V. and Wöll, C.
PHYSICA STATUS SOLIDI (B) BASIC RESEARCH. Volume: 250 (2013) - 2013 • 2318
Dynamic nuclear polarization and Hanle effect in (In,Ga)As/GaAs quantum dots. Role of nuclear spin fluctuations
Gerlovin, I.Ya. and Cherbunin, R.V. and Ignatiev, I.V. and Kuznetsova, M.S. and Verbin, S.Yu. and Flisinski, K. and Reuter, D. and Wieck, A.D. and Yakovlev, D.R. and Bayer, M.
AIP CONFERENCE PROCEEDINGS. Volume: 1566 (2013) - 2013 • 2317
Optically generated sub-100 nm structures for biomedical and technical applications
König, K. and Ostendorf, A.
PHYSICS PROCEDIA. Volume: 41 (2013) - 2013 • 2316
Analytic bond-order potential expansion of recursion-based methods
Seiser, B. and Pettifor, D.G. and Drautz, R.
PHYSICAL REVIEW B - CONDENSED MATTER AND MATERIALS PHYSICS. Volume: 87 (2013) - 2013 • 2315
Optical velocity measurements of electrolytic boundary layer flows influenced by magnetic fields
König, J. and Neumann, M. and Mühlenhoff, S. and Tschulik, K. and Albrecht, T. and Eckert, K. and Uhlemann, M. and Weier, T. and Büttner, L. and Czarske, J.
EUROPEAN PHYSICAL JOURNAL: SPECIAL TOPICS. Volume: 220 (2013) - 2013 • 2314
Direct evidence for the formation of ordered carbides in a ferrite-based low-density Fe-Mn-Al-C alloy studied by transmission electron microscopy and atom probe tomography
Seol, J.-B. and Raabe, D. and Choi, P. and Park, H.-S. and Kwak, J.-H. and Park, C.-G.
SCRIPTA MATERIALIA. Volume: 68 (2013) - 2013 • 2313
High-resolution mass spectrometer for liquid metal ion sources
Wortmann, M. and Ludwig, Ar. and Meijer, J. and Reuter, D. and Wieck, A.D.
REVIEW OF SCIENTIFIC INSTRUMENTS. Volume: 84 (2013) - 2013 • 2312
Analysis of the electrolyte convection inside the concentration boundary layer during structured electrodeposition of copper in high magnetic gradient fields
König, J. and Tschulik, K. and Büttner, L. and Uhlemann, M. and Czarske, J.
ANALYTICAL CHEMISTRY. Volume: 85 (2013) - 2013 • 2311
Thermodynamic modeling of chromium: Strong and weak magnetic coupling
Körmann, F. and Grabowski, B. and Söderlind, P. and Palumbo, M. and Fries, S.G. and Hickel, T. and Neugebauer, J.
JOURNAL OF PHYSICS CONDENSED MATTER. Volume: 25 (2013) - 2013 • 2310
Coupling osmium complexes to epoxy-functionalised polymers to provide mediated enzyme electrodes for glucose oxidation
Ó Conghaile, P. and Pöller, S. and MacAodha, D. and Schuhmann, W. and Leech, D.
BIOSENSORS AND BIOELECTRONICS. Volume: 43 (2013) - 2013 • 2309
Extreme optical properties tuned through phase substitution in a structurally optimized biological photonic polycrystal
Wu, X. and Erbe, A. and Raabe, D. and Fabritius, H.-O.
ADVANCED FUNCTIONAL MATERIALS. Volume: 23 (2013) - 2013 • 2308
Counting statistics of hole transfer in a p-type GaAs quantum dot with dense excitation spectrum
Komijani, Y. and Choi, T. and Nichele, F. and Ensslin, K. and Ihn, T. and Reuter, D. and Wieck, A.D.
PHYSICAL REVIEW B - CONDENSED MATTER AND MATERIALS PHYSICS. Volume: 88 (2013) - 2013 • 2307
Origins of conductance anomalies in a p-type GaAs quantum point contact
Komijani, Y. and Csontos, M. and Ihn, T. and Ensslin, K. and Meir, Y. and Reuter, D. and Wieck, A.D.
PHYSICAL REVIEW B - CONDENSED MATTER AND MATERIALS PHYSICS. Volume: 87 (2013) - 2013 • 2306
Anisotropic Zeeman shift in p-type GaAs quantum point contacts
Komijani, Y. and Csontos, M. and Shorubalko, I. and Zülicke, U. and Ihn, T. and Ensslin, K. and Reuter, D. and Wieck, A.D.
EPL. Volume: 102 (2013) - 2013 • 2305
A cryogenically flexible covalent organic framework for efficient hydrogen isotope separation by quantum sieving
Oh, H. and Kalidindi, S.B. and Um, Y. and Bureekaew, S. and Schmid, R. and Fischer, R.A. and Hirscher, M.
ANGEWANDTE CHEMIE - INTERNATIONAL EDITION. Volume: 52 (2013) - 2013 • 2304
Creation of surface defects on carbon nanofibers by steam treatment
Shao, Z. and Pang, M. and Xia, W. and Muhler, M. and Liang, C.
JOURNAL OF ENERGY CHEMISTRY. Volume: 22 (2013) - 2013 • 2303
On the annealing mechanism of AuGe/Ni/Au ohmic contacts to a two-dimensional electron gas in GaAs/AlxGa1-xAs heterostructures
Koop, E.J. and Iqbal, M.J. and Limbach, F. and Boute, M. and Van Wees, B.J. and Reuter, D. and Wieck, A.D. and Kooi, B.J. and Van Der Wal, C.H.
SEMICONDUCTOR SCIENCE AND TECHNOLOGY. Volume: 28 (2013) - 2013 • 2302
Characteristics of the stress-induced formation of R-phase in ultrafine-grained NiTi shape memory wire
Olbricht, J. and Yawny, A. and Pelegrina, J.L. and Eggeler, G. and Yardley, V.A.
JOURNAL OF ALLOYS AND COMPOUNDS. Volume: 579 (2013) - 2013 • 2301
Optimization of a membraneless glucose/oxygen enzymatic fuel cell based on a bioanode with high coulombic efficiency and current density
Shao, M. and Zafar, M.N. and Falk, M. and Ludwig, R. and Sygmund, C. and Peterbauer, C.K. and Guschin, D.A. and MacAodha, D. and Conghaile, P.Ó. and Leech, D. and Toscano, M.D. and Shleev, S. and Schuhmann, W. and Gorton, L.
CHEMPHYSCHEM. Volume: 14 (2013) - 2013 • 2300
Slippery liquid-infused porous surfaces showing marine antibiofouling properties
Xiao, L. and Li, J. and Mieszkin, S. and Di Fino, A. and Clare, A.S. and Callow, M.E. and Callow, J.A. and Grunze, M. and Rosenhahn, A. and Levkin, P.A.
ACS APPLIED MATERIALS AND INTERFACES. Volume: 5 (2013) - 2013 • 2299
Spin-orbit coupling in Fe-based superconductors
Korshunov, M.M. and Togushova, Y.N. and Eremin, I. and Hirschfeld, P.J.
JOURNAL OF SUPERCONDUCTIVITY AND NOVEL MAGNETISM. Volume: 26 (2013) - 2013 • 2298
Abnormal grain growth in ferritic-martensitic Eurofer-97 steel
de Oliveira, V.B. and Padilha, A.F. and Möslang, A. and Raabe, D. and Zschommler Sandim, H.R.
MATERIALS SCIENCE FORUM. Volume: 753 (2013) - 2013 • 2297
A low-potential glucose biofuel cell anode based on a toluidine blue modified redox polymer and the flavodehydrogenase domain of cellobiose dehydrogenase
Shao, M. and Pöller, S. and Sygmund, C. and Ludwig, R. and Schuhmann, W.
ELECTROCHEMISTRY COMMUNICATIONS. Volume: 29 (2013) - 2013 • 2296
Hot embossed microtopographic gradients reveal morphological cues that guide the settlement of zoospores
Xiao, L. and Thompson, S.E.M. and Röhrig, M. and Callow, M.E. and Callow, J.A. and Grunze, M. and Rosenhahn, A.
LANGMUIR. Volume: 29 (2013) - 2013 • 2295
Zr(NEtMe)2(guan-NEtMe2)2] as a novel atomic layer deposition precursor: ZrO2 film growth and mechanistic studies
Blanquart, T. and Niinistö, J. and Aslam, N. and Banerjee, M. and Tomczak, Y. and Gavagnin, M. and Longo, V. and Puukilainen, E. and Wanzenboeck, H.D. and Kessels, W.M.M. and Devi, A. and Hoffmann-Eifert, S. and Ritala, M. and Leskelä, M.
CHEMISTRY OF MATERIALS. Volume: 25 (2013) - 2013 • 2294
Three-orbital model for Fe-pnictides
Korshunov, M.M. and Togushova, Y.N. and Eremin, I.
JOURNAL OF SUPERCONDUCTIVITY AND NOVEL MAGNETISM. Volume: 26 (2013) - 2013 • 2293
Nano-gold diggers: Au-Assisted SiO2-decomposition and desorption in supported nanocatalysts
Ono, L.K. and Behafarid, F. and Cuenya, B.R.
ACS NANO. Volume: 7 (2013) - 2013 • 2292
Mutual enhancement of the current density and the coulombic efficiency for a bioanode by entrapping bi-enzymes with Os-complex modified electrodeposition paints
Shao, M. and Nadeem Zafar, M. and Sygmund, C. and Guschin, D.A. and Ludwig, R. and Peterbauer, C.K. and Schuhmann, W. and Gorton, L.
BIOSENSORS AND BIOELECTRONICS. Volume: 40 (2013) - 2013 • 2291
Influence of water on the initial growth rate of carbon nanotubes from ethylene over a cobalt-based catalyst
Xie, K. and Muhler, M. and Xia, W.
INDUSTRIAL AND ENGINEERING CHEMISTRY RESEARCH. Volume: 52 (2013) - 2013 • 2290
High-throughput study of the structural stability and thermoelectric properties of transition metal silicides
Opahle, I. and Parma, A. and McEniry, E.J. and Drautz, R. and Madsen, G.K.H.
NEW JOURNAL OF PHYSICS. Volume: 15 (2013) - 2013 • 2289
Combination of a photosystem 1-based photocathode and a photosystem 2-based photoanode to a z-scheme mimic for biophotovoltaic applications
Kothe, T. and Plumeré, N. and Badura, A. and Nowaczyk, M.M. and Guschin, D.A. and Rögner, M. and Schuhmann, W.
ANGEWANDTE CHEMIE - INTERNATIONAL EDITION. Volume: 52 (2013) - 2013 • 2288
Compact unfocused antenna setup for X-band free-space dielectric measurements based on line-network-network calibration method
Orlob, C. and Reinecke, T. and Denicke, E. and Geck, B. and Rolfes, I.
IEEE TRANSACTIONS ON INSTRUMENTATION AND MEASUREMENT. Volume: 62 (2013) - 2013 • 2287
Effect of Sn surface states on the photocatalytic activity of anatase TiO2
Oropeza, F.E. and Mei, B. and Sinev, I. and Becerikli, A.E. and Muhler, M. and Strunk, J.
APPLIED CATALYSIS B: ENVIRONMENTAL. Volume: 140-141 (2013) - 2013 • 2286
Relaxor behavior of ferroelectric Ca0.22Sr0.12Ba 0.66Nb2O6
Shekhar Pandey, C. and Schreuer, J. and Burianek, M. and Mühlberg, M.
APPLIED PHYSICS LETTERS. Volume: 102 (2013) - 2013 • 2285
Atomic layer deposition of Er2O3 thin films from Er tris-guanidinate and water: Process optimization, film analysis and electrical properties
Xu, K. and Chaudhuri, A.R. and Parala, H. and Schwendt, D. and Arcos, T.D.L. and Osten, H.J. and Devi, A.
JOURNAL OF MATERIALS CHEMISTRY C. Volume: 1 (2013) - 2013 • 2284
At-line measurement of lactose in dairy-processing plants
Glithero, N. and Clark, C. and Gorton, L. and Schuhmann, W. and Pasco, N.
ANALYTICAL AND BIOANALYTICAL CHEMISTRY. Volume: 405 (2013) - 2013 • 2283
Hydrogen-assisted failure in a twinning-induced plasticity steel studied under in situ hydrogen charging by electron channeling contrast imaging
Koyama, M. and Akiyama, E. and Tsuzaki, K. and Raabe, D.
ACTA MATERIALIA. Volume: 61 (2013) - 2013 • 2282
Textural characterization of micro- and mesoporous carbons using combined gas adsorption and n -nonane preadsorption
Oschatz, M. and Borchardt, L. and Rico-Francés, S. and Rodríguez-Reinoso, F. and Kaskel, S. and Silvestre-Albero, J.
LANGMUIR. Volume: 29 (2013) - 2013 • 2281
Em characterization of precipitates in as-cast and annealed Ni45.5Ti45.5Nb9 shape memory alloys
Shi, H. and Frenzel, J. and Schryvers, D.
MATERIALS SCIENCE FORUM. Volume: 738-739 (2013) - 2013 • 2280
A solid-solution approach to mixed-metal metal-organic frameworks - Detailed characterization of local structures, defects and breathing behaviour of Al/V frameworks
Kozachuk, O. and Meilikhov, M. and Yusenko, K. and Schneemann, A. and Jee, B. and Kuttatheyil, A.V. and Bertmer, M. and Sternemann, C. and Pöppl, A. and Fischer, R.A.
EUROPEAN JOURNAL OF INORGANIC CHEMISTRY. Volume: (2013) - 2013 • 2279
A new route for the preparation of mesoporous carbon materials with high performance in lithium–sulphur battery cathodes
Oschatz, M. and Thieme, S. and Borchardt, L. and Lohe, M.R. and Biemelt, T. and Brückner, J. and Althues, H. and Kaskel, S.
CHEMICAL COMMUNICATIONS. Volume: 49 (2013) - 2013 • 2278
Re effects on phase stability and mechanical properties of Mo SS+Mo3Si+Mo5SiB2 alloys
Yang, Y. and Bei, H. and Tiley, J. and George, E.P.
JOURNAL OF ALLOYS AND COMPOUNDS. Volume: 556 (2013) - 2013 • 2277
Continuum and kinetic simulations of the neutral gas flow in an industrial physical vapor deposition reactor
Bobzin, K. and Brinkmann, R.P. and Mussenbrock, T. and Bagcivan, N. and Brugnara, R.H. and Schäfer, M. and Trieschmann, J.
SURFACE AND COATINGS TECHNOLOGY. Volume: 237 (2013) - 2013 • 2276
Quantitative phase analysis through scattering media by depth-filtered digital holography
Goebel, S. and Jaedicke, V. and Koukourakis, N. and Wiethoff, H. and Adinda-Ougba, A. and Gerhardt, N.C. and Welp, H. and Hofmann, M.R.
PROGRESS IN BIOMEDICAL OPTICS AND IMAGING - PROCEEDINGS OF SPIE. Volume: 8589 (2013) - 2013 • 2275
Carbon dioxide activated carbide-derived carbon monoliths as high performance adsorbents
Oschatz, M. and Borchardt, L. and Senkovska, I. and Klein, N. and Leistner, M. and Kaskel, S.
CARBON. Volume: 56 (2013) - 2013 • 2274
Kinetic simulation of the sheath dynamics in the intermediate radio frequency regime
Shihab, M. and Elgendy, A.T. and Korolov, I. and Derzsi, A. and Schulze, J. and Eremin, D. and Mussenbrock, T. and Donkó, Z. and Brinkmann, R.P.
PLASMA SCIENCE AND TECHNOLOGY. Volume: 22 (2013) - 2013 • 2273
Correlative imaging of structural and elemental composition of bacterial Biofilms
Yang, Y. and Heine, R. and Xu, F. and Suhonen, H. and Helfen, L. and Rosenhahn, A. and Gorniak, T. and Kirchen, S. and Schwartz, T. and Baumbach, T.
JOURNAL OF PHYSICS: CONFERENCE SERIES. Volume: 463 (2013) - 2013 • 2272
Lack of coupling between superconductivity and orthorhombic distortion in stoichiometric single-crystalline FeSe
Böhmer, A.E. and Hardy, F. and Eilers, F. and Ernst, D. and Adelmann, P. and Schweiss, P. and Wolf, T. and Meingast, C.
PHYSICAL REVIEW B - CONDENSED MATTER AND MATERIALS PHYSICS. Volume: 87 (2013) - 2013 • 2271
Resonant probing system comprising a high accurate uniaxial nanoprobe and a new evaluation unit
Goj, B. and Dressler, L. and Hoffmann, M.
JOURNAL OF MICROMECHANICS AND MICROENGINEERING. Volume: 23 (2013) - 2013 • 2270
A Z′ = 6 crystal structure of (E)-N,N′-dicyclohexylacetamidine
Krasnopolski, M. and Seidel, R.W. and Goddard, R. and Breidung, J. and Winter, M.V. and Devi, A. and Fischer, R.A.
JOURNAL OF MOLECULAR STRUCTURE. Volume: 1031 (2013) - 2013 • 2269
Optical tweezers in microassembly
Ostendorf, A. and Ghadiri, R. and Ksouri, S.I.
PROCEEDINGS OF SPIE - THE INTERNATIONAL SOCIETY FOR OPTICAL ENGINEERING. Volume: 8607 (2013) - 2013 • 2268
Spatial Distributions of Alloying Elements Obtained from Atom Probe Tomography of the Amorphous Ribbon Fe75C11Si2B8Cr4
Shin, J. and Yi, S. and Pradeep, K.G. and Choi, P.P. and Raabe, D.
KOREAN JOURNAL OF MATERIALS RESEARCH. Volume: 23 (2013) - 2013 • 2267
A family of binary magnetic icosahedral quasicrystals based on rare earths and cadmium
Goldman, A.I. and Kong, T. and Kreyssig, A. and Jesche, A. and Ramazanoglu, M. and Dennis, K.W. and Bud'Ko, S.L. and Canfield, P.C.
NATURE MATERIALS. Volume: 12 (2013) - 2013 • 2266
Homoleptic gadolinium amidinates as precursors for MOCVD of oriented gadolinium nitride (GdN) thin films
Krasnopolski, M. and Hrib, C.G. and Seidel, R.W. and Winter, M. and Becker, H.-W. and Rogalla, D. and Fischer, R.A. and Edelmann, F.T. and Devi, A.
INORGANIC CHEMISTRY. Volume: 52 (2013) - 2013 • 2265
A new catalyst platform: Zeolite Beta from template-free synthesis
Yilmaz, B. and Müller, U. and Feyen, M. and Maurer, S. and Zhang, H. and Meng, X. and Xiao, F.-S. and Bao, X. and Zhang, W. and Imai, H. and Yokoi, T. and Tatsumi, T. and Gies, H. and De Baerdemaeker, T. and De Vos, D.
CATALYSIS SCIENCE AND TECHNOLOGY. Volume: 3 (2013) - 2013 • 2264
Ion-induced oxidation of aluminum during reactive magnetron sputtering
Kreiter, O. and Grosse-Kreul, S. and Corbella, C. and von Keudell, A.
JOURNAL OF APPLIED PHYSICS. Volume: 113 (2013) - 2013 • 2263
Twin polymerization at spherical hard templates: An approach to size-adjustable carbon hollow spheres with micro- or mesoporous shells
Böttger-Hiller, F. and Kempe, P. and Cox, G. and Panchenko, A. and Janssen, N. and Petzold, A. and Thurn-Albrecht, T. and Borchardt, L. and Rose, M. and Kaskel, S. and Georgi, C. and Lang, H. and Spange, S.
ANGEWANDTE CHEMIE - INTERNATIONAL EDITION. Volume: 52 (2013) - 2013 • 2262
Enhancing the activity of Pd on carbon nanofibers for deoxygenation of amphiphilic fatty acid molecules through support polarity
Gosselink, R.W. and Xia, W. and Muhler, M. and De Jong, K.P. and Bitter, J.H.
ACS CATALYSIS. Volume: 3 (2013) - 2013 • 2261
Influence of supersaturated carbon on the diffusion of Ni in ferrite determined by atom probe tomography
Kresse, T. and Li, Y.J. and Boll, T. and Borchers, C. and Choi, P. and Al-Kassab, T. and Raabe, D. and Kirchheim, R.
SCRIPTA MATERIALIA. Volume: 69 (2013) - 2013 • 2260
The influences of temperature and microstructure on the tensile properties of a CoCrFeMnNi high-entropy alloy
Otto, F. and Dlouhý, A. and Somsen, C. and Bei, H. and Eggeler, G. and George, E.P.
ACTA MATERIALIA. Volume: 61 (2013) - 2013 • 2259
Maintaining the equipartition theorem in small heterogeneous molecular dynamics ensembles
Siboni, N.H. and Raabe, D. and Varnik, F.
PHYSICAL REVIEW E - STATISTICAL, NONLINEAR, AND SOFT MATTER PHYSICS. Volume: 87 (2013) - 2013 • 2258
Antiferromagnetic order and the structural order-disorder transition in the Cd 6Ho quasicrystal approximant
Kreyssig, A. and Beutier, G. and Hiroto, T. and Kim, M.G. and Tucker, G.S. and Boissieu, M.D. and Tamura, R. and I.goldman, A.
PHILOSOPHICAL MAGAZINE LETTERS. Volume: 93 (2013) - 2013 • 2257
A silicon-heteroaromatic system as photosensitizer for light-driven hydrogen production by hydrogenase mimics
Goy, R. and Apfel, U.-P. and Elleouet, C. and Escudero, D. and Elstner, M. and Görls, H. and Talarmin, J. and Schollhammer, P. and González, L. and Weigand, W.
EUROPEAN JOURNAL OF INORGANIC CHEMISTRY. Volume: (2013) - 2013 • 2256
Relative effects of enthalpy and entropy on the phase stability of equiatomic high-entropy alloys
Otto, F. and Yang, Y. and Bei, H. and George, E.P.
ACTA MATERIALIA. Volume: 61 (2013) - 2013 • 2255
Synchrotron X-ray measurement techniques for thermal barrier coated cylindrical samples under thermal gradients
Siddiqui, S.F. and Knipe, K. and Manero, A. and Meid, C. and Wischek, J. and Okasinski, J. and Almer, J. and Karlsson, A.M. and Bartsch, M. and Raghavan, S.
REVIEW OF SCIENTIFIC INSTRUMENTS. Volume: 84 (2013) - 2013 • 2254
Strain mapping of crack extension in pseudoelastic NiTi shape memory alloys during static loading
Young, M.L. and Gollerthan, S. and Baruj, A. and Frenzel, J. and Schmahl, W.W. and Eggeler, G.
ACTA MATERIALIA. Volume: 61 (2013) - 2013 • 2253
Interaction of electrolyte molecules with carbon materials of well-defined porosity: Characterization by solid-state NMR spectroscopy
Borchardt, L. and Oschatz, M. and Paasch, S. and Kaskel, S. and Brunner, E.
PHYSICAL CHEMISTRY CHEMICAL PHYSICS. Volume: 15 (2013) - 2013 • 2252
High-throughput compositional and structural evaluation of a Li a(NixMnyCoz)Or thin film battery materials library
Borhani-Haghighi, S. and Kieschnick, M. and Motemani, Y. and Savan, A. and Rogalla, D. and Becker, H.-W. and Meijer, J. and Ludwig, Al.
ACS COMBINATORIAL SCIENCE. Volume: 15 (2013) - 2013 • 2251
Crossover from tumbling to tank-treading-like motion in dense simulated suspensions of red blood cells
Krüger, T. and Gross, M. and Raabe, D. and Varnik, F.
SOFT MATTER. Volume: 9 (2013) - 2013 • 2250
Relaxor behavior of CaxBa1-xNb2O 6 (0.18≤x≤0.35) tuned by Ca/Ba ratio and investigated by resonant ultrasound spectroscopy
Pandey, C.S. and Schreuer, J. and Burianek, M. and Mühlberg, M.
PHYSICAL REVIEW B - CONDENSED MATTER AND MATERIALS PHYSICS. Volume: 87 (2013) - 2013 • 2249
Improving the current density and the coulombic efficiency by a cascade reaction of glucose oxidizing enzymes
Zafar, M.N. and Shao, M. and Ludwig, R. and Leech, D. and Schuhmann, W. and Gorton, L.
ECS TRANSACTIONS. Volume: 53 (2013) - 2013 • 2248
Crystallographic, electronic, thermal, and magnetic properties of single-crystal SrCo2As2
Pandey, A. and Quirinale, D.G. and Jayasekara, W. and Sapkota, A. and Kim, M.G. and Dhaka, R.S. and Lee, Y. and Heitmann, T.W. and Stephens, P.W. and Ogloblichev, V. and Kreyssig, A. and McQueeney, R.J. and Goldman, A.I. and Kaminski, A. and Harmon, B.N. and Furukawa, Y. and Johnston, D.C.
PHYSICAL REVIEW B - CONDENSED MATTER AND MATERIALS PHYSICS. Volume: 88 (2013) - 2013 • 2247
Coexistence of half-metallic itinerant ferromagnetism with local-moment antiferromagnetism in Ba0.60K0.40Mn2As 2
Pandey, A. and Ueland, B.G. and Yeninas, S. and Kreyssig, A. and Sapkota, A. and Zhao, Y. and Helton, J.S. and Lynn, J.W. and McQueeney, R.J. and Furukawa, Y. and Goldman, A.I. and Johnston, D.C.
PHYSICAL REVIEW LETTERS. Volume: 111 (2013) - 2013 • 2246
Optimal discriminating designs for several competing regression models
Braess, D. and Dette, H.
ANNALS OF STATISTICS. Volume: 41 (2013) - 2013 • 2245
Mo(VI)-melamine hybrid as single-source precursor to pure-phase β-Mo2C for the selective hydrogenation of naphthalene to tetralin
Pang, M. and Wang, X. and Xia, W. and Muhler, M. and Liang, C.
INDUSTRIAL AND ENGINEERING CHEMISTRY RESEARCH. Volume: 52 (2013) - 2013 • 2244
Controlled growth of protein resistant PHEMA brushes via S-RAFT polymerization
Zamfir, M. and Rodriguez-Emmenegger, C. and Bauer, S. and Barner, L. and Rosenhahn, A. and Barner-Kowollik, C.
JOURNAL OF MATERIALS CHEMISTRY B. Volume: 1 (2013) - 2013 • 2243
Design of ordered mesoporous composite materials and their electrocatalytic activities for water oxidation
Grewe, T. and Deng, X. and Weidenthaler, C. and Schüth, F. and Tüysüz, H.
CHEMISTRY OF MATERIALS. Volume: 25 (2013) - 2013 • 2242
Time- and space-resolved high-throughput characterization of stresses during sputtering and thermal processing of Al-Cr-N thin films
Grochla, D. and Siegel, A. and Hamann, S. and Buenconsejo, P.J.S. and Kieschnick, M. and Brunken, H. and König, D. and Ludwig, Al.
JOURNAL OF PHYSICS D: APPLIED PHYSICS. Volume: 46 (2013) - 2013 • 2241
Discrete element analysis of experiments on mixing and bulk transport of wood pellets on a forward acting grate in discontinuous operation
Kruggel-Emden, H. and Kačianauskas, R.
CHEMICAL ENGINEERING SCIENCE. Volume: 92 (2013) - 2013 • 2240
In situ visualization of Li-ion intercalation and formation of the solid electrolyte interphase on TiO2 based paste electrodes using scanning electrochemical microscopy
Zampardi, G. and Ventosa, E. and La Mantia, F. and Schuhmann, W.
CHEMICAL COMMUNICATIONS. Volume: 49 (2013) - 2013 • 2239
An experimental investigation of mixing of wood pellets on a forward acting grate in discontinuous operation
Kruggel-Emden, H. and Wirtz, S. and Scherer, V.
POWDER TECHNOLOGY. Volume: 233 (2013) - 2013 • 2238
Thermal analysis on conversion of MoO3 to MoO2 and its silicothermic reduction
Paul, B. and Kishor, J. and Chakraborty, S.P. and Suri, A.K.
JOURNAL OF THERMAL ANALYSIS AND CALORIMETRY. Volume: 112 (2013) - 2013 • 2237
Formation of Silicide Based Oxidation Resistant Coating Over Mo-30 wt% W Alloy
Paul, B. and Chakraborty, S.P. and Suri, A.K.
TRANSACTIONS OF THE INDIAN CERAMIC SOCIETY. Volume: 72 (2013) - 2013 • 2236
Optical spectroscopy of Spin noise
Zapasskii, V.S. and Greilich, A. and Crooker, S.A. and Li, Y. and Kozlov, G.G. and Yakovlev, D.R. and Reuter, D. and Wieck, A.D. and Bayer, M.
PHYSICAL REVIEW LETTERS. Volume: 110 (2013) - 2013 • 2235
Dynamic of the growth flux at the substrate during high-power pulsed magnetron sputtering (HiPIMS) of titanium
Breilmann, W. and Maszl, C. and Benedikt, J. and von Keudell, A.
JOURNAL OF PHYSICS D-APPLIED PHYSICS. Volume: 46 (2013) - 2013 • 2234
Determination of temperature gradients with micrometric resolution by local open circuit potential measurements at a scanning microelectrode
Sode, A. and Nebel, M. and Pinyou, P. and Schmaderer, S. and Szeponik, J. and Plumeré, N. and Schuhmann, W.
ELECTROANALYSIS. Volume: 25 (2013) - 2013 • 2233
Investigations of concrete scaling at edge beams of bridges due to freeze deicing salt attacks
Breitenbücher, R. and Youn, B.-Y.
BETON- UND STAHLBETONBAU. Volume: 108 (2013) - 2013 • 2232
Viscous coalescence of droplets: A lattice Boltzmann study
Gross, M. and Steinbach, I. and Raabe, D. and Varnik, F.
PHYSICS OF FLUIDS. Volume: 25 (2013) - 2013 • 2231
A modular assembling platform for manufacturing of microsystems by optical tweezers
Ksouri, S.I. and Aumann, A. and Ghadiri, R. and Prüfer, M. and Baer, S. and Ostendorf, A.
PROCEEDINGS OF SPIE - THE INTERNATIONAL SOCIETY FOR OPTICAL ENGINEERING. Volume: 8810 (2013) - 2013 • 2230
Inelastic neutron scattering study of a nonmagnetic collapsed tetragonal phase in nonsuperconducting CaFe2As2: Evidence of the impact of spin fluctuations on superconductivity in the iron-arsenide compounds
Soh, J.H. and Tucker, G.S. and Pratt, D.K. and Abernathy, D.L. and Stone, M.B. and Ran, S. and Bud'Ko, S.L. and Canfield, P.C. and Kreyssig, A. and McQueeney, R.J. and Goldman, A.I.
PHYSICAL REVIEW LETTERS. Volume: 111 (2013) - 2013 • 2229
Interfacial roughening in nonideal fluids: Dynamic scaling in the weak- and strong-damping regime
Gross, M. and Varnik, F.
PHYSICAL REVIEW E - STATISTICAL, NONLINEAR, AND SOFT MATTER PHYSICS. Volume: 87 (2013) - 2013 • 2228
Optical micro-assembling of non-spherical particles
Ksouri, S. I. and Aumann, A. and Ghadiri, R. and Ostendorf, A.
COMPLEX LIGHT AND OPTICAL FORCES VII. Volume: 8637 (2013) - 2013 • 2227
Ab initio and atomistic study of generalized stacking fault energies in Mg and Mg-Y alloys
Pei, Z. and Zhu, L.-F. and Friák, M. and Sandlöbes, S. and Von Pezold, J. and Sheng, H.W. and Race, C.P. and Zaefferer, S. and Svendsen, B. and Raabe, D. and Neugebauer, J.
NEW JOURNAL OF PHYSICS. Volume: 15 (2013) - 2013 • 2226
Colliding pulse modelocked lasers for terahertz photomixing
Brenner, C. and Horstkemper, H. and Mayorga, I.C. and Klehr, A. and Erbert, G. and Hofmann, M.R.
2013 CONFERENCE ON LASERS AND ELECTRO-OPTICS EUROPE AND INTERNATIONAL QUANTUM ELECTRONICS CONFERENCE, CLEO/EUROPE-IQEC 2013. Volume: (2013) - 2013 • 2225
Surface Modification of Polypropylene (PP) by Argon Ions and UV Photons
Grosse-Kreul, S. and Corbella, C. and von Keudell, A. and Ozkaya, B. and Grundmeier, G.
PLASMA PROCESSES AND POLYMERS. Volume: 10 (2013) - 2013 • 2224
Chemical and Physical Sputtering of Polyethylene Terephthalate (PET)
Grosse-Kreul, S. and Corbella, C. and von Keudell, A.
PLASMA PROCESSES AND POLYMERS. Volume: 10 (2013) - 2013 • 2223
Reply to "comment on 'First-principles study of the influence of (110)-oriented strain on the ferroelectric properties of rutile TiO2' "
Grünebohm, A. and Ederer, C. and Entel, P.
PHYSICAL REVIEW B - CONDENSED MATTER AND MATERIALS PHYSICS. Volume: 88 (2013) - 2013 • 2222
Charge noise and spin noise in a semiconductor quantum device
Kuhlmann, A.V. and Houel, J. and Ludwig, Ar. and Greuter, L. and Reuter, D. and Wieck, A.D. and Poggio, M. and Warburton, R.J.
NATURE PHYSICS. Volume: 9 (2013) - 2013 • 2221
Atomic-scale investigation of ε and θ precipitates in bainite in 100Cr6 bearing steel by atom probe tomography and ab initio calculations
Song, W. and Von Appen, J. and Choi, P. and Dronskowski, R. and Raabe, D. and Bleck, W.
ACTA MATERIALIA. Volume: 61 (2013) - 2013 • 2220
Mechanical properties of zirconia composite ceramics
Zhang, Y. and Malzbender, J. and Mack, D.E. and Jarligo, M.O. and Cao, X. and Li, Q. and Vaßen, R. and Stöver, D.
CERAMICS INTERNATIONAL. Volume: 39 (2013) - 2013 • 2219
Ab initio study of the electronic and magnetic structure of the TiO 2 rutile (110)/Fe interface
Grünebohm, A. and Entel, P. and Herper, H.C.
PHYSICAL REVIEW B - CONDENSED MATTER AND MATERIALS PHYSICS. Volume: 88 (2013) - 2013 • 2218
A dark-field microscope for background-free detection of resonance fluorescence from single semiconductor quantum dots operating in a set-and-forget mode
Kuhlmann, A.V. and Houel, J. and Brunner, D. and Ludwig, Ar. and Reuter, D. and Wieck, A.D. and Warburton, R.J.
REVIEW OF SCIENTIFIC INSTRUMENTS. Volume: 84 (2013) - 2013 • 2217
Effect of oxygen content in NiCoCrAlY bondcoat on the lifetimes of EB-PVD and APS thermal barrier coatings
Song, P. and Naumenko, D. and Vassen, R. and Singheiser, L. and Quadakkers, W.J.
SURFACE AND COATINGS TECHNOLOGY. Volume: 221 (2013) - 2013 • 2216
Insights into the atomic and electronic structure triggered by ordered nitrogen vacancies in CrN
Zhang, Z. and Li, H. and Daniel, R. and Mitterer, C. and Dehm, G.
PHYSICAL REVIEW B - CONDENSED MATTER AND MATERIALS PHYSICS. Volume: 87 (2013) - 2013 • 2215
First-principles investigation of incipient ferroelectric trends of rutile TiO2 in bulk and at the (110) surface
Grünebohm, A. and Entel, P. and Ederer, C.
PHYSICAL REVIEW B - CONDENSED MATTER AND MATERIALS PHYSICS. Volume: 87 (2013) - 2013 • 2214
Trends in the Binding Strength of Surface Species on Nanoparticles: How Does the Adsorption Energy Scale with the Particle Size?
Peter, M. and Camacho, J. M. F. and Adamovski, S. and Ono, L. K. and Dostert, K. H. and O'Brien, C. P. and Cuenya, B. R. and Schauermann, S. and Freund, H. J.
ANGEWANDTE CHEMIE-INTERNATIONAL EDITION. Volume: 52 (2013) - 2013 • 2213
Direct methane oxidation over Pt-modified nitrogen-doped carbons
Soorholtz, M. and White, R.J. and Zimmermann, T. and Titirici, M.-M. and Antonietti, M. and Palkovits, R. and Schüth, F.
CHEMICAL COMMUNICATIONS. Volume: 49 (2013) - 2013 • 2212
Metal-supported catalysts encapsulated in mesoporous solids: Challenges and opportunities of a model concept
Grünert, W. and Gies, H. and Muhler, M. and Polarz, S. and Lehmann, C.W. and Großmann, D. and van den Berg, M. and Tkachenko, O.P. and De Toni, A. and Sinev, I. and Bandyopadhyay, M. and Narkhede, V. and Dreier, A. and Klementiev, K.V. and Birkner, A. and Löffler, E.
PHYSICA STATUS SOLIDI (B) BASIC RESEARCH. Volume: 250 (2013) - 2013 • 2211
Fast crystallization of the phase change compound GeTe by large-scale molecular dynamics simulations
Sosso, G.C. and Miceli, G. and Caravati, S. and Giberti, F. and Behler, J. and Bernasconi, M.
JOURNAL OF PHYSICAL CHEMISTRY LETTERS. Volume: 4 (2013) - 2013 • 2210
Rational synthesis of Beta zeolite with improved quality by decreasing crystallization temperature in organotemplate-free route
Zhang, H. and Xie, B. and Meng, X. and Müller, U. and Yilmaz, B. and Feyen, M. and Maurer, S. and Gies, H. and Tatsumi, T. and Bao, X. and Zhang, W. and De Vos, D. and Xiao, F.-S.
MICROPOROUS AND MESOPOROUS MATERIALS. Volume: 180 (2013) - 2013 • 2209
Long electron spin coherence in ion-implanted GaN: The role of localization
Bu, J.H. and Rudolph, J. and Shvarkov, S. and Hardtdegen, H. and Wieck, A.D. and Hägele, D.
APPLIED PHYSICS LETTERS. Volume: 102 (2013) - 2013 • 2208
Breakdown of Stokes-Einstein relation in the supercooled liquid state of phase change materials [Phys. Status Solidi B 249, No. 10, 1880-1885 (2012)]
Sosso, G.C. and Behler, J. and Bernasconi, M.
PHYSICA STATUS SOLIDI (B) BASIC RESEARCH. Volume: 250 (2013) - 2013 • 2207
Mechanisms of anisotropic friction in nanotwinned Cu revealed by atomistic simulations
Zhang, J.J. and Hartmaier, A. and Wei, Y.J. and Yan, Y.D. and Sun, T.
MODELLING AND SIMULATION IN MATERIALS SCIENCE AND ENGINEERING. Volume: 21 (2013) - 2013 • 2206
Compositional trends and magnetic excitations in binary and ternary Fe-Pd-X magnetic shape memory alloys
Gruner, M.E. and Hamann, S. and Brunken, H. and Ludwig, Al. and Entel, P.
JOURNAL OF ALLOYS AND COMPOUNDS. Volume: 577 (2013) - 2013 • 2205
Ab Initio Based conformational study of the crystalline α-chitin
Petrov, M. and Lymperakis, L. and Friák, M. and Neugebauer, J.
BIOPOLYMERS. Volume: 99 (2013) - 2013 • 2204
Interfacial structure and chemistry of GaN on Ge(111)
Zhang, S. and Zhang, Y. and Cui, Y. and Freysoldt, C. and Neugebauer, J. and Lieten, R.R. and Barnard, J.S. and Humphreys, C.J.
PHYSICAL REVIEW LETTERS. Volume: 111 (2013) - 2013 • 2203
Size versus electronic factors in transition metal carbide and TCP phase stability
Pettifor, D.G. and Seiser, B. and Margine, E.R. and Kolmogorov, A.N. and Drautz, R.
PHILOSOPHICAL MAGAZINE. Volume: 93 (2013) - 2013 • 2202
Diffuse-interface modeling of solute trapping in rapid solidification: Predictions of the hyperbolic phase-field model and parabolic model with finite interface dissipation
Zhang, L. and Danilova, E.V. and Steinbach, I. and Medvedev, D. and Galenko, P.K.
ACTA MATERIALIA. Volume: 61 (2013) - 2013 • 2201
Dangling-bond defect in a-Si:H: Characterization of network and strain effects by first-principles calculation of the EPR parameters
Pfanner, G. and Freysoldt, C. and Neugebauer, J. and Inam, F. and Drabold, D. and Jarolimek, K. and Zeman, M.
PHYSICAL REVIEW B - CONDENSED MATTER AND MATERIALS PHYSICS. Volume: 87 (2013) - 2013 • 2200
Hanle effect in (In,Ga)As quantum dots: Role of nuclear spin fluctuations
Kuznetsova, M.S. and Flisinski, K. and Gerlovin, I.Y. and Ignatiev, I.V. and Kavokin, K.V. and Verbin, S.Y. and Yakovlev, D.R. and Reuter, D. and Wieck, A.D. and Bayer, M.
PHYSICAL REVIEW B - CONDENSED MATTER AND MATERIALS PHYSICS. Volume: 87 (2013) - 2013 • 2199
On the crystallographic anisotropy of nanoindentation in pseudoelastic NiTi
Pfetzing-Micklich, J. and Somsen, C. and Dlouhy, A. and Begau, C. and Hartmaier, A. and Wagner, M.F.-X. and Eggeler, G.
ACTA MATERIALIA. Volume: 61 (2013) - 2013 • 2198
Insights into the topotactic conversion process from layered silicate RUB-36 to FER-type zeolite by layer reassembly
Zhao, Z. and Zhang, W. and Ren, P. and Han, X. and Müller, U. and Yilmaz, B. and Feyen, M. and Gies, H. and Xiao, F.-S. and De Vos, D. and Tatsumi, T. and Bao, X.
CHEMISTRY OF MATERIALS. Volume: 25 (2013) - 2013 • 2197
Multiplier bootstrap of tail copulas with applications
Bücher, A. and Dette, H.
BERNOULLI. Volume: 19 (2013) - 2013 • 2196
A stochastic model for the size dependence of spherical indentation pop-in
Phani, P. S. and Johanns, K. E. and George, E. P. and Pharr, G. M.
JOURNAL OF MATERIALS RESEARCH. Volume: 28 (2013) - 2013 • 2195
Bulk combinatorial design of ductile martensitic stainless steels through confined martensite-to-austenite reversion
Springer, H. and Belde, M. and Raabe, D.
MATERIALS SCIENCE AND ENGINEERING A. Volume: 582 (2013) - 2013 • 2194
N-doped carbon synthesized from N-containing polymers as metal-free catalysts for the oxygen reduction under alkaline conditions
Zhao, A. and Masa, J. and Muhler, M. and Schuhmann, W. and Xia, W.
ELECTROCHIMICA ACTA. Volume: 98 (2013) - 2013 • 2193
Adsorption of amino acids on the magnetite-(111)-surface: A force field study
Bürger, A. and Magdans, U. and Gies, H.
JOURNAL OF MOLECULAR MODELING. Volume: 19 (2013) - 2013 • 2192
Design of next generation thermal barrier coatings - Experiments and modelling
Gupta, M. and Curry, N. and Nylén, P. and Markocsan, N. and Vaßen, R.
SURFACE AND COATINGS TECHNOLOGY. Volume: 220 (2013) - 2013 • 2191
Characterization of deformation anisotropies in an α-Ti alloy by nanoindentation and electron microscopy
Kwon, J. and Brandes, M.C. and Sudharshan Phani, P. and Pilchak, A.P. and Gao, Y.F. and George, E.P. and Pharr, G.M. and Mills, M.J.
ACTA MATERIALIA. Volume: 61 (2013) - 2013 • 2190
Activation and stabilization of nitrogen-doped carbon nanotubes as electrocatalysts in the oxygen reduction reaction at strongly alkaline conditions
Zhao, A. and Masa, J. and Schuhmann, W. and Xia, W.
JOURNAL OF PHYSICAL CHEMISTRY C. Volume: 117 (2013) - 2013 • 2189
MOF-FF - A flexible first-principles derived force field for metal-organic frameworks
Bureekaew, S. and Amirjalayer, S. and Tafipolsky, M. and Spickermann, C. and Roy, T.K. and Schmid, R.
PHYSICA STATUS SOLIDI (B) BASIC RESEARCH. Volume: 250 (2013) - 2013 • 2188
Development of Ni-Cu materials library by using combinatorial pulsed electrodeposition
Srinivas, P. and Hamann, S. and Wambach, M. and Kieschnick, M. and Ludwig, Al. and Dey, S.R.
TRANSACTIONS OF THE INDIAN INSTITUTE OF METALS. Volume: 66 (2013) - 2013 • 2187
Composition-dependent crystal structure and martensitic transformation in Heusler Ni-Mn-Sn alloys
Zheng, H. and Wang, W. and Xue, S. and Zhai, Q. and Frenzel, J. and Luo, Z.
ACTA MATERIALIA. Volume: 61 (2013) - 2013 • 2186
Hypothetical 3D-periodic covalent organic frameworks: Exploring the possibilities by a first principles derived force field
Bureekaew, S. and Schmid, R.
CRYSTENGCOMM. Volume: 15 (2013) - 2013 • 2185
Transition metal nitride thin films grown by MOCVD using amidinato based complexes [M(NtBu)2{(iPrN)2CMe}2] (M=Mo, W) as precursors
Srinivasan, N.B. and Thiede, T.B. and de los Arcos, T. and Gwildies, V. and Krasnopolski, M. and Becker, H.-W. and Rogalla, D. and Devi, A. and Fischer, R.A.
SURFACE AND COATINGS TECHNOLOGY. Volume: 230 (2013) - 2013 • 2184
Athermal nature of the martensitic transformation in Heusler alloy Ni-Mn-Sn
Zheng, H. and Wang, W. and Wu, D. and Xue, S. and Zhai, Q. and Frenzel, J. and Luo, Z.
INTERMETALLICS. Volume: 36 (2013) - 2013 • 2183
Strong Pauli-limiting behavior of Hc2 and uniaxial pressure dependencies in KFe2As2
Burger, P. and Hardy, F. and Aoki, D. and Böhmer, A.E. and Eder, R. and Heid, R. and Wolf, T. and Schweiss, P. and Fromknecht, R. and Jackson, M.J. and Paulsen, C. and Meingast, C.
PHYSICAL REVIEW B - CONDENSED MATTER AND MATERIALS PHYSICS. Volume: 88 (2013) - 2013 • 2182
Coupling of electron channeling with EBSD: Toward the quantitative characterization of deformation structures in the sem
Gutierrez-Urrutia, I. and Zaefferer, S. and Raabe, D.
JOM. Volume: 65 (2013) - 2013 • 2181
Using a tunable quantum wire to measure the large out-of-plane spin splitting of quasi two-dimensional holes in a GaAs nanostructure
Srinivasan, A. and Yeoh, L.A. and Klochan, O. and Martin, T.P. and Chen, J.C.H. and Micolich, A.P. and Hamilton, A.R. and Reuter, D. and Wieck, A.D.
NANO LETTERS. Volume: 13 (2013) - 2013 • 2180
Interaction between recrystallization and phase transformation during intercritical annealing in a cold-rolled dual-phase steel: A cellular automaton model
Zheng, C. and Raabe, D.
ACTA MATERIALIA. Volume: 61 (2013) - 2013 • 2179
Microbanding mechanism in an Fe-Mn-C high-Mn twinning-induced plasticity steel
Gutierrez-Urrutia, I. and Raabe, D.
SCRIPTA MATERIALIA. Volume: 69 (2013) - 2013 • 2178
Experimental and theoretical investigation of molybdenum carbide and nitride as catalysts for ammonia decomposition
Zheng, W. and Cotter, T.P. and Kaghazchi, P. and Jacob, T. and Frank, B. and Schlichte, K. and Zhang, W. and Su, D.S. and Schüth, F. and Schlögl, R.
JOURNAL OF THE AMERICAN CHEMICAL SOCIETY. Volume: 135 (2013) - 2013 • 2177
Magneto-optical studies of Gd-implanted GaN: No spin alignment of conduction band electrons
Buß, J.H. and Rudolph, J. and Shvarkov, S. and Semond, F. and Reuter, D. and Wieck, A.D. and Hägele, D.
APPLIED PHYSICS LETTERS. Volume: 103 (2013) - 2013 • 2176
Influence of Al content and precipitation state on the mechanical behavior of austenitic high-Mn low-density steels
Gutierrez-Urrutia, I. and Raabe, D.
SCRIPTA MATERIALIA. Volume: 68 (2013) - 2013 • 2175
Electron spin relaxation dynamics in GaN: Inuence of temperature, doping density, and crystal orientation
Buß, J.H. and Rudolph, J. and Starosielec, S. and Schaefer, A. and Semond, F. and Hägele, D.
PROCEEDINGS OF SPIE - THE INTERNATIONAL SOCIETY FOR OPTICAL ENGINEERING. Volume: 8623 (2013) - 2013 • 2174
Persistence of local-moment antiferromagnetic order in Ba 1-xKxMn2As2
Lamsal, J. and Tucker, G.S. and Heitmann, T.W. and Kreyssig, A. and Jesche, A. and Pandey, A. and Tian, W. and McQueeney, R.J. and Johnston, D.C. and Goldman, A.I.
PHYSICAL REVIEW B - CONDENSED MATTER AND MATERIALS PHYSICS. Volume: 87 (2013) - 2013 • 2173
A three-phase thermo-hydro-mechanical finite element model for freezing soils
Zhou, M.M. and Meschke, G.
INTERNATIONAL JOURNAL FOR NUMERICAL AND ANALYTICAL METHODS IN GEOMECHANICS. Volume: 37 (2013) - 2013 • 2172
Grazing-incidence X-ray diffraction of single GaAs nanowires at locations defined by focused ion beams
Bussone, G. and Schott, R. and Biermanns, A. and Davydok, A. and Reuter, D. and Carbone, G. and Schülli, T.U. and Wieck, A.D. and Pietsch, U.
JOURNAL OF APPLIED CRYSTALLOGRAPHY. Volume: 46 (2013) - 2013 • 2171
Dense membranes for oxygen and hydrogen separation (DEMOYS): Project overview and first results
Pinacci, P. and Louradour, E. and Wimbert, L. and Gindrat, M. and Jarligo, M.O. and Vassen, R. and Comite, A. and Serra, J.M. and Jewulski, J. and Mancuso, L. and Chiesa, P. and Prestat, M. and Ivers-Tiffée, E.
ENERGY PROCEDIA. Volume: 37 (2013) - 2013 • 2170
Utilization of low-pressure plasma to inactivate bacterial spores on stainless steel screws
Stapelmann, K. and Fiebrandt, M. and Raguse, M. and Awakowicz, P. and Reitz, G. and Moeller, R.
ASTROBIOLOGY. Volume: 13 (2013) - 2013 • 2169
Strength homogenization for partially frozen soil using linear comparison composite approach
Zhou, M.-M. and Meschke, G.
POROMECHANICS V - PROCEEDINGS OF THE 5TH BIOT CONFERENCE ON POROMECHANICS. Volume: (2013) - 2013 • 2168
Functionalised porous nanocomposites: A multidisciplinary approach to investigate designed structures for supercapacitor applications
Pinkert, K. and Giebeler, L. and Herklotz, M. and Oswald, S. and Thomas, J. and Meier, A. and Borchardt, L. and Kaskel, S. and Ehrenberg, H. and Eckert, J.
JOURNAL OF MATERIALS CHEMISTRY A. Volume: 1 (2013) - 2013 • 2167
Ab initio study of single-crystalline and polycrystalline elastic properties of Mg-substituted calcite crystals
Zhu, L.-F. and Friák, M. and Lymperakis, L. and Titrian, H. and Aydin, U. and Janus, A.M. and Fabritius, H.-O. and Ziegler, A. and Nikolov, S. and Hemzalová, P. and Raabe, D. and Neugebauer, J.
JOURNAL OF THE MECHANICAL BEHAVIOR OF BIOMEDICAL MATERIALS. Volume: 20 (2013) - 2013 • 2166
Comparison of analytic and numerical bond-order potentials for W and Mo
Čák, M. and Hammerschmidt, T. and Drautz, R.
JOURNAL OF PHYSICS CONDENSED MATTER. Volume: 25 (2013) - 2013 • 2165
On dislocation involvement in Ti-Nb gum metal plasticity
Plancher, E. and Tasan, C.C. and Sandloebes, S. and Raabe, D.
SCRIPTA MATERIALIA. Volume: 68 (2013) - 2013 • 2164
Additive manufacturing of shape memory devices and pseudoelastic components
Haberland, C. and Elahinia, M. and Walker, J. and Meier, H. and Frenzel, J.
ASME 2013 CONFERENCE ON SMART MATERIALS, ADAPTIVE STRUCTURES AND INTELLIGENT SYSTEMS, SMASIS 2013. Volume: 1 (2013) - 2013 • 2163
The biocompatibility of dense and porous Nickel-Titanium produced by selective laser melting
Habijan, T. and Haberland, C. and Meier, H. and Frenzel, J. and Wittsiepe, J. and Wuwer, C. and Greulich, C. and Schildhauer, T.A. and Köller, M.
MATERIALS SCIENCE AND ENGINEERING C. Volume: 33 (2013) - 2013 • 2162
High-temperature deformation and recrystallization: A variational analysis and its application to olivine aggregates
Hackl, K. and Renner, J.
JOURNAL OF GEOPHYSICAL RESEARCH: SOLID EARTH. Volume: 118 (2013) - 2013 • 2161
Active plasma resonance spectroscopy: A functional analytic description
Lapke, M. and Oberrath, J. and Mussenbrock, T. and Brinkmann, R.P.
PLASMA SOURCES SCIENCE AND TECHNOLOGY. Volume: 22 (2013) - 2013 • 2160
A variational approach to grooving and wetting
Hackl, K. and Fischer, F.D. and Klevakina, K. and Renner, J. and Svoboda, J.
ACTA MATERIALIA. Volume: 61 (2013) - 2013 • 2159
Why solidification? Why phase-field?
Steinbach, I.
JOM. Volume: 65 (2013) - 2013 • 2158
Band offsets at zincblende-wurtzite GaAs nanowire sidewall surfaces
Capiod, P. and Xu, T. and Nys, J.P. and Berthe, M. and Patriarche, G. and Lymperakis, L. and Neugebauer, J. and Caroff, P. and Dunin-Borkowski, R.E. and Ebert, P. and Grandidier, B.
APPLIED PHYSICS LETTERS. Volume: 103 (2013) - 2013 • 2157
Carbon Cloth/Carbon Nanotube Electrodes for Biofuel Cells Development
Haddad, R. and Xia, W. and Guschin, D.A. and Pöller, S. and Shao, M. and Vivekananthan, J. and Muhler, M. and Schuhmann, W.
ELECTROANALYSIS. Volume: 25 (2013) - 2013 • 2156
Stabilizing redox polymer films by electrochemically induced crosslinking
Pöller, S. and Koster, D. and Schuhmann, W.
ELECTROCHEMISTRY COMMUNICATIONS. Volume: 34 (2013) - 2013 • 2155
Phase-field model for microstructure evolution at the mesoscopic scale
Steinbach, I.
ANNUAL REVIEW OF MATERIALS RESEARCH. Volume: 43 (2013) - 2013 • 2154
Ergodic versus diffusive decoherence in mesoscopic devices
Capron, T. and Texier, C. and Montambaux, G. and Mailly, D. and Wieck, A.D. and Saminadayar, L.
PHYSICAL REVIEW B - CONDENSED MATTER AND MATERIALS PHYSICS. Volume: 87 (2013) - 2013 • 2153
Effect of climb on dislocation mechanisms and creep rates in γ′-strengthened Ni base superalloy single crystals: A discrete dislocation dynamics study
Hafez Haghighat, S.M. and Eggeler, G. and Raabe, D.
ACTA MATERIALIA. Volume: 61 (2013) - 2013 • 2152
Low potential biofuel cell anodes based on redox polymers with covalently bound phenothiazine derivatives for wiring flavin adenine dinucleotide-dependent enzymes
Pöller, S. and Shao, M. and Sygmund, C. and Ludwig, R. and Schuhmann, W.
ELECTROCHIMICA ACTA. Volume: 110 (2013) - 2013 • 2151
Combined influence of Coulomb interaction and polarons on the carrier dynamics in InGaAs quantum dots
Steinhoff, A. and Kurtze, H. and Gartner, P. and Florian, M. and Reuter, D. and Wieck, A.D. and Bayer, M. and Jahnke, F.
PHYSICAL REVIEW B - CONDENSED MATTER AND MATERIALS PHYSICS. Volume: 88 (2013) - 2013 • 2150
High-throughput ab initio screening of binary solid solutions in olivine phosphates for Li-ion battery cathodes
Hajiyani, H.R. and Preiss, U. and Drautz, R. and Hammerschmidt, T.
MODELLING AND SIMULATION IN MATERIALS SCIENCE AND ENGINEERING. Volume: 21 (2013) - 2013 • 2149
Sulfur-infiltrated micro- and mesoporous silicon carbide-derived carbon cathode for high-performance lithium sulfur batteries
Lee, J.T. and Zhao, Y. and Thieme, S. and Kim, H. and Oschatz, M. and Borchardt, L. and Magasinski, A. and Cho, W.-I. and Kaskel, S. and Yushin, G.
ADVANCED MATERIALS. Volume: 25 (2013) - 2013 • 2148
La-Sr-Fe-Co oxygen transport membranes on metal supports deposited by low pressure plasma spraying-physical vapour deposition
Zotov, N. and Baumann, S. and Meulenberg, W.A. and Vaßen, R.
JOURNAL OF MEMBRANE SCIENCE. Volume: 442 (2013) - 2013 • 2147
The effect of (NH4)2Sx passivation on the (311)A GaAs surface and its use in AlGaAs/GaAs heterostructure devices
Carrad, D.J. and Burke, A.M. and Reece, P.J. and Lyttleton, R.W. and Waddington, D.E.J. and Rai, A. and Reuter, D. and Wieck, A.D. and Micolich, A.P.
JOURNAL OF PHYSICS CONDENSED MATTER. Volume: 25 (2013) - 2013 • 2146
Synthesis of Au microwires by selective oxidation of Au-W thin-film composition spreads
Hamann, S. and Brunken, H. and Salomon, S. and Meyer, R. and Savan, A. and Ludwig, Al.
SCIENCE AND TECHNOLOGY OF ADVANCED MATERIALS. Volume: 14 (2013) - 2013 • 2145
Nanoparticle impacts show high-ionic-strength citrate avoids aggregation of silver nanoparticles
Lees, J.C. and Ellison, J. and Batchelor-Mcauley, C. and Tschulik, K. and Damm, C. and Omanovic̈, D. and Compton, R.G.
CHEMPHYSCHEM. Volume: 14 (2013) - 2013 • 2144
Revealing the strain-hardening behavior of twinning-induced plasticity steels: Theory, simulations, experiments
Steinmetz, D.R. and Jäpel, T. and Wietbrock, B. and Eisenlohr, P. and Gutierrez-Urrutia, I. and Saeed-Akbari, A. and Hickel, T. and Roters, F. and Raabe, D.
ACTA MATERIALIA. Volume: 61 (2013) - 2013 • 2143
Elucidating elementary processes at Cu/ZnO interfaces: A microscopical approach
Zychma, A. and Wansing, R. and Schott, V. and Köhler, U. and Wöll, C. and Muhler, M. and Birkner, A.
PHYSICA STATUS SOLIDI (B) BASIC RESEARCH. Volume: 250 (2013) - 2013 • 2142
Mechanocatalytic depolymerization of dry (Ligno)cellulose as an entry process for high-yield production of furfurals
Carrasquillo-Flores, R. and Käldström, M. and Schüth, F. and Dumesic, J.A. and Rinaldi, R.
ACS CATALYSIS. Volume: 3 (2013) - 2013 • 2141
Current-voltage characteristic features of diodes irradiated with 170 MeV xenon ions
Poklonski, N.A. and Gorbachuk, N.I. and Nha, V.Q. and Tarasik, M.I. and Shpakovski, S.V. and Filipenia, V.A. and Skuratov, V.A. and Wieck, A. and Kołtunowicz, T.N.
ACTA PHYSICA POLONICA A. Volume: 123 (2013) - 2013 • 2140
Topologically close-packed phases in binary transition-metal compounds: Matching high-throughput ab initio calculations to an empirical structure map
Hammerschmidt, T. and Bialon, A.F. and Pettifor, D.G. and Drautz, R.
NEW JOURNAL OF PHYSICS. Volume: 15 (2013) - 2013 • 2139
Direct prediction of the desalination performance of porous carbon electrodes for capacitive deionization
Porada, S. and Borchardt, L. and Oschatz, M. and Bryjak, M. and Atchison, J.S. and Keesman, K.J. and Kaskel, S. and Biesheuvel, P.M. and Presser, V.
ENERGY AND ENVIRONMENTAL SCIENCE. Volume: 6 (2013) - 2013 • 2138
Characterization of atmospheric-pressure ac micro-discharge in He-N 2 mixture using time- and space-resolved optical emission spectroscopy
Pothiraja, R. and Ruhrmann, C. and Engelhardt, M. and Bibinov, N. and Awakowicz, P.
JOURNAL OF PHYSICS D: APPLIED PHYSICS. Volume: 46 (2013) - 2013 • 2137
Online monitoring of the passivation breakthrough during deep reactive ion etching of silicon using optical plasma emission spectroscopy
Leopold, S. and Mueller, L. and Kremin, C. and Hoffmann, M.
JOURNAL OF MICROMECHANICS AND MICROENGINEERING. Volume: 23 (2013) - 2013 • 2136
Interface-directed spinodal decomposition in TiAlN/CrN multilayer hard coatings studied by atom probe tomography
Povstugar, I. and Choi, P.-P. and Tytko, D. and Ahn, J.-P. and Raabe, D.
ACTA MATERIALIA. Volume: 61 (2013) - 2013 • 2135
MOEMS tunable microlens made of aluminum nitride membranes
Leopold, S. and Polster, T. and Paetz, D. and Knoebber, F. and Ambacher, O. and Sinzinger, S. and Hoffmann, M.
JOURNAL OF MICRO/NANOLITHOGRAPHY, MEMS, AND MOEMS. Volume: 12 (2013) - 2013 • 2134
Atomic-scale compositional characterization of a nanocrystalline AlCrCuFeNiZn high-entropy alloy using atom probe tomography
Pradeep, K.G. and Wanderka, N. and Choi, P. and Banhart, J. and Murty, B.S. and Raabe, D.
ACTA MATERIALIA. Volume: 61 (2013) - 2013 • 2133
Characterization of low-pressure microwave and radio frequency discharges in oxygen applying optical emission spectroscopy and multipole resonance probe
Steves, S. and Styrnoll, T. and Mitschker, F. and Bienholz, S. and Nikita, B. and Awakowicz, P.
JOURNAL OF PHYSICS D: APPLIED PHYSICS. Volume: 46 (2013) - 2013 • 2132
Microcompression and cyclic deformation behaviors of coaxial copper bicrystals with a single twin boundary
Li, L.L. and An, X.H. and Imrich, P.J. and Zhang, P. and Zhang, Z.J. and Dehm, G. and Zhang, Z.F.
SCRIPTA MATERIALIA. Volume: 69 (2013) - 2013 • 2131
Silicon oxide barrier films deposited on PET foils in pulsed plasmas: Influence of substrate bias on deposition process and film properties
Steves, S. and Ozkaya, B. and Liu, C.-N. and Ozcan, O. and Bibinov, N. and Grundmeier, G. and Awakowicz, P.
JOURNAL OF PHYSICS D: APPLIED PHYSICS. Volume: 46 (2013) - 2013 • 2130
Incipient plasticity and deformation mechanisms in single-crystal Mg during spherical nanoindentation
Catoor, D. and Gao, Y.F. and Geng, J. and Prasad, M.J.N.V. and Herbert, E.G. and Kumar, K.S. and Pharr, G.M. and George, E.P.
ACTA MATERIALIA. Volume: 61 (2013) - 2013 • 2129
Erratum: Checkerboard to stripe charge ordering transition in TbBaFe 2O5 (Physical Review B - Condensed Matter and Materials Physics (2013) 87 (045127))
Pratt, D.K. and Chang, S. and Tian, W. and Taskin, A.A. and Ando, Y. and Zarestky, J.L. and Kreyssig, A. and Goldman, A.I. and McQueeney, R.J.
PHYSICAL REVIEW B - CONDENSED MATTER AND MATERIALS PHYSICS. Volume: 87 (2013) - 2013 • 2128
Atomic engineering of platinum alloy surfaces
Li, T. and Bagot, P.A.J. and Marquis, E.A. and Edman Tsang, S.C. and Smith, G.D.W.
ULTRAMICROSCOPY. Volume: 132 (2013) - 2013 • 2127
Checkerboard to stripe charge ordering transition in TbBaFe 2O5
Pratt, D.K. and Chang, S. and Tian, W. and Taskin, A.A. and Ando, Y. and Zarestky, J.L. and Kreyssig, A. and Goldman, A.I. and McQueeney, R.J.
PHYSICAL REVIEW B - CONDENSED MATTER AND MATERIALS PHYSICS. Volume: 87 (2013) - 2013 • 2126
An imbricate finite element method (i-fem) using full, reduced, and smoothed integration
Cazes, F. and Meschke, G.
COMPUTATIONAL MECHANICS. Volume: 52 (2013) - 2013 • 2125
Atomic scale investigation of redistribution of alloying elements in pearlitic steel wires upon cold-drawing and annealing
Li, Y.J. and Choi, P. and Goto, S. and Borchers, C. and Raabe, D. and Kirchheim, R.
ULTRAMICROSCOPY. Volume: 132 (2013) - 2013 • 2124
Frequency-Stabilized Source of Single Photons from a Solid-State Qubit
Prechtel, J. H. and Kuhlmann, A. V. and Houel, J. and Greuter, L. and Ludwig, Ar. and Reuter, D. and Wieck, A. D. and Warburton, R. J.
PHYSICAL REVIEW X. Volume: 3 (2013) - 2013 • 2123
An edge-based smoothed finite element method for 3D analysis ofsolid mechanics problems
Cazes, F. and Meschke, G.
INTERNATIONAL JOURNAL FOR NUMERICAL METHODS IN ENGINEERING. Volume: 94 (2013) - 2013 • 2122
Evidence of strong correlations and coherence-incoherence crossover in the iron pnictide superconductor KFe2As2
Hardy, F. and Böhmer, A.E. and Aoki, D. and Burger, P. and Wolf, T. and Schweiss, P. and Heid, R. and Adelmann, P. and Yao, Y.X. and Kotliar, G. and Schmalian, J. and Meingast, C.
PHYSICAL REVIEW LETTERS. Volume: 111 (2013) - 2013 • 2121
Triptycene-based, carboxylate-bridged biomimetic diiron(II) complexes
Li, Y. and Soe, C.M.M. and Wilson, J.J. and Tuang, S.L. and Apfel, U.-P. and Lippard, S.J.
EUROPEAN JOURNAL OF INORGANIC CHEMISTRY. Volume: (2013) - 2013 • 2120
A permeation model for the electrochemical interface
Preiss, U. and Borukhovich, E. and Alemayehu, N. and Steinbach, I. and LaMantia, F.
MODELLING AND SIMULATION IN MATERIALS SCIENCE AND ENGINEERING. Volume: 21 (2013) - 2013 • 2119
Finite-time boundedness analysis of a class of linear discrete descriptor systems: An LMI approach
Stojanovic, S.B. and Debeljkovic, D.L. and Nestorovic, T. and Antic, D.S.
2013 3RD INTERNATIONAL CONFERENCE ON SYSTEMS AND CONTROL, ICSC 2013. Volume: (2013) - 2013 • 2118
Bimodal substrate biasing to control gamma-Al2O3 deposition during reactive magnetron sputtering
Prenzel, M. and Kortmann, A. and Stein, A. and von Keudell, A. and Nahif, F. and Schneider, J. M.
JOURNAL OF APPLIED PHYSICS. Volume: 114 (2013) - 2013 • 2117
Further results on stability of singular time delay systems in the sense of non-Lyapunov: A new delay dependent conditions
Stojanovic, S.B. and Debeljkovic, D.L. and Nestorovic, T. and Antic, D.S.
2013 3RD INTERNATIONAL CONFERENCE ON SYSTEMS AND CONTROL, ICSC 2013. Volume: (2013) - 2013 • 2116
Microstructural characterization of porous thermal barrier coatings by IR gas porosimetry and sintering forecasts
Cernuschi, F. and Golosnoy, I.O. and Bison, P. and Moscatelli, A. and Vassen, R. and Bossmann, H.-P. and Capelli, S.
ACTA MATERIALIA. Volume: 61 (2013) - 2013 • 2115
Transmission electron microscopy characterization of CrN films on MgO(001)
Harzer, T.P. and Daniel, R. and Mitterer, C. and Dehm, G. and Zhang, Z.L.
THIN SOLID FILMS. Volume: 545 (2013) - 2013 • 2114
Formation of crystalline gamma-Al2O3 induced by variable substrate biasing during reactive magnetron sputtering
Prenzel, M. and Kortmann, A. and von Keudell, A. and Nahif, F. and Schneider, J. M. and Shihab, M. and Brinkmann, R. P.
JOURNAL OF PHYSICS D-APPLIED PHYSICS. Volume: 46 (2013) - 2013 • 2113
A test for stationarity based on empirical processes
Preuß, P. and Vetter, M. and Dette, H.
BERNOULLI. Volume: 19 (2013) - 2013 • 2112
Transition to Renewable Energy Systems
Stolten, D. and Scherer, V.
TRANSITION TO RENEWABLE ENERGY SYSTEMS. Volume: (2013) - 2013 • 2111
Testing semiparametric hypotheses in locally stationary processes
Preuss, P. and Vetter, M. and Dette, H.
SCANDINAVIAN JOURNAL OF STATISTICS. Volume: 40 (2013) - 2013 • 2110
Polymer modified jute fibre as reinforcing agent controlling the physical and mechanical characteristics of cement mortar
Chakraborty, S. and Kundu, S.P. and Roy, A. and Adhikari, B. and Majumder, S.B.
CONSTRUCTION AND BUILDING MATERIALS. Volume: 49 (2013) - 2013 • 2109
A robust nickel catalyst for cyanomethylation of aldehydes: Activation of acetonitrile under base-free conditions
Chakraborty, S. and Patel, Y.J. and Krause, J.A. and Guan, H.
ANGEWANDTE CHEMIE - INTERNATIONAL EDITION. Volume: 52 (2013) - 2013 • 2108
The formation of zeolites from solution - Analysis by mass spectrometry
Lim, I.H. and Schrader, W. and Schüth, F.
MICROPOROUS AND MESOPOROUS MATERIALS. Volume: 166 (2013) - 2013 • 2107
Hot carrier effects on lateral electron spin diffusion in n-type GaAs
Quast, J.-H. and Henn, T. and Kiessling, T. and Ossau, W. and Molenkamp, L.W. and Reuter, D. and Wieck, A.D.
PHYSICAL REVIEW B - CONDENSED MATTER AND MATERIALS PHYSICS. Volume: 87 (2013) - 2013 • 2106
Effect of jute as fiber reinforcement controlling the hydration characteristics of cement matrix
Chakraborty, S. and Kundu, S.P. and Roy, A. and Adhikari, B. and Majumder, S.B.
INDUSTRIAL AND ENGINEERING CHEMISTRY RESEARCH. Volume: 52 (2013) - 2013 • 2105
Pincer-ligated nickel hydridoborate complexes: The dormant species in catalytic reduction of carbon dioxide with boranes
Chakraborty, S. and Zhang, J. and Patel, Y.J. and Krause, J.A. and Guan, H.
INORGANIC CHEMISTRY. Volume: 52 (2013) - 2013 • 2104
Selective oxidation of ethanol in the liquid phase over Au/TiO2
Heeskens, D. and Aghaei, P. and Kaluza, S. and Strunk, J. and Muhler, M.
PHYSICA STATUS SOLIDI (B) BASIC RESEARCH. Volume: 250 (2013) - 2013 • 2103
A chemical lift-off process: Removing non-specific adsorption in an electrochemical Epstein-Barr virus immunoassay
Stratmann, L. and Gebala, M. and Schuhmann, W.
CHEMPHYSCHEM. Volume: 14 (2013) - 2013 • 2102
Para-aminosalicylic acid acts as an alternative substrate of folate metabolism in Mycobacterium tuberculosis
Chakraborty, S. and Gruber, T. and Barry III, C.E. and Boshoff, H.I. and Rhee, K.Y.
SCIENCE. Volume: 339 (2013) - 2013 • 2101
Combined in situ FTIR-spectroscopic and electrochemical analysis of nanopores in ultra-thin SiOx-like plasma polymer barrier films
Liu, C.-N. and Ozkaya, B. and Steves, S. and Awakowicz, P. and Grundmeier, G.
JOURNAL OF PHYSICS D: APPLIED PHYSICS. Volume: 46 (2013) - 2013 • 2100
Crystal and magnetic structure of CaCo1.86As2 studied by x-ray and neutron diffraction
Quirinale, D.G. and Anand, V.K. and Kim, M.G. and Pandey, A. and Huq, A. and Stephens, P.W. and Heitmann, T.W. and Kreyssig, A. and McQueeney, R.J. and Johnston, D.C. and Goldman, A.I.
PHYSICAL REVIEW B - CONDENSED MATTER AND MATERIALS PHYSICS. Volume: 88 (2013) - 2013 • 2099
Improvement of the mechanical properties of jute fibre reinforced cement mortar: A statistical approach
Chakraborty, S. and Kundu, S.P. and Roy, A. and Basak, R.K. and Adhikari, B. and Majumder, S.B.
CONSTRUCTION AND BUILDING MATERIALS. Volume: 38 (2013) - 2013 • 2098
Influence of substrate surface-induced defects on the interface state between NaCl(100) and Ag(111)
Heidorn, S. and Bertram, C. and Koch, J. and Boom, K. and Matthaei, F. and Safiei, A. and Henzl, J. and Morgenstern, K.
JOURNAL OF PHYSICAL CHEMISTRY C. Volume: 117 (2013) - 2013 • 2097
Segregation engineering enables nanoscale martensite to austenite phase transformation at grain boundaries: A pathway to ductile martensite
Raabe, D. and Sandlöbes, S. and Millán, J. and Ponge, D. and Assadi, H. and Herbig, M. and Choi, P.-P.
ACTA MATERIALIA. Volume: 61 (2013) - 2013 • 2096
Electrochemical detection of commercial silver nanoparticles: Identification, sizing and detection in environmental media
Stuart, E.J.E. and Tschulik, K. and Omanović, D. and Cullen, J.T. and Jurkschat, K. and Crossley, A. and Compton, R.G.
NANOTECHNOLOGY. Volume: 24 (2013) - 2013 • 2095
Bimetallic aerogels: High-performance electrocatalysts for the oxygen reduction reaction
Liu, W. and Rodriguez, P. and Borchardt, L. and Foelske, A. and Yuan, J. and Herrmann, A.-K. and Geiger, D. and Zheng, Z. and Kaskel, S. and Gaponik, N. and Kötz, R. and Schmidt, T.J. and Eychmüller, A.
ANGEWANDTE CHEMIE - INTERNATIONAL EDITION. Volume: 52 (2013) - 2013 • 2094
Interface Segregation in Advanced Steels Studied at the Atomic Scale
Raabe, D. and Ponge, D. and Kirchheim, R. and Assadi, H. and Li, Y. and Goto, S. and Kostka, A. and Herbig, M. and Sandl, S. and Kuzmina, M. and Millán, J. and Yuan, L. and Choi, P.-P.
MICROSTRUCTURAL DESIGN OF ADVANCED ENGINEERING MATERIALS. Volume: (2013) - 2013 • 2093
Process diagnostics and monitoring using the multipole resonance probe in an inhomogeneous plasma for ion-assisted deposition of optical coatings
Styrnoll, T. and Harhausen, J. and Lapke, M. and Storch, R. and Brinkmann, R.P. and Foest, R. and Ohl, A. and Awakowicz, P.
PLASMA SOURCES SCIENCE AND TECHNOLOGY. Volume: 22 (2013) - 2013 • 2092
Effect of high pressure and high temperature on the microstructural evolution of a single crystal Ni-based superalloy
Lopez-Galilea, I. and Huth, S. and Theisen, W. and Fockenberg, T. and Chakraborty, S.
JOURNAL OF MATERIALS SCIENCE. Volume: 48 (2013) - 2013 • 2091
Bending rotation HCF testing of pseudoelastic Ni-Ti shape memory alloys
Rahim, M. and Frenzel, J. and Frotscher, M. and Heuwer, B. and Hiebeler, J. and Eggeler, G.
MATERIALWISSENSCHAFT UND WERKSTOFFTECHNIK. Volume: 44 (2013) - 2013 • 2090
Impurity levels and fatigue lives of pseudoelastic NiTi shape memory alloys
Rahim, M. and Frenzel, J. and Frotscher, M. and Pfetzing-Micklich, J. and Steegmüller, R. and Wohlschlögel, M. and Mughrabi, H. and Eggeler, G.
ACTA MATERIALIA. Volume: 61 (2013) - 2013 • 2089
Fabrication of Borassus fruit lignocellulose fiber/PP composites and comparison with jute, sisal and coir fibers
Sudhakara, P. and Jagadeesh, D. and Wang, Y. and Venkata Prasad, C. and Devi, A.P.K. and Balakrishnan, G. and Kim, B.S. and Song, J.I.
CARBOHYDRATE POLYMERS. Volume: 98 (2013) - 2013 • 2088
Ionization by bulk heating of electrons in capacitive radio frequency atmospheric pressure microplasmas
Hemke, T. and Eremin, D. and Mussenbrock, T. and Derzsi, A. and Donkó, Z. and Dittmann, K. and Meichsner, J. and Schulze, J.
PLASMA SOURCES SCIENCE AND TECHNOLOGY. Volume: 22 (2013) - 2013 • 2087
A simple stochastic model for yielding in specimens with limited number of dislocations
Sudharshan Phani, P. and Johanns, K.E. and George, E.P. and Pharr, G.M.
ACTA MATERIALIA. Volume: 61 (2013) - 2013 • 2086
Ab initio study of thermodynamic, electronic, magnetic, structural, and elastic properties of Ni4N allotropes
Hemzalová, P. and Friák, M. and Šob, M. and Ma, D. and Udyansky, A. and Raabe, D. and Neugebauer, J.
PHYSICAL REVIEW B - CONDENSED MATTER AND MATERIALS PHYSICS. Volume: 88 (2013) - 2013 • 2085
Nanocarbon Paste Electrodes
Lowinsohn, D. and Gan, P. and Tschulik, K. and Foord, J.S. and Compton, R.G.
ELECTROANALYSIS. Volume: 25 (2013) - 2013 • 2084
Electrochemical micromachining of passive electrodes
Sueptitz, R. and Dunne, P. and Tschulik, K. and Uhlemann, M. and Eckert, J. and Gebert, A.
ELECTROCHIMICA ACTA. Volume: 109 (2013) - 2013 • 2083
Atomistic aspects of 〈1 1 1〉 screw dislocation behavior in α-iron and the derivation of microscopic yield criterion
Chen, Z.M. and Mrovec, M. and Gumbsch, P.
MODELLING AND SIMULATION IN MATERIALS SCIENCE AND ENGINEERING. Volume: 21 (2013) - 2013 • 2082
Mixed aerogels from Au and CdTe nanoparticles
Hendel, T. and Lesnyak, V. and Kühn, L. and Herrmann, A.-K. and Bigall, N.C. and Borchardt, L. and Kaskel, S. and Gaponik, N. and Eychmüller, A.
ADVANCED FUNCTIONAL MATERIALS. Volume: 23 (2013) - 2013 • 2081
Aging-associated enzyme human clock-1: Substrate-mediated reduction of the diiron center for 5-demethoxyubiquinone hydroxylation
Lu, T.-T. and Lee, S.J. and Apfel, U.-P. and Lippard, S.J.
BIOCHEMISTRY. Volume: 52 (2013) - 2013 • 2080
Two-dimensional magnetic interactions in LaFeAsO
Ramazanoglu, M. and Lamsal, J. and Tucker, G.S. and Yan, J.-Q. and Calder, S. and Guidi, T. and Perring, T. and McCallum, R.W. and Lograsso, T.A. and Kreyssig, A. and Goldman, A.I. and McQueeney, R.J.
PHYSICAL REVIEW B - CONDENSED MATTER AND MATERIALS PHYSICS. Volume: 87 (2013) - 2013 • 2079
Purified oxygen- and nitrogen-modified multi-walled carbon nanotubes as metal-free catalysts for selective olefin hydrogenation
Chen, P. and Chew, L.M. and Kostka, A. and Xie, K. and Muhler, M. and Xia, W.
JOURNAL OF ENERGY CHEMISTRY. Volume: 22 (2013) - 2013 • 2078
Massive anisotropic thermal expansion and thermo-responsive breathing in metal-organic frameworks modulated by linker functionalization
Henke, S. and Schneemann, A. and Fischer, R.A.
ADVANCED FUNCTIONAL MATERIALS. Volume: 23 (2013) - 2013 • 2077
Vibrational sum frequency generation spectroscopy of the water liquid-vapor interface from density functional theory-based molecular dynamics simulations
Sulpizi, M. and Salanne, M. and Sprik, M. and Gaigeot, M.-P.
JOURNAL OF PHYSICAL CHEMISTRY LETTERS. Volume: 4 (2013) - 2013 • 2076
The structural and electronic promoting effect of nitrogen-doped carbon nanotubes on supported Pd nanoparticles for selective olefin hydrogenation
Chen, P. and Chew, L.M. and Kostka, A. and Muhler, M. and Xia, W.
CATALYSIS SCIENCE AND TECHNOLOGY. Volume: 3 (2013) - 2013 • 2075
Picosecond real-space imaging of electron spin diffusion in GaAs
Henn, T. and Kiessling, T. and Ossau, W. and Molenkamp, L.W. and Reuter, D. and Wieck, A.D.
PHYSICAL REVIEW B - CONDENSED MATTER AND MATERIALS PHYSICS. Volume: 88 (2013) - 2013 • 2074
Interaction effects and transport properties of Pt capped Co nanoparticles
Ludwig, Ar. and Agudo, L. and Eggeler, G. and Ludwig, Al. and Wieck, A.D. and Petracic, O.
JOURNAL OF APPLIED PHYSICS. Volume: 113 (2013) - 2013 • 2073
Ag-stabilized few-layer graphene dispersions in low boiling point solvents for versatile nonlinear optical applications
Sun, Z. and Dong, N. and Wang, K. and König, D. and Nagaiah, T.C. and Sánchez, M.D. and Ludwig, Al. and Cheng, X. and Schuhmann, W. and Wang, J. and Muhler, M.
CARBON. Volume: 62 (2013) - 2013 • 2072
Hot carrier effects on the magneto-optical detection of electron spins in GaAs
Henn, T. and Heckel, A. and Beck, M. and Kiessling, T. and Ossau, W. and Molenkamp, L.W. and Reuter, D. and Wieck, A.D.
PHYSICAL REVIEW B - CONDENSED MATTER AND MATERIALS PHYSICS. Volume: 88 (2013) - 2013 • 2071
Nanostructured few-layer graphene with superior optical limiting properties fabricated by a catalytic steam etching process
Sun, Z. and Dong, N. and Xie, K. and Xia, W. and König, D. and Nagaiah, T.C. and Sánchez, M.D. and Ebbinghaus, P. and Erbe, A. and Zhang, X. and Ludwig, Al. and Schuhmann, W. and Wang, J. and Muhler, M.
JOURNAL OF PHYSICAL CHEMISTRY C. Volume: 117 (2013) - 2013 • 2070
Nanocrystalline Fe-C alloys produced by ball milling of iron and graphite
Chen, Y.Z. and Herz, A. and Li, Y.J. and Borchers, C. and Choi, P. and Raabe, D. and Kirchheim, R.
ACTA MATERIALIA. Volume: 61 (2013) - 2013 • 2069
High-yield exfoliation of graphite in acrylate polymers: A stable few-layer graphene nanofluid with enhanced thermal conductivity
Sun, Z. and Pöller, S. and Huang, X. and Guschin, D. and Taetz, C. and Ebbinghaus, P. and Masa, J. and Erbe, A. and Kilzer, A. and Schuhmann, W. and Muhler, M.
CARBON. Volume: 64 (2013) - 2013 • 2068
Eu-doped ZnO nanowire arrays grown by electrodeposition
Lupan, O. and Pauporté, T. and Viana, B. and Aschehoug, P. and Ahmadi, M. and Cuenya, B.R. and Rudzevich, Y. and Lin, Y. and Chow, L.
APPLIED SURFACE SCIENCE. Volume: 282 (2013) - 2013 • 2067
The influence of the residual growth catalyst in functionalized carbon nanotubes on supported Pt nanoparticles applied in selective olefin hydrogenation
Chen, P. and Chew, L.M. and Xia, W.
JOURNAL OF CATALYSIS. Volume: 307 (2013) - 2013 • 2066
Preparation of thin film Cu-Pt(1 1 1) near-surface alloys: One small step towards up-scaling model single crystal surfaces
Henry, J.B. and Maljusch, A. and Tymoczko, J. and Schuhmann, W. and Bandarenka, A.S.
ELECTROCHIMICA ACTA. Volume: 112 (2013) - 2013 • 2065
Reorientation of a single bond within an adsorbed molecule by tunneling electrons
Henzl, J. and Boom, K. and Morgenstern, K.
JOURNAL OF THE AMERICAN CHEMICAL SOCIETY. Volume: 135 (2013) - 2013 • 2064
A disposable sticky electrode for the detection of commercial silver NPs in seawater
Cheng, W. and Stuart, E.J.E. and Tschulik, K. and Cullen, J.T. and Compton, R.G.
NANOTECHNOLOGY. Volume: 24 (2013) - 2013 • 2063
Hidden surface states at non-polar GaN (101̄0) facets: Intrinsic pinning of nanowires
Lymperakis, L. and Weidlich, P.H. and Eisele, H. and Schnedler, M. and Nys, J.-P. and Grandidier, B. and Stiévenard, D. and Dunin-Borkowski, R.E. and Neugebauer, J. and Ebert, Ph.
APPLIED PHYSICS LETTERS. Volume: 103 (2013) - 2013 • 2062
Fast and efficient single electron transfer between distant quantum dots
Hermelin, S. and Takada, S. and Yamamoto, M. and Tarucha, S. and Wieck, A.D. and Saminadayar, L. and Bäuerle, C. and Meunier, T.
JOURNAL OF APPLIED PHYSICS. Volume: 113 (2013) - 2013 • 2061
Density functional theory simulation of liquid helium-4 in aerogel
Lysogorskiy, Y.V. and Tayurskii, D.A.
JETP LETTERS. Volume: 98 (2013) - 2013 • 2060
Molybdenum-based catalysts for the decomposition of ammonia: In situ X-ray diffraction studies, microstructure, and catalytic properties
Tagliazucca, V. and Schlichte, K. and Schüth, F. and Weidenthaler, C.
JOURNAL OF CATALYSIS. Volume: 305 (2013) - 2013 • 2059
Comparison of insulin aspart vs. regular human insulin with or without insulin detemir concerning adipozytokines and metabolic effects in patients with type 2 diabetes mellitus
Herrmann, B.L. and Kasser, C. and Keuthage, W. and Huptas, M. and Dette, H. and Klute, A.
EXPERIMENTAL AND CLINICAL ENDOCRINOLOGY AND DIABETES. Volume: 121 (2013) - 2013 • 2058
Iron metal-organic frameworks MIL-88B and NH2-MIL-88B for the loading and delivery of the gasotransmitter carbon monoxide
Ma, M. and Noei, H. and Mienert, B. and Niesel, J. and Bill, E. and Muhler, M. and Fischer, R.A. and Wang, Y. and Schatzschneider, U. and Metzler-Nolte, N.
CHEMISTRY - A EUROPEAN JOURNAL. Volume: 19 (2013) - 2013 • 2057
Growth and crystallization of TiO2 thin films by atomic layer deposition using a novel amido guanidinate titanium source and tetrakis-dimethylamido-titanium
Reiners, M. and Xu, K. and Aslam, N. and Devi, A. and Waser, R. and Hoffmann-Eifert, S.
CHEMISTRY OF MATERIALS. Volume: 25 (2013) - 2013 • 2056
Ab initio calculation of traction separation laws for a grain boundary in molybdenum with segregated C impurites
Tahir, A.M. and Janisch, R. and Hartmaier, A.
MODELLING AND SIMULATION IN MATERIALS SCIENCE AND ENGINEERING. Volume: 21 (2013) - 2013 • 2055
Synthesis and characterization of Cu-doped ZnO one-dimensional structures for miniaturized sensor applications with faster response
Chow, L. and Lupan, O. and Chai, G. and Khallaf, H. and Ono, L.K. and Roldan Cuenya, B. and Tiginyanu, I.M. and Ursaki, V.V. and Sontea, V. and Schulte, A.
SENSORS AND ACTUATORS, A: PHYSICAL. Volume: 189 (2013) - 2013 • 2054
Ab initio identified design principles of solid-solution strengthening in Al
Ma, D. and Friák, M. and Pezold, J.V. and Raabe, D. and Neugebauer, J.
SCIENCE AND TECHNOLOGY OF ADVANCED MATERIALS. Volume: 14 (2013) - 2013 • 2053
Development of the sputtering yields of ArF photoresist after the onset of argon ion bombardment
Takeuchi, T. and Corbella, C. and Grosse-Kreul, S. and von Keudell, A. and Ishikawa, K. and Kondo, H. and Takeda, K. and Sekine, M. and Hori, M.
JOURNAL OF APPLIED PHYSICS. Volume: 113 (2013) - 2013 • 2052
Comparative studies on the accumulation of strain and recovery ratio of Veriflex®, a shape-memory polymer: Infrared and laser experiments
Chowdhury, A.M.S. and Schmidt, C. and Neuking, K. and Eggeler, G.
HIGH PERFORMANCE POLYMERS. Volume: 25 (2013) - 2013 • 2051
Adherent cells avoid polarization gradients on periodically poled LiTaO3 ferroelectrics
Christophis, C. and Cavalcanti-Adam, E.A. and Hanke, M. and Kitamura, K. and Gruverman, A. and Grunze, M. and Dowben, P.A. and Rosenhahn, A.
BIOINTERPHASES. Volume: 8 (2013) - 2013 • 2050
Single pulse coherence measurements in the water window at the free-electron laser FLASH
Mai, D.D. and Hallmann, J. and Reusch, T. and Osterhoff, M. and Dusterer, S. and Treusch, R. and Singer, A. and Beckers, M. and Gorniak, T. and Senkbeil, T. and Dronyak, R. and Gulden, J. and Yefanov, O.M. and Al-Shemmary, A. and Rosenhahn, A. and Mancuso, A.P. and Vartanyants, I.A. and Salditt, T.
OPTICS EXPRESS. Volume: 21 (2013) - 2013 • 2049
Cyclic deformation and lifetime of Alloy 617B during thermo-mechanical fatigue
Maier, G. and Riedel, H. and Nieweg, B. and Somsen, C. and Eggeler, G. and Klöwer, J. and Mohrmann, R.
MATERIALS AT HIGH TEMPERATURES. Volume: 30 (2013) - 2013 • 2048
Cyclic deformation and lifetime of Alloy 617B during isothermal low cycle fatigue
Maier, G. and Riedel, H. and Somsen, C.
INTERNATIONAL JOURNAL OF FATIGUE. Volume: 55 (2013) - 2013 • 2047
An elevated temperature study of a Ti adhesion layer on polyimide
Taylor, A.A. and Cordill, M.J. and Bowles, L. and Schalko, J. and Dehm, G.
THIN SOLID FILMS. Volume: 531 (2013) - 2013 • 2046
Single-particle fluctuations and directional correlations in driven hard-sphere glasses
Mandal, S. and Chikkadi, V. and Nienhuis, B. and Raabe, D. and Schall, P. and Varnik, F.
PHYSICAL REVIEW E - STATISTICAL, NONLINEAR, AND SOFT MATTER PHYSICS. Volume: 88 (2013) - 2013 • 2045
Flow heterogeneity and correlations in a sheared hard sphere glass: Insight from computer simulations
Mandal, S. and Gross, M. and Raabe, D. and Varnik, F.
AIP CONFERENCE PROCEEDINGS. Volume: 1518 (2013) - 2013 • 2044
Atom Probe Tomography Studies on the Cu(In,Ga)Se-2 Grain Boundaries
Cojocaru-Miredin, O. and Schwarz, T. and Choi, P. P. and Herbig, M. and Wuerz, R. and Raabe, D.
JOVE-JOURNAL OF VISUALIZED EXPERIMENTS. Volume: (2013) - 2013 • 2043
Parallel simulation of brownian dynamics on shared memory systems with OpenMP and unified parallel C
Teijeiro, C. and Sutmann, G. and Taboada, G.L. and Touriño, J.
JOURNAL OF SUPERCOMPUTING. Volume: 65 (2013) - 2013 • 2042
Mechanocatalytic depolymerization of cellulose combined with hydrogenolysis as a highly efficient pathway to sugar alcohols
Hilgert, J. and Meine, N. and Rinaldi, R. and Schüth, F.
ENERGY AND ENVIRONMENTAL SCIENCE. Volume: 6 (2013) - 2013 • 2041
Structural mimicking of inorganic catalyst supports with polydivinylbenzene to improve performance in the selective aerobic oxidation of ethanol and glycerol in water
Richter, F.H. and Meng, Y. and Klasen, T. and Sahraoui, L. and Schüth, F.
JOURNAL OF CATALYSIS. Volume: 308 (2013) - 2013 • 2040
Parallel Brownian dynamics simulations with the message-passing and PGAS programming models
Teijeiro, C. and Sutmann, G. and Taboada, G.L. and Touriño, J.
COMPUTER PHYSICS COMMUNICATIONS. Volume: 184 (2013) - 2013 • 2039
Particle beam experiments for the analysis of reactive sputtering processes in metals and polymer surfaces
Corbella, C. and Grosse-Kreul, S. and Kreiter, O. and de los Arcos, T. and Benedikt, J. and von Keudell, A.
REVIEW OF SCIENTIFIC INSTRUMENTS. Volume: 84 (2013) - 2013 • 2038
GaN(0001) surface states: Experimental and theoretical fingerprints to identify surface reconstructions
Himmerlich, M. and Lymperakis, L. and Gutt, R. and Lorenz, P. and Neugebauer, J. and Krischok, S.
PHYSICAL REVIEW B - CONDENSED MATTER AND MATERIALS PHYSICS. Volume: 88 (2013) - 2013 • 2037
Nonlinear evolution of surface morphology under shadowing
Manz, P. and Fedorczak, N. and Dittmar, T. and Baloniak, T. and von Keudell, A.
PHYSICAL REVIEW E. Volume: 87 (2013) - 2013 • 2036
Set of acidic resin catalysts to correlate structure and reactivity in fructose conversion to 5-hydroxymethylfurfural
Richter, F.H. and Pupovac, K. and Palkovits, R. and Schüth, F.
ACS CATALYSIS. Volume: 3 (2013) - 2013 • 2035
Multi-scale correlative microscopy investigation of both structure and chemistry of deformation twin bundles in Fe-Mn-C steel
Marceau, R.K.W. and Gutierrez-Urrutia, I. and Herbig, M. and Moore, K.L. and Lozano-Perez, S. and Raabe, D.
MICROSCOPY AND MICROANALYSIS. Volume: 19 (2013) - 2013 • 2034
Understanding the detection of carbon in austenitic high-Mn steel using atom probe tomography
Marceau, R.K.W. and Choi, P. and Raabe, D.
ULTRAMICROSCOPY. Volume: 132 (2013) - 2013 • 2033
Coupled DEM/CFD simulation of heat transfer in a generic grate system agitated by bars
Rickelt, S. and Sudbrock, F. and Wirtz, S. and Scherer, V.
POWDER TECHNOLOGY. Volume: 249 (2013) - 2013 • 2032
Renewable pencil electrodes for highly sensitive anodic stripping voltammetric determination of 3-hydroxyflavone and morin in bulk form and in biological fluids
Temerk, Y.M. and Ibrahim, M.S. and Kotb, M. and Schuhmann, W.
ELECTROANALYSIS. Volume: 25 (2013) - 2013 • 2031
Metal nanoparticle catalysts beginning to shape-up
Cuenya, B.R.
ACCOUNTS OF CHEMICAL RESEARCH. Volume: 46 (2013) - 2013 • 2030
Interaction of antitumor flavonoids with dsDNA in the absence and presence of Cu(II)
Temerk, Y.M. and Ibrahim, M.S. and Kotb, M. and Schuhmann, W.
ANALYTICAL AND BIOANALYTICAL CHEMISTRY. Volume: 405 (2013) - 2013 • 2029
Shape-selected bimetallic nanoparticle electrocatalysts: Evolution of their atomic-scale structure, chemical composition, and electrochemical reactivity under various chemical environments
Cui, C. and Ahmadi, M. and Behafarid, F. and Gan, L. and Neumann, M. and Heggen, M. and Cuenya, B.R. and Strasser, P.
FARADAY DISCUSSIONS. Volume: 162 (2013) - 2013 • 2028
Anisotropic transport and magnetic properties and magnetic-field tuned states of CeZn11 single crystals
Hodovanets, H. and Bud'Ko, S.L. and Lin, X. and Taufour, V. and Kim, M.G. and Pratt, D.K. and Kreyssig, A. and Canfield, P.C.
PHYSICAL REVIEW B - CONDENSED MATTER AND MATERIALS PHYSICS. Volume: 88 (2013) - 2013 • 2027
Scanning droplet cell microscopy on a wide range hafnium-niobium thin film combinatorial library
Mardare, A.I. and Ludwig, Al. and Savan, A. and Hassel, A.W.
ELECTROCHIMICA ACTA. Volume: 110 (2013) - 2013 • 2026
Three-Dimensional Diagnosis and Treatment Planning of Dentoalveolar Problems
Ritter, L. and Neugebauer, J. and Mischkowski, R. and Scheer, M. and Zöller, J.E.
THREE-DIMENSIONAL IMAGING FOR ORTHODONTICS AND MAXILLOFACIAL SURGERY. Volume: (2013) - 2013 • 2025
Experimental and numerical investigation on the influence of particle shape and shape approximation on hopper discharge using the discrete element method
Höhner, D. and Wirtz, S. and Scherer, V.
POWDER TECHNOLOGY. Volume: 235 (2013) - 2013 • 2024
High capacity micro-mesoporous carbon-sulfur nanocomposite cathodes with enhanced cycling stability prepared by a solvent-free procedure
Thieme, S. and Brückner, J. and Bauer, I. and Oschatz, M. and Borchardt, L. and Althues, H. and Kaskel, S.
JOURNAL OF MATERIALS CHEMISTRY A. Volume: 1 (2013) - 2013 • 2023
Blocking growth by an electrically active subsurface layer: The effect of si as an antisurfactant in the growth of GaN
Markurt, T. and Lymperakis, L. and Neugebauer, J. and Drechsel, P. and Stauss, P. and Schulz, T. and Remmele, T. and Grillo, V. and Rotunno, E. and Albrecht, M.
PHYSICAL REVIEW LETTERS. Volume: 110 (2013) - 2013 • 2022
Conceptual design of a dielectric hemispherical lens antenna with a congruent radiation pattern for beam steering applications
Dahl, C. and Will, B. and Schulz, C. and Rolfes, I. and Baer, C. and Musch, T.
IEEE ANTENNAS AND PROPAGATION SOCIETY, AP-S INTERNATIONAL SYMPOSIUM (DIGEST). Volume: (2013) - 2013 • 2021
Spin injection, transport, and relaxation in spin light-emitting diodes: Magnetic field effects
Höpfner, H. and Fritsche, C. and Ludwig, Ar. and Ludwig, As. and Stromberg, F. and Wende, H. and Keune, W. and Reuter, D. and Wieck, A.D. and Gerhardt, N.C. and Hofmann, M.R.
PROCEEDINGS OF SPIE - THE INTERNATIONAL SOCIETY FOR OPTICAL ENGINEERING. Volume: 8813 (2013) - 2013 • 2020
Polarization effects due to thickness fluctuations in nonpolar InGaN/GaN quantum wells
Marquardt, O. and Hickel, T. and Neugebauer, J. and Van De Walle, C.G.
APPLIED PHYSICS LETTERS. Volume: 103 (2013) - 2013 • 2019
Spin relaxation length in quantum dot spin LEDs
Höpfner, H. and Fritsche, C. and Ludwig, Ar. and Ludwig, As. and Stromberg, F. and Wende, H. and Keune, W. and Reuter, D. and Wieck, A.D. and Gerhardt, N.C. and Hofmann, M.R.
PHYSICA STATUS SOLIDI (C) CURRENT TOPICS IN SOLID STATE PHYSICS. Volume: 10 (2013) - 2013 • 2018
A thermodynamic approach for the development of austenitic steels with a high resistance to hydrogen gas embrittlement
Martín, M. and Weber, S. and Theisen, W.
INTERNATIONAL JOURNAL OF HYDROGEN ENERGY. Volume: 38 (2013) - 2013 • 2017
Spin relaxation in spin light-emitting diodes: Effects of magnetic field and temperature
Höpfner, H. and Fritsche, C. and Ludwig, Ar. and Ludwig, As. and Stromberg, F. and Wende, H. and Keune, W. and Reuter, D. and Wieck, A.D. and Gerhardt, N.C. and Hofmann, M.R.
PROCEEDINGS OF SPIE - THE INTERNATIONAL SOCIETY FOR OPTICAL ENGINEERING. Volume: 8623 (2013) - 2013 • 2016
Development of a stable high-aluminum austenitic stainless steel for hydrogen applications
Martin, M. and Weber, S. and Theisen, W. and Michler, T. and Naumann, J.
INTERNATIONAL JOURNAL OF HYDROGEN ENERGY. Volume: 38 (2013) - 2013 • 2015
Lignocellulosic jute fiber as a bioadsorbent for the removal of azo dye from its aqueous solution: Batch and column studies
Roy, A. and Chakraborty, S. and Kundu, S.P. and Adhikari, B. and Majumder, S.B.
JOURNAL OF APPLIED POLYMER SCIENCE. Volume: 129 (2013) - 2013 • 2014
Titanium carbide and carbide-derived carbon composite nanofibers by electrospinning of Ti-resin precursor
Martin, J.R. and Borchardt, L. and Oschatz, M. and Mondin, G. and Kaskel, S.
CHEMIE-INGENIEUR-TECHNIK. Volume: 85 (2013) - 2013 • 2013
Surface grafting of Corchorus olitorius fibre: A green approach for the development of activated bioadsorbent
Roy, A. and Chakraborty, S. and Kundu, S.P. and Majumder, S.B. and Adhikari, B.
CARBOHYDRATE POLYMERS. Volume: 92 (2013) - 2013 • 2012
Solutal gradients in strained equilibrium
Darvishi Kamachali, R. and Borukhovich, E. and Shchyglo, O. and Steinbach, I.
PHILOSOPHICAL MAGAZINE LETTERS. Volume: 93 (2013) - 2013 • 2011
Tuning the Reactivity of a Cu/ZnO Nanocatalyst via Gas Phase Pressure
Martinez-Suarez, L. and Frenzel, J. and Marx, D. and Meyer, B.
PHYSICAL REVIEW LETTERS. Volume: 110 (2013) - 2013 • 2010
Trace metal residues promote the activity of supposedly metal-free nitrogen-modified carbon catalysts for the oxygen reduction reaction
Masa, J. and Zhao, A. and Xia, W. and Sun, Z. and Mei, B. and Muhler, M. and Schuhmann, W.
ELECTROCHEMISTRY COMMUNICATIONS. Volume: 34 (2013) - 2013 • 2009
Timescales of crustal melting in the Higher Himalayan Crystallines (Sikkim, Eastern Himalaya) inferred from trace element-constrained monazite and zircon chronology
Rubatto, D. and Chakraborty, S. and Dasgupta, S.
CONTRIBUTIONS TO MINERALOGY AND PETROLOGY. Volume: 165 (2013) - 2013 • 2008
Elastoplastic buckling as source of misinterpretation of micropillar tests
Daum, B. and Dehm, G. and Clemens, H. and Rester, M. and Fischer, F.D. and Rammerstorfer, F.G.
ACTA MATERIALIA. Volume: 61 (2013) - 2013 • 2007
Fundamental studies on the electrocatalytic properties of metal macrocyclics and other complexes for the electroreduction of O2
Masa, J. and Ozoemena, K.I. and Schuhmann, W. and Zagal, J.H.
LECTURE NOTES IN ENERGY. Volume: 9 (2013) - 2013 • 2006
Dyakonov-perel electron spin relaxation in a highly degenerate wurtzite semiconductor
Rudolph, J. and Buß, J.H. and Semond, F. and Hägele, D.
AIP CONFERENCE PROCEEDINGS. Volume: 1566 (2013) - 2013 • 2005
Catalytic applications of OSDA-free Beta zeolite
De Baerdemaeker, T. and Yilmaz, B. and Müller, U. and Feyen, M. and Xiao, F.-S. and Zhang, W. and Tatsumi, T. and Gies, H. and Bao, X. and De Vos, D.
JOURNAL OF CATALYSIS. Volume: 308 (2013) - 2013 • 2004
Systematic selection of metalloporphyrin-based catalysts for oxygen reduction by modulation of the donor-acceptor intermolecular hardness
Masa, J. and Schuhmann, W.
CHEMISTRY - A EUROPEAN JOURNAL. Volume: 19 (2013) - 2013 • 2003
Femtosecond laser ablation of ITO/ZnO for thin film solar cells
Abreu Fernandes, S. and Schoeps, B. and Kowalick, K. and Nett, R. and Esen, C. and Pickshaus, M. and Ostendorf, A.
PHYSICS PROCEDIA. Volume: 41 (2013) - 2013 • 2002
Gas-phase oxidation of 2-propanol over Au/TiO2 catalysts to probe metal-support interactions
Holz, M.C. and Kähler, K. and Tölle, K. and van Veen, A.C. and Muhler, M.
PHYSICA STATUS SOLIDI (B) BASIC RESEARCH. Volume: 250 (2013) - 2013 • 2001
Insight into the Reaction Scheme of SiO2 Film Deposition at Atmospheric Pressure
Rugner, K. and Reuter, R. and Ellerweg, D. and de los Arcos, T. and von Keudell, A. and Benedikt, J.
PLASMA PROCESSES AND POLYMERS. Volume: 10 (2013) - 2013 • 2000
Combining emission and absorption spectroscopy at rare earth spectral lines: Plasma temperature measurements in ceramic metal halide lamps
Ruhrmann, C. and Westermeier, M. and Höbing, T. and Bergner, A. and Denissen, C. and Suijker, J. and Awakowicz, P. and Mentel, J.
JOURNAL OF PHYSICS D: APPLIED PHYSICS. Volume: 46 (2013) - 2013 • 1999
High-temperature and low-stress creep anisotropy of single-crystal superalloys
Agudo Jácome, L. and Nörtershäuser, P. and Heyer, J.-K. and Lahni, A. and Frenzel, J. and Dlouhy, A. and Somsen, C. and Eggeler, G.
ACTA MATERIALIA. Volume: 61 (2013) - 2013 • 1998
Particle stimulated nucleation in coarse-grained ferritic stainless steel
De Siqueira, R.P. and Sandim, H.R.Z. and Raabe, D.
METALLURGICAL AND MATERIALS TRANSACTIONS A: PHYSICAL METALLURGY AND MATERIALS SCIENCE. Volume: 44 (2013) - 2013 • 1997
Electrochemical formation and surface characterisation of Cu2-x Te thin films with adjustable content of Cu
Huang, M. and Maljusch, A. and Calle-Vallejo, F. and Henry, J.B. and Koper, M.T.M. and Schuhmann, W. and Bandarenka, A.S.
RSC ADVANCES. Volume: 3 (2013) - 2013 • 1996
Process development and coating characteristics of plasma spray-PVD
Mauer, G. and Hospach, A. and Vaßen, R.
SURFACE AND COATINGS TECHNOLOGY. Volume: 220 (2013) - 2013 • 1995
Optimal Designs for Regression Models with a Constant Coefficient of Variation
Dette, H. and Müller, W.G.
JOURNAL OF STATISTICAL THEORY AND PRACTICE. Volume: 7 (2013) - 2013 • 1994
Plasma spraying of efficient photoactive TiO2 coatings
Mauer, G. and Guignard, A. and Vaßen, R.
SURFACE AND COATINGS TECHNOLOGY. Volume: 220 (2013) - 2013 • 1993
Long-range segregation phenomena in shape-selected bimetallic nanoparticles: Chemical state effects
Ahmadi, M. and Behafarid, F. and Cui, C. and Strasser, P. and Cuenya, B.R.
ACS NANO. Volume: 7 (2013) - 2013 • 1992
Comments on: An updated review of Goodness-of-Fit tests for regression models
Dette, H.
TEST. Volume: 22 (2013) - 2013 • 1991
Plasma-sprayed thermal barrier coatings: New materials, processing issues, and Solutions
Mauer, G. and Jarligo, M.O. and Mack, D.E. and Vaßen, R.
JOURNAL OF THERMAL SPRAY TECHNOLOGY. Volume: 22 (2013) - 2013 • 1990
Robust T-optimal discriminating designs
Dette, H. and Melas, V.B. and Shpilev, P.
ANNALS OF STATISTICS. Volume: 41 (2013) - 2013 • 1989
Process conditions and microstructures of ceramic coatings by gas phase deposition based on plasma spraying
Mauer, G. and Hospach, A. and Zotov, N. and Vaßen, R.
JOURNAL OF THERMAL SPRAY TECHNOLOGY. Volume: 22 (2013) - 2013 • 1988
Evolution of the multiband Ruderman-Kittel-Kasuya-Yosida interaction: Application to iron pnictides and chalcogenides
Akbari, A. and Thalmeier, P. and Eremin, I.
NEW JOURNAL OF PHYSICS. Volume: 15 (2013) - 2013 • 1987
Complete classes of designs for nonlinear regression models and principal representations of moment spaces
Dette, H. and Schorning, K.
ANNALS OF STATISTICS. Volume: 41 (2013) - 2013 • 1986
Investigation and comparison of in-flight particle velocity during the plasma-spray process as measured by laser doppler anemometry and DPV-2000
Mauer, G. and Vaßen, R. and Zimmermann, S. and Biermordt, T. and Heinrich, M. and Marques, J.-L. and Landes, K. and Schein, J.
JOURNAL OF THERMAL SPRAY TECHNOLOGY. Volume: 22 (2013) - 2013 • 1985
High temperature wear testing of a Ni-based superalloy pin on a cast iron disc
Rynio, C. and Hattendorf, H. and Klöwer, J. and Lüdecke, H.-G. and Eggeler, G.
MATERIALWISSENSCHAFT UND WERKSTOFFTECHNIK. Volume: 44 (2013) - 2013 • 1984
Theory of nonequilibrium dynamics of multiband superconductors
Akbari, A. and Schnyder, A.P. and Manske, D. and Eremin, I.
EPL. Volume: 101 (2013) - 2013 • 1983
On the efficiency of two-stage response-adaptive designs
Dette, H. and Bornkamp, B. and Bretz, F.
STATISTICS IN MEDICINE. Volume: 32 (2013) - 2013 • 1982
A Copula-Based Non-parametric Measure of Regression Dependence
Dette, H. and Siburg, K.F. and Stoimenov, P.A.
SCANDINAVIAN JOURNAL OF STATISTICS. Volume: 40 (2013) - 2013 • 1981
Environmental tight-binding modeling of nickel and cobalt clusters
McEniry, E.J. and Drautz, R. and Madsen, G.K.H.
JOURNAL OF PHYSICS CONDENSED MATTER. Volume: 25 (2013) - 2013 • 1980
Nonparametric comparison of quantile curves: a stochastic process approach
Dette, H. and Wagener, J. and Volgushev, S.
JOURNAL OF NONPARAMETRIC STATISTICS. Volume: 25 (2013) - 2013 • 1979
Fluid pumping cell of photonic - Plasmonic microcavity sensor for biomedical application
Saetchnikov, V.A. and Tcherniavskaia, E.A. and Saetchnikov, A.V. and Schweiger, G. and Ostendorf, A.
PROCEEDINGS OF THE INTERNATIONAL CONFERENCE ON ADVANCED OPTOELECTRONICS AND LASERS, CAOL. Volume: (2013) - 2013 • 1978
Diffusion in fracturing porous materials: Characterizing topological effects using cascade micromechanics and phase-field models
Timothy, J.J. and Meschke, G.
POROMECHANICS V - PROCEEDINGS OF THE 5TH BIOT CONFERENCE ON POROMECHANICS. Volume: (2013) - 2013 • 1977
Optimal design for linear models with correlated observations
Dette, H. and Pepelyshev, A. and Zhigljavsky, A.
ANNALS OF STATISTICS. Volume: 41 (2013) - 2013 • 1976
Simulating mobile dendrites in a flow
Medvedev, D. and Varnik, F. and Steinbach, I.
PROCEDIA COMPUTER SCIENCE. Volume: 18 (2013) - 2013 • 1975
Diagnostics of biomedical agents by whispering gallery mode optical resonance based sensor
Saetchnikov, V.A. and Tcherniavskaia, E.A. and Saetchnikov, A.V. and Schweiger, G. and Ostendorf, A.
PROCEEDINGS OF THE INTERNATIONAL CONFERENCE ON ADVANCED OPTOELECTRONICS AND LASERS, CAOL. Volume: (2013) - 2013 • 1974
Comment
Dette, H.
JOURNAL OF THE AMERICAN STATISTICAL ASSOCIATION. Volume: 108 (2013) - 2013 • 1973
Understanding the microscopic origin of gold nanoparticle anisotropic growth from molecular dynamics simulations
Meena, S.K. and Sulpizi, M.
LANGMUIR. Volume: 29 (2013) - 2013 • 1972
Drag detection and identification by whispering gallery mode optical resonance based sensor
Saetchnikov, V.A. and Tcherniavskaia, E.A. and Saetchnikov, A.V. and Schweiger, G. and Ostendorf, A.
PROGRESS IN BIOMEDICAL OPTICS AND IMAGING - PROCEEDINGS OF SPIE. Volume: 8801 (2013) - 2013 • 1971
Self-consistent scale-bridging approach to compute the elasticity of multi-phase polycrystalline materials
Titrian, H. and Aydin, U. and Friák, M. and Ma, D. and Raabe, D. and Neugebauer, J.
MATERIALS RESEARCH SOCIETY SYMPOSIUM PROCEEDINGS. Volume: 1524 (2013) - 2013 • 1970
Least squares estimation in high dimensional sparse heteroscedastic models
Dette, H. and Wagener, J.
ROBUSTNESS AND COMPLEX DATA STRUCTURES: FESTSCHRIFT IN HONOUR OF URSULA GATHER. Volume: (2013) - 2013 • 1969
Variation of the intrinsic stress gradient in thin aluminum nitride films
Mehner, H. and Leopold, S. and Hoffmann, M.
JOURNAL OF MICROMECHANICS AND MICROENGINEERING. Volume: 23 (2013) - 2013 • 1968
A novel [FeFe] hydrogenase model with a (SCH2)2Pi=O moiety
Almazahreh, L.R. and Apfel, U.-P. and Imhof, W. and Rudolph, M. and Görls, H. and Talarmin, J. and Schollhammer, P. and El-Khateeb, M. and Weigand, W.
ORGANOMETALLICS. Volume: 32 (2013) - 2013 • 1967
Goodness-of-fit tests in long-range dependent processes under fixed alternatives
Dette, H. and Sen, K.
ESAIM - PROBABILITY AND STATISTICS. Volume: 17 (2013) - 2013 • 1966
Electrochemical detection of chloride levels in sweat using silver nanoparticles: A basis for the preliminary screening for cystic fibrosis
Toh, H.S. and Batchelor-Mcauley, C. and Tschulik, K. and Compton, R.G.
ANALYST. Volume: 138 (2013) - 2013 • 1965
GASPI - A partitioned global address space programming interface
Alrutz, T. and Backhaus, J. and Brandes, T. and End, V. and Gerhold, T. and Geiger, A. and Grünewald, D. and Heuveline, V. and Jägersküpper, J. and Knüpfer, A. and Krzikalla, O. and Kügeler, E. and Lojewski, C. and Lonsdale, G. and Müller-Pfefferkorn, R. and Nagel, W. and Oden, L. and Pfreundt, F.-J. and Rahn, M. and Sattler, M. and Schmidtobreick, M. and Schiller, A. and Simmendinger, C. and Soddemann, T. and Sutmann, G. and Weber, H. and Weiss, J.-P.
LECTURE NOTES IN COMPUTER SCIENCE (INCLUDING SUBSERIES LECTURE NOTES IN ARTIFICIAL INTELLIGENCE AND LECTURE NOTES IN BIOINFORMATICS). Volume: 7686 LNCS (2013) - 2013 • 1964
'Old chemistries' for new applications: Perspectives for development of precursors for MOCVD and ALD applications
Devi, A.
COORDINATION CHEMISTRY REVIEWS. Volume: 257 (2013) - 2013 • 1963
Measuring composition in InGaN from HAADF-STEM images and studying the temperature dependence of Z-contrast
Mehrtens, T. and Schowalter, M. and Tytko, D. and Choi, P. and Raabe, D. and Hoffmann, L. and Jönen, H. and Rossow, U. and Hangleiter, A. and Rosenauer, A.
JOURNAL OF PHYSICS: CONFERENCE SERIES. Volume: 471 (2013) - 2013 • 1962
The anodic stripping voltammetry of nanoparticles: Electrochemical evidence for the surface agglomeration of silver nanoparticles
Toh, H.S. and Batchelor-McAuley, C. and Tschulik, K. and Uhlemann, M. and Crossley, A. and Compton, R.G.
NANOSCALE. Volume: 5 (2013) - 2013 • 1961
An orders-of-magnitude increase in the rate of the solid-catalyzed co oxidation by in situ ball milling
Immohr, S. and Felderhoff, M. and Weidenthaler, C. and Schüth, F.
ANGEWANDTE CHEMIE - INTERNATIONAL EDITION. Volume: 52 (2013) - 2013 • 1960
Measurement of the indium concentration in high indium content InGaN layers by scanning transmission electron microscopy and atom probe tomography
Mehrtens, T. and Schowalter, M. and Tytko, D. and Choi, P. and Raabe, D. and Hoffmann, L. and Jönen, H. and Rossow, U. and Hangleiter, A. and Rosenauer, A.
APPLIED PHYSICS LETTERS. Volume: 102 (2013) - 2013 • 1959
Robotic heavy metal anodic stripping voltammetry: Ease and efficacy for trace lead and cadmium electroanalysis
Intarakamhang, S. and Schuhmann, W. and Schulte, A.
JOURNAL OF SOLID STATE ELECTROCHEMISTRY. Volume: 17 (2013) - 2013 • 1958
Evidence for metal-support interactions in Au modified TiO x/SBA-15 materials prepared by photodeposition
Mei, B. and Wiktor, C. and Turner, S. and Pougin, A. and Van Tendeloo, G. and Fischer, R.A. and Muhler, M. and Strunk, J.
ACS CATALYSIS. Volume: 3 (2013) - 2013 • 1957
Odd and even Kondo effects from emergent localization in quantum point contacts
Iqbal, M.J. and Levy, R. and Koop, E.J. and Dekker, J.B. and De Jong, J.P. and Van Der Velde, J.H.M. and Reuter, D. and Wieck, A.D. and Aguado, R. and Meir, Y. and Van Der Wal, C.H.
NATURE. Volume: 501 (2013) - 2013 • 1956
Beneficial effect of Nb doping on the photoelectrochemical properties of TiO2 and TiO2-polyheptazine hybrids
Mei, B. and Byford, H. and Bledowski, M. and Wang, L. and Strunk, J. and Muhler, M. and Beranek, R.
SOLAR ENERGY MATERIALS AND SOLAR CELLS. Volume: 117 (2013) - 2013 • 1955
Split-gate quantum point contacts with tunable channel length
Iqbal, M.J. and De. Jong, J.P. and Reuter, D. and Wieck, A.D. and Van Der Wal, C.H.
JOURNAL OF APPLIED PHYSICS. Volume: 113 (2013) - 2013 • 1954
Influence of photodeposited gold nanoparticles on the photocatalytic activity of titanate species in the reduction of CO2 to hydrocarbons
Mei, B. and Pougin, A. and Strunk, J.
JOURNAL OF CATALYSIS. Volume: 306 (2013) - 2013 • 1953
Application of SECM in tracing of hydrogen peroxide at multicomponent non-noble electrocatalyst films for the oxygen reduction reaction
Dobrzeniecka, A. and Zeradjanin, A. and Masa, J. and Puschhof, A. and Stroka, J. and Kulesza, P.J. and Schuhmann, W.
CATALYSIS TODAY. Volume: 202 (2013) - 2013 • 1952
Surface reaction of 2-propanol on modified Keggin type polyoxometalates: In situ IR spectroscopic investigation of the surface acid-base properties
Iwanowa, X. and Strunk, J. and Löffler, E. and Muhler, M. and Merz, K.
PHYSICA STATUS SOLIDI (B) BASIC RESEARCH. Volume: 250 (2013) - 2013 • 1951
Direct electron transfer of Trametes hirsuta laccase adsorbed at unmodified nanoporous gold electrodes
Salaj-Kosla, U. and Pöller, S. and Schuhmann, W. and Shleev, S. and Magner, E.
BIOELECTROCHEMISTRY. Volume: 91 (2013) - 2013 • 1950
SIMS analysis on austenitic stainless steel: The influence of type of oxide surface layer on hydrogen embrittlement
Izawa, C. and Wagner, S. and Martin, M. and Weber, S. and Pargeter, R. and Michler, T. and Uchida, H.H. and Pundt, A.
JOURNAL OF ALLOYS AND COMPOUNDS. Volume: 580 (2013) - 2013 • 1949
Ion energy distribution functions behind the sheaths of magnetized and non-magnetized radio frequency discharges
Trieschmann, J. and Shihab, M. and Szeremley, D. and Elgendy, A.E. and Gallian, S. and Eremin, D. and Brinkmann, R.P. and Mussenbrock, T.
JOURNAL OF PHYSICS D: APPLIED PHYSICS. Volume: 46 (2013) - 2013 • 1948
Biomimetic assembly of the [FeFe] hydrogenase: Synthetic mimics in a biological shell
Apfel, U.-P. and Weigand, W.
CHEMBIOCHEM. Volume: 14 (2013) - 2013 • 1947
Charge conversion of nearly free and impurity bound magneto-trions immersed in 2D electron or hole gas with optically tunable concentration
Jadczak, J. and Bryja, L. and Misiewicz, J. and Wójs, A. and Potemski, M. and Liu, F. and Yakovlev, D.R. and Bayer, M. and Reuter, D. and Wieck, A.D. and Nicoll, C.A. and Farrer, I. and Ritchie, D.A.
JOURNAL OF PHYSICS: CONFERENCE SERIES. Volume: 456 (2013) - 2013 • 1946
Detection of nitric oxide and nitroxyl with benzoresorufin-based fluorescent sensors
Apfel, U.-P. and Buccella, D. and Wilson, J.J. and Lippard, S.J.
INORGANIC CHEMISTRY. Volume: 52 (2013) - 2013 • 1945
Coexistence of nearly free and strongly bound trions from magneto-photoluminescence of two-dimensional quantum structures with tunable electron or hole concentration
Jadczak, J. and Bryja, L. and Misiewicz, J. and Wójs, A. and Potemski, M. and Liu, F. and Yakovlev, D.R. and Bayer, M. and Reuter, D. and Wieck, A. and Nicoll, C.A. and Farrer, I. and Ritchie, D.A.
AIP CONFERENCE PROCEEDINGS. Volume: 1566 (2013) - 2013 • 1944
Ab initio prediction of the critical thickness of a precipitate
Sampath, S. and Janisch, R.
JOURNAL OF PHYSICS CONDENSED MATTER. Volume: 25 (2013) - 2013 • 1943
Coulometric sizing of nanoparticles: Cathodic and anodic impact experiments open two independent routes to electrochemical sizing of Fe3O4 nanoparticles
Tschulik, K. and Haddou, B. and Omanović, D. and Rees, N.V. and Compton, R.G.
NANO RESEARCH. Volume: 6 (2013) - 2013 • 1942
Comparison of different metrics for analysis and visualization in spectroscopic optical coherence tomography
Jaedicke, V. and Agcaer, S. and Robles, F.E. and Steinert, M. and Jones, D. and Goebel, S. and Gerhardt, N.C. and Welp, H. and Hofmann, M.R.
BIOMEDICAL OPTICS EXPRESS. Volume: 4 (2013) - 2013 • 1941
Correlating catalytic methanol oxidation with the structure and oxidation state of size-selected pt nanoparticles
Merte, L.R. and Ahmadi, M. and Behafarid, F. and Ono, L.K. and Lira, E. and Matos, J. and Li, L. and Yang, J.C. and Cuenya, B.R.
ACS CATALYSIS. Volume: 3 (2013) - 2013 • 1940
Formation of carbon nanofilms on single crystal quartz
Samsonau, S.V. and Dzedzits, E. and Shvarkov, S.D. and Meinerzhagen, F. and Wieck, A.D. and Zaitsev, A.M.
SENSORS AND ACTUATORS, B: CHEMICAL. Volume: 186 (2013) - 2013 • 1939
'Sticky electrodes' for the detection of silver nanoparticles
Tschulik, K. and Palgrave, R.G. and Batchelor-Mcauley, C. and Compton, R.G.
NANOTECHNOLOGY. Volume: 24 (2013) - 2013 • 1938
Contrast enhancement methods in Optical Coherence Tomography using spectral features
Jaedicke, V. and Aǧcaer, S. and Goebel, S. and Gerhardt, N.C. and Welp, H. and Hofmann, M.R.
BIOMEDIZINISCHE TECHNIK. Volume: 58 (2013) - 2013 • 1937
Parallelized computational modeling of pile-soil interactions in mechanized tunneling
Meschke, G. and Ninić, J. and Stascheit, J. and Alsahly, A.
ENGINEERING STRUCTURES. Volume: 47 (2013) - 2013 • 1936
Growth of graphene-like films for NO2 detection
Samsonau, S.V. and Shvarkov, S.D. and Meinerzhagen, F. and Wieck, A.D. and Zaitsev, A.M.
SENSORS AND ACTUATORS, B: CHEMICAL. Volume: 182 (2013) - 2013 • 1935
Comparison of scalable fast methods for long-range interactions
Arnold, A. and Fahrenberger, F. and Holm, C. and Lenz, O. and Bolten, M. and Dachsel, H. and Halver, R. and Kabadshow, I. and Gähler, F. and Heber, F. and Iseringhausen, J. and Hofmann, M. and Pippig, M. and Potts, D. and Sutmann, G.
PHYSICAL REVIEW E - STATISTICAL, NONLINEAR, AND SOFT MATTER PHYSICS. Volume: 88 (2013) - 2013 • 1934
Spectroscopic optical coherence tomography with graphics processing unit based analysis of three dimensional data sets
Jaedicke, V. and Aǧcaer, S. and Goebel, S. and Gerhardt, N.C. and Welp, H. and Hofmann, M.R.
PROGRESS IN BIOMEDICAL OPTICS AND IMAGING - PROCEEDINGS OF SPIE. Volume: 8592 (2013) - 2013 • 1933
Surface anchored metal-organic frameworks as stimulus responsive antifouling coatings
Sancet, M.P.A. and Hanke, M. and Wang, Z. and Bauer, S. and Azucena, C. and Arslan, H.K. and Heinle, M. and Gliemann, H. and Wöll, C. and Rosenhahn, A.
BIOINTERPHASES. Volume: 8 (2013) - 2013 • 1932
Neural network potentials for metals and oxides - First applications to copper clusters at zinc oxide
Artrith, N. and Hiller, B. and Behler, J.
PHYSICA STATUS SOLIDI (B) BASIC RESEARCH. Volume: 250 (2013) - 2013 • 1931
Grain boundary segregation in a bronze-route Nb3Sn superconducting wire studied by atom probe tomography
Sandim, M.J.R. and Tytko, D. and Kostka, A. and Choi, P. and Awaji, S. and Watanabe, K. and Raabe, D.
SUPERCONDUCTOR SCIENCE AND TECHNOLOGY. Volume: 26 (2013) - 2013 • 1930
Basal and non-basal dislocation slip in Mg–Y
Sandlöbes, S. and Friák, M. and Neugebauer, J. and Raabe, D.
MATERIALS SCIENCE AND ENGINEERING A. Volume: 576 (2013) - 2013 • 1929
Simulation benchmarks for low-pressure plasmas: Capacitive discharges
Turner, M.M. and Derzsi, A. and Donkó, Z. and Eremin, D. and Kelly, S.J. and Lafleur, T. and Mussenbrock, T.
PHYSICS OF PLASMAS. Volume: 20 (2013) - 2013 • 1928
S-N fatigue properties of a stable high-aluminum austenitic stainless steel for hydrogen applications
Michler, T. and Naumann, J. and Weber, S. and Martin, M. and Pargeter, R.
INTERNATIONAL JOURNAL OF HYDROGEN ENERGY. Volume: 38 (2013) - 2013 • 1927
Topological fingerprints for intermetallic compounds for the automated classification of atomistic simulation data
Schablitzki, T. and Rogal, J. and Drautz, R.
MODELLING AND SIMULATION IN MATERIALS SCIENCE AND ENGINEERING. Volume: 21 (2013) - 2013 • 1926
A versatile electrochemical cell for the preparation and characterisation of model electrocatalytic systems
Tymoczko, J. and Schuhmann, W. and Bandarenka, A.S.
PHYSICAL CHEMISTRY CHEMICAL PHYSICS. Volume: 15 (2013) - 2013 • 1925
Element-resolved corrosion analysis of stainless-type glass-forming steels
Duarte, M.J. and Klemm, J. and Klemm, S.O. and Mayrhofer, K.J.J. and Stratmann, M. and Borodin, S. and Romero, A.H. and Madinehei, M. and Crespo, D. and Serrano, J. and Gerstl, S.S.A. and Choi, P.P. and Raabe, D. and Renner, F.U.
SCIENCE. Volume: 341 (2013) - 2013 • 1924
Nonexponential photoluminescence transients in a Ga(NAsP) lattice matched to a (001) silicon substrate
Jandieri, K. and Kunert, B. and Liebich, S. and Zimprich, M. and Volz, K. and Stolz, W. and Gebhard, F. and Baranovskii, S.D. and Koukourakis, N. and Gerhardt, N.C. and Hofmann, M.R.
PHYSICAL REVIEW B - CONDENSED MATTER AND MATERIALS PHYSICS. Volume: 87 (2013) - 2013 • 1923
The constant phase element reveals 2D phase transitions in adsorbate layers at the electrode/electrolyte interfaces
Tymoczko, J. and Schuhmann, W. and Bandarenka, A.S.
ELECTROCHEMISTRY COMMUNICATIONS. Volume: 27 (2013) - 2013 • 1922
High-pressure water intrusion investigation of pure silica ITQ-7 zeolite
Tzanis, L. and Marler, B. and Gies, H. and Patarin, J.
JOURNAL OF PHYSICAL CHEMISTRY C. Volume: 117 (2013) - 2013 • 1921
Stripe antiferromagnetic spin fluctuations in SrCo2As 2
Jayasekara, W. and Lee, Y. and Pandey, A. and Tucker, G.S. and Sapkota, A. and Lamsal, J. and Calder, S. and Abernathy, D.L. and Niedziela, J.L. and Harmon, B.N. and Kreyssig, A. and Vaknin, D. and Johnston, D.C. and Goldman, A.I. and McQueeney, R.J.
PHYSICAL REVIEW LETTERS. Volume: 111 (2013) - 2013 • 1920
Strong enhancement of Eu+3 luminescence in europium-implanted GaN by Si and Mg codoping
Mishra, J.K. and Langer, T. and Rossow, U. and Shvarkov, S. and Wieck, A. and Hangleiter, A.
APPLIED PHYSICS LETTERS. Volume: 102 (2013) - 2013 • 1919
Failure mechanisms of magnesia alumina spinel abradable coatings under thermal cyclic loading
Ebert, S. and Mücke, R. and Mack, D. and Vaßen, R. and Stöver, D. and Wobst, T. and Gebhard, S.
JOURNAL OF THE EUROPEAN CERAMIC SOCIETY. Volume: 33 (2013) - 2013 • 1918
Time-resolved measurement of film growth during reactive high power pulsed magnetron sputtering (HIPIMS) of titanium nitride
Mitschker, F. and Prenzel, M. and Benedikt, J. and Maszl, C. and von Keudell, A.
JOURNAL OF PHYSICS D-APPLIED PHYSICS. Volume: 46 (2013) - 2013 • 1917
Scanning electrochemical microscopy at variable temperatures
Schäfer, D. and Puschhof, A. and Schuhmann, W.
PHYSICAL CHEMISTRY CHEMICAL PHYSICS. Volume: 15 (2013) - 2013 • 1916
Pulsed high-power plasmas for deposition of nanostructured thin films
Awakowicz, P. and Czarnetzki, U.
JOURNAL OF PHYSICS D: APPLIED PHYSICS. Volume: 46 (2013) - 2013 • 1915
Simple synthesis of superparamagnetic magnetite nanoparticles as highly efficient contrast agent
Jha, D.K. and Shameem, M. and Patel, A.B. and Kostka, A. and Schneider, P. and Erbe, A. and Deb, P.
MATERIALS LETTERS. Volume: 95 (2013) - 2013 • 1914
Time-resolved measurement of film growth during high-power pulsed magnetron sputtering (HIPIMS) of titanium
Mitschker, F. and Prenzel, M. and Benedikt, J. and Maszl, C. and von Keudell, A.
JOURNAL OF PHYSICS D-APPLIED PHYSICS. Volume: 46 (2013) - 2013 • 1913
Structured electrodeposition in magnetic gradient fields
Uhlemann, M. and Tschulik, K. and Gebert, A. and Mutschke, G. and Fröhlich, J. and Bund, A. and Yang, X. and Eckert, K.
EUROPEAN PHYSICAL JOURNAL: SPECIAL TOPICS. Volume: 220 (2013) - 2013 • 1912
Lattice Boltzmann modeling of advection-diffusion-reaction equations: Pattern formation under uniform differential advection
Ayodele, S.G. and Raabe, D. and Varnik, F.
COMMUNICATIONS IN COMPUTATIONAL PHYSICS. Volume: 13 (2013) - 2013 • 1911
Simulation of shear banding in heterophase co-deformation: Example of plane strain compressed Cu-Ag and Cu-Nb metal matrix composites
Jia, N. and Roters, F. and Eisenlohr, P. and Raabe, D. and Zhao, X.
ACTA MATERIALIA. Volume: 61 (2013) - 2013 • 1910
On the cyclic material stability of shape memory polymer
Mogharebi, S. and Kazakeviciute-Makovska, R. and Steeb, H. and Eggeler, G. and Neuking, K.
MATERIALWISSENSCHAFT UND WERKSTOFFTECHNIK. Volume: 44 (2013) - 2013 • 1909
Universal method for protein immobilization on chemically functionalized germanium investigated by ATR-FTIR difference spectroscopy
Schartner, J. and Güldenhaupt, J. and Mei, B. and Rögner, M. and Muhler, M. and Gerwert, K. and Kötting, C.
JOURNAL OF THE AMERICAN CHEMICAL SOCIETY. Volume: 135 (2013) - 2013 • 1908
Get more out of your data: A new approach to agglomeration and aggregation studies using nanoparticle impact experiments
Ellison, J. and Tschulik, K. and Stuart, E.J.E. and Jurkschat, K. and Omanović, D. and Uhlemann, M. and Crossley, A. and Compton, R.G.
CHEMISTRYOPEN. Volume: 2 (2013) - 2013 • 1907
Analysis of the interaction of the molybdenum hydroxylase PaoABC from Escherichia coli with positively and negatively charged metal complexes
Badalyan, A. and Yoga, E.G. and Schwuchow, V. and Pöller, S. and Schuhmann, W. and Leimkühler, S. and Wollenberger, U.
ELECTROCHEMISTRY COMMUNICATIONS. Volume: 37 (2013) - 2013 • 1906
A monostatic antenna-reflector system for ultra-short-range radar applications
Baer, C. and Musch, T. and Schulz, C. and Will, B. and Rolfes, I.
IEEE ANTENNAS AND PROPAGATION SOCIETY, AP-S INTERNATIONAL SYMPOSIUM (DIGEST). Volume: (2013) - 2013 • 1905
Characterization of crocodile teeth: Correlation of composition, microstructure, and hardness
Enax, J. and Fabritius, H.-O. and Rack, A. and Prymak, O. and Raabe, D. and Epple, M.
JOURNAL OF STRUCTURAL BIOLOGY. Volume: 184 (2013) - 2013 • 1904
Biexcitons in semiconductor quantum dot ensembles
Moody, G. and Singh, R. and Li, H. and Akimov, I.A. and Bayer, M. and Reuter, D. and Wieck, A.D. and Bracker, A.S. and Gammon, D. and Cundiff, S.T.
PHYSICA STATUS SOLIDI (B) BASIC RESEARCH. Volume: 250 (2013) - 2013 • 1903
Correlation and dephasing effects on the non-radiative coherence between bright excitons in an InAs QD ensemble measured with 2D spectroscopy
Moody, G. and Singh, R. and Li, H. and Akimov, I.A. and Bayer, M. and Reuter, D. and Wieck, A.D. and Cundiff, S.T.
SOLID STATE COMMUNICATIONS. Volume: 163 (2013) - 2013 • 1902
A millimeter-wave based measuring method for the differentiation of atherosclerotic plaques
Baer, C. and Notzon, G. and Dahl, C. and Schulz, C. and Will, B. and Rolfes, I. and Musch, T.
2013 IEEE MTT-S INTERNATIONAL MICROWAVE WORKSHOP SERIES ON RF AND WIRELESS TECHNOLOGIES FOR BIOMEDICAL AND HEALTHCARE APPLICATIONS, IMWS-BIO 2013 - PROCEEDINGS. Volume: (2013) - 2013 • 1901
Combined experimental and numerical approach for linking microstructure and mechanical properties on different length scales for near γ-TiAl alloys
Kabir, M.R. and Bartsch, M. and Chernova, L. and Schneider, J. and Kelm, K.
MATERIALS SCIENCE FORUM. Volume: 750 (2013) - 2013 • 1900
Fifth-order nonlinear optical response of excitonic states in an InAs quantum dot ensemble measured with two-dimensional spectroscopy
Moody, G. and Singh, R. and Li, H. and Akimov, I.A. and Bayer, M. and Reuter, D. and Wieck, A.D. and Cundiff, S.T.
PHYSICAL REVIEW B - CONDENSED MATTER AND MATERIALS PHYSICS. Volume: 87 (2013) - 2013 • 1899
JTST special issue on "coatings for energy applications"
Vardelle, A. and Vassen, R.
JOURNAL OF THERMAL SPRAY TECHNOLOGY. Volume: 22 (2013) - 2013 • 1898
Methanol oxidation as probe reaction for active sites in Au/ZnO and Au/TiO2 catalysts
Kähler, K. and Holz, M.C. and Rohe, M. and Van Veen, A.C. and Muhler, M.
JOURNAL OF CATALYSIS. Volume: 299 (2013) - 2013 • 1897
Influence of confinement on biexciton binding in semiconductor quantum dot ensembles measured with two-dimensional spectroscopy
Moody, G. and Singh, R. and Li, H. and Akimov, I.A. and Bayer, M. and Reuter, D. and Wieck, A.D. and Bracker, A.S. and Gammon, D. and Cundiff, S.T.
PHYSICAL REVIEW B - CONDENSED MATTER AND MATERIALS PHYSICS. Volume: 87 (2013) - 2013 • 1896
Simulation of viscous sintering using the lattice Boltzmann method
Varnik, F. and Rios, A. and Gross, M. and Steinbach, I.
MODELLING AND SIMULATION IN MATERIALS SCIENCE AND ENGINEERING. Volume: 21 (2013) - 2013 • 1895
Compositionally zoned crystals and real-time degassing data reveal changes in magma transfer dynamics during the 2006 summit eruptive episodes of Mt. Etna
Kahl, M. and Chakraborty, S. and Costa, F. and Pompilio, M. and Liuzzo, M. and Viccaro, M.
BULLETIN OF VOLCANOLOGY. Volume: 75 (2013) - 2013 • 1894
Spray roasting of iron chloride FeCl2: Numerical modelling of industrial scale reactors
Schiemann, M. and Wirtz, S. and Scherer, V. and Bärhold, F.
POWDER TECHNOLOGY. Volume: 245 (2013) - 2013 • 1893
Temperature dependence of hole spin coherence in (In,Ga)As quantum dots measured by mode-locking and echo techniques
Varwig, S. and René, A. and Greilich, A. and Yakovlev, D.R. and Reuter, D. and Wieck, A.D. and Bayer, M.
PHYSICAL REVIEW B - CONDENSED MATTER AND MATERIALS PHYSICS. Volume: 87 (2013) - 2013 • 1892
Surface pre-treatment for barrier coatings on polyethylene terephthalate
Bahre, H. and Bahroun, K. and Behm, H. and Steves, S. and Awakowicz, P. and Böke, M. and Hopmann, Ch. and Winter, J.
JOURNAL OF PHYSICS D: APPLIED PHYSICS. Volume: 46 (2013) - 2013 • 1891
A density-functional theory-based neural network potential for water clusters including van der waals corrections
Morawietz, T. and Behler, J.
JOURNAL OF PHYSICAL CHEMISTRY A. Volume: 117 (2013) - 2013 • 1890
Thermal Barrier Coatings
Vaßen, R.
CERAMICS SCIENCE AND TECHNOLOGY. Volume: 4-4 (2013) - 2013 • 1889
A full-dimensional neural network potential-energy surface for water clusters up to the hexamer
Morawietz, T. and Behler, J.
ZEITSCHRIFT FUR PHYSIKALISCHE CHEMIE. Volume: 227 (2013) - 2013 • 1888
Simulations of electromagnetic effects in high-frequency capacitively coupled discharges using the Darwin approximation
Eremin, D. and Hemke, T. and Brinkmann, R.P. and Mussenbrock, T.
JOURNAL OF PHYSICS D: APPLIED PHYSICS. Volume: 46 (2013) - 2013 • 1887
Many-body correlations of electrostatically trapped dipolar excitons
Schinner, G.J. and Repp, J. and Schubert, E. and Rai, A.K. and Reuter, D. and Wieck, A.D. and Govorov, A.O. and Holleitner, A.W. and Kotthaus, J.P.
PHYSICAL REVIEW B - CONDENSED MATTER AND MATERIALS PHYSICS. Volume: 87 (2013) - 2013 • 1886
Ammonia-annealed TiO2 as a negative electrode material in Li-Ion batteries: N doping or oxygen deficiency?
Ventosa, E. and Xia, W. and Klink, S. and Mantia, F.L. and Mei, B. and Muhler, M. and Schuhmann, W.
CHEMISTRY - A EUROPEAN JOURNAL. Volume: 19 (2013) - 2013 • 1885
High peak power pulses from dispersion optimised modelocked semiconductor laser
Balzer, J.C. and Schlauch, T. and Klehr, A. and Erbert, G. and Tränkle, G. and Hofmann, M.R.
ELECTRONICS LETTERS. Volume: 49 (2013) - 2013 • 1884
Controlled manipulation of single atoms and small molecules using the scanning tunnelling microscope
Morgenstern, K. and Lorente, N. and Rieder, K.-H.
PHYSICA STATUS SOLIDI (B) BASIC RESEARCH. Volume: 250 (2013) - 2013 • 1883
Confinement and interaction of single indirect excitons in a voltage-controlled trap formed inside double InGaAs quantum wells
Schinner, G.J. and Repp, J. and Schubert, E. and Rai, A.K. and Reuter, D. and Wieck, A.D. and Govorov, A.O. and Holleitner, A.W. and Kotthaus, J.P.
PHYSICAL REVIEW LETTERS. Volume: 110 (2013) - 2013 • 1882
TiO2(B)/anatase composites synthesized by spray drying as high performance negative electrode material in Li-ion batteries
Ventosa, E. and Mei, B. and Xia, W. and Muhler, M. and Schuhmann, W.
CHEMSUSCHEM. Volume: 6 (2013) - 2013 • 1881
Localized electrochemical impedance spectroscopy: Visualization of spatial distributions of the key parameters describing solid/liquid interfaces
Bandarenka, A.S. and Eckhard, K. and Maljusch, A. and Schuhmann, W.
ANALYTICAL CHEMISTRY. Volume: 85 (2013) - 2013 • 1880
Quantum Hall signatures of dipolar Mahan excitons
Schinner, G.J. and Repp, J. and Kowalik-Seidl, K. and Schubert, E. and Stallhofer, M.P. and Rai, A.K. and Reuter, D. and Wieck, A.D. and Govorov, A.O. and Holleitner, A.W. and Kotthaus, J.P.
PHYSICAL REVIEW B - CONDENSED MATTER AND MATERIALS PHYSICS. Volume: 87 (2013) - 2013 • 1879
Optical investigation of carbon nanotube agglomerate growth on single catalyst particles
Voelskow, K. and Nickelsen, L. and Becker, M.J. and Xia, W. and Muhler, M. and Kunz, U. and Weber, A.P. and Turek, T.
CHEMICAL ENGINEERING JOURNAL. Volume: 234 (2013) - 2013 • 1878
Monitoring bound HA1(H1N1) and HA1(H5N1) on freely suspended graphene over plasmonic platforms with infrared spectroscopy
Banerjee, A. and Chakraborty, S. and Altan-Bonnet, N. and Grebel, H.
CHEMICAL PHYSICS LETTERS. Volume: 582 (2013) - 2013 • 1877
Size control of gold nanoparticles during laser ablation in liquids with different functional molecules
Essaidi, A. and Chakif, M. and Schöps, B. and Aumman, A. and Xiao, S. and Esen, C. and Ostendorf, A.
JOURNAL OF LASER MICRO NANOENGINEERING. Volume: 8 (2013) - 2013 • 1876
Computational model for the cell-mechanical response of the osteocyte cytoskeleton based on self-stabilizing tensegrity structures
Kardas, D. and Nackenhorst, U. and Balzani, D.
BIOMECHANICS AND MODELING IN MECHANOBIOLOGY. Volume: 12 (2013) - 2013 • 1875
Nonparametric quantile regression for twice censored data
Volgushev, S. and Dette, H.
BERNOULLI. Volume: 19 (2013) - 2013 • 1874
Shift of the blocking temperature of Co nanoparticles by Cr capping
Ewerlin, M. and Petracic, O. and Demirbas, D. and Agudo, L. and Eggeler, G. and Brüssing, F. and Abrudan, R. and Zabel, H.
JOURNAL OF APPLIED PHYSICS. Volume: 114 (2013) - 2013 • 1873
Fe-Mg interdiffusion rates in clinopyroxene: Experimental data and implications for Fe-Mg exchange geothermometers
Müller, T. and Dohmen, R. and Becker, H.W. and ter Heege, J.H. and Chakraborty, S.
CONTRIBUTIONS TO MINERALOGY AND PETROLOGY. Volume: 166 (2013) - 2013 • 1872
Significance testing in quantile regression
Volgushev, S. and Birke, M. and Dette, H. and Neumeyer, N.
ELECTRONIC JOURNAL OF STATISTICS. Volume: 7 (2013) - 2013 • 1871
Mg in plagioclase: Experimental calibration of a new geothermometer and diffusion coefficients
Faak, K. and Chakraborty, S. and Coogan, L.A.
GEOCHIMICA ET COSMOCHIMICA ACTA. Volume: 123 (2013) - 2013 • 1870
Interplay between Coulomb interaction and quantum-confined Stark-effect in polar and nonpolar wurtzite InN/GaN quantum dots
Barthel, S. and Schuh, K. and Marquardt, O. and Hickel, T. and Neugebauer, J. and Jahnke, F. and Czycholl, G.
EUROPEAN PHYSICAL JOURNAL B. Volume: 86 (2013) - 2013 • 1869
Modelling the lattice dynamics in SixGe1-x alloys
Katre, A. and Drautz, R. and Madsen, G.K.H.
JOURNAL OF PHYSICS CONDENSED MATTER. Volume: 25 (2013) - 2013 • 1868
Diffraction and Spectroscopy of Porous Solids
Schmidt, W. and Schüth, F. and Weidenthaler, C.
COMPREHENSIVE INORGANIC CHEMISTRY II (SECOND EDITION): FROM ELEMENTS TO APPLICATIONS. Volume: 5 (2013) - 2013 • 1867
Nanotoxicity - An electrochemist's perspective
Batchelor-McAuley, C. and Tschulik, K. and Compton, R.G.
PORTUGALIAE ELECTROCHIMICA ACTA. Volume: 31 (2013) - 2013 • 1866
Chain deformation in translocation phenomena
Farahpour, F. and Maleknejad, A. and Varnik, F. and Ejtehadi, M.R.
SOFT MATTER. Volume: 9 (2013) - 2013 • 1865
The adaptive lasso in high-dimensional sparse heteroscedastic models
Wagener, J. and Dette, H.
MATHEMATICAL METHODS OF STATISTICS. Volume: 22 (2013) - 2013 • 1864
Molecular understanding of reactivity and selectivity for methanol oxidation at the Au/TiO2 interface
Farnesicamellone, M. and Zhao, J. and Jin, L. and Wang, Y. and Muhler, M. and Marx, D.
ANGEWANDTE CHEMIE - INTERNATIONAL EDITION. Volume: 52 (2013) - 2013 • 1863
A critical assessment of experimental methods for determining the dynamic mechanical characteristics of shape memory polymers
Kazakevičiute-Makovska, R. and Mogharebi, S. and Steeb, H. and Eggeler, G. and Neuking, K.
ADVANCED ENGINEERING MATERIALS. Volume: 15 (2013) - 2013 • 1862
Adhesion of marine fouling organisms on hydrophilic and amphiphilic polysaccharides
Bauer, S. and Arpa-Sancet, M.P. and Finlay, J.A. and Callow, M.E. and Callow, J.A. and Rosenhahn, A.
LANGMUIR. Volume: 29 (2013) - 2013 • 1861
Electrical and spectroscopic characterization of a surgical argon plasma discharge
Keller, S. and Bibinov, N. and Neugebauer, A. and Awakowicz, P.
JOURNAL OF PHYSICS D: APPLIED PHYSICS. Volume: 46 (2013) - 2013 • 1860
Ferritic stainless steels for high-temperature applications: Stabilization of the microstructure by solid state precipitation of MX carbonitrides
Nabiran, N. and Weber, S. and Theisen, W.
HIGH TEMPERATURE MATERIALS AND PROCESSES. Volume: 32 (2013) - 2013 • 1859
Electrochemical deposition of Co(Cu)/Cu multilayered nanowires
Fedorov, F.S. and Mönch, I. and Mickel, C. and Tschulik, K. and Zhao, B. and Uhlemann, M. and Gebert, A. and Eckert, J.
JOURNAL OF THE ELECTROCHEMICAL SOCIETY. Volume: 160 (2013) - 2013 • 1858
Influence of vacuum heat treatment parameters on the surface composition of MCrAlY coatings
Keller, I. and Naumenko, D. and Quadakkers, W.J. and Vaßen, R. and Singheiser, L.
SURFACE AND COATINGS TECHNOLOGY. Volume: 215 (2013) - 2013 • 1857
Electrochemically deposited Pd-Pt and Pd-Au codeposits on graphite electrodes for electrocatalytic H2O2 reduction
Nagaiah, T.C. and Schäfer, D. and Schuhmann, W. and Dimcheva, N.
ANALYTICAL CHEMISTRY. Volume: 85 (2013) - 2013 • 1856
Capacitance performance of cobalt hydroxide-based capacitors with utilization of near-neutral electrolytes
Fedorov, F.S. and Linnemann, J. and Tschulik, K. and Giebeler, L. and Uhlemann, M. and Gebert, A.
ELECTROCHIMICA ACTA. Volume: 90 (2013) - 2013 • 1855
Transition from diffusive to displacive austenite reversion in low-alloy steel
Nakada, N. and Tsuchiyama, T. and Takaki, S. and Ponge, D. and Raabe, D.
ISIJ INTERNATIONAL. Volume: 53 (2013) - 2013 • 1854
Separating the initial growth rate from the rate of deactivation in the growth kinetics of multi-walled carbon nanotubes from ethene over a cobalt-based bulk catalyst in a fixed-bed reactor
Becker, M.J. and Xia, W. and Xie, K. and Dittmer, A. and Voelskow, K. and Turek, T. and Muhler, M.
CARBON. Volume: 58 (2013) - 2013 • 1853
Inheritance of dislocations and crystallographic texture during martensitic reversion into austenite
Nakada, N. and Fukagawa, R. and Tsuchiyama, T. and Takaki, S. and Ponge, D. and Raabe, D.
ISIJ INTERNATIONAL. Volume: 53 (2013) - 2013 • 1852
Highly porous nitrogen-doped polyimine-based carbons with adjustable microstructures for CO2 capture
Wang, J. and Senkovska, I. and Oschatz, M. and Lohe, M.R. and Borchardt, L. and Heerwig, A. and Liu, Q. and Kaskel, S.
JOURNAL OF MATERIALS CHEMISTRY A. Volume: 1 (2013) - 2013 • 1851
Investigation of the formation of nanoparticles during laser remote welding
Scholz, T. and Dickmann, K. and Ostendorf, A.
PHYSICS PROCEDIA. Volume: 41 (2013) - 2013 • 1850
Detection of programme making and special event devices in indoor channels
Nalobin, A. and Dortmund, S. and Rolfes, I.
IEEE ANTENNAS AND PROPAGATION SOCIETY, AP-S INTERNATIONAL SYMPOSIUM (DIGEST). Volume: (2013) - 2013 • 1849
Chemical activity of thin oxide layers: Strong interactions with the support yield a new thin-film phase of zno
Schott, V. and Oberhofer, H. and Birkner, A. and Xu, M. and Wang, Y. and Muhler, M. and Reuter, K. and Wöll, C.
ANGEWANDTE CHEMIE - INTERNATIONAL EDITION. Volume: 52 (2013) - 2013 • 1848
A kinetic study of oxygen reduction reaction and characterization on electrodeposited gold nanoparticles of diameter between 17 nm and 40 nm in 0.5 M sulfuric acid
Wang, Y. and Laborda, E. and Ward, K.R. and Tschulik, K. and Compton, R.G.
NANOSCALE. Volume: 5 (2013) - 2013 • 1847
Scaling between magnetic and lattice fluctuations in iron pnictide superconductors
Fernandes, R.M. and Böhmer, A.E. and Meingast, C. and Schmalian, J.
PHYSICAL REVIEW LETTERS. Volume: 111 (2013) - 2013 • 1846
Femtosecond-laser processing of nitrobiphenylthiol self-assembled monolayers
Schröter, A. and Franzka, S. and Koch, J. and Chichkov, B.N. and Ostendorf, A. and Hartmann, N.
APPLIED SURFACE SCIENCE. Volume: 278 (2013) - 2013 • 1845
Drift correction in ptychographic diffractive imaging
Beckers, M. and Senkbeil, T. and Gorniak, T. and Giewekemeyer, K. and Salditt, T. and Rosenhahn, A.
ULTRAMICROSCOPY. Volume: 126 (2013) - 2013 • 1844
Visualization of oxygen consumption of single living cells by scanning electrochemical microscopy: The influence of the faradaic tip reaction
Nebel, M. and Grützke, S. and Diab, N. and Schulte, A. and Schuhmann, W.
ANGEWANDTE CHEMIE - INTERNATIONAL EDITION. Volume: 52 (2013) - 2013 • 1843
Influence of surface states on quantum and transport lifetimes in high-quality undoped heterostructures
Wang, D.Q. and Chen, J.C.H. and Klochan, O. and Das Gupta, K. and Reuter, D. and Wieck, A.D. and Ritchie, D.A. and Hamilton, A.R.
PHYSICAL REVIEW B - CONDENSED MATTER AND MATERIALS PHYSICS. Volume: 87 (2013) - 2012 • 1842
Ordered mesoporous carbide-derived carbons prepared by soft templating
Borchardt, L. and Oschatz, M. and Lohe, M. and Presser, V. and Gogotsi, Y. and Kaskel, S.
CARBON. Volume: 50 (2012) - 2012 • 1841
Preparation and application of cellular and nanoporous carbides
Borchardt, L. and Hoffmann, C. and Oschatz, M. and Mammitzsch, L. and Petasch, U. and Herrmann, M. and Kaskel, S.
CHEMICAL SOCIETY REVIEWS. Volume: 41 (2012) - 2012 • 1840
Prediction of post-dynamic austenite-to-ferrite transformation and reverse transformation in a low-carbon steel by cellular automaton modeling
Zheng, C. and Raabe, D. and Li, D.
ACTA MATERIALIA. Volume: 60 (2012) - 2012 • 1839
Transition metal loaded silicon carbide-derived carbons with enhanced catalytic properties
Borchardt, L. and Hasché, F. and Lohe, M.R. and Oschatz, M. and Schmidt, F. and Kockrick, E. and Ziegler, C. and Lescouet, T. and Bachmatiuk, A. and Büchner, B. and Farrusseng, D. and Strasser, P. and Kaskel, S.
CARBON. Volume: 50 (2012) - 2012 • 1838
Thermally induced structural changes in incommensurate calcium barium niobate Ca 0.28Ba 0.72Nb 2O 6 (CBN28)
Graetsch, H.A. and Schreuer, J. and Burianek, M. and Mühlberg, M.
JOURNAL OF SOLID STATE CHEMISTRY. Volume: 196 (2012) - 2012 • 1837
Advanced methods and tools for reconstruction and analysis of grain boundaries from 3D-EBSD data sets
Konijnenberg, P.J. and Zaefferer, S. and Lee, S.-B. and Rollett, A.D. and Rohrer, G.S. and Raabe, D.
MATERIALS SCIENCE FORUM. Volume: 702-703 (2012) - 2012 • 1836
High throughput density functional investigations of the stability, electronic structure and thermoelectric properties of binary silicides
Opahle, I. and Madsen, G.K.H. and Drautz, R.
PHYSICAL CHEMISTRY CHEMICAL PHYSICS. Volume: 14 (2012) - 2012 • 1835
Numerical simulation of dynamic strain-induced austenite-ferrite transformation and post-dynamic kinetics in a low carbon steel
Zheng, C. and Raabe, D. and Li, D.
MATERIALS SCIENCE FORUM. Volume: 706-709 (2012) - 2012 • 1834
Multicomponent diffusion in garnets I: General theoretical considerations and experimental data for Fe-Mg systems
Borinski, S.A. and Hoppe, U. and Chakraborty, S. and Ganguly, J. and Bhowmik, S.K.
CONTRIBUTIONS TO MINERALOGY AND PETROLOGY. Volume: 164 (2012) - 2012 • 1833
Incommensurate modulation of calcium barium niobate (CBN28 and Ce:CBN28)
Graetsch, H.A. and Pandey, C.S. and Schreuer, J. and Burianek, M. and Mühlberg, M.
ACTA CRYSTALLOGRAPHICA SECTION B: STRUCTURAL SCIENCE. Volume: 68 (2012) - 2012 • 1832
Depth-filtered digital holography
Koukourakis, N. and Jaedicke, V. and Adinda-Ougba, A. and Goebel, S. and Wiethoff, H. and Höpfner, H. and Gerhardt, N.C. and Hofmann, M.R.
OPTICS EXPRESS. Volume: 20 (2012) - 2012 • 1831
CuO/ZnO nanocomposite gas sensors developed by a plasma-assisted route
Simon, Q. and Barreca, D. and Gasparotto, A. and MacCato, C. and Tondello, E. and Sada, C. and Comini, E. and Sberveglieri, G. and Banerjee, M. and Xu, K. and Devi, A. and Fischer, R.A.
CHEMPHYSCHEM. Volume: 13 (2012) - 2012 • 1830
First-principles study of the thermodynamic and elastic properties of eutectic Fe-Ti alloys
Zhu, L.-F. and Friák, M. and Dick, A. and Grabowski, B. and Hickel, T. and Liot, F. and Holec, D. and Schlieter, A. and Kühn, U. and Eckert, J. and Ebrahimi, Z. and Emmerich, H. and Neugebauer, J.
ACTA MATERIALIA. Volume: 60 (2012) - 2012 • 1829
Photoluminescence and optical gain of Ga(NAsP) heterostructures pseudomorphically grown on silicon (001) substrate
Koukourakis, N. and Klimasch, M. and Funke, D.A. and Gerhardt, N.C. and Hofmann, M.R. and Liebich, S. and Zimprich, M. and Kunert, B. and Volz, K. and Stolz, W.
PROCEEDINGS OF SPIE - THE INTERNATIONAL SOCIETY FOR OPTICAL ENGINEERING. Volume: 8266 (2012) - 2012 • 1828
Ag/ZnO nanomaterials as high performance sensors for flammable and toxic gases
Simon, Q. and Barreca, D. and Gasparotto, A. and MacCato, C. and Tondello, E. and Sada, C. and Comini, E. and Devi, A. and Fischer, R.A.
NANOTECHNOLOGY. Volume: 23 (2012) - 2012 • 1827
High room-temperature optical gain in Ga(NAsP)/Si heterostructures
Koukourakis, N. and Bückers, C. and Funke, D.A. and Gerhardt, N.C. and Liebich, S. and Chatterjee, S. and Lange, C. and Zimprich, M. and Volz, K. and Stolz, W. and Kunert, B. and Koch, S.W. and Hofmann, M.R.
APPLIED PHYSICS LETTERS. Volume: 100 (2012) - 2012 • 1826
Influence of particle diameter and material properties on mixing of monodisperse spheres on a grate: Experiments and discrete element simulation
Simsek, E. and Sudbrock, F. and Wirtz, S. and Scherer, V.
POWDER TECHNOLOGY. Volume: 221 (2012) - 2012 • 1825
Spatial and temporal coherence properties of single free-electron laser pulses
Singer, A. and Sorgenfrei, F. and Mancuso, A.P. and Gerasimova, N. and Yefanov, O.M. and Gulden, J. and Gorniak, T. and Senkbeil, T. and Sakdinawat, A. and Liu, Y. and Attwood, D. and Dziarzhytski, S. and Mai, D.D. and Treusch, R. and Weckert, E. and Salditt, T. and Rosenhahn, A. and Wurth, W. and Vartanyants, I.A.
OPTICS EXPRESS. Volume: 20 (2012) - 2012 • 1824
Hydrogen-induced cracking at grain and twin boundaries in an Fe-Mn-C austenitic steel
Koyama, M. and Akiyama, E. and Sawaguchi, T. and Raabe, D. and Tsuzaki, K.
SCRIPTA MATERIALIA. Volume: 66 (2012) - 2012 • 1823
Synthesis of high surface area ZnO powder by continuous precipitation
Boz, I. and Kaluza, S. and Boroǧlu, M.S. and Muhler, M.
MATERIALS RESEARCH BULLETIN. Volume: 47 (2012) - 2012 • 1822
Carbide-derived carbon monoliths with hierarchical pore architectures
Oschatz, M. and Borchardt, L. and Thommes, M. and Cychosz, K.A. and Senkovska, I. and Klein, N. and Frind, R. and Leistner, M. and Presser, V. and Gogotsi, Y. and Kaskel, S.
ANGEWANDTE CHEMIE - INTERNATIONAL EDITION. Volume: 51 (2012) - 2012 • 1821
Nano photoelectron ioniser chip using LaB 6 for ambient pressure trace gas detection
Zimmer, C.M. and Kieschnick, M. and Kallis, K.T. and Schubert, J. and Kunze, U. and Doll, T.
MICROELECTRONIC ENGINEERING. Volume: 98 (2012) - 2012 • 1820
Deposition of La 1-xSr xFe 1-yCo yO 3-δ coatings with different phase compositions and microstructures by low-pressure plasma spraying-thin film (LPPS-TF) processes
Zotov, N. and Hospach A. and Mauer G. and Sebold D. and Vaßen, R.
JOURNAL OF THERMAL SPRAY TECHNOLOGY. Volume: 21 (2012) - 2012 • 1819
Gas-solid interactions during nonisothermal heat treatment of a high-strength CrMnCN austenitic steel powder: Influence of atmospheric conditions and heating rate on the densification behavior
Krasokha, N. and Weber, S. and Huth, S. and Zumsande, K. and Theisen, W.
METALLURGICAL AND MATERIALS TRANSACTIONS A: PHYSICAL METALLURGY AND MATERIALS SCIENCE. Volume: 43 (2012) - 2012 • 1818
Laser direct writing of nanocompounds
Ostendorf, A. and Chakif, M. and Guo, Q.
MATERIALS RESEARCH SOCIETY SYMPOSIUM PROCEEDINGS. Volume: 1365 (2012) - 2012 • 1817
Surfactant-induced nonhydrolytic synthesis of phase-pure ZrO2 nanoparticles from metal-organic and oxocluster precursors
Sliem, M.A. and Schmidt, D.A. and Bétard, A. and Kalidindi, S.B. and Gross, S. and Havenith, M. and Devi, A. and Fischer, R.A.
CHEMISTRY OF MATERIALS. Volume: 24 (2012) - 2012 • 1816
Characterization of the surface of Fe-19Mn-18Cr-C-N during heat treatment in a high vacuum - An XPS study
Zumsande, K. and Weddeling, A. and Hryha, E. and Huth, S. and Nyborg, L. and Weber, S. and Krasokha, N. and Theisen, W.
MATERIALS CHARACTERIZATION. Volume: 71 (2012) - 2012 • 1815
Preparation, microstructure characterization and catalytic performance of Cu/ZnO and ZnO/Cu composite nanoparticles for liquid phase methanol synthesis
Sliem, M.A. and Turner, S. and Heeskens, D. and Kalidindi, S.B. and Tendeloo, G.V. and Muhler, M. and Fischer, R.A.
PHYSICAL CHEMISTRY CHEMICAL PHYSICS. Volume: 14 (2012) - 2012 • 1814
In situ investigation of the gas-solid interaction between high-alloyed steel powder and nitrogen by energy dispersive diffraction
Zumsande, K. and Krasokha, N. and Huth, S. and Weber, S. and Theisen, W.
JOURNAL OF MATERIALS SCIENCE. Volume: 47 (2012) - 2012 • 1813
Surface acoustic wave controlled carrier injection into self-assembled quantum dots and quantum posts
Krenner, H.J. and Völk, S. and Schülein, F.J.R. and Knall, F. and Wixforth, A. and Reuter, D. and Wieck, A.D. and Kim, H. and Truong, T.A. and Petroff, P.M.
PHYSICA STATUS SOLIDI (C) CURRENT TOPICS IN SOLID STATE PHYSICS. Volume: 9 (2012) - 2012 • 1812
Domain structure in the tetragonal phase of BaTiO 3-From bulk to nanoparticles
Grnebohm, A. and Gruner, M.E. and Entel, P.
FERROELECTRICS. Volume: 426 (2012) - 2012 • 1811
Thermal expansion and elastic properties of mullite-type Bi 2Ga 4O 9 and Bi 2Fe 4O 9 single crystals
Krenzel, T.F. and Schreuer, J. and Gesing, T.M. and Burianek, M. and Mühlberg, M. and Schneider, H.
INTERNATIONAL JOURNAL OF MATERIALS RESEARCH. Volume: 103 (2012) - 2012 • 1810
A sodium-containing quasicrystal: Using gold to enhance sodium's covalency in intermetallic compounds
Smetana, V. and Lin, Q. and Pratt, D.K. and Kreyssig, A. and Ramazanoglu, M. and Corbett, J.D. and Goldman, A.I. and Miller, G.J.
ANGEWANDTE CHEMIE - INTERNATIONAL EDITION. Volume: 51 (2012) - 2012 • 1809
A scheme to combine molecular dynamics and dislocation dynamics
Brinckmann, S. and Mahajan, D.K. and Hartmaier, A.
MODELLING AND SIMULATION IN MATERIALS SCIENCE AND ENGINEERING. Volume: 20 (2012) - 2012 • 1808
On the effect of grain boundary segregation on creep and creep rupture
Otto, F. and Viswanathan, G.B. and Payton, E.J. and Frenzel, J. and Eggeler, G.
ACTA MATERIALIA. Volume: 60 (2012) - 2012 • 1807
Novel temperature dependent tensile test of freestanding copper thin film structures
Smolka, M. and Motz, C. and Detzel, T. and Robl, W. and Griesser, T. and Wimmer, A. and Dehm, G.
REVIEW OF SCIENTIFIC INSTRUMENTS. Volume: 83 (2012) - 2012 • 1806
Thermal behavior of MOCVD-grown Cu-clusters on ZnO(1010)
Kroll, M. and Löber, T. and Schott, V. and Wöll, C. and Köhler, U.
PHYSICAL CHEMISTRY CHEMICAL PHYSICS. Volume: 14 (2012) - 2012 • 1805
The effectiveness of coincidence site lattice criteria in predicting creep cavitation resistance
Otto, F. and Payton, E.J. and Frenzel, J. and Eggeler, G.
JOURNAL OF MATERIALS SCIENCE. Volume: 47 (2012) - 2012 • 1804
Critical dynamics of an isothermal compressible nonideal fluid
Gross, M. and Varnik, F.
PHYSICAL REVIEW E - STATISTICAL, NONLINEAR, AND SOFT MATTER PHYSICS. Volume: 86 (2012) - 2012 • 1803
Experimental and numerical investigation of the bulk behavior of wood pellets on a model type grate
Kruggel-Emden, H. and Sudbrock, F. and Wirtz, S. and Scherer, V.
GRANULAR MATTER. Volume: 14 (2012) - 2012 • 1802
Simulation of static critical phenomena in nonideal fluids with the lattice Boltzmann method
Gross, M. and Varnik, F.
PHYSICAL REVIEW E - STATISTICAL, NONLINEAR, AND SOFT MATTER PHYSICS. Volume: 85 (2012) - 2012 • 1801
Simulation of cluster sintering, dipolar chain formation, and ferroelectric nanoparticulate systems
Grünebohm, A. and Hucht, A. and Meyer, R. and Comtesse, D. and Entel, P.
NANOSCIENCE AND TECHNOLOGY. Volume: 79 (2012) - 2012 • 1800
First-principles study of the influence of (110) strain on the ferroelectric trends of TiO 2
GrüNebohm, A. and Siewert, M. and Ederer, C. and Entel, P.
FERROELECTRICS. Volume: 429 (2012) - 2012 • 1799
Layered precursors for new zeolitic materials: Synthesis and characterization of B-RUB-39 and its condensation product B-RUB-41
Grünewald-Lüke, A. and Gies, H. and Müller, U. and Yilmaz, B. and Imai, H. and Tatsumi, T. and Xie, B. and Xiao, F.-S. and Bao, X. and Zhang, W. and De Vos, D.
MICROPOROUS AND MESOPOROUS MATERIALS. Volume: 147 (2012) - 2012 • 1798
Thermodynamic and physical properties of FeAl and Fe 3Al: An atomistic study by EAM simulation
Ouyang, Y. and Tong, X. and Li, C. and Chen, H. and Tao, X. and Hickel, T. and Du, Y.
PHYSICA B: CONDENSED MATTER. Volume: 407 (2012) - 2012 • 1797
Kinetics and driving forces of abnormal grain growth in thin Cu films
Sonnweber-Ribic, P. and Gruber, P.A. and Dehm, G. and Strunk, H.P. and Arzt, E.
ACTA MATERIALIA. Volume: 60 (2012) - 2012 • 1796
Detection of DNA hybridization using electrochemical impedance spectroscopy and surface enhanced Raman scattering
Grützke, S. and Abdali, S. and Schuhmann, W. and Gebala, M.
ELECTROCHEMISTRY COMMUNICATIONS. Volume: 19 (2012) - 2012 • 1795
Impedance of single-walled carbon nanotube fibers
Ksenevich, V.K. and Gorbachuk, N.I. and Poklonski, N.A. and Samuilov, V.A. and Kozlov, M.E. and Wieck, A.D.
FULLERENES NANOTUBES AND CARBON NANOSTRUCTURES. Volume: 20 (2012) - 2012 • 1794
Mass transport controlled oxygen reduction at anthraquinone modified 3D-CNT electrodes with immobilized Trametes hirsuta laccase
Sosna, M. and Stoica, L. and Wright, E. and Kilburn, J.D. and Schuhmann, W. and Bartlett, P.N.
PHYSICAL CHEMISTRY CHEMICAL PHYSICS. Volume: 14 (2012) - 2012 • 1793
Thermal transport in phase-change materials from atomistic simulations
Sosso, G.C. and Donadio, D. and Caravati, S. and Behler, J. and Bernasconi, M.
PHYSICAL REVIEW B - CONDENSED MATTER AND MATERIALS PHYSICS. Volume: 86 (2012) - 2012 • 1792
Neural network interatomic potential for the phase change material GeTe
Sosso, G.C. and Miceli, G. and Caravati, S. and Behler, J. and Bernasconi, M.
PHYSICAL REVIEW B - CONDENSED MATTER AND MATERIALS PHYSICS. Volume: 85 (2012) - 2012 • 1791
Cyclotron-resonant exciton transfer between the nearly free and strongly localized radiative states of a two-dimensional hole gas in a high magnetic field
Bryja, L. and Jadczak, J. and Wójs, A. and Bartsch, G. and Yakovlev, D.R. and Bayer, M. and Plochocka, P. and Potemski, M. and Reuter, D. and Wieck, A.D.
PHYSICAL REVIEW B - CONDENSED MATTER AND MATERIALS PHYSICS. Volume: 85 (2012) - 2012 • 1790
Ba 1-xK xMn 2As 2: An antiferromagnetic local-moment metal
Pandey, A. and Dhaka, R.S. and Lamsal, J. and Lee, Y. and Anand, V.K. and Kreyssig, A. and Heitmann, T.W. and McQueeney, R.J. and Goldman, A.I. and Harmon, B.N. and Kaminski, A. and Johnston, D.C.
PHYSICAL REVIEW LETTERS. Volume: 108 (2012) - 2012 • 1789
Lamina cribrosa thickening in early glaucoma predicted by a microstructure motivated growth and remodeling approach
Grytz, R. and Sigal, I.A. and Ruberti, J.W. and Meschke, G. and Crawford Downs, J.
MECHANICS OF MATERIALS. Volume: 44 (2012) - 2012 • 1788
The mechanical shear behavior of Al single crystals and grain boundaries
Pang, X. and Ahmed, N. and Janisch, R. and Hartmaier, A.
JOURNAL OF APPLIED PHYSICS. Volume: 112 (2012) - 2012 • 1787
Activated carbon supported molybdenum carbides as cheap and highly efficient catalyst in the selective hydrogenation of naphthalene to tetralin
Pang, M. and Liu, C. and Xia, W. and Muhler, M. and Liang, C.
GREEN CHEMISTRY. Volume: 14 (2012) - 2012 • 1786
Noise thermometry in narrow two-dimensional electron gas heat baths connected to a quasi-one-dimensional interferometer
Buchholz, S.S. and Sternemann, E. and Chiatti, O. and Reuter, D. and Wieck, A.D. and Fischer, S.F.
PHYSICAL REVIEW B - CONDENSED MATTER AND MATERIALS PHYSICS. Volume: 85 (2012) - 2012 • 1785
Chemically modified jute fibre reinforced non-pressure (NP) concrete pipes with improved mechanical properties
Kundu, S.P. and Chakraborty, S. and Roy, A. and Adhikari, B. and Majumder, S.B.
CONSTRUCTION AND BUILDING MATERIALS. Volume: 37 (2012) - 2012 • 1784
Au, @ZrO 2 yolk-shell catalysts for CO oxidation: Study of particle size effect by ex-post size control of Au cores
Güttel, R. and Paul, M. and Galeano, C. and Schüth, F.
JOURNAL OF CATALYSIS. Volume: 289 (2012) - 2012 • 1783
Synthesis of an improved hierarchical carbon-fiber composite as a catalyst support for platinum and its application in electrocatalysis
Kundu, S. and Nagaiah, T.C. and Chen, X. and Xia, W. and Bron, M. and Schuhmann, W. and Muhler, M.
CARBON. Volume: 50 (2012) - 2012 • 1782
A test for Archimedeanity in bivariate copula models
Bücher, A. and Dette, H. and Volgushev, S.
JOURNAL OF MULTIVARIATE ANALYSIS. Volume: 110 (2012) - 2012 • 1781
Hyperfine interaction mediated exciton spin relaxation in (In,Ga)As quantum dots
Kurtze, H. and Yakovlev, D.R. and Reuter, D. and Wieck, A.D. and Bayer, M.
PHYSICAL REVIEW B - CONDENSED MATTER AND MATERIALS PHYSICS. Volume: 85 (2012) - 2012 • 1780
Rapid alloy prototyping: Compositional and thermo-mechanical high throughput bulk combinatorial design of structural materials based on the example of 30Mn-1.2C-xAl triplex steels
Springer, H. and Raabe, D.
ACTA MATERIALIA. Volume: 60 (2012) - 2012 • 1779
Preparation of 24 ternary thin film materials libraries on a single substrate in one experiment for irreversible high-throughput studies
Buenconsejo, P.J.S. and Siegel, A. and Savan, A. and Thienhaus, S. and Ludwig, Al.
ACS COMBINATORIAL SCIENCE. Volume: 14 (2012) - 2012 • 1778
Deposition and characteristics of submicrometer-structured thermal barrier coatings by suspension plasma spraying
Guignard, A. and Mauer, G. and Vaßen, R. and Stöver, D.
JOURNAL OF THERMAL SPRAY TECHNOLOGY. Volume: 21 (2012) - 2012 • 1777
On the presence of work-hardened zones around fibers in a short-fiber-reinforced Al metal matrix composite
Kurumlu, D. and Payton, E.J. and Somsen, C. and Dlouhy, A. and Eggeler, G.
ACTA MATERIALIA. Volume: 60 (2012) - 2012 • 1776
Bond order potentials for fracture, wear, and plasticity
Pastewka, L. and Mrovec, M. and Moseler, M. and Gumbsch, P.
MRS BULLETIN. Volume: 37 (2012) - 2012 • 1775
Orbital directing effects in copper and zinc based paddle-wheel metal organic frameworks: The origin of flexibility
Bureekaew, S. and Amirjalayer, S. and Schmid, R.
JOURNAL OF MATERIALS CHEMISTRY. Volume: 22 (2012) - 2012 • 1774
High-temperature strength and damage evolution in short fiber reinforced aluminum alloys studied by miniature creep testing and synchrotron microtomography
Kurumlu, D. and Payton, E.J. and Young, M.L. and Schöbel, M. and Requena, G. and Eggeler, G.
ACTA MATERIALIA. Volume: 60 (2012) - 2012 • 1773
Evaluation of different methods to eliminate adherent endotoxin of polyethylene wear particles
Paulus, A.C. and Schröder, C. and Sievers, B. and Frenzel, J. and Jansson, V. and Utzschneider, S.
WEAR. Volume: 294-295 (2012) - 2012 • 1772
Laser direct writing of high refractive index polymer/TiO 2 nanocomposites
Guo, Q. and Ghadiri, R. and Xiao, S. and Esen, C. and Medenbach, O. and Ostendorf, A.
PROCEEDINGS OF SPIE - THE INTERNATIONAL SOCIETY FOR OPTICAL ENGINEERING. Volume: 8243 (2012) - 2012 • 1771
Single crystal growth and characterization of mullite-type Bi 2Mn 4O 10
Burianek, M. and Krenzel, T.F. and Schmittner, M. and Schreuer, J. and Fischer, R.X. and Mühlberg, M. and Nénertd, G. and Schneider, H. and Gesing, T.M.
INTERNATIONAL JOURNAL OF MATERIALS RESEARCH. Volume: 103 (2012) - 2012 • 1770
Using laser microfabrication to write conductive polymer/swnts nanocomposites
Guo, Q. and Xiao, S. and Aumann, A. and Jaeger, M. and Chakif, M. and Ghadiri, R. and Esen, C. and Ma, M. and Ostendorf, A.
JOURNAL OF LASER MICRO NANOENGINEERING. Volume: 7 (2012) - 2012 • 1769
Pearlite revisited
Steinbach, I. and Plapp, M.
CONTINUUM MECHANICS AND THERMODYNAMICS. Volume: 24 (2012) - 2012 • 1768
Origin of gate hysteresis in p-type Si-doped AlGaAs/GaAs heterostructures
Burke, A.M. and Waddington, D.E.J. and Carrad, D.J. and Lyttleton, R.W. and Tan, H.H. and Reece, P.J. and Klochan, O. and Hamilton, A.R. and Rai, A. and Reuter, D. and Wieck, A.D. and Micolich, A.P.
PHYSICAL REVIEW B - CONDENSED MATTER AND MATERIALS PHYSICS. Volume: 86 (2012) - 2012 • 1767
On the nature of internal interfaces in a tempered martensite ferritic steel and their evolution during long-term creep
Payton, E.J. and Aghajani, A. and Otto, F. and Eggeler, G. and Yardley, V.A.
SCRIPTA MATERIALIA. Volume: 66 (2012) - 2012 • 1766
Phase-field model with finite interface dissipation
Steinbach, I. and Zhang, L. and Plapp, M.
ACTA MATERIALIA. Volume: 60 (2012) - 2012 • 1765
Enhanced direct electron transfer between laccase and hierarchical carbon microfibers/carbon nanotubes composite electrodes. Comparison of three enzyme immobilization methods
Gutiérrez-Sánchez, C. and Jia, W. and Beyl, Y. and Pita, M. and Schuhmann, W. and De Lacey, A.L. and Stoica, L.
ELECTROCHIMICA ACTA. Volume: 82 (2012) - 2012 • 1764
Combinatorial development of nanoporous WO 3 thin film photoelectrodes for solar water splitting by dealloying of binary alloys
Stepanovich, A. and Sliozberg, K. and Schuhmann, W. and Ludwig, Al.
INTERNATIONAL JOURNAL OF HYDROGEN ENERGY. Volume: 37 (2012) - 2012 • 1763
Multistage strain hardening through dislocation substructure and twinning in a high strength and ductile weight-reduced Fe-Mn-Al-C steel
Gutierrez-Urrutia, I. and Raabe, D.
ACTA MATERIALIA. Volume: 60 (2012) - 2012 • 1762
Grain size effect on strain hardening in twinning-induced plasticity steels
Gutierrez-Urrutia, I. and Raabe, D.
SCRIPTA MATERIALIA. Volume: 66 (2012) - 2012 • 1761
Dislocation density measurement by electron channeling contrast imaging in a scanning electron microscope
Gutierrez-Urrutia, I. and Raabe, D.
SCRIPTA MATERIALIA. Volume: 66 (2012) - 2012 • 1760
Microstructure evolution during recrystallization in dual-phase steels
Peranio, N. and Roters, F. and Raabe, D.
MATERIALS SCIENCE FORUM. Volume: 715-716 (2012) - 2012 • 1759
Optimizing the deposition of hydrogen evolution sites on suspended semiconductor particles using on-line photocatalytic reforming of aqueous methanol solutions
Busser, G.W. and Mei, B. and Muhler, M.
CHEMSUSCHEM. Volume: 5 (2012) - 2012 • 1758
Study of deformation twinning and planar slip in a TWIP steel by electron channeling contrast imaging in a SEM
Gutierrez-Urrutia, I. and Raabe, D.
MATERIALS SCIENCE FORUM. Volume: 702-703 (2012) - 2012 • 1757
Spark plasma sintering synthesis and mechanical spectroscopy of the ω-Al 0.7Cu 0.2Fe 0.1 phase
Laplanche, G. and Gadaud, P. and Bonneville, J. and Joulain, A. and Gauthier-Brunet, V. and Dubois, S. and Jay, F.
JOURNAL OF MATERIALS SCIENCE. Volume: 47 (2012) - 2012 • 1756
New insights on quantitative microstructure characterization by electron channeling contrast imaging under controlled diffraction conditions in SEM
Gutierrez-Urrutia, I. and Raabe, D.
MICROSCOPY AND MICROANALYSIS. Volume: 18 (2012) - 2012 • 1755
Detailed kinetic modeling of methanol synthesis over a ternary copper catalyst
Peter, M. and Fichtl, M.B. and Ruland, H. and Kaluza, S. and Muhler, M. and Hinrichsen, O.
CHEMICAL ENGINEERING JOURNAL. Volume: 203 (2012) - 2012 • 1754
On the effect of manganese on grain size stability and hardenability in ultrafine-grained ferrite/martensite dual-phase steels
Calcagnotto, M. and Ponge, D. and Raabe, D.
METALLURGICAL AND MATERIALS TRANSACTIONS A: PHYSICAL METALLURGY AND MATERIALS SCIENCE. Volume: 43 (2012) - 2012 • 1753
The origin of gate hysteresis in p-type Si-doped AlGaAs/GaAs heterostructures
Carrad, D. and Burke, A.M. and Waddington, D. and Lyttleton, R. and Tan, H.H. and Reece, P.J. and Klochan, O. and Hamilton, A.R. and Rai, A. and Reuter, D. and Wieck, A.D. and Micolich, A.P.
CONFERENCE ON OPTOELECTRONIC AND MICROELECTRONIC MATERIALS AND DEVICES, PROCEEDINGS, COMMAD. Volume: (2012) - 2012 • 1752
Enzymatic fuel cells: Recent progress
Leech, D. and Kavanagh, P. and Schuhmann, W.
ELECTROCHIMICA ACTA. Volume: 84 (2012) - 2012 • 1751
Electron spin orientation under in-plane optical excitation in GaAs quantum wells
Pfalz, S. and Winkler, R. and Ubbelohde, N. and Hägele, D. and Oestreich, M.
PHYSICAL REVIEW B - CONDENSED MATTER AND MATERIALS PHYSICS. Volume: 86 (2012) - 2012 • 1750
On the properties of Ni-rich NiTi shape memory parts produced by selective laser melting
Haberland, C. and Meier, H. and Frenzel, J.
ASME 2012 CONFERENCE ON SMART MATERIALS, ADAPTIVE STRUCTURES AND INTELLIGENT SYSTEMS, SMASIS 2012. Volume: 1 (2012) - 2012 • 1749
Ab initio EPR parameters for dangling-bond defect complexes in silicon: Effect of Jahn-Teller distortion
Pfanner, G. and Freysoldt, C. and Neugebauer, J. and Gerstmann, U.
PHYSICAL REVIEW B - CONDENSED MATTER AND MATERIALS PHYSICS. Volume: 85 (2012) - 2012 • 1748
Suspension and air plasma-sprayed ceramic thermal barrier coatings with high infrared reflectance
Stuke, A. and Kassner, H. and Marqués, J.-L. and Vassen, R. and Stöver, D. and Carius, R.
INTERNATIONAL JOURNAL OF APPLIED CERAMIC TECHNOLOGY. Volume: 9 (2012) - 2012 • 1747
An edge-based imbricate finite element method (EI-FEM) with full and reduced integration
Cazes, F. and Meschke, G.
COMPUTERS AND STRUCTURES. Volume: 106-107 (2012) - 2012 • 1746
Orientation dependence of stress-induced phase transformation and dislocation plasticity in NiTi shape memory alloys on the micro scale
Pfetzing-Micklich, J. and Ghisleni, R. and Simon, T. and Somsen, C. and Michler, J. and Eggeler, G.
MATERIALS SCIENCE AND ENGINEERING A. Volume: 538 (2012) - 2012 • 1745
Micropatterning of Fe-based bulk metallic glass surfaces by pulsed electrochemical micromachining
Sueptitz, R. and Tschulik, K. and Becker, C. and Stoica, M. and Uhlemann, M. and Eckert, J. and Gebert, A.
JOURNAL OF MATERIALS RESEARCH. Volume: 27 (2012) - 2012 • 1744
Polycrystal model of the mechanical behavior of a Mo-TiC 30 vol.% metal-ceramic composite using a three-dimensional microstructure map obtained by dual beam focused ion beam scanning electron microscopy
Cédat, D. and Fandeur, O. and Rey, C. and Raabe, D.
ACTA MATERIALIA. Volume: 60 (2012) - 2012 • 1743
A model for the evolution of laminates in finite-strain elastoplasticity
Hackl, K. and Heinz, S. and Mielke, A.
ZAMM ZEITSCHRIFT FUR ANGEWANDTE MATHEMATIK UND MECHANIK. Volume: 92 (2012) - 2012 • 1742
All-optical tunability of microdisk lasers via photo-adressable polyelectrolyte functionalization
Piegdon, K.A. and Lexow, M. and Grundmeier, G. and Kitzerow, H.-S. and Pärschke, K. and Mergel, D. and Reuter, D. and Wieck, A.D. and Meier, C.
OPTICS EXPRESS. Volume: 20 (2012) - 2012 • 1741
In situ study of γ-TiAl lamellae formation in supersaturated α 2-Ti 3Al grains
Cha, L. and Schmoelzer, T. and Zhang, Z. and Mayer, S. and Clemens, H. and Staron, P. and Dehm, G.
ADVANCED ENGINEERING MATERIALS. Volume: 14 (2012) - 2012 • 1740
Generation and evolution of inelastic microstructures - An overview
Hackl, K. and Hoppe, U. and Kochmann, D.M.
GAMM MITTEILUNGEN. Volume: 35 (2012) - 2012 • 1739
The silica-water interface: How the silanols determine the surface acidity and modulate the water properties
Sulpizi, M. and Gaigeot, M.-P. and Sprik, M.
JOURNAL OF CHEMICAL THEORY AND COMPUTATION. Volume: 8 (2012) - 2012 • 1738
Catalytic properties of nickel bis(phosphinite) pincer complexes in the reduction of CO 2 to methanol derivatives
Chakraborty, S. and Patel, Y.J. and Krause, J.A. and Guan, H.
POLYHEDRON. Volume: 32 (2012) - 2012 • 1737
Influence of impurity elements on the nucleation and growth of Si in high purity melt-spun Al-Si-based alloys
Li, J.H. and Zarif, M.Z. and Dehm, G. and Schumacher, P.
PHILOSOPHICAL MAGAZINE. Volume: 92 (2012) - 2012 • 1736
Scale effects in convoluted thermal/spatial statistics of plasticity initiation in small stressed volumes during nanoindentation
Li, T.L. and Bei, H. and Morris, J.R. and George, E.P. and Gao, Y.F.
MATERIALS SCIENCE AND TECHNOLOGY (UNITED KINGDOM). Volume: 28 (2012) - 2012 • 1735
Rapid and surfactant-free synthesis of bimetallic Pt-Cu nanoparticles simply via ultrasound-assisted redox replacement
Sun, Z. and Masa, J. and Xia, W. and König, D. and Ludwig, Al. and Li, Z.-A. and Farle, M. and Schuhmann, W. and Muhler, M.
ACS CATALYSIS. Volume: 2 (2012) - 2012 • 1734
Highly concentrated aqueous dispersions of graphene exfoliated by sodium taurodeoxycholate: Dispersion behavior and potential application as a catalyst support for the oxygen-reduction reaction
Sun, Z. and Masa, J. and Liu, Z. and Schuhmann, W. and Muhler, M.
CHEMISTRY - A EUROPEAN JOURNAL. Volume: 18 (2012) - 2012 • 1733
Characterization of oxidation and reduction of Pt-Ru and Pt-Rh-Ru alloys by atom probe tomography and comparison with Pt-Rh
Li, T. and Bagot, P.A.J. and Marquis, E.A. and Tsang, S.C.E. and Smith, G.D.W.
JOURNAL OF PHYSICAL CHEMISTRY C. Volume: 116 (2012) - 2012 • 1732
Prediction of thermodynamic properties of natural gases using Monte Carlo simulations
Piyanzina, I. and Lysogorskiy, Yu. and Nedopekin, O.
JOURNAL OF PHYSICS: CONFERENCE SERIES. Volume: 394 (2012) - 2012 • 1731
Characterization of oxidation and reduction of a Palladium-Rhodium alloy by atom-probe tomography
Li, T. and Bagot, P.A.J. and Marquis, E.A. and Tsang, S.C.E. and Smith, G.D.W.
JOURNAL OF PHYSICAL CHEMISTRY C. Volume: 116 (2012) - 2012 • 1730
A new synthesis route for Os-complex modified redox polymers for potential biofuel cell applications
Pöller, S. and Beyl, Y. and Vivekananthan, J. and Guschin, D.A. and Schuhmann, W.
BIOELECTROCHEMISTRY. Volume: 87 (2012) - 2012 • 1729
Microstructural impact of anodic coatings on the electrochemical chlorine evolution reaction
Chen, R. and Trieu, V. and Zeradjanin, A.R. and Natter, H. and Teschner, D. and Kintrup, J. and Bulan, A. and Schuhmann, W. and Hempelmann, R.
PHYSICAL CHEMISTRY CHEMICAL PHYSICS. Volume: 14 (2012) - 2012 • 1728
Quantitative studies on the oxygen and nitrogen functionalization of carbon Nanotubes Performed in the Gas Phase
Li, C. and Zhao, A. and Xia, W. and Liang, C. and Muhler, M.
JOURNAL OF PHYSICAL CHEMISTRY C. Volume: 116 (2012) - 2012 • 1727
Fabrication and characterization of ambipolar devices on an undoped AlGaAs/GaAs heterostructure
Chen, J.C.H. and Wang, D.Q. and Klochan, O. and Micolich, A.P. and Das Gupta, K. and Sfigakis, F. and Ritchie, D.A. and Reuter, D. and Wieck, A.D. and Hamilton, A.R.
APPLIED PHYSICS LETTERS. Volume: 100 (2012) - 2012 • 1726
Evolution of strength and microstructure during annealing of heavily cold-drawn 6.3 GPa hypereutectoid pearlitic steel wire
Li, Y.J. and Choi, P. and Goto, S. and Borchers, C. and Raabe, D. and Kirchheim, R.
ACTA MATERIALIA. Volume: 60 (2012) - 2012 • 1725
On the correlation between thermal cycle and formation of intermetallic phases at the interface of laser-welded aluminum-steel overlap joints
Szczepaniak, A. and Fan, J. and Kostka, A. and Raabe, D.
ADVANCED ENGINEERING MATERIALS. Volume: 14 (2012) - 2012 • 1724
Structural Stability of Topologically Close-Packed Phases: Understanding Experimental Trends in Terms of the Electronic Structure
Hammerschmidt, T. and Seiser, B. and Cak, M. and Drautz, R. and Pettifor, D.G.
PROCEEDINGS OF THE INTERNATIONAL SYMPOSIUM ON SUPERALLOYS 2012. Volume: (2012) - 2012 • 1723
Synthesis, crystal structures, and hydrogen-storage properties of Eu(AlH 4) 2 and Sr(AlH 4) 2 and of their decomposition intermediates, EuAlH 5 and SrAlH 5
Pommerin, A. and Wosylus, A. and Felderhoff, M. and Schüth, F. and Weidenthaler, C.
INORGANIC CHEMISTRY. Volume: 51 (2012) - 2012 • 1722
Hole Localization and Thermochemistry of Oxidative Dehydrogenation of Aqueous Rutile TiO 2(110)
Cheng, J. and Sulpizi, M. and Vandevondele, J. and Sprik, M.
CHEMCATCHEM. Volume: 4 (2012) - 2012 • 1721
9-GHz wideband CMOS RX and TX front-ends for universal radio applications
Hampel, S.K. and Schmitz, O. and Tiebout, M. and Mertens, K. and Rolfes, I.
IEEE TRANSACTIONS ON MICROWAVE THEORY AND TECHNIQUES. Volume: 60 (2012) - 2012 • 1720
Stability of platinum nanoparticles supported on SiO2/Si(111): A high-pressure X-ray photoelectron spectroscopy study
Porsgaard, S. and Merte, L.R. and Ono, L.K. and Behafarid, F. and Matos, J. and Helveg, S. and Salmeron, M. and Roldan Cuenya, B. and Besenbacher, F.
ACS NANO. Volume: 6 (2012) - 2012 • 1719
Shear-induced anisotropic decay of correlations in hard-sphere colloidal glasses
Chikkadi, V. and Mandal, S. and Nienhuis, B. and Raabe, D. and Varnik, F. and Schall, P.
EPL. Volume: 100 (2012) - 2012 • 1718
Intrinsic spin fluctuations reveal the dynamical response function of holes coupled to nuclear spin baths in (In,Ga)as quantum dots
Li, Y. and Sinitsyn, N. and Smith, D.L. and Reuter, D. and Wieck, A.D. and Yakovlev, D.R. and Bayer, M. and Crooker, S.A.
PHYSICAL REVIEW LETTERS. Volume: 108 (2012) - 2012 • 1717
Film deposition on the inner surface of tubes using atmospheric-pressure Ar-CH 4, Ar-C 2H 2 and Ar-C 2H 2-H 2 plasmas: Interpretation of film properties from plasma-chemical kinetics
Pothiraja, R. and Engelhardt, M. and Bibinov, N. and Awakowicz, P.
JOURNAL OF PHYSICS D: APPLIED PHYSICS. Volume: 45 (2012) - 2012 • 1716
Dislocation starvation and exhaustion hardening in Mo alloy nanofibers
Chisholm, C. and Bei, H. and Lowry, M.B. and Oh, J. and Syed Asif, S.A. and Warren, O.L. and Shan, Z.W. and George, E.P. and Minor, A.M.
ACTA MATERIALIA. Volume: 60 (2012) - 2012 • 1715
The biocompatibility of metal-organic framework coatings: An investigation on the stability of SURMOFs with regard to water and selected cell culture media
Hanke, M. and Arslan, H.K. and Bauer, S. and Zybaylo, O. and Christophis, C. and Gliemann, H. and Rosenhahn, A. and Wöll, C.
LANGMUIR. Volume: 28 (2012) - 2012 • 1714
Biological decontamination using pulsed filamentary microplasma jet
Pothiraja, R. and Lackmann, J.-W. and Keil, G. and Bibinov, N. and Awakowicz, P.
NATO SCIENCE FOR PEACE AND SECURITY SERIES A: CHEMISTRY AND BIOLOGY. Volume: (2012) - 2012 • 1713
Superconducting and martensitic transitions of V3Si and Nb 3Sn under high pressure
Tanaka, S. and Handoko and Miyake, A. and Kagayama, T. and Shimizu, K. and Böhmer, Anna.E. and Burger, P. and Hardy, F. and Meingast, C. and Tsutsumi, H. and Onuki, Y.
JOURNAL OF THE PHYSICAL SOCIETY OF JAPAN. Volume: 81 (2012) - 2012 • 1712
In Situ TEM Observations of Defect Interactions in Mo-Alloy Nanofibers
Chisholm, C. and Bei, H. and George, E.P. and Minor, A.M.
MICROSCOPY AND MICROANALYSIS. Volume: 18 (2012) - 2012 • 1711
A light-driven turbine-like micro-rotor and study on its light-to-mechanical power conversion efficiency
Lin, X.-F. and Hu, G.-Q. and Chen, Q.-D. and Niu, L.-G. and Li, Q.-S. and Ostendorf, A. and Sun, H.-B.
APPLIED PHYSICS LETTERS. Volume: 101 (2012) - 2012 • 1710
Embedded argon as a tool for sampling local structure in thin plasma deposited aluminum oxide films
Prenzel, M. and de los Arcos, T. and Kortmann, A. and Winter, J. and von Keudell, A.
JOURNAL OF APPLIED PHYSICS. Volume: 112 (2012) - 2012 • 1709
On the limits of the interfacial yield model for fragmentation testing of brittle films on polymer substrates
Taylor, A.A. and Cordill, M.J. and Dehm, G.
PHILOSOPHICAL MAGAZINE. Volume: 92 (2012) - 2012 • 1708
Dependence of the yield stress of Fe3Al on heat treatment
Hasemann, G. and Schneibel, J.H. and George, E.P.
INTERMETALLICS. Volume: 21 (2012) - 2012 • 1707
Superfluid hydrodynamic in fractal dimension space
Tayurskii, D.A. and Lysogorskiy, Yu.V.
JOURNAL OF PHYSICS: CONFERENCE SERIES. Volume: 394 (2012) - 2012 • 1706
DFT-based tight-binding modeling of iron-carbon
Hatcher, N. and Madsen, G.K.H. and Drautz, R.
PHYSICAL REVIEW B - CONDENSED MATTER AND MATERIALS PHYSICS. Volume: 86 (2012) - 2012 • 1705
Absolute acidity of clay edge sites from ab-initio simulations
Tazi, S. and Rotenberg, B. and Salanne, M. and Sprik, M. and Sulpizi, M.
GEOCHIMICA ET COSMOCHIMICA ACTA. Volume: 94 (2012) - 2012 • 1704
A note on asymptotic uniform confidence bands in a multivariate statistical deconvolution problem
Proksch, K. and Bissantz, N. and Dette, H.
AIP CONFERENCE PROCEEDINGS. Volume: 1479 (2012) - 2012 • 1703
Ab initio study of the interaction of H with substitutional solute atoms in α-Fe: Trends across the transition-metal series
Psiachos, D. and Hammerschmidt, T. and Drautz, R.
COMPUTATIONAL MATERIALS SCIENCE. Volume: 65 (2012) - 2012 • 1702
Simulation of dislocation penetration through a general low-angle grain boundary
Liu, B. and Eisenlohr, P. and Roters, F. and Raabe, D.
ACTA MATERIALIA. Volume: 60 (2012) - 2012 • 1701
Friction-stir dissimilar welding of aluminium alloy to high strength steels: Mechanical properties and their relation to microstructure
Coelho, R.S. and Kostka, A. and dos Santos, J.F. and Kaysser-Pyzalla, A.
MATERIALS SCIENCE AND ENGINEERING A. Volume: 556 (2012) - 2012 • 1700
Structure-property relations and thermodynamic properties of monoclinic petalite, LiAlSi 4O 10
Haussühl, E. and Schreuer, J. and Winkler, B. and Haussühl, S. and Bayarjargal, L. and Milman, V.
JOURNAL OF PHYSICS CONDENSED MATTER. Volume: 24 (2012) - 2012 • 1699
Exploring the p-n junction region in Cu(In,Ga)Se 2 thin-film solar cells at the nanometer-scale
Cojocaru-Mirédin, O. and Choi, P. and Wuerz, R. and Raabe, D.
APPLIED PHYSICS LETTERS. Volume: 101 (2012) - 2012 • 1698
High-performance electrocatalysis on palladium aerogels
Liu, W. and Herrmann, A.-K. and Geiger, D. and Borchardt, L. and Simon, F. and Kaskel, S. and Gaponik, N. and Eychmüller, A.
ANGEWANDTE CHEMIE - INTERNATIONAL EDITION. Volume: 51 (2012) - 2012 • 1697
Influences of deformation strain, strain rate and cooling rate on the Burgers orientation relationship and variants morphology during β→α phase transformation in a near α titanium alloy
He, D. and Zhu, J.C. and Zaefferer, S. and Raabe, D. and Liu, Y. and Lai, Z.L. and Yang, X.W.
MATERIALS SCIENCE AND ENGINEERING A. Volume: 549 (2012) - 2012 • 1696
Microsegregation and secondary phase formation during directional solidification of the single-crystal Ni-based superalloy LEK94
Lopez-Galilea, I. and Huth, S. and Fries, S.G. and Warnken, N. and Steinbach, I. and Theisen, W.
METALLURGICAL AND MATERIALS TRANSACTIONS A: PHYSICAL METALLURGY AND MATERIALS SCIENCE. Volume: 43 (2012) - 2012 • 1695
A few-electron quadruple quantum dot in a closed loop
Thalineau, R. and Hermelin, S. and Wieck, A.D. and Bäuerle, C. and Saminadayar, L. and Meunier, T.
APPLIED PHYSICS LETTERS. Volume: 101 (2012) - 2012 • 1694
Improved single- and multi-contact life-time testing of dental restorative materials using key characteristics of the human masticatory system and a force/position-controlled robotic dental wear simulator
Raabe, D. and Harrison, A. and Ireland, A. and Alemzadeh, K. and Sandy, J. and Dogramadzi, S. and Melhuish, C. and Burgess, S.
BIOINSPIRATION & BIOMIMETICS. Volume: 7 (2012) - 2012 • 1693
Robust mechanical performance of chromium-coated polyethylene terephthalate over a broad range of conditions
Cordill, M.J. and Taylor, A.A. and Berger, J. and Schmidegg, K. and Dehm, G.
PHILOSOPHICAL MAGAZINE. Volume: 92 (2012) - 2012 • 1692
Functional plasma polymers deposited in capacitively and inductively coupled plasmas
Hegemann, D. and Korner, E. and Chen, S. and Benedikt, J. and von Keudell, A.
APPLIED PHYSICS LETTERS. Volume: 100 (2012) - 2012 • 1691
Conditioning of surfaces by macromolecules and its implication for the settlement of zoospores of the green alga Ulva linza
Thome, I. and Pettitt, M.E. and Callow, M.E. and Callow, J.A. and Grunze, M. and Rosenhahn, A.
BIOFOULING. Volume: 28 (2012) - 2012 • 1690
Structural properties of metal-organic frameworks within the density-functional based tight-binding method
Lukose, B. and Supronowicz, B. and St Petkov, P. and Frenzel, J. and Kuc, A. B. and Seifert, G. and Vayssilov, G. N. and Heine, T.
PHYSICA STATUS SOLIDI B-BASIC SOLID STATE PHYSICS. Volume: 249 (2012) - 2012 • 1689
Quantitative characterization of a dielectric barrier discharge in air applying non-calibrated spectrometer, current measurement and numerical simulation
Rajasekaran, P. and Bibinov, N. and Awakowicz, P.
MEASUREMENT SCIENCE AND TECHNOLOGY. Volume: 23 (2012) - 2012 • 1688
Highly sensitive and selective hydrogen single-nanowire nanosensor
Lupan, O. and Chow, L. and Pauporté, T. and Ono, L.K. and Roldan Cuenya, B. and Chai, G.
SENSORS AND ACTUATORS, B: CHEMICAL. Volume: 173 (2012) - 2012 • 1687
Size-controlled synthesis and microstructure investigation of Co 3O 4 nanoparticles for low-temperature CO oxidation
Dangwal Pandey, A. and Jia, C. and Schmidt, W. and Leoni, M. and Schwickardi, M. and Schüth, F. and Weidenthaler, C.
JOURNAL OF PHYSICAL CHEMISTRY C. Volume: 116 (2012) - 2012 • 1686
Enhanced performance of surface-modified TiO2 photocatalysts prepared via a visible-light photosynthetic route
Ramakrishnan, A. and Neubert, S. and Mei, B. and Strunk, J. and Wang, L. and Bledowski, M. and Muhler, M. and Beranek, R.
CHEMICAL COMMUNICATIONS. Volume: 48 (2012) - 2012 • 1685
Copper nanoparticles stabilized on nitrogen-doped carbon nanotubes as efficient and recyclable catalysts for alkyne/aldehyde/cyclic amine A 3-type coupling reactions
Ramu, V.G. and Bordoloi, A. and Nagaiah, T.C. and Schuhmann, W. and Muhler, M. and Cabrele, C.
APPLIED CATALYSIS A: GENERAL. Volume: 431-432 (2012) - 2012 • 1684
3-D phase-field simulation of grain growth: Topological analysis versus mean-field approximations
Darvishi Kamachali, R. and Steinbach, I.
ACTA MATERIALIA. Volume: 60 (2012) - 2012 • 1683
Directing the breathing behavior of pillared-layered metal-organic frameworks via a systematic library of functionalized linkers bearing flexible substituents
Henke, S. and Schneemann, A. and Wütscher, A. and Fischer, R.A.
JOURNAL OF THE AMERICAN CHEMICAL SOCIETY. Volume: 134 (2012) - 2012 • 1682
Control of magnetic, nonmagnetic, and superconducting states in annealed Ca(Fe 1-xCo x) 2As 2
Ran, S. and Bud'Ko, S.L. and Straszheim, W.E. and Soh, J. and Kim, M.G. and Kreyssig, A. and Goldman, A.I. and Canfield, P.C.
PHYSICAL REVIEW B - CONDENSED MATTER AND MATERIALS PHYSICS. Volume: 85 (2012) - 2012 • 1681
Magnetically polarized Ir dopant atoms in superconducting Ba(Fe 1-xIr x) 2As 2
Dean, M.P.M. and Kim, M.G. and Kreyssig, A. and Kim, J.W. and Liu, X. and Ryan, P.J. and Thaler, A. and Bud'ko, S.L. and Strassheim, W. and Canfield, P.C. and Hill, J.P. and Goldman, A.I.
PHYSICAL REVIEW B - CONDENSED MATTER AND MATERIALS PHYSICS. Volume: 85 (2012) - 2012 • 1680
Zinc-1,4-benzenedicarboxylate-bipyridine frameworks - Linker functionalization impacts network topology during solvothermal synthesis
Henke, S. and Schneemann, A. and Kapoor, S. and Winter, R. and Fischer, R.A.
JOURNAL OF MATERIALS CHEMISTRY. Volume: 22 (2012) - 2012 • 1679
A new approach to the stability of discrete descriptor time delay systems in the sense of non-Lyapunov delay independent conditions
Debeljkovic, D.Lj. and Buzurovic, I.M. and Nestorovic, T. and Popov, D.
PROCEEDINGS OF THE 2012 24TH CHINESE CONTROL AND DECISION CONFERENCE, CCDC 2012. Volume: (2012) - 2012 • 1678
Advanced buckyball joints: Synthesis, complex formation and computational simulations of centrohexaindane-extended tribenzotriquinacene receptors for C 60 fullerene
Henne, S. and Bredenkötter, B. and Dehghan Baghi, A.A. and Schmid, R. and Volkmer, D.
DALTON TRANSACTIONS. Volume: 41 (2012) - 2012 • 1677
LMI approach to non-Lyapunov stability of discrete descriptor time delay systems
Debeljkovic, D.Lj. and Buzurovic, I.M. and Stojanovic, S.B. and Nestorovic, T.
INES 2012 - IEEE 16TH INTERNATIONAL CONFERENCE ON INTELLIGENT ENGINEERING SYSTEMS, PROCEEDINGS. Volume: (2012) - 2012 • 1676
Study of energy fluctuation effect on the statistical mechanics of equilibrium systems
Lysogorskiy, Yu.V. and Wang, Q.A. and Tayurskii, D.A.
JOURNAL OF PHYSICS: CONFERENCE SERIES. Volume: 394 (2012) - 2012 • 1675
Ab initio studying of topological insulator Bi2Se3 under the stress
Lysogorskiy, Y.V. and Kijamov, A.G. and Nedopekin, O.V. and Tayurskii, D.A.
JOURNAL OF PHYSICS: CONFERENCE SERIES. Volume: 394 (2012) - 2012 • 1674
Ab-initio investigation of spin states of sodium cobaltate Na 2/3CoO2
Lysogorskiy, Y.V. and Nedopekin, O.V. and Krivenko, S.A. and Minisini, B. and Tayurskii, D.A.
JOURNAL OF PHYSICS: CONFERENCE SERIES. Volume: 394 (2012) - 2012 • 1673
Thin-film Cu-Pt(111) near-surface alloys: Active electrocatalysts for the oxygen reduction reaction
Henry, J.B. and Maljusch, A. and Huang, M. and Schuhmann, W. and Bondarenko, A.S.
ACS CATALYSIS. Volume: 2 (2012) - 2012 • 1672
Photoisomerization for a molecular switch in contact with a surface
Henzl, J. and Puschnig, P. and Ambrosch-Draxl, C. and Schaate, A. and Ufer, B. and Behrens, P. and Morgenstern, K.
PHYSICAL REVIEW B - CONDENSED MATTER AND MATERIALS PHYSICS. Volume: 85 (2012) - 2012 • 1671
Nanomechanical testing in materials research and development III
Dehm, G. and Pharr, G.M. and Michler, J.
PHILOSOPHICAL MAGAZINE. Volume: 92 (2012) - 2012 • 1670
Tensile deformation characteristics of bulk ultrafine-grained austenitic stainless steel produced by thermal cycling
Ravi Kumar, B. and Raabe, D.
SCRIPTA MATERIALIA. Volume: 66 (2012) - 2012 • 1669
In-Situ Electron Microscopy: Applications in Physics, Chemistry and Meterials Science
Dehm, G. and Howe, J.M. and Zweck, J.
IN-SITU ELECTRON MICROSCOPY: APPLICATIONS IN PHYSICS, CHEMISTRY AND MATERIALS SCIENCE. Volume: (2012) - 2012 • 1668
Recrystallization during Annealing of a Cold Rolled Lean Duplex Stainless Steel
Herrera, C. and Ponge, D. and Raabe, D.
RECRYSTALLIZATION AND GRAIN GROWTH IV. Volume: 715-716 (2012) - 2012 • 1667
Annealing effect on structural and magnetic properties of Cu 2MnAL heusler alloy films
Topkaya, R. and Yilgin, R. and Kazan, S. and Akdoǧan, N. and Obaida., M. and Inam, H. and Westerholt, K.
JOURNAL OF SUPERCONDUCTIVITY AND NOVEL MAGNETISM. Volume: 25 (2012) - 2012 • 1666
In-Situ TEM Straining Experiments: Recent Progress in Stages and Small-Scale Mechanics
Dehm, G. and Legros, M. and Kiener, D.
IN-SITU ELECTRON MICROSCOPY: APPLICATIONS IN PHYSICS, CHEMISTRY AND MATERIALS SCIENCE. Volume: (2012) - 2012 • 1665
Mechanisms of crazing in glassy polymers revealed by molecular dynamics simulations
Mahajan, D.K. and Hartmaier, A.
PHYSICAL REVIEW E - STATISTICAL, NONLINEAR, AND SOFT MATTER PHYSICS. Volume: 86 (2012) - 2012 • 1664
Aqueous redox chemistry and the electronic band structure of liquid water
Adriaanse, C. and Cheng, J. and Chau, V. and Sulpizi, M. and Vandevondele, J. and Sprik, M.
JOURNAL OF PHYSICAL CHEMISTRY LETTERS. Volume: 3 (2012) - 2012 • 1663
Quantitative Approaches for in situ SEM and TEM Deformation Studies
Dehm, G. and Kiener, D. and Motz, C. and Smolka, M. and Pippan, R.
MICROSCOPY AND MICROANALYSIS. Volume: 18 (2012) - 2012 • 1662
Elastic properties of face-centred cubic Fe-Mn-C studied by nanoindentation and ab initio calculations
Reeh, S. and Music, D. and Gebhardt, T. and Kasprzak, M. and Jäpel, T. and Zaefferer, S. and Raabe, D. and Richter, S. and Schwedt, A. and Mayer, J. and Wietbrock, B. and Hirt, G. and Schneider, J.M.
ACTA MATERIALIA. Volume: 60 (2012) - 2012 • 1661
Influence of process parameters on the crystallinity, morphology and composition of tungsten oxide-based thin films grown by metalorganic chemical vapor deposition
De Los Arcos, T. and Cwik, S. and Milanov, A.P. and Gwildies, V. and Parala, H. and Wagner, T. and Birkner, A. and Rogalla, D. and Becker, H.-W. and Winter, J. and Ludwig, Al. and Fischer, R.A. and Devi, A.
THIN SOLID FILMS. Volume: 522 (2012) - 2012 • 1660
Performance of mixed and enhanced finite elements for strain localization in hypoplasticity
Trinh, B.T. and Hackl, K.
INTERNATIONAL JOURNAL FOR NUMERICAL AND ANALYTICAL METHODS IN GEOMECHANICS. Volume: 36 (2012) - 2012 • 1659
Zeros and ratio asymptotics for matrix orthogonal polynomials
Delvaux, S. and Dette, H.
JOURNAL D'ANALYSE MATHEMATIQUE. Volume: 118 (2012) - 2012 • 1658
Electrodeposition of Catechol on Glassy Carbon Electrode and Its Electrocatalytic Activity Toward NADH Oxidation
Maleki, A. and Nematollahi, D. and Clausmeyer, J. and Henig, J. and Plumeré, N. and Schuhmann, W.
ELECTROANALYSIS. Volume: 24 (2012) - 2012 • 1657
Ordered mesoporous materials with MFI structured microporous walls - Synthesis and proof of wall microporosity
Reichinger, M. and Schmidt, W. and Narkhede, V.V. and Zhang, W. and Gies, H. and Grünert, W.
MICROPOROUS AND MESOPOROUS MATERIALS. Volume: 164 (2012) - 2012 • 1656
Advanced scanning transmission stereo electron microscopy of structural and functional engineering materials
Agudo Jácome, L. and Eggeler, G. and Dlouhý, A.
ULTRAMICROSCOPY. Volume: 122 (2012) - 2012 • 1655
Thickness and temperature dependence of exchange bias in Co/CoO bilayers
Demirci, E. and Öztürk, M. and Topkaya, R. and Kazan, S. and Akdoǧan, N. and Obaida, M. and Westerholt, K.
JOURNAL OF SUPERCONDUCTIVITY AND NOVEL MAGNETISM. Volume: 25 (2012) - 2012 • 1654
Three dimensional tracking of exploratory behavior of barnacle cyprids using stereoscopy
Maleschlijski, S. and Sendra, G.H. and Di Fino, A. and Leal-Taixé, L. and Thome, I. and Terfort, A. and Aldred, N. and Grunze, M. and Clare, A.S. and Rosenhahn, B. and Rosenhahn, A.
BIOINTERPHASES. Volume: 7 (2012) - 2012 • 1653
Electrodeposition of separated metallic structures in superimposed magnetic gradient fields
Tschulik, K. and Cierpka, Ch. and Uhlemann, M. and Gebert, A. and Schultz, L.
ECS TRANSACTIONS. Volume: 41 (2012) - 2012 • 1652
Relationship between yield point phenomena and the nanoindentation pop-in behavior of steel
Ahn, T.-H. and Oh, C.-S. and Lee, K. and George, E.P. and Han, H.N.
JOURNAL OF MATERIALS RESEARCH. Volume: 27 (2012) - 2012 • 1651
Settlement behavior of zoospores of Ulva linza during surface selection studied by digital holographic microscopy
Heydt, M. and Pettitt, M.E. and Cao, X. and Callow, M.E. and Callow, J.A. and Grunze, M. and Rosenhahn, A.
BIOINTERPHASES. Volume: 7 (2012) - 2012 • 1650
Combined high resolution Scanning Kelvin probe - Scanning electrochemical microscopy investigations for the visualization of local corrosion processes
Maljusch, A. and Senöz, C. and Rohwerder, M. and Schuhmann, W.
ELECTROCHIMICA ACTA. Volume: 82 (2012) - 2012 • 1649
Clarifying the mechanism of reverse structuring during electrodeposition in magnetic gradient fields
Tschulik, K. and Cierpka, C. and Mutschke, G. and Gebert, A. and Schultz, L. and Uhlemann, M.
ANALYTICAL CHEMISTRY. Volume: 84 (2012) - 2012 • 1648
Plasma sterilization of pharmaceutical products: From basics to production
Denis, B. and Steves, S. and Semmler, E. and Bibinov, N. and Novak, W. and Awakowicz, P.
PLASMA PROCESSES AND POLYMERS. Volume: 9 (2012) - 2012 • 1647
A quick method for the preparation of Pt(111)-like thin films
Maljusch, A. and Henry, J.B. and Schuhmann, W. and Bondarenko, A.S.
ELECTROCHEMISTRY COMMUNICATIONS. Volume: 16 (2012) - 2012 • 1646
Competition between stripe and checkerboard magnetic instabilities in Mn-doped BaFe 2As 2
Tucker, G.S. and Pratt, D.K. and Kim, M.G. and Ran, S. and Thaler, A. and Granroth, G.E. and Marty, K. and Tian, W. and Zarestky, J.L. and Lumsden, M.D. and Bud'Ko, S.L. and Canfield, P.C. and Kreyssig, A. and Goldman, A.I. and McQueeney, R.J.
PHYSICAL REVIEW B - CONDENSED MATTER AND MATERIALS PHYSICS. Volume: 86 (2012) - 2012 • 1645
Optimal designs for quantile regression models
Dette, H. and Trampisch, M.
JOURNAL OF THE AMERICAN STATISTICAL ASSOCIATION. Volume: 107 (2012) - 2012 • 1644
Ab initio-based prediction of phase diagrams: Application to magnetic shape memory alloys
Hickel, T. and Uijttewaal, M. and Al-Zubi, A. and Dutta, B. and Grabowski, B. and Neugebauer, J.
ADVANCED ENGINEERING MATERIALS. Volume: 14 (2012) - 2012 • 1643
Component interactions after long-term operation of an SOFC stack with LSM cathode
Malzbender, J. and Batfalsky, P. and Vaßen, R. and Shemet, V. and Tietz, F.
JOURNAL OF POWER SOURCES. Volume: 201 (2012) - 2012 • 1642
Ordered Mesoporous Materials as Catalysts
Tüysüz, H. and Schüth, F.
ADVANCES IN CATALYSIS. Volume: 55 (2012) - 2012 • 1641
Distributions on unbounded moment spaces and random moment sequences
Dette, H. and Nagel, J.
ANNALS OF PROBABILITY. Volume: 40 (2012) - 2012 • 1640
Advancing density functional theory to finite temperatures: Methods and applications in steel design
Hickel, T. and Grabowski, B. and Körmann, F. and Neugebauer, J.
JOURNAL OF PHYSICS CONDENSED MATTER. Volume: 24 (2012) - 2012 • 1639
Pseudoelastic deformation and size effects during in situ transmission electron microscopy tensile testing of NiTi
Manchuraju, S. and Kroeger, A. and Somsen, C. and Dlouhy, A. and Eggeler, G. and Sarosi, P.M. and Anderson, P.M. and Mills, M.J.
ACTA MATERIALIA. Volume: 60 (2012) - 2012 • 1638
A crystal structure analysis and magnetic investigation on highly ordered mesoporous Cr 2O 3
Tüysüz, H. and Weidenthaler, C. and Grewe, T. and Salabaş, E.L. and Benitez Romero, M.J. and Schüth, F.
INORGANIC CHEMISTRY. Volume: 51 (2012) - 2012 • 1637
Testing for a constant coefficient of variation in nonparametric regression by empirical processes
Dette, H. and Marchlewski, M. and Wagener, J.
ANNALS OF THE INSTITUTE OF STATISTICAL MATHEMATICS. Volume: 64 (2012) - 2012 • 1636
Influence of processing and heat treatment on corrosion resistance and properties of high alloyed steel coatings
Hill, H. and Weber, S. and Raab, U. and Theisen, W. and Wagner, L.
JOURNAL OF THERMAL SPRAY TECHNOLOGY. Volume: 21 (2012) - 2012 • 1635
Heterogeneous shear in hard sphere glasses
Mandal, S. and Gross, M. and Raabe, D. and Varnik, F.
PHYSICAL REVIEW LETTERS. Volume: 108 (2012) - 2012 • 1634
Synthesis of hard magnetic ordered mesoporous Co3O 4/CoFe2O4 nanocomposites
Tüysüz, H. and Salabaş, E.L. and Bill, E. and Bongard, H. and Spliethoff, B. and Lehmann, C.W. and Schüth, F.
CHEMISTRY OF MATERIALS. Volume: 24 (2012) - 2012 • 1633
Scale Checks in Censored Regression
Dette, H. and Heuchenne, C.
SCANDINAVIAN JOURNAL OF STATISTICS. Volume: 39 (2012) - 2012 • 1632
Correlation of processing route and heat treatment with the abrasive wear resistance of a plastic mold steel
Hill, H. and Kunze, M. and Heet, C. and Petsch, A. and Weber, S. and Reiter, R. and Giese, P. and Theisen, W. and Wielage, B. and Wesling, V.
MATERIALWISSENSCHAFT UND WERKSTOFFTECHNIK. Volume: 43 (2012) - 2012 • 1631
Modification of pineapple leaf fibers and graft copolymerization of acrylonitrile onto modified fibers
Maniruzzaman, M. and Rahman, M.A. and Gafur, M.A. and Fabritius, H. and Raabe, D.
JOURNAL OF COMPOSITE MATERIALS. Volume: 46 (2012) - 2012 • 1630
A strategy for the synthesis of mesostructured metal oxides with lower oxidation states
Tüysüz, H. and Weidenthaler, C. and Schüth, F.
CHEMISTRY - A EUROPEAN JOURNAL. Volume: 18 (2012) - 2012 • 1629
Optimal designs for composed models in pharmacokinetic-pharmacodynamic experiments
Dette, H. and Pepelyshev, A. and Wong, W.K.
JOURNAL OF PHARMACOKINETICS AND PHARMACODYNAMICS. Volume: 39 (2012) - 2012 • 1628
Do cement nanotubes exist?
Manzano, H. and Enyashin, A.N. and Dolado, J.S. and Ayuela, A. and Frenzel, J. and Seifert, G.
ADVANCED MATERIALS. Volume: 24 (2012) - 2012 • 1627
Microstructural evolution of a Ni-based superalloy (617B) at 700 °c studied by electron microscopy and atom probe tomography
Tytko, D. and Choi, P.-P. and Klöwer, J. and Kostka, A. and Inden, G. and Raabe, D.
ACTA MATERIALIA. Volume: 60 (2012) - 2012 • 1626
Matrix Measures, Random Moments, and Gaussian Ensembles
Dette, H. and Nagel, J.
JOURNAL OF THEORETICAL PROBABILITY. Volume: 25 (2012) - 2012 • 1625
The Role of Oxygen and Surface Reactions in the Deposition of Silicon Oxide like Films from HMDSO at Atmospheric Pressure
Reuter, R. and Rugner, K. and Ellerweg, D. and de los Arcos, T. and von Keudell, A. and Benedikt, J.
PLASMA PROCESSES AND POLYMERS. Volume: 9 (2012) - 2012 • 1624
A note on testing hypotheses for stationary processes in the frequency domain
Dette, H. and Hildebrandt, T.
JOURNAL OF MULTIVARIATE ANALYSIS. Volume: 104 (2012) - 2012 • 1623
Atomistic simulation study of 〈1 1 0〉 dislocations in strontium titanate
Hirel, P. and Mrovec, M. and Elsässer, C.
ACTA MATERIALIA. Volume: 60 (2012) - 2012 • 1622
T-optimal designs for discrimination between two polynomial models
Dette, H. and Melas, V.B. and Shpilev, P.
ANNALS OF STATISTICS. Volume: 40 (2012) - 2012 • 1621
Rare-earth substituted HfO2 thin films grown by metalorganic chemical vapor deposition
Devi, A. and Cwik, S. and Xu, K. and Milanov, A.P. and Noei, H. and Wang, Y. and Barreca, D. and Meijer, J. and Rogalla, D. and Kahn, D. and Cross, R. and Parala, H. and Paul, S.
THIN SOLID FILMS. Volume: 520 (2012) - 2012 • 1620
Experimental and numerical investigation on the discharge of wood pellets from a hopper with the discrete element method
Höhner, D. and Wirtz, S. and Scherer, V.
ASME INTERNATIONAL MECHANICAL ENGINEERING CONGRESS AND EXPOSITION, PROCEEDINGS (IMECE). Volume: 1 (2012) - 2012 • 1619
RUB-55, a new hydrous layer silicate with silicate layers known as motives of the sodalite and octadecasil frameworks: Synthesis and crystal structure
Marler, B. and Grünewald-Lüke, A. and Grabowski, S. and Gies, H.
ZEITSCHRIFT FUR KRISTALLOGRAPHIE. Volume: 227 (2012) - 2012 • 1618
A numerical study on the influence of particle shape on hopper discharge within the polyhedral and multi-sphere discrete element method
Höhner, D. and Wirtz, S. and Scherer, V.
POWDER TECHNOLOGY. Volume: 226 (2012) - 2012 • 1617
Hydrous layer silicates as precursors for zeolites obtained through topotactic condensation: A review
Marler, B. and Gies, H.
EUROPEAN JOURNAL OF MINERALOGY. Volume: 24 (2012) - 2012 • 1616
The influence of particle size and spacing on the fragmentation of nanocomposite anodes for Li batteries
Dimitrijevic, B.J. and Aifantis, K.E. and Hackl, K.
JOURNAL OF POWER SOURCES. Volume: 206 (2012) - 2012 • 1615
A flexible, plane-wave based multiband k ·p model
Marquardt, O. and Schulz, S. and Freysoldt, C. and Boeck, S. and Hickel, T. and O'Reilly, E.P. and Neugebauer, J.
OPTICAL AND QUANTUM ELECTRONICS. Volume: 44 (2012) - 2012 • 1614
A micromechanical damage simulation of dual phase steels using XFEM
Vajragupta, N. and Uthaisangsuk, V. and Schmaling, B. and Münstermann, S. and Hartmaier, A. and Bleck, W.
COMPUTATIONAL MATERIALS SCIENCE. Volume: 54 (2012) - 2012 • 1613
Influence of post-treatment on the microstructural evolution of thermally sprayed Fe-base MMC containing TiC and Cr 3C 2
Röttger, A. and Weber, S.L. and Theisen, W.
SURFACE AND COATINGS TECHNOLOGY. Volume: 209 (2012) - 2012 • 1612
65th birthday of Professor Wolfbeis
Valentin, K. and Diaz-Garcia, M. E. and Stulik, K. and Gluckwunsch, H. and Fabry, L. and Trojanowicz, M. and Bakker, E. and Buchberger, W. and Niessner, R. and Broekaert, J. A. C. and Schuhmann, W. and da Costa-Lima, J. L. F. and Soukka, T. and Karst, U. and Lev, O.
MICROCHIMICA ACTA. Volume: 178 (2012) - 2012 • 1611
NADH oxidation using modified electrodes based on lactate and glucose dehydrogenase entrapped between an electrocatalyst film and redox catalyst-modified polymers
Al-Jawadi, E. and Pöller, S. and Haddad, R. and Schuhmann, W.
MICROCHIMICA ACTA. Volume: 177 (2012) - 2012 • 1610
Magnetic field dependence of the spin relaxation length in spin light-emitting diodes
Höpfner, H. and Fritsche, C. and Ludwig, As. and Ludwig, Ar. and Stromberg, F. and Wende, H. and Keune, W. and Reuter, D. and Wieck, A.D. and Gerhardt, N.C. and Hofmann, M.R.
APPLIED PHYSICS LETTERS. Volume: 101 (2012) - 2012 • 1609
Supersolidus liquid-phase sintering of ultrahigh-boron high-carbon steels for wear-protection applications
Röttger, A. and Weber, S. and Theisen, W.
MATERIALS SCIENCE AND ENGINEERING A. Volume: 532 (2012) - 2012 • 1608
Room temperature spin relaxation in quantum dot based spin-optoelectronic devices
Höpfner, H. and Li, M. and Ludwig, Ar. and Ludwig, As. and Stromberg, F. and Wende, H. and Keune, W. and Reuter, D. and Wieck, A.D. and Gerhardt, N.C. and Hofmann, M.R.
PROCEEDINGS OF SPIE - THE INTERNATIONAL SOCIETY FOR OPTICAL ENGINEERING. Volume: 8260 (2012) - 2012 • 1607
Size-dependent evolution of the atomic vibrational density of states and thermodynamic properties of isolated Fe nanoparticles
Roldan Cuenya, B. and Ono, L.K. and Croy, J.R. and Paredis, K. and Kara, A. and Heinrich, H. and Zhao, J. and Alp, E.E. and Delariva, A.T. and Datye, A. and Stach, E.A. and Keune, W.
PHYSICAL REVIEW B - CONDENSED MATTER AND MATERIALS PHYSICS. Volume: 86 (2012) - 2012 • 1606
Adsorption of hydrocarbons in metal-organic frameworks: A force field benchmark on the example of benzene in metal-organic framework 5
Amirjalayer, S. and Schmid, R.
JOURNAL OF PHYSICAL CHEMISTRY C. Volume: 116 (2012) - 2012 • 1605
Surface sensing and settlement strategies of marine biofouling organisms
Rosenhahn, A. and Sendra, G.H.
BIOINTERPHASES. Volume: 7 (2012) - 2012 • 1604
Flow and Rheological Response of Model Glasses
Varnik, F. and Mandal, S. and Gross, M.
TRANSACTIONS OF THE INDIAN CERAMIC SOCIETY. Volume: 71 (2012) - 2012 • 1603
Prediction of structure and properties of boron-based covalent organic frameworks by a first-principles derived force field
Amirjalayer, S. and Snurr, R.Q. and Schmid, R.
JOURNAL OF PHYSICAL CHEMISTRY C. Volume: 116 (2012) - 2012 • 1602
Distance-dependent enhancement of a measurement based channel model for large concert halls
Dortmund, S. and Sczyslo, S. and Rolfes, I.
IEEE ANTENNAS AND PROPAGATION SOCIETY, AP-S INTERNATIONAL SYMPOSIUM (DIGEST). Volume: (2012) - 2012 • 1601
Trends in the elastic response of binary early transition metal nitrides
Holec, D. and Friák, M. and Neugebauer, J. and Mayrhofer, P.H.
PHYSICAL REVIEW B - CONDENSED MATTER AND MATERIALS PHYSICS. Volume: 85 (2012) - 2012 • 1600
DAMASK: The Düsseldorf advanced material simulation kit for studying crystal plasticity using an fe based or a spectral numerical solver
Roters, F. and Eisenlohr, P. and Kords, C. and Tjahjanto, D.D. and Diehl, M. and Raabe, D.
PROCEDIA IUTAM. Volume: 3 (2012) - 2012 • 1599
Hole spin precession in a (In,Ga)As quantum dot ensemble: From resonant spin amplification to spin mode locking
Varwig, S. and Schwan, A. and Barmscheid, D. and Müller, C. and Greilich, A. and Yugova, I.A. and Yakovlev, D.R. and Reuter, D. and Wieck, A.D. and Bayer, M.
PHYSICAL REVIEW B - CONDENSED MATTER AND MATERIALS PHYSICS. Volume: 86 (2012) - 2012 • 1598
Crystal growth and physical properties of SrCu2As2, SrCu2Sb2, and BaCu2Sb2
Anand, V.K. and Perera, P.K. and Pandey, A. and Goetsch, R.J. and Kreyssig, A. and Johnston, D.C.
PHYSICAL REVIEW B - CONDENSED MATTER AND MATERIALS PHYSICS. Volume: 85 (2012) - 2012 • 1597
Efficient Algorithms for Optimal Designs with Correlated Observations in Pharmacokinetics and Dose-Finding Studies
Holland-Letz, T. and Dette, H. and Renard, D.
BIOMETRICS. Volume: 68 (2012) - 2012 • 1596
Processing of Ceramics by Plasma Spraying
Vaßen, R.
CERAMICS AND COMPOSITES PROCESSING METHODS. Volume: (2012) - 2012 • 1595
Experimental analysis of the particle oscillations in acoustic levitation
Andrade, M.A.B. and Buiochi, F. and Baer, S. and Esen, C. and Ostendorf, A. and Adamowski, J.C.
IEEE INTERNATIONAL ULTRASONICS SYMPOSIUM, IUS. Volume: (2012) - 2012 • 1594
Dynamics of colloidal crystals studied by pump-probe experiments at FLASH
Dronyak, R. and Gulden, J. and Yefanov, O.M. and Singer, A. and Gorniak, T. and Senkbeil, T. and Meijer, J.-M. and Al-Shemmary, A. and Hallmann, J. and Mai, D.D. and Reusch, T. and Dzhigaev, D. and Kurta, R.P. and Lorenz, U. and Petukhov, A.V. and Düsterer, S. and Treusch, R. and Strikhanov, M.N. and Weckert, E. and Mancuso, A.P. and Salditt, T. and Rosenhahn, A. and Vartanyants, I.A.
PHYSICAL REVIEW B - CONDENSED MATTER AND MATERIALS PHYSICS. Volume: 86 (2012) - 2012 • 1593
Enhanced electrocatalytic stability of platinum nanoparticles supported on a nitrogen-doped composite of carbon nanotubes and mesoporous titania under oxygen reduction conditions
Masa, J. and Bordoloi, A. and Muhler, M. and Schuhmann, W. and Xia, W.
CHEMSUSCHEM. Volume: 5 (2012) - 2012 • 1592
HVOF spraying of Fe-based MMC coatings with in situ formation of hard particles by hot isostatic pressing
Röttger, A. and Weber, S.L. and Theisen, W. and Rajasekaran, B. and Vaßen, R.
JOURNAL OF THERMAL SPRAY TECHNOLOGY. Volume: 21 (2012) - 2012 • 1591
Testing and evaluation of thermal-barrier coatings
Vaßen, R. and Kagawa, Y. and Subramanian, R. and Zombo, P. and Zhu, D.
MRS BULLETIN. Volume: 37 (2012) - 2012 • 1590
Diffusion of hydrogen within idealized grains of bcc Fe: A kinetic Monte Carlo study
Du, Y.A. and Rogal, J. and Drautz, R.
PHYSICAL REVIEW B - CONDENSED MATTER AND MATERIALS PHYSICS. Volume: 86 (2012) - 2012 • 1589
Oxygen reduction reaction using N 4-metallomacrocyclic catalysts: Fundamentals on rational catalyst design
Masa, J. and Ozoemena, K. and Schuhmann, W. and Zagal, J.H.
JOURNAL OF PORPHYRINS AND PHTHALOCYANINES. Volume: 16 (2012) - 2012 • 1588
Spin excitations in layered antiferromagnetic metals and superconductors
Rowe, W. and Knolle, J. and Eremin, I. and Hirschfeld, P.J.
PHYSICAL REVIEW B - CONDENSED MATTER AND MATERIALS PHYSICS. Volume: 86 (2012) - 2012 • 1587
Study on the effects of wet ball milling and boron nitride additive on Li-N-H hydrogen storage system
Du, L. and Mauer, G. and Vaßen, R.
ENERGY PROCEDIA. Volume: 29 (2012) - 2012 • 1586
Electrochemical synthesis of metal-polypyrrole composites and their activation for electrocatalytic reduction of oxygen by thermal treatment
Masa, J. and Schilling, T. and Bron, M. and Schuhmann, W.
ELECTROCHIMICA ACTA. Volume: 60 (2012) - 2012 • 1585
Adsorption of anionic-azo dye from aqueous solution by lignocellulose- biomass jute fiber: Equilibrium, kinetics, and thermodynamics study
Roy, A. and Chakraborty, S. and Kundu, S.P. and Adhikari, B. and Majumder, S.B.
INDUSTRIAL AND ENGINEERING CHEMISTRY RESEARCH. Volume: 51 (2012) - 2012 • 1584
Improvement in mechanical properties of jute fibres through mild alkali treatment as demonstrated by utilisation of the Weibull distribution model
Roy, A. and Chakraborty, S. and Kundu, S.P. and Basak, R.K. and Basu Majumder, S. and Adhikari, B.
BIORESOURCE TECHNOLOGY. Volume: 107 (2012) - 2012 • 1583
In situ coarsening study of inverse micelle-prepared Pt nanoparticles supported on γ-Al 2O 3: Pretreatment and environmental effects
Matos, J. and Ono, L.K. and Behafarid, F. and Croy, J.R. and Mostafa, S. and Delariva, A.T. and Datye, A.K. and Frenkel, A.I. and Roldan Cuenya, B.
PHYSICAL CHEMISTRY CHEMICAL PHYSICS. Volume: 14 (2012) - 2012 • 1582
Coulomb attraction during the carpet growth mode of NaCl
Matthaei, F. and Heidorn, S. and Boom, K. and Bertram, C. and Safiei, A. and Henzl, J. and Morgenstern, K.
JOURNAL OF PHYSICS CONDENSED MATTER. Volume: 24 (2012) - 2012 • 1581
Plasma Spray-PVD: Plasma characteristics and impact on coating properties
Mauer, G. and Vaßen, R.
JOURNAL OF PHYSICS: CONFERENCE SERIES. Volume: 406 (2012) - 2012 • 1580
Characteristics of ceramic coatings made by thin film low pressure plasma spraying (LPPS-TF)
Hospach, A. and Mauer, G. and Vaßen, R. and Stöver, D.
JOURNAL OF THERMAL SPRAY TECHNOLOGY. Volume: 21 (2012) - 2012 • 1579
Improving atmospheric plasma spraying of zirconate thermal barrier coatings based on particle diagnostics
Mauer, G. and Sebold, D. and Vaßen, R. and Stöver, D.
JOURNAL OF THERMAL SPRAY TECHNOLOGY. Volume: 21 (2012) - 2012 • 1578
Noncontact technique for measuring the electrical resistivity and magnetic susceptibility of electrostatically levitated materials
Rustan, G.E. and Spyrison, N.S. and Kreyssig, A. and Prozorov, R. and Goldman, A.I.
REVIEW OF SCIENTIFIC INSTRUMENTS. Volume: 83 (2012) - 2012 • 1577
Influence of surface functional groups on lithium ion intercalation of carbon cloth
Ventosa, E. and Xia, W. and Klink, S. and La Mantia, F. and Muhler, M. and Schuhmann, W.
ELECTROCHIMICA ACTA. Volume: 65 (2012) - 2012 • 1576
Probing single-charge fluctuations at a GaAs/AlAs interface using laser spectroscopy on a nearby InGaAs quantum dot
Houel, J. and Kuhlmann, A.V. and Greuter, L. and Xue, F. and Poggio, M. and Warburton, R.J. and Gerardot, B.D. and Dalgarno, P.A. and Badolato, A. and Petroff, P.M. and Ludwig, Ar. and Reuter, D. and Wieck, A.D.
PHYSICAL REVIEW LETTERS. Volume: 108 (2012) - 2012 • 1575
A CFD model for thermal conversion of thermally thick biomass particles
Mehrabian, R. and Zahirovic, S. and Scharler, R. and Obernberger, I. and Kleditzsch, S. and Wirtz, S. and Scherer, V. and Lu, H. and Baxter, L.L.
FUEL PROCESSING TECHNOLOGY. Volume: 95 (2012) - 2012 • 1574
CNTs grown on oxygen-deficient anatase TiO 2-δ as high-rate composite electrode material for lithium ion batteries
Ventosa, E. and Chen, P. and Schuhmann, W. and Xia, W.
ELECTROCHEMISTRY COMMUNICATIONS. Volume: 25 (2012) - 2012 • 1573
Adiabatic temperature increase associated with deformation twinning and dislocation plasticity
Eisenlohr, A. and Gutierrez-Urrutia, I. and Raabe, D.
ACTA MATERIALIA. Volume: 60 (2012) - 2012 • 1572
Temperature dependence of Z-Contrast for InGaN
Mehrtens, T. and Schowalter, M. and Tytko, D. and Choi, P.-P. and Raabe, D. and Hoffmann, L. and Hangleiter, A. and Rosenauer, A.
MICROSCOPY AND MICROANALYSIS. Volume: 18 (2012) - 2012 • 1571
Model checks for the volatility under microstructure noise
Vetter, M. and Dette, H.
BERNOULLI. Volume: 18 (2012) - 2012 • 1570
Tuning the acid/base and structural properties of titanate-loaded mesoporous silica by grafting of zinc oxide
Mei, B. and Becerikli, A. and Pougin, A. and Heeskens, D. and Sinev, I. and Grünert, W. and Muhler, M. and Strunk, J.
JOURNAL OF PHYSICAL CHEMISTRY C. Volume: 116 (2012) - 2012 • 1569
Microfluidic assay to quantify the adhesion of marine bacteria
Arpa-Sancet, M.P. and Christophis, C. and Rosenhahn, A.
BIOINTERPHASES. Volume: 7 (2012) - 2012 • 1568
Electron-nuclei spin coupling in GaAs-Free versus localized electrons
Huang, J. and Chen, Y.S. and Ludwig, Ar. and Reuter, D. and Wieck, A.D. and Bacher, G.
APPLIED PHYSICS LETTERS. Volume: 100 (2012) - 2012 • 1567
Unexpected O and O-3 production in the effluent of He/O-2 microplasma jets emanating into ambient air
Ellerweg, D. and von Keudell, A. and Benedikt, J.
PLASMA SOURCES SCIENCE & TECHNOLOGY. Volume: 21 (2012) - 2012 • 1566
Erratum: High-dimensional neural-network potentials for multicomponent systems: Applications to zinc oxide (Physical Review B - Condensed Matter and Materials Physics (2011) 83 (153101))
Artrith, N. and Morawietz, T. and Behler, J.
PHYSICAL REVIEW B - CONDENSED MATTER AND MATERIALS PHYSICS. Volume: 86 (2012) - 2012 • 1565
Probing electrode/electrolyte interface during intercalation of Cu into Te
Huang, M. and Maljusch, A. and Henry, J.B. and Schuhmann, W. and Bondarenko, A.S.
ELECTROCHEMISTRY COMMUNICATIONS. Volume: 20 (2012) - 2012 • 1564
Stability investigations of electrocatalysts on the nanoscale
Meier, J.C. and Katsounaros, I. and Galeano, C. and Bongard, H.J. and Topalov, A.A. and Kostka, A. and Karschin, A. and Schüth, F. and Mayrhofer, K.J.J.
ENERGY AND ENVIRONMENTAL SCIENCE. Volume: 5 (2012) - 2012 • 1563
High-dimensional neural network potentials for metal surfaces: A prototype study for copper
Artrith, N. and Behler, J.
PHYSICAL REVIEW B - CONDENSED MATTER AND MATERIALS PHYSICS. Volume: 85 (2012) - 2012 • 1562
Towards a detailed in situ characterization of non-stationary electrocatalytic systems
Huang, M. and Henry, J.B. and Berkes, B.B. and Maljusch, A. and Schuhmann, W. and Bondarenko, A.S.
ANALYST. Volume: 137 (2012) - 2012 • 1561
Degradation mechanisms of Pt/C fuel cell catalysts under simulated start-stop conditions
Meier, J.C. and Galeano, C. and Katsounaros, I. and Topalov, A.A. and Kostka, A. and Schüth, F. and Mayrhofer, K.J.J.
ACS CATALYSIS. Volume: 2 (2012) - 2012 • 1560
Solvent-Free catalytic depolymerization of cellulose to water-soluble oligosaccharides
Meine, N. and Rinaldi, R. and Schüth, F.
CHEMSUSCHEM. Volume: 5 (2012) - 2012 • 1559
Thermal expansion and grüneisen parameters of Ba(Fe 1-xCo x) 2As 2: A thermodynamic quest for quantum criticality
Meingast, C. and Hardy, F. and Heid, R. and Adelmann, P. and Böhmer, A. and Burger, P. and Ernst, D. and Fromknecht, R. and Schweiss, P. and Wolf, T.
PHYSICAL REVIEW LETTERS. Volume: 108 (2012) - 2012 • 1558
Classification of antibiotics by neural network analysis of optical resonance data of whispering gallery modes in dielectric microspheres
Saetchnikov, V.A. and Tcherniavskaia, E.A. and Schweiger, G. and Ostendorf, A.
PROCEEDINGS OF SPIE - THE INTERNATIONAL SOCIETY FOR OPTICAL ENGINEERING. Volume: 8424 (2012) - 2012 • 1557
Structure, composition, and mechanical properties of shark teeth
Enax, J. and Prymak, O. and Raabe, D. and Epple, M.
JOURNAL OF STRUCTURAL BIOLOGY. Volume: 178 (2012) - 2012 • 1556
Impact of single basepair mismatches on electron-transfer processes at Fc-PNA·DNA modified gold surfaces
Hüsken, N. and Gȩbala, M. and Battistel, A. and La Mantia, F. and Schuhmann, W. and Metzler-Nolte, N.
CHEMPHYSCHEM. Volume: 13 (2012) - 2012 • 1555
Direct electron transfer of bilirubin oxidase (Myrothecium verrucaria) at an unmodified nanoporous gold biocathode
Salaj-Kosla, U. and Pöller, S. and Beyl, Y. and Scanlon, M.D. and Beloshapkin, S. and Shleev, S. and Schuhmann, W. and Magner, E.
ELECTROCHEMISTRY COMMUNICATIONS. Volume: 16 (2012) - 2012 • 1554
Configurational dependence of the magnetization dynamics in spin valve systems: Influence of spin pumping and domain wall induced coupling
Salikhov, R. and Abrudan, R. and Brüssing, F. and Gross, K. and Luo, C. and Westerholt, K. and Zabel, H. and Radu, F. and Garifullin, I.A.
PHYSICAL REVIEW B - CONDENSED MATTER AND MATERIALS PHYSICS. Volume: 86 (2012) - 2012 • 1553
Bridge estimators and the adaptive lasso under heteroscedasticity
Wagener, J. and Dette, H.
MATHEMATICAL METHODS OF STATISTICS. Volume: 21 (2012) - 2012 • 1552
The Role of Oxygen- and Nitrogen-containing Surface Groups on the Sintering of Iron Nanoparticles on Carbon Nanotubes in Different Atmospheres
Sánchez, M.D. and Chen, P. and Reinecke, T. and Muhler, M. and Xia, W.
CHEMCATCHEM. Volume: 4 (2012) - 2012 • 1551
The quantile process under random censoring
Wagener, J. and Volgushev, S. and Dette, H.
MATHEMATICAL METHODS OF STATISTICS. Volume: 21 (2012) - 2012 • 1550
Continuum simulation of the evolution of dislocation densities during nanoindentation
Engels, P. and Ma, A. and Hartmaier, A.
INTERNATIONAL JOURNAL OF PLASTICITY. Volume: 38 (2012) - 2012 • 1549
Status of solid oxide fuel cell development at forschungszentrum jülich
Menzler, N.H. and Blum, L. and Buchkremer, H.P. and Groß, S.M. and De Haart, L.G.J. and Malzbender, J. and Mücke, R. and Quadakkers, W.J. and Peksen, M. and Peters, R. and Remmel, J. and Steinberger-Wilckens, R. and Tietz, F. and Uhlenbruck, S. and Vaßen, R.
PROCEDIA ENGINEERING. Volume: 44 (2012) - 2012 • 1548
Electrochemical oxidation of size-selected pt nanoparticles studied using in situ high-energy-resolution X-ray absorption spectroscopy
Merte, L.R. and Behafarid, F. and Miller, D.J. and Friebel, D. and Cho, S. and Mbuga, F. and Sokaras, D. and Alonso-Mori, R. and Weng, T.-C. and Nordlund, D. and Nilsson, A. and Roldan Cuenya, B.
ACS CATALYSIS. Volume: 2 (2012) - 2012 • 1547
Grain structure and irreversibility line of a bronze route CuNb reinforced NbNb3Sn multifilamentary wire
Sandim, M.J.R. and Stamopoulos, D. and Aristomenopoulou, E. and Zaefferer, S. and Raabe, D. and Awaji, S. and Watanabe, K.
PHYSICS PROCEDIA. Volume: 36 (2012) - 2012 • 1546
Optical emission spectroscopy as a tool for characterization of technical plasmas in medical applications
Awakowicz, P. and Baldus, S. and Stapelmann, K. and Engelhardt, M. and Bibinov, N. and Denis, B.
PLASMA MEDICINE. Volume: 2 (2012) - 2012 • 1545
Computational simulation of mechanized tunneling as part of an integrated decision support platform
Meschke, G. and Nagel, F. and Stascheit, J.
INTERNATIONAL JOURNAL OF GEOMECHANICS. Volume: 11 (2012) - 2012 • 1544
The relation between ductility and stacking fault energies in Mg and Mg-Y alloys
Sandlöbes, S. and Friák, M. and Zaefferer, S. and Dick, A. and Yi, S. and Letzig, D. and Pei, Z. and Zhu, L.-F. and Neugebauer, J. and Raabe, D.
ACTA MATERIALIA. Volume: 60 (2012) - 2012 • 1543
Dual features of magnetic susceptibility in superconducting cuprates: A comparison to inelastic neutron scattering
Eremin, M.V. and Shigapov, I.M. and Eremin, I.M.
EUROPEAN PHYSICAL JOURNAL B. Volume: 85 (2012) - 2012 • 1542
Electrochemical quartz crystal microbalance study of the Fe-Ga co-deposition
Iselt, D. and Tschulik, K. and Oswald, S. and Pohl, D. and Schultz, L. and Schlörb, H.
JOURNAL OF THE ELECTROCHEMICAL SOCIETY. Volume: 159 (2012) - 2012 • 1541
Solution enthalpy of hydrogen in fourth row elements: Systematic trends derived from first principles
Aydin, U. and Ismer, L. and Hickel, T. and Neugebauer, J.
PHYSICAL REVIEW B - CONDENSED MATTER AND MATERIALS PHYSICS. Volume: 85 (2012) - 2012 • 1540
Microscopic origins of the anomalous melting behavior of sodium under high pressure
Eshet, H. and Khaliullin, R.Z. and Kühne, T.D. and Behler, J. and Parrinello, M.
PHYSICAL REVIEW LETTERS. Volume: 108 (2012) - 2012 • 1539
Precipitation behavior of v and/or cu bearing middle carbon steels
Iwasa, N. and Sakata, N. and Takahashi, J. and Raabe, D. and Takemoto, Y. and Senuma, T.
TETSU-TO-HAGANE/JOURNAL OF THE IRON AND STEEL INSTITUTE OF JAPAN. Volume: 98 (2012) - 2012 • 1538
Molecular dynamics and experimental study of conformation change of poly(N -isopropylacrylamide) hydrogels in mixtures of water and methanol
Walter, J. and Sehrt, J. and Vrabec, J. and Hasse, H.
JOURNAL OF PHYSICAL CHEMISTRY B. Volume: 116 (2012) - 2012 • 1537
Microgradient-heaters as tools for high-throughput experimentation
Meyer, R. and Hamann, S. and Ehmann, M. and Thienhaus, S. and Jaeger, S. and Thiede, T. and Devi, A. and Fischer, R.A. and Ludwig, Al.
ACS COMBINATORIAL SCIENCE. Volume: 14 (2012) - 2012 • 1536
Development of a single-axis ultrasonic levitator and the study of the radial particle oscillations
Baer, S. and Andrade, M.A.B. and Esen, C. and Adamowski, J.C. and Ostendorf, A.
AIP CONFERENCE PROCEEDINGS. Volume: 1433 (2012) - 2012 • 1535
A planar orthomode transducer for broadband applications at 25 GHz using a stepped waveguide technique
Baer, C. and Schulz, C. and Will, B. and Rolfes, I. and Musch, T.
ASIA-PACIFIC MICROWAVE CONFERENCE PROCEEDINGS, APMC. Volume: (2012) - 2012 • 1534
Investigation of early degradation processes at coated metals by AC-scanning electrochemical microscopy
Santana, J.J. and Souto, R.M. and González, S. and Pähler, M. and Schuhmann, W.
ECS TRANSACTIONS. Volume: 41 (2012) - 2012 • 1533
Dynamics of photogenerated holes in TiO2-polyheptazine hybrid photoanodes for visible light-driven water splitting
Wang, L. and Bledowski, M. and Ramakrishnan, A. and König, D. and Ludwig, Al. and Beranek, R.
JOURNAL OF THE ELECTROCHEMICAL SOCIETY. Volume: 159 (2012) - 2012 • 1532
Petrology and tectonic significance of metabasite slivers in the Lesser and Higher Himalayan domains of Sikkim, India
Faak, K. and Chakraborty, S. and Dasgupta, S.
JOURNAL OF METAMORPHIC GEOLOGY. Volume: 30 (2012) - 2012 • 1531
Direct evidence of early blister formation in polymer-coated metals from exposure to chloride-containing electrolytes by alternating-current scanning electrochemical microscopy
Santana, J.J. and Pähler, M. and Souto, R.M. and Schuhmann, W.
ELECTROCHIMICA ACTA. Volume: 77 (2012) - 2012 • 1530
Dehydrogenase-Based Reagentless Biosensors: Electrochemically Assisted Deposition of Sol-Gel Thin Films on Functionalized Carbon Nanotubes
Wang, Z. and Etienne, M. and Pöller, S. and Schuhmann, W. and Kohring, G.-W. and Mamane, V. and Walcarius, A.
ELECTROANALYSIS. Volume: 24 (2012) - 2012 • 1529
Correlation of structure, composition and local mechanical properties in the dorsal carapace of the edible crab cancer pagurus
Fabritius, H.-O. and Karsten, E.S. and Balasundaram, K. and Hild, S. and Huemer, K. and Raabe, D.
ZEITSCHRIFT FUR KRISTALLOGRAPHIE. Volume: 227 (2012) - 2012 • 1528
Decomposition of Ba(Mg 1/3Ta 2/3)O 3 perovskite during atmospheric plasma spraying
Jarligo, M.O. and Mauer, G. and Sebold, D. and Mack, D.E. and Vaßen, R. and Stöver, D.
SURFACE AND COATINGS TECHNOLOGY. Volume: 206 (2012) - 2012 • 1527
Hydrogen environment embrittlement of stable austenitic steels
Michler, T. and San Marchi, C. and Naumann, J. and Weber, S. and Martin, M.
INTERNATIONAL JOURNAL OF HYDROGEN ENERGY. Volume: 37 (2012) - 2012 • 1526
Investigation of copper corrosion inhibition with frequency-dependent alternating-current scanning electrochemical microscopy
Santana, J.J. and Pähler, M. and Schuhmann, W. and Souto, R.M.
CHEMPLUSCHEM. Volume: 77 (2012) - 2012 • 1525
Annealing behavior of nano-crystalline austenitic SUS316L produced by HPT
Wang, H. and Shuro, I. and Umemoto, M. and Ho-Hung, K. and Todaka, Y.
MATERIALS SCIENCE AND ENGINEERING A. Volume: 556 (2012) - 2012 • 1524
Caloric effects in ferroic materials: New concepts for cooling
Fähler, S. and Rößler, U.K. and Kastner, O. and Eckert, J. and Eggeler, G. and Emmerich, H. and Entel, P. and Müller, S. and Quandt, E. and Albe, K.
ADVANCED ENGINEERING MATERIALS. Volume: 14 (2012) - 2012 • 1523
Synthesis, characterization, and hydrogen storage capacities of hierarchical porous carbide derived carbon monolith
Wang, J. and Oschatz, M. and Biemelt, T. and Borchardt, L. and Senkovska, I. and Lohe, M.R. and Kaskel, S.
JOURNAL OF MATERIALS CHEMISTRY. Volume: 22 (2012) - 2012 • 1522
Fungi-based porous carbons for CO 2 adsorption and separation
Wang, J. and Heerwig, A. and Lohe, M.R. and Oschatz, M. and Borchardt, L. and Kaskel, S.
JOURNAL OF MATERIALS CHEMISTRY. Volume: 22 (2012) - 2012 • 1521
Transport through side-coupled double quantum dots: From weak to strong interdot coupling
Baines, D.Y. and Meunier, T. and Mailly, D. and Wieck, A.D. and Bäuerle, C. and Saminadayar, L. and Cornaglia, P.S. and Usaj, G. and Balseiro, C.A. and Feinberg, D.
PHYSICAL REVIEW B - CONDENSED MATTER AND MATERIALS PHYSICS. Volume: 85 (2012) - 2012 • 1520
Nearly itinerant ferromagnetism in CaNi 2 and CaNi 3
Jesche, A. and Dennis, K.W. and Kreyssig, A. and Canfield, P.C.
PHYSICAL REVIEW B - CONDENSED MATTER AND MATERIALS PHYSICS. Volume: 85 (2012) - 2012 • 1519
Sc 2O 3, Er 2O 3, and Y 2O 3 thin films by MOCVD from volatile guanidinate class of rare-earth precursors
Milanov, A.P. and Xu, K. and Cwik, S. and Parala, H. and De Los Arcos, T. and Becker, H.-W. and Rogalla, D. and Cross, R. and Paul, S. and Devi, A.
DALTON TRANSACTIONS. Volume: 41 (2012) - 2012 • 1518
Impact of heat treatment on the mechanical properties of AISI 304L austenitic stainless steel in high-pressure hydrogen gas
Weber, S. and Martin, M. and Theisen, W.
JOURNAL OF MATERIALS SCIENCE. Volume: 47 (2012) - 2012 • 1517
Determination of pre-steady-state rate constants on the escherichia coli pyruvate dehydrogenase complex reveals that loop movement controls the rate-limiting step
Balakrishnan, A. and Nemeria, N.S. and Chakraborty, S. and Kakalis, L. and Jordan, F.
JOURNAL OF THE AMERICAN CHEMICAL SOCIETY. Volume: 134 (2012) - 2012 • 1516
Development of lean alloyed austenitic stainless steels with reduced tendency to hydrogen environment embrittlement
Weber, S. and Martin, M. and Theisen, W.
MATERIALS SCIENCE FORUM. Volume: 706-709 (2012) - 2012 • 1515
Solid-state nuclear magnetic resonance studies delineate the role of the protein in activation of both aromatic rings of thiamin
Balakrishnan, A. and Paramasivam, S. and Chakraborty, S. and Polenova, T. and Jordan, F.
JOURNAL OF THE AMERICAN CHEMICAL SOCIETY. Volume: 134 (2012) - 2012 • 1514
Glucose oxidase/horseradish peroxidase Co-immobilized at a CNT-modified graphite electrode: Towards potentially implantable biocathodes
Jia, W. and Jin, C. and Xia, W. and Muhler, M. and Schuhmann, W. and Stoica, L.
CHEMISTRY - A EUROPEAN JOURNAL. Volume: 18 (2012) - 2012 • 1513
Electron-phonon coupling in the conventional superconductor YNi 2B 2C at high phonon energies studied by time-of-flight neutron spectroscopy
Weber, F. and Rosenkranz, S. and Pintschovius, L. and Castellan, J.-P. and Osborn, R. and Reichardt, W. and Heid, R. and Bohnen, K.-P. and Goremychkin, E.A. and Kreyssig, A. and Hradil, K. and Abernathy, D.L.
PHYSICAL REVIEW LETTERS. Volume: 109 (2012) - 2012 • 1512
Intestinal aspartate proteases TiCatD and TiCatD2 of the haematophagous bug Triatoma infestans (Reduviidae): Sequence characterisation, expression pattern and characterisation of proteolytic activity
Balczun, C. and Siemanowski, J. and Pausch, J.K. and Helling, S. and Marcus, K. and Stephan, C. and Meyer, H.E. and Schneider, T. and Cizmowski, C. and Oldenburg, M. and Höhn, S. and Meiser, C.K. and Schuhmann, W. and Schaub, G.A.
INSECT BIOCHEMISTRY AND MOLECULAR BIOLOGY. Volume: 42 (2012) - 2012 • 1511
Orientation dependence of shear banding in face-centered-cubic single crystals
Jia, N. and Eisenlohr, P. and Roters, F. and Raabe, D. and Zhao, X.
ACTA MATERIALIA. Volume: 60 (2012) - 2012 • 1510
Time-resolved measurement of film growth during high-power pulsed magnetron sputtering (HPPMS) of titanium: the rotating shutter concept
Mitschker, F. and Prenzel, M. and Benedikt, J. and von Keudell, A.
JOURNAL OF PHYSICS D-APPLIED PHYSICS. Volume: 45 (2012) - 2012 • 1509
Non-crystallographic shear banding in crystal plasticity FEM simulations: Example of texture evolution in α-brass
Jia, N. and Roters, F. and Eisenlohr, P. and Kords, C. and Raabe, D.
ACTA MATERIALIA. Volume: 60 (2012) - 2012 • 1508
CCS separation techniques - Review on existing technologies and developments [CCS-Abscheidetechniken: Stand der Technik und Entwicklungen]
Scherer, V. and Stolten, D. and Franz, J. and Riensche, E.
CHEMIE-INGENIEUR-TECHNIK. Volume: 84 (2012) - 2012 • 1507
Parallel simulation of patient-specific atherosclerotic arteries for the enhancement of intravascular ultrasound diagnostics
Balzani, D. and Böse, D. and Brands, D. and Erbel, R. and Klawonn, A. and Rheinbach, O. and Schröder, J.
ENGINEERING COMPUTATIONS (SWANSEA, WALES). Volume: 29 (2012) - 2012 • 1506
One-pot synthesis of mesoporous Cu-γ-Al 2O 3 as bifunctional catalyst for direct dimethyl ether synthesis
Jiang, H. and Bongard, H. and Schmidt, W. and Schüth, F.
MICROPOROUS AND MESOPOROUS MATERIALS. Volume: 164 (2012) - 2012 • 1505
Relaxed incremental variational formulation for damage at large strains with application to fiber-reinforced materials and materials with truss-like microstructures
Balzani, D. and Ortiz, M.
INTERNATIONAL JOURNAL FOR NUMERICAL METHODS IN ENGINEERING. Volume: 92 (2012) - 2012 • 1504
An equilibrium thermostatistics of a nonextensive finite system: Canonical distribution and entropy
Jiang, J. and Wang, R. and Lysogorskii, Y. and Zvezdov, D. and Tayurskii, D. and Wang, Q.A.
PHYSICA A: STATISTICAL MECHANICS AND ITS APPLICATIONS. Volume: 391 (2012) - 2012 • 1503
Combined ab initio, experimental, and CALPHAD approach for an improved thermodynamic evaluation of the Mg-Si system
Schick, M. and Hallstedt, B. and Glensk, A. and Grabowski, B. and Hickel, T. and Hampl, M. and Gröbner, J. and Neugebauer, J. and Schmid-Fetzer, R.
CALPHAD: COMPUTER COUPLING OF PHASE DIAGRAMS AND THERMOCHEMISTRY. Volume: 37 (2012) - 2012 • 1502
Co-templating ionothermal synthesis and structure characterization of two new 2D layered aluminophosphates
Wei, Y. and Marler, B. and Zhang, L. and Tian, Z. and Graetsch, H. and Gies, H.
DALTON TRANSACTIONS. Volume: 41 (2012) - 2012 • 1501
Constitutive framework for the modeling of damage in collagenous soft tissues with application to arterial walls
Balzani, D. and Brinkhues, S. and Holzapfel, G.A.
COMPUTER METHODS IN APPLIED MECHANICS AND ENGINEERING. Volume: 213-216 (2012) - 2012 • 1500
On the role of the residual iron growth catalyst in the gasification of multi-walled carbon nanotubes with carbon dioxide
Jin, C. and Xia, W. and Chen, P. and Muhler, M.
CATALYSIS TODAY. Volume: 186 (2012) - 2012 • 1499
Whispering gallery mode pressure sensing
Weigel, T. and Esen, C. and Schweiger, G. and Ostendorf, A.
PROCEEDINGS OF SPIE - THE INTERNATIONAL SOCIETY FOR OPTICAL ENGINEERING. Volume: 8439 (2012) - 2012 • 1498
All semiconductor high power fs laser system with variable repetition rate
Balzer, J.C. and Schlauch, T. and Klehr, A. and Erbert, G. and Hofmann, M.R.
PROCEEDINGS OF SPIE - THE INTERNATIONAL SOCIETY FOR OPTICAL ENGINEERING. Volume: 8277 (2012) - 2012 • 1497
A neural network potential-energy surface for the water dimer based on environment-dependent atomic energies and charges
Morawietz, T. and Sharma, V. and Behler, J.
JOURNAL OF CHEMICAL PHYSICS. Volume: 136 (2012) - 2012 • 1496
Spray roasting of iron chloride FeCl 2: Laboratory scale experiments and a model for numerical simulation
Schiemann, M. and Wirtz, S. and Scherer, V. and Bärhold, F.
POWDER TECHNOLOGY. Volume: 228 (2012) - 2012 • 1495
Fabrication of ZrO 2 and ZrN films by metalorganic chemical vapor deposition employing new Zr precursors
Banerjee, M. and Srinivasan, N.B. and Zhu, H. and Kim, S.J. and Xu, K. and Winter, M. and Becker, H.-W. and Rogalla, D. and De Los Arcos, T. and Bekermann, D. and Barreca, D. and Fischer, R.A. and Devi, A.
CRYSTAL GROWTH AND DESIGN. Volume: 12 (2012) - 2012 • 1494
In-situ tensile testing of single-crystal molybdenum-alloy fibers with various dislocation densities in a scanning electron microscope
Johanns, K.E. and Sedlmayr, A. and Sudharshan Phani, P. and Mönig, R. and Kraft, O. and George, E.P. and Pharr, G.M.
JOURNAL OF MATERIALS RESEARCH. Volume: 27 (2012) - 2012 • 1493
Preemptive nematic order, pseudogap, and orbital order in the iron pnictides
Fernandes, R.M. and Chubukov, A.V. and Knolle, J. and Eremin, I. and Schmalian, J.
PHYSICAL REVIEW B - CONDENSED MATTER AND MATERIALS PHYSICS. Volume: 85 (2012) - 2012 • 1492
Construction of high-dimensional neural network potentials using environment-dependent atom pairs
Jose, K.V.J. and Artrith, N. and Behler, J.
JOURNAL OF CHEMICAL PHYSICS. Volume: 136 (2012) - 2012 • 1491
Determination of plastic material properties by analysis of residual imprint geometry of indentation
Schmaling, B. and Hartmaier, A.
JOURNAL OF MATERIALS RESEARCH. Volume: 27 (2012) - 2012 • 1490
β-Fe2 O3 nanomaterials from an iron(ii) diketonate-diamine complex: A study from molecular precursor to growth process
Barreca, D. and Carraro, G. and Devi, A. and Fois, E. and Gasparotto, A. and Seraglia, R. and MacCato, C. and Sada, C. and Tabacchi, G. and Tondello, E. and Venzo, A. and Winter, M.
DALTON TRANSACTIONS. Volume: 41 (2012) - 2012 • 1489
Impact of Anxiety Parameters on Prospective and Experienced Pain Intensity in Implant Surgery
Weisensee, W. and Scheer, M. and Muller, L. and Rothamel, D. and Kistler, F. and Bayer, G. and Johren, P. and Neugebauer, J.
IMPLANT DENTISTRY. Volume: 21 (2012) - 2012 • 1488
A quantitative 3D motility analysis of Trypanosoma brucei by use of digital in-line holographic microscopy
Weiße, S. and Heddergott, N. and Heydt, M. and Pflästerer, D. and Maier, T. and Haraszti, T. and Grunze, M. and Engstler, M. and Rosenhahn, A.
PLOS ONE. Volume: 7 (2012) - 2012 • 1487
Microforming process for embossing of LTCC tapes
Bartsch, H. and Albrecht, A. and Hoffmann, M. and Müller, J.
JOURNAL OF MICROMECHANICS AND MICROENGINEERING. Volume: 22 (2012) - 2012 • 1486
Modelling the kinetics of a triple junction
Fischer, F.D. and Svoboda, J. and Hackl, K.
ACTA MATERIALIA. Volume: 60 (2012) - 2012 • 1485
Reconstructions and electronic structure of (112̄2) and (112̄2 ) semipolar AlN surfaces
Kalesaki, E. and Lymperakis, L. and Kioseoglou, J. and Neugebauer, J. and Karakostas, T. and Komninou, P.
JOURNAL OF APPLIED PHYSICS. Volume: 112 (2012) - 2012 • 1484
A switch based on self-assembled thymine
Kalkan, F. and Mehlhorn, M. and Morgenstern, K.
JOURNAL OF PHYSICS-CONDENSED MATTER. Volume: 24 (2012) - 2012 • 1483
Crystal chemistry and properties of mullite-type Bi 2M 4O 9: An overview
Schneider, H. and Fischer, R.X. and Gesing, T.M. and Schreuer, J. and Mühlberg, M.
INTERNATIONAL JOURNAL OF MATERIALS RESEARCH. Volume: 103 (2012) - 2012 • 1482
Application of the thru-network-line self-calibration method for free space material characterizations
Will, B. and Rolfes, I.
PROCEEDINGS OF THE 2012 INTERNATIONAL CONFERENCE ON ELECTROMAGNETICS IN ADVANCED APPLICATIONS, ICEAA'12. Volume: (2012) - 2012 • 1481
Momentum matching in the tunneling between 2-dimensional and 0-dimensional electron systems
Beckel, A. and Zhou, D. and Marquardt, B. and Reuter, D. and Wieck, A.D. and Geller, M. and Lorke, A.
APPLIED PHYSICS LETTERS. Volume: 100 (2012) - 2012 • 1480
A scanning tunneling microscope with a scanning range from hundreds of micrometers down to nanometer resolution
Kalkan, F. and Zaum, C. and Morgenstern, K.
REVIEW OF SCIENTIFIC INSTRUMENTS. Volume: 83 (2012) - 2012 • 1479
A new approach on broadband calibration methods for free space applications
Will, B. and Rolfes, I.
IEEE MTT-S INTERNATIONAL MICROWAVE SYMPOSIUM DIGEST. Volume: (2012) - 2012 • 1478
A time domain transmission measurement system for dielectric characterizations
Will, B. and Gerding, M. and Schulz, C. and Baer, C. and Musch, T. and Rolfes, I.
INTERNATIONAL JOURNAL OF MICROWAVE AND WIRELESS TECHNOLOGIES. Volume: 4 (2012) - 2012 • 1477
Influence of grain boundary mobility on microstructure evolution during recrystallisation
Winning, M. and Raabe, D.
MATERIALS SCIENCE FORUM. Volume: 715-716 (2012) - 2012 • 1476
Multispectral photoacoustic coded excitation using pseudorandom codes
Beckmann, M.F. and Friedrich, C.-S. and Mienkina, M.P. and Gerhardt, N.C. and Hofmann, M.R. and Schmitz, G.
PROGRESS IN BIOMEDICAL OPTICS AND IMAGING - PROCEEDINGS OF SPIE. Volume: 8223 (2012) - 2012 • 1475
Electronic-structure calculations of large cadmium chalcogenide nanoparticles
Frenzel, J. and Joswig, J. O.
PHYSICA STATUS SOLIDI B-BASIC SOLID STATE PHYSICS. Volume: 249 (2012) - 2012 • 1474
Surface Diels-Alder reactions as an effective method to synthesize functional carbon materials
Kaper, H. and Grandjean, A. and Weidenthaler, C. and Schüth, F. and Goettmann, F.
CHEMISTRY - A EUROPEAN JOURNAL. Volume: 18 (2012) - 2012 • 1473
A novel approach to study dislocation density tensors and lattice rotation patterns in atomistic simulations
Begau, C. and Hua, J. and Hartmaier, A.
JOURNAL OF THE MECHANICS AND PHYSICS OF SOLIDS. Volume: 60 (2012) - 2012 • 1472
The dangling-bond defect in amorphous silicon: Statistical random versus kinetically driven defect geometries
Freysoldt, C. and Pfanner, G. and Neugebauer, J.
JOURNAL OF NON-CRYSTALLINE SOLIDS. Volume: 358 (2012) - 2012 • 1471
The stacking fault energy and its dependence on the interstitial content in various austenitic steels
Mujica, L. and Weber, S. and Theisen, W.
MATERIALS SCIENCE FORUM. Volume: 706-709 (2012) - 2012 • 1470
Electronic properties and charge transfer phenomena in Pt nanoparticles on γ-Al 2O 3: Size, shape, support, and adsorbate effects
Behafarid, F. and Ono, L.K. and Mostafa, S. and Croy, J.R. and Shafai, G. and Hong, S. and Rahman, T.S. and Bare, S.R. and Roldan Cuenya, B.
PHYSICAL CHEMISTRY CHEMICAL PHYSICS. Volume: 14 (2012) - 2012 • 1469
Theory-guided materials design of multi-phase Ti-Nb alloys with bone-matching elastic properties
Friák, M. and Counts, W.A. and Ma, D. and Sander, B. and Holec, D. and Raabe, D. and Neugebauer, J.
MATERIALS. Volume: 5 (2012) - 2012 • 1468
Welding of twinning-induced plasticity steels
Mújica Roncery, L. and Weber, S. and Theisen, W.
SCRIPTA MATERIALIA. Volume: 66 (2012) - 2012 • 1467
Coarsening phenomena of metal nanoparticles and the influence of the support pre-treatment: Pt/TiO 2(110)
Behafarid, F. and Roldan Cuenya, B.
SURFACE SCIENCE. Volume: 606 (2012) - 2012 • 1466
Mechanical properties of (20-30)Mn12Cr(0.56-0.7)CN corrosion resistant austenitic TWIP steels
Mújica Roncery, L. and Weber, S. and Theisen, W.
STEEL RESEARCH INTERNATIONAL. Volume: 83 (2012) - 2012 • 1465
Nano pinstripes: TiO 2 nanostripe formation by nanoparticle-mediated pinning of step edges
Behafarid, F. and Cuenya, B.R.
JOURNAL OF PHYSICAL CHEMISTRY LETTERS. Volume: 3 (2012) - 2012 • 1464
Choice is suffering: A Focused Information Criterion for model selection
Behl, P. and Dette, H. and Frondel, M. and Tauchmann, H.
ECONOMIC MODELLING. Volume: 29 (2012) - 2012 • 1463
Interfacial interaction driven CO oxidation: Nanostructured Ce 1-xLa xO 2-δ/TiO 2 solid solutions
Katta, L. and Reddy, B.M. and Muhler, M. and Grünert, W.
CATALYSIS SCIENCE AND TECHNOLOGY. Volume: 2 (2012) - 2012 • 1462
Enhancing magnetocrystalline anisotropy of the Fe 70Pd 30 magnetic shape memory alloy by adding Cu
Kauffmann-Weiss, S. and Hamann, S. and Gruner, M.E. and Schultz, L. and Ludwig, Al. and Fähler, S.
ACTA MATERIALIA. Volume: 60 (2012) - 2012 • 1461
Modeling and Simulation of Ion Energy Distribution Functions in Technological Plasmas
Mussenbrock, T.
CONTRIBUTIONS TO PLASMA PHYSICS. Volume: 52 (2012) - 2012 • 1460
Understanding the magnetic shape memory system Fe-Pd-X by thin film experiments and first principle calculations
Kauffmann-Weiss, S. and Hamann, S. and Gruner, M.E. and Buschbeck, J. and Ludwig, Al. and Schultz, L. and Fähler, S.
ADVANCED ENGINEERING MATERIALS. Volume: 14 (2012) - 2012 • 1459
The electrical asymmetry effect in geometrically asymmetric capacitive radio frequency plasmas
Schüngel, E. and Eremin, D. and Schulze, J. and Mussenbrock, T. and Czarnetzki, U.
JOURNAL OF APPLIED PHYSICS. Volume: 112 (2012) - 2012 • 1458
Influence of laves phase precipitation and coarsening on high-temperature strength of ferritic stainless steels
Nabiran, N. and Weber, S. and Theisen, W.
STEEL RESEARCH INTERNATIONAL. Volume: 83 (2012) - 2012 • 1457
Chemistry of materials-your journal for high science in 2012
Schüth, F.
CHEMISTRY OF MATERIALS. Volume: 24 (2012) - 2012 • 1456
Characterization of thin anodic oxides of Ti-Nb alloys by electrochemical impedance spectroscopy
Woldemedhin, M.T. and Raabe, D. and Hassel, A.W.
ELECTROCHIMICA ACTA. Volume: 82 (2012) - 2012 • 1455
Complete and partial oxidation of methane on ceria/platinum silicon carbide nanocomposites
Frind, R. and Borchardt, L. and Kockrick, E. and Mammitzsch, L. and Petasch, U. and Herrmann, M. and Kaskel, S.
CATALYSIS SCIENCE AND TECHNOLOGY. Volume: 2 (2012) - 2012 • 1454
Ammonia as a possible element in an energy infrastructure: Catalysts for ammonia decomposition
Schüth, F. and Palkovits, R. and Schlögl, R. and Su, D.S.
ENERGY AND ENVIRONMENTAL SCIENCE. Volume: 5 (2012) - 2012 • 1453
Characterization of transient discharges under atmospheric-pressure conditions applying nitrogen photoemission and current measurements
Keller, S. and Rajasekaran, P. and Bibinov, N. and Awakowicz, P.
JOURNAL OF PHYSICS D: APPLIED PHYSICS. Volume: 45 (2012) - 2012 • 1452
Strong dipole coupling in nonpolar nitride quantum dots due to Coulomb effects
Schuh, K. and Barthel, S. and Marquardt, O. and Hickel, T. and Neugebauer, J. and Czycholl, G. and Jahnke, F.
APPLIED PHYSICS LETTERS. Volume: 100 (2012) - 2012 • 1451
Oxide/water interfaces: How the surface chemistry modifies interfacial water properties
Gaigeot, M.-P. and Sprik, M. and Sulpizi, M.
JOURNAL OF PHYSICS CONDENSED MATTER. Volume: 24 (2012) - 2012 • 1450
Scanning electrochemical microscopy (SECM) in proton exchange membrane fuel cell research and development
Schuhmann, W. and Bron, M.
POLYMER ELECTROLYTE MEMBRANE AND DIRECT METHANOL FUEL CELL TECHNOLOGY: IN SITU CHARACTERIZATION TECHNIQUES FOR LOW TEMPERATURE FUEL CELLS. Volume: (2012) - 2012 • 1449
Co 3O 4/ZnO nanocomposites: From plasma synthesis to gas sensing applications
Bekermann, D. and Gasparotto, A. and Barreca, D. and Maccato, C. and Comini, E. and Sada, C. and Sberveglieri, G. and Devi, A. and Fischer, R.A.
ACS APPLIED MATERIALS AND INTERFACES. Volume: 4 (2012) - 2012 • 1448
Mesoporous nitrogen-rich carbon materials as catalysts for the oxygen reduction reaction in alkaline solution
Nagaiah, T.C. and Bordoloi, A. and Sánchez, M.D. and Muhler, M. and Schuhmann, W.
CHEMSUSCHEM. Volume: 5 (2012) - 2012 • 1447
Nitrogen- and Oxygen-Functionalized Multiwalled Carbon Nanotubes Used as Support in Iron-Catalyzed, High-Temperature Fischer-Tropsch Synthesis
Schulte, H.J. and Graf, B. and Xia, W. and Muhler, M.
CHEMCATCHEM. Volume: 4 (2012) - 2012 • 1446
Numerical simulation of interactions between the shield-supported tunnel construction process and the response of soft water-saturated soils
Nagel, F. and Stascheit, J. and Meschke, G.
INTERNATIONAL JOURNAL OF GEOMECHANICS. Volume: 12 (2012) - 2012 • 1445
Single live cell topography and activity imaging with the shear-force-based constant-distance scanning electrochemical microscope
Schulte, A. and Nebel, M. and Schuhmann, W.
METHODS IN ENZYMOLOGY. Volume: 504 (2012) - 2012 • 1444
Structural and magnetic properties of Co 2MnSi thin films
Belmeguenai, M. and Zighem, F. and Faurie, D. and Tuzcuoglu, H. and Chérif, S.-M. and Moch, P. and Westerholt, K. and Seiler, W.
PHYSICA STATUS SOLIDI (A) APPLICATIONS AND MATERIALS SCIENCE. Volume: 209 (2012) - 2012 • 1443
Toward highly stable electrocatalysts via nanoparticle pore confinement
Galeano, C. and Meier, J.C. and Peinecke, V. and Bongard, H. and Katsounaros, I. and Topalov, A.A. and Lu, A. and Mayrhofer, K.J.J. and Schüth, F.
JOURNAL OF THE AMERICAN CHEMICAL SOCIETY. Volume: 134 (2012) - 2012 • 1442
Atomic ordering effect in Ni 50Mn 37Sn 13 magnetocaloric ribbons
Wu, D. and Xue, S. and Frenzel, J. and Eggeler, G. and Zhai, Q. and Zheng, H.
MATERIALS SCIENCE AND ENGINEERING A. Volume: 534 (2012) - 2012 • 1441
Transverse rectification in density-modulated two-dimensional electron gases
Ganczarczyk, A. and Rojek, S. and Quindeau, A. and Geller, M. P. and Hucht, A. and Notthoff, C. and König, J. and Lorke, A. and Reuter, D. and Wieck, A.D.
PHYSICAL REVIEW B - CONDENSED MATTER AND MATERIALS PHYSICS. Volume: 86 (2012) - 2012 • 1440
Numerical and experimental investigation on lap shear fracture of Al/CFRP laminates
Naghipour, P. and Schulze, K. and Hausmann, J. and Bartsch, M.
COMPOSITES SCIENCE AND TECHNOLOGY. Volume: 72 (2012) - 2012 • 1439
A novel radio-frequency plasma probe for monitoring systems in dielectric deposition processes
Schulz, C. and Styrnoll, T. and Lapke, M. and Oberrath, J. and Storch, R. and Awakowicz, P. and Brinkmann, R.P. and Musch, T. and Mussenbrock, T. and Rolfes, I.
PROCEEDINGS OF THE 2012 INTERNATIONAL CONFERENCE ON ELECTROMAGNETICS IN ADVANCED APPLICATIONS, ICEAA'12. Volume: (2012) - 2012 • 1438
Modelling of dendritic growth and bubble formation
Wu, W. and Zhu, M.F. and Sun, D.K. and Dai, T. and Han, Q.Y. and Raabe, D.
IOP CONFERENCE SERIES: MATERIALS SCIENCE AND ENGINEERING. Volume: 33 (2012) - 2012 • 1437
Quadrupole mass spectrometry of reactive plasmas
Benedikt, J. and Hecimovic, A. and Ellerweg, D. and von Keudell, A.
JOURNAL OF PHYSICS D-APPLIED PHYSICS. Volume: 45 (2012) - 2012 • 1436
A multistatic feeding concept for beam steering based on a dielectric ellipsoidal antenna
Schulz, C. and Baer, C. and Pohl, N. and Musch, T. and Rolfes, I.
ASIA-PACIFIC MICROWAVE CONFERENCE PROCEEDINGS, APMC. Volume: (2012) - 2012 • 1435
Recrystallization and grain growth in ultra fine grained materials produced by high pressure torsion
Khorashadizadeh, A. and Winning, M. and Zaefferer, S. and Raabe, D.
RECRYSTALLIZATION AND GRAIN GROWTH IV. Volume: 715-716 (2012) - 2012 • 1434
Dislocation engineering and its effect on the oxidation behaviour
Naraparaju, R. and Christ, H.-J. and Renner, F.U. and Kostka, A.
MATERIALS AT HIGH TEMPERATURES. Volume: 29 (2012) - 2012 • 1433
Incubation effect and its influence on laser patterning of ITO thin film
Xiao, S. and Gurevich, E.L. and Ostendorf, A.
APPLIED PHYSICS A: MATERIALS SCIENCE AND PROCESSING. Volume: 107 (2012) - 2012 • 1432
Influence of Cs + and Na + on specific adsorption of *oH, *o, and *h at platinum in acidic sulfuric media
Berkes, B.B. and Inzelt, G. and Schuhmann, W. and Bondarenko, A.S.
JOURNAL OF PHYSICAL CHEMISTRY C. Volume: 116 (2012) - 2012 • 1431
Synthesis of titanium carbonitride coating layers with star-shaped crystallite morphology
Garcia, J. and Pitonak, R. and Agudo, L. and Kostka, A.
MATERIALS LETTERS. Volume: 68 (2012) - 2012 • 1430
Advanced nanomechanics in the TEM: Effects of thermal annealing on FIB prepared Cu samples
Kiener, D. and Zhang, Z. and Šturm, S. and Cazottes, S. and Imrich, P.J. and Kirchlechner, C. and Dehm, G.
PHILOSOPHICAL MAGAZINE. Volume: 92 (2012) - 2012 • 1429
Selective Ablation of Thin Films by Ultrashort Laser Pulses
Xiao, S. Z. and Schops, B. and Ostendorf, A.
LASER ASSISTED NET SHAPE ENGINEERING 7 (LANE 2012). Volume: 39 (2012) - 2012 • 1428
Effects of transition metal substitutions on the incommensurability and spin fluctuations in BaFe 2As 2 by elastic and inelastic neutron scattering
Kim, M.G. and Lamsal, J. and Heitmann, T.W. and Tucker, G.S. and Pratt, D.K. and Khan, S.N. and Lee, Y.B. and Alam, A. and Thaler, A. and Ni, N. and Ran, S. and Bud'Ko, S.L. and Marty, K.J. and Lumsden, M.D. and Canfield, P.C. and Harmon, B.N. and Johnson, D.D. and Kreyssig, A. and McQueeney, R.J. and Goldman, A.I.
PHYSICAL REVIEW LETTERS. Volume: 109 (2012) - 2012 • 1427
Atomic layer deposition of HfO 2 thin films employing a heteroleptic hafnium precursor
Xu, K. and Milanov, A.P. and Parala, H. and Wenger, C. and Baristiran-Kaynak, C. and Lakribssi, K. and Toader, T. and Bock, C. and Rogalla, D. and Becker, H.-W. and Kunze, U. and Devi, A.
CHEMICAL VAPOR DEPOSITION. Volume: 18 (2012) - 2012 • 1426
Ehrlich-Schwoebel barrier and interface-limited decay in island kinetics on Ag(100)
Ge, X. and Morgenstern, K.
PHYSICAL REVIEW B - CONDENSED MATTER AND MATERIALS PHYSICS. Volume: 85 (2012) - 2012 • 1425
Antiferromagnetic order in the quasicrystal approximant Cd 6Tb studied by x-ray resonant magnetic scattering
Kim, M.G. and Beutier, G. and Kreyssig, A. and Hiroto, T. and Yamada, T. and Kim, J.W. and De Boissieu, M. and Tamura, R. and Goldman, A.I.
PHYSICAL REVIEW B - CONDENSED MATTER AND MATERIALS PHYSICS. Volume: 85 (2012) - 2012 • 1424
Photoluminescence lineshape features of carbon δ-doped GaAs heterostructures
Schuster, J. and Kim, T.Y. and Batke, E. and Reuter, D. and Wieck, A.D.
JOURNAL OF PHYSICS CONDENSED MATTER. Volume: 24 (2012) - 2012 • 1423
Atomic layer deposition of Gd 2O 3 and Dy 2O 3: A study of the ALD characteristics and structural and electrical properties
Xu, K. and Ranjith, R. and Laha, A. and Parala, H. and Milanov, A.P. and Fischer, R.A. and Bugiel, E. and Feydt, J. and Irsen, S. and Toader, T. and Bock, C. and Rogalla, D. and Osten, H.-J. and Kunze, U. and Devi, A.
CHEMISTRY OF MATERIALS. Volume: 24 (2012) - 2012 • 1422
Fabrication of a CO2-selective membrane by stepwise liquid-phase deposition of an alkylether functionalized pillared-layered metal-organic framework [Cu2L2P]n on a macroporous support
Bétard, A. and Bux, H. and Henke, S. and Zacher, D. and Caro, J. and Fischer, R.A.
MICROPOROUS AND MESOPOROUS MATERIALS. Volume: 150 (2012) - 2012 • 1421
Understanding properties of electrified interfaces as a prerequisite for label-free DNA hybridization detection
Gebala, M. and Schuhmann, W.
PHYSICAL CHEMISTRY CHEMICAL PHYSICS. Volume: 14 (2012) - 2012 • 1420
Fe K-edge X-ray resonant magnetic scattering from Ba(Fe1-xCox)2As2 superconductors
Kim, M.G. and Kreyssig, A. and Lee, Y.B. and McQueeney, R.J. and Harmon, B.N. and Goldman, A.I.
EUROPEAN PHYSICAL JOURNAL: SPECIAL TOPICS. Volume: 208 (2012) - 2012 • 1419
Vacancy formation energies in fcc metals: Influence of exchange-correlation functionals and correction schemes
Nazarov, R. and Hickel, T. and Neugebauer, J.
PHYSICAL REVIEW B - CONDENSED MATTER AND MATERIALS PHYSICS. Volume: 85 (2012) - 2012 • 1418
Non-resonant optical excitation of mode-locked electron spin coherence in (In,Ga)As/GaAs quantum dot ensemble
Schwan, A. and Varwig, S. and Greilich, A. and Yakovlev, D.R. and Reuter, D. and Wieck, A.D. and Bayer, M.
APPLIED PHYSICS LETTERS. Volume: 100 (2012) - 2012 • 1417
The surface science approach for understanding reactions on oxide powders: The importance of IR spectroscopy
Xu, M. and Noei, H. and Fink, K. and Muhler, M. and Wang, Y. and Wöll, C.
ANGEWANDTE CHEMIE - INTERNATIONAL EDITION. Volume: 51 (2012) - 2012 • 1416
Growth optimization and characterization of lattice-matched Al 0.82In 0.18N optical confinement layer for edge emitting nitride laser diodes
Kim-Chauveau, H. and Frayssinet, E. and Damilano, B. and De Mierry, P. and Bodiou, L. and Nguyen, L. and Vennéguès, P. and Chauveau, J.-M. and Cordier, Y. and Duboz, J.Y. and Charash, R. and Vajpeyi, A. and Lamy, J.-M. and Akhter, M. and Maaskant, P.P. and Corbett, B. and Hangleiter, A. and Wieck, A.
JOURNAL OF CRYSTAL GROWTH. Volume: 338 (2012) - 2012 • 1415
Dissociation of formic acid on anatase TiO 2(1 0 1) probed by vibrational spectroscopy
Xu, M. and Noei, H. and Buchholz, M. and Muhler, M. and Wöll, C. and Wang, Y.
CATALYSIS TODAY. Volume: 182 (2012) - 2012 • 1414
Electrical control of a solid-state flying qubit
Yamamoto, M. and Takada, S. and Bäuerle, C. and Watanabe, K. and Wieck, A.D. and Tarucha, S.
NATURE NANOTECHNOLOGY. Volume: 7 (2012) - 2012 • 1413
Dual frequency capacitive plasmas in Fe and Ni sputter applications: Correlation of discharge properties on thin film properties
Bienholz, S. and Semmler, E. and Awakowicz, P. and Brunken, H. and Ludwig, Al.
PLASMA SOURCES SCIENCE AND TECHNOLOGY. Volume: 21 (2012) - 2012 • 1412
Strain-induced phase transitions in epitaxial NaNbO3 thin films grown by metal-organic chemical vapour deposition
Schwarzkopf, J. and Schmidbauer, M. and Remmele, T. and Duk, A. and Kwasniewski, A. and Bin Anooz, S. and Devi, A. and Fornari, R.
JOURNAL OF APPLIED CRYSTALLOGRAPHY. Volume: 45 (2012) - 2012 • 1411
Yield stress influenced by the ratio of wire diameter to grain size - A competition between the effects of specimen microstructure and dimension in micro-sized polycrystalline copper wires
Yang, B. and Motz, C. and Rester, M. and Dehm, G.
PHILOSOPHICAL MAGAZINE. Volume: 92 (2012) - 2012 • 1410
Spin-controlled vertical-cavity surface-emitting lasers
Gerhardt, N.C. and Hofmann, M.R.
ADVANCES IN OPTICAL TECHNOLOGIES. Volume: (2012) - 2012 • 1409
The influence of secondary phase carbide particles on the passivity behaviour of NiTi shape memory alloys
Neelakantan, L. and Monchev, B. and Frotscher, M. and Eggeler, G.
MATERIALS AND CORROSION. Volume: 63 (2012) - 2012 • 1408
Enrichment of paramagnetic ions from homogeneous solutions in inhomogeneous magnetic fields
Yang, X. and Tschulik, K. and Uhlemann, M. and Odenbach, S. and Eckert, K.
JOURNAL OF PHYSICAL CHEMISTRY LETTERS. Volume: 3 (2012) - 2012 • 1407
Investigation of reversible plasticity in a micron-sized, single crystalline copper bending beam by X-ray μlaue diffraction
Kirchlechner, C. and Grosinger, W. and Kapp, M.W. and Imrich, P.J. and Micha, J.-S. and Ulrich, O. and Keckes, J. and Dehm, G. and Motz, C.
PHILOSOPHICAL MAGAZINE. Volume: 92 (2012) - 2012 • 1406
PEDOT-CNT composite microelectrodes for recording and electrostimulation applications: Fabrication, morphology, and electrical properties
Gerwig, R. and Fuchsberger, K. and Schroeppel, B. and Link, G.S. and Heusel, G. and Kraushaar, U. and Schuhmann, W. and Stett, A. and Stelzle, M.
FRONTIERS IN NEUROENGINEERING. Volume: (2012) - 2012 • 1405
Expected and unexpected plastic behavior at the micron scale: An in situ μlaue tensile study
Kirchlechner, C. and Imrich, P.J. and Grosinger, W. and Kapp, M.W. and Keckes, J. and Micha, J.S. and Ulrich, O. and Thomas, O. and Labat, S. and Motz, C. and Dehm, G.
ACTA MATERIALIA. Volume: 60 (2012) - 2012 • 1404
Experimental model identification and vibration control of a smart cantilever beam using piezoelectric actuators and sensors
Nestorović, T. and Durrani, N. and Trajkov, M.
JOURNAL OF ELECTROCERAMICS. Volume: 29 (2012) - 2012 • 1403
On the fidelity of ultra-wideband antennas
Sczyslo, S. and Rolfes, I. and Kaiser, T.
PROCEEDINGS OF THE 2012 IEEE-APS TOPICAL CONFERENCE ON ANTENNAS AND PROPAGATION IN WIRELESS COMMUNICATIONS, APWC'12. Volume: (2012) - 2012 • 1402
New zeolite Al-COE-4: Reaching highly shape-selective catalytic performance through interlayer expansion
Yilmaz, B. and Müller, U. and Feyen, M. and Zhang, H. and Xiao, F.-S. and De Baerdemaeker, T. and Tijsebaert, B. and Jacobs, P. and De Vos, D. and Zhang, W. and Bao, X. and Imai, H. and Tatsumi, T. and Gies, H.
CHEMICAL COMMUNICATIONS. Volume: 48 (2012) - 2012 • 1401
Implementation of a user defined piezoelectric shell element for analysis of active structures
Nestorović, T. and Marinković, D. and Chandrashekar, G. and Marinković, Z. and Trajkov, M.
FINITE ELEMENTS IN ANALYSIS AND DESIGN. Volume: 52 (2012) - 2012 • 1400
Determination of the delay spread of an indoor channel measurement campaign in the UHF band
Sczyslo, S. and Dortmund, S. and Rolfes, I.
IEEE ANTENNAS AND PROPAGATION SOCIETY, AP-S INTERNATIONAL SYMPOSIUM (DIGEST). Volume: (2012) - 2012 • 1399
A DFT study of formation energies of Fe-Zn-Al intermetallics and solutes
Klaver, T.P.C. and Madsen, G.K.H. and Drautz, R.
INTERMETALLICS. Volume: 31 (2012) - 2012 • 1398
Microassembly of complex and three-dimensional microstructures using holographic optical tweezers
Ghadiri, R. and Weigel, T. and Esen, C. and Ostendorf, A.
JOURNAL OF MICROMECHANICS AND MICROENGINEERING. Volume: 22 (2012) - 2012 • 1397
Nanoindentation of pseudoelastic NiTi containing Ni4Ti 3 precipitates
Young, M.L. and Frotscher, M. and Bei, H. and Simon, T. and George, E.P. and Eggeler, G.
INTERNATIONAL JOURNAL OF MATERIALS RESEARCH. Volume: 103 (2012) - 2012 • 1396
Process limitations in microassembling using holographic optical tweezers
Ghadiri, R. and Guo, Q. and Yeoh, I. and Esen, C. and Ostendorf, A.
PROCEEDINGS OF SPIE - THE INTERNATIONAL SOCIETY FOR OPTICAL ENGINEERING. Volume: 8244 (2012) - 2012 • 1395
Cast-replicated NiTiCu foams with superelastic properties
Young, M.L. and Defouw, J.D. and Frenzel, J. and Dunand, D.C.
METALLURGICAL AND MATERIALS TRANSACTIONS A: PHYSICAL METALLURGY AND MATERIALS SCIENCE. Volume: 43 (2012) - 2012 • 1394
High mobility ZnO thin film transistors using the novel deposition of high-k dielectrics
Ngwashi, D.K. and Cross, R.B.M. and Paul, S. and Milanov, A.P. and Devi, A.
MATERIALS RESEARCH SOCIETY SYMPOSIUM PROCEEDINGS. Volume: 1315 (2012) - 2012 • 1393
Non-syngas direct steam reforming of methanol to hydrogen and carbon dioxide at low temperature
Yu, K.M.K. and Tong, W. and West, A. and Cheung, K. and Li, T. and Smith, G. and Guo, Y. and Tsang, S.C.E.
NATURE COMMUNICATIONS. Volume: 3 (2012) - 2012 • 1392
Interlayer expansion of the hydrous layer silicate rub-36 to a functionalized, microporous framework silicate: Crystal structure analysis and physical and chemical characterization
Gies, H. and Müller, U. and Yilmaz, B. and Feyen, M. and Tatsumi, T. and Imai, H. and Zhang, H. and Xie, B. and Xiao, F.-S. and Bao, X. and Zhang, W. and Baerdemaeker, T.D. and De Vos, D.
CHEMISTRY OF MATERIALS. Volume: 24 (2012) - 2012 • 1391
Nanoscale austenite reversion through partitioning, segregation and kinetic freezing: Example of a ductile 2 GPa Fe-Cr-C steel
Yuan, L. and Ponge, D. and Wittig, J. and Choi, P. and Jiménez, J.A. and Raabe, D.
ACTA MATERIALIA. Volume: 60 (2012) - 2012 • 1390
Application of the multiscale fem to the modeling of nonlinear composites with a random microstructure
Klinge, S. and Hackl, K.
INTERNATIONAL JOURNAL FOR MULTISCALE COMPUTATIONAL ENGINEERING. Volume: 10 (2012) - 2012 • 1389
Contribution of the reflection to the attenuation properties of cancellous bone
Klinge, S. and Hackl, K.
COMPLEX VARIABLES AND ELLIPTIC EQUATIONS. Volume: 57 (2012) - 2012 • 1388
Model Checks in Inverse Regression Models with Convolution-Type Operators
Bissantz, N. and Dette, H. and Proksch, K.
SCANDINAVIAN JOURNAL OF STATISTICS. Volume: 39 (2012) - 2012 • 1387
Damage characterization of thermal barrier coatings by acoustic emission and thermography
Nies, D. and Rehmer, B. and Skrotzki, B. and Vaßen, R.
ADVANCED ENGINEERING MATERIALS. Volume: 14 (2012) - 2012 • 1386
Hologram reconstruction corrected for measurements through layers with different refractive indices in digital in-line holographic microscopy
Sendra, G.H. and Weisse, S. and Maleschlijski, S. and Rosenhahn, A.
APPLIED OPTICS. Volume: 51 (2012) - 2012 • 1385
Computer simulation of two-step atomization in graphite furnaces for analytical atomic spectrometry
Zakharov, Yu.A. and Kokorina, O.B. and Lysogorskii, Yu.V. and Staroverov, A.E.
JOURNAL OF ANALYTICAL CHEMISTRY. Volume: 67 (2012) - 2012 • 1384
Low-Temperature CO Oxidation over Cu-Based Metal-Organic Frameworks Monitored by using FTIR Spectroscopy
Noei, H. and Amirjalayer, S. and Müller, M. and Zhang, X. and Schmid, R. and Muhler, M. and Fischer, R.A. and Wang, Y.
CHEMCATCHEM. Volume: 4 (2012) - 2012 • 1383
SECM and SKPFM Studies of the Local Corrosion Mechanism of Al Alloys - A Pathway to an Integrated SKP-SECM System
Senöz, C. and Maljusch, A. and Rohwerder, M. and Schuhmann, W.
ELECTROANALYSIS. Volume: 24 (2012) - 2012 • 1382
Orientation informed nanoindentation of α-titanium: Indentation pileup in hexagonal metals deforming by prismatic slip
Zambaldi, C. and Yang, Y. and Bieler, T.R. and Raabe, D.
JOURNAL OF MATERIALS RESEARCH. Volume: 27 (2012) - 2012 • 1381
Probing the mechanism of low-temperature CO oxidation on Au/ZnO catalysts by vibrational spectroscopy
Noei, H. and Birkner, A. and Merz, K. and Muhler, M. and Wang, Y.
JOURNAL OF PHYSICAL CHEMISTRY C. Volume: 116 (2012) - 2012 • 1380
Atomic scale effects of alloying, partitioning, solute drag and austempering on the mechanical properties of high-carbon bainitic-austenitic TRIP steels
Seol, J.-B. and Raabe, D. and Choi, P.-P. and Im, Y.-R. and Park, C.-G.
ACTA MATERIALIA. Volume: 60 (2012) - 2012 • 1379
Tailoring of CNT surface oxygen groups by gas-phase oxidation and its implications for lithium ion batteries
Klink, S. and Ventosa, E. and Xia, W. and La Mantia, F. and Muhler, M. and Schuhmann, W.
ELECTROCHEMISTRY COMMUNICATIONS. Volume: 15 (2012) - 2012 • 1378
Fluctuation-induced magnetization dynamics and criticality at the interface of a topological insulator with a magnetically ordered layer
Nogueira, F.S. and Eremin, I.
PHYSICAL REVIEW LETTERS. Volume: 109 (2012) - 2012 • 1377
Effect of tensile stress on the in-plane resistivity anisotropy in BaFe 2As 2
Blomberg, E.C. and Kreyssig, A. and Tanatar, M.A. and Fernandes, R.M. and Kim, M.G. and Thaler, A. and Schmalian, J. and Bud'Ko, S.L. and Canfield, P.C. and Goldman, A.I. and Prozorov, R.
PHYSICAL REVIEW B - CONDENSED MATTER AND MATERIALS PHYSICS. Volume: 85 (2012) - 2012 • 1376
The importance of cell geometry for electrochemical impedance spectroscopy in three-electrode lithium ion battery test cells
Klink, S. and Madej, E. and Ventosa, E. and Lindner, A. and Schuhmann, W. and La Mantia, F.
ELECTROCHEMISTRY COMMUNICATIONS. Volume: 22 (2012) - 2012 • 1375
Strong-coupling topological Josephson effect in quantum wires
Nogueira, F.S. and Eremin, I.
JOURNAL OF PHYSICS CONDENSED MATTER. Volume: 24 (2012) - 2012 • 1374
High-throughput study of martensitic transformations in the complete Ti-Ni-Cu system
Zarnetta, R. and Buenconsejo, P.J.S. and Savan, A. and Thienhaus, S. and Ludwig, Al.
INTERMETALLICS. Volume: 26 (2012) - 2012 • 1373
SintClad: A new approach for the production of wear-resistant tools
Blüm, M. and Hill, H. and Moll, H. and Weber, S. and Theisen, W.
JOURNAL OF MATERIALS ENGINEERING AND PERFORMANCE. Volume: 21 (2012) - 2012 • 1372
A model for the devolatilisation of large thermoplastic particles under co-firing conditions
Bluhm-Drenhaus, T. and Becker, A. and Wirtz, S. and Scherer, V.
FUEL. Volume: 101 (2012) - 2012 • 1371
Utilization of the catalyst layer of dimensionally stable anodes - Interplay of morphology and active surface area
Zeradjanin, A.R. and La Mantia, F. and Masa, J. and Schuhmann, W.
ELECTROCHIMICA ACTA. Volume: 82 (2012) - 2012 • 1370
Incommensurate magnetic fluctuations and Fermi surface topology in LiFeAs
Knolle, J. and Zabolotnyy, V.B. and Eremin, I. and Borisenko, S.V. and Qureshi, N. and Braden, M. and Evtushinsky, D.V. and Kim, T.K. and Kordyuk, A.A. and Sykora, S. and Hess, C. and Morozov, I.V. and Wurmehl, S. and Moessner, R. and Büchner, B.
PHYSICAL REVIEW B - CONDENSED MATTER AND MATERIALS PHYSICS. Volume: 86 (2012) - 2012 • 1369
Influence of Microstructural Features on the Propagation of Microstructurally Short Fatigue Cracks in Structural Steels
Sharaf, M. and Lian, J. and Vajragupta, N. and Münstermann, S. and Bleck, W. and Schmaling, B. and Ma, A. and Hartmaier, A.
FATIGUE OF MATERIALS II: ADVANCES AND EMERGENCES IN UNDERSTANDING. Volume: (2012) - 2012 • 1368
Evaluation of the catalytic performance of gas-evolving electrodes using local electrochemical noise measurements
Zeradjanin, A.R. and Ventosa, E. and Bondarenko, A.S. and Schuhmann, W.
CHEMSUSCHEM. Volume: 5 (2012) - 2012 • 1367
The antiferromagnetic phase of iron-based superconductors: An itinerant approach
Knolle, J. and Eremin, I.
IRON-BASED SUPERCONDUCTORS: MATERIALS, PROPERTIES AND MECHANISMS. Volume: (2012) - 2012 • 1366
Role of Water in the Chlorine Evolution Reaction at RuO 2-Based Electrodesa-Understanding Electrocatalysis as a Resonance Phenomenon
Zeradjanin, A.R. and Menzel, N. and Strasser, P. and Schuhmann, W.
CHEMSUSCHEM. Volume: 5 (2012) - 2012 • 1365
Martensitic phase transformations in Ni-Ti-based shape memory alloys: The Landau theory
Shchyglo, O. and Salman, U. and Finel, A.
ACTA MATERIALIA. Volume: 60 (2012) - 2012 • 1364
Study of the human tooth using a low–voltage CS-corrected TEM
Zhang, Z.L. and Dehm, G.
MICROSCOPY AND MICROANALYSIS. Volume: 18 (2012) - 2012 • 1363
Influence of structural properties on ballistic transport in nanoscale epitaxial graphene cross junctions
Bock, C. and Weingart, S. and Karaissaridis, E. and Kunze, U. and Speck, F. and Seyller, T.
NANOTECHNOLOGY. Volume: 23 (2012) - 2012 • 1362
Direct monophasic replacement of fatty acid by DMSA on SPION surface
Gogoi, M. and Deb, P. and Vasan, G. and Keil, P. and Kostka, A. and Erbe, A.
APPLIED SURFACE SCIENCE. Volume: 258 (2012) - 2012 • 1361
Elastic and piezoelectric constants of tourmaline single crystals at non-ambient temperatures determined by resonant ultrasound spectroscopy
Shekhar Pandey, C. and Schreuer, J.
JOURNAL OF APPLIED PHYSICS. Volume: 111 (2012) - 2012 • 1360
Dealloying strategy to fabricate ultrafine nanoporous gold-based alloys with high structural stability and tunable magnetic properties
Zhang, Z. and Zhang, C. and Gao, Y. and Frenzel, J. and Sun, J. and Eggeler, G.
CRYSTENGCOMM. Volume: 14 (2012) - 2012 • 1359
Differential tunability effect on the optical properties of doped and undoped quantum dots
Gogoi, M. and Deb, P. and Kostka, A.
PHYSICA STATUS SOLIDI (A) APPLICATIONS AND MATERIALS SCIENCE. Volume: 209 (2012) - 2012 • 1358
Thin-film β-MoO 3 Supported on α-Fe 2O 3 as a Shell-Core Catalyst for the Selective Oxidation of Methanol to Formaldehyde
Shi, G. and Franzke, T. and Sánchez, M.D. and Xia, W. and Weis, F. and Seipenbusch, M. and Kasper, G. and Muhler, M.
CHEMCATCHEM. Volume: 4 (2012) - 2012 • 1357
Leaf-like dislocation substructures and the decrease of martensitic start temperatures: A new explanation for functional fatigue during thermally induced martensitic transformations in coarse-grained Ni-rich Ti-Ni shape memory alloys
Zhang, J. and Somsen, C. and Simon, T. and Ding, X. and Hou, S. and Ren, S. and Ren, X. and Eggeler, G. and Otsuka, K. and Sun, J.
ACTA MATERIALIA. Volume: 60 (2012) - 2012 • 1356
Atomistic simulation of the influence of nanomachining-induced deformation on subsequent nanoindentation
Zhang, J.J. and Sun, T. and Hartmaier, A. and Yan, Y.D.
COMPUTATIONAL MATERIALS SCIENCE. Volume: 59 (2012) - 2012 • 1355
Thermodynamic phase diagram, phase competition, and uniaxial pressure effects in BaFe 2(As 1-xP x) 2 studied by thermal expansion
Böhmer, A.E. and Burger, P. and Hardy, F. and Wolf, T. and Schweiss, P. and Fromknecht, R. and V. Löhneysen, H. and Meingast, C. and Mak, H.K. and Lortz, R. and Kasahara, S. and Terashima, T. and Shibauchi, T. and Matsuda, Y.
PHYSICAL REVIEW B - CONDENSED MATTER AND MATERIALS PHYSICS. Volume: 86 (2012) - 2012 • 1354
Thickness-dependence of the B2-B19 martensitic transformation in nanoscale shape memory alloy thin films: Zero-hysteresis in 75 nm thick Ti 51Ni38Cu11 thin films
König, D. and Buenconsejo, P.J.S. and Grochla, D. and Hamann, S. and Pfetzing-Micklich, J. and Ludwig, Al.
ACTA MATERIALIA. Volume: 60 (2012) - 2012 • 1353
Twin boundary spacing-dependent friction in nanotwinned copper
Zhang, J. and Wei, Y. and Sun, T. and Hartmaier, A. and Yan, Y. and Li, X.
PHYSICAL REVIEW B - CONDENSED MATTER AND MATERIALS PHYSICS. Volume: 85 (2012) - 2012 • 1352
High-resolution thermal expansion of isovalently substituted BaFe 2(As1-xPx)2
Böhmer, A.E. and Burger, P. and Hardy, F. and Wolf, T. and Schweiss, P. and Fromknecht, R. and Von Löhneysen, H. and Meingast, C. and Kasahara, S. and Terashima, T. and Shibauchi, T. and Matsuda, Y.
JOURNAL OF PHYSICS: CONFERENCE SERIES. Volume: 391 (2012) - 2012 • 1351
Atomic forces at finite magnetic temperatures: Phonons in paramagnetic iron
Körmann, F. and Dick, A. and Grabowski, B. and Hickel, T. and Neugebauer, J.
PHYSICAL REVIEW B - CONDENSED MATTER AND MATERIALS PHYSICS. Volume: 85 (2012) - 2012 • 1350
Phase-field model with finite interface dissipation: Extension to multi-component multi-phase alloys
Zhang, L. and Steinbach, I.
ACTA MATERIALIA. Volume: 60 (2012) - 2012 • 1349
Atomistically informed crystal plasticity model for body-centered cubic iron
Koester, A. and Ma, A. and Hartmaier, A.
ACTA MATERIALIA. Volume: 60 (2012) - 2012 • 1348
Effect of Si addition on the oxidation resistance of Co-Re-Cr-alloys: Recent attainments in the development of novel alloys
Gorr, B. and Burk, S. and Depka, T. and Somsen, C. and Abu-Samra, H. and Christ, H.-J. and Eggeler, G.
INTERNATIONAL JOURNAL OF MATERIALS RESEARCH. Volume: 103 (2012) - 2012 • 1347
Effect of exchange bias on magnetic anisotropies in fe/coo bilayers
Öztürk, M. and Demirci, E. and Topkaya, R. and Kazan, S. and Akdoǧan, N. and Obaida, M. and Westerholt, K.
JOURNAL OF SUPERCONDUCTIVITY AND NOVEL MAGNETISM. Volume: 25 (2012) - 2012 • 1346
Gas phase oxidation as a tool to introduce oxygen containing groups on metal-loaded carbon nanofibers
Gosselink, R.W. and Van Den Berg, R. and Xia, W. and Muhler, M. and De Jong, K.P. and Bitter, J.H.
CARBON. Volume: 50 (2012) - 2011 • 1345
Influence of dislocation density on the pop-in behavior and indentation size effect in CaF2 single crystals: Experiments and molecular dynamics simulations
Lodes, M.A. and Hartmaier, A. and Göken, M. and Durst, K.
ACTA MATERIALIA. Volume: 59 (2011) - 2011 • 1344
Space-resolved characterization of high frequency atmospheric-pressure plasma in nitrogen, applying optical emission spectroscopy and numerical simulation
Rajasekaran, P. and Ruhrmann, C. and Bibinov, N. and Awakowicz, P.
JOURNAL OF PHYSICS D: APPLIED PHYSICS. Volume: 44 (2011) - 2011 • 1343
Structurally designed synthesis of mechanically stable poly(benzoxazine-co- resol)-based porous carbon monoliths and their application as high-performance CO2 capture sorbents
Hao, G.-P. and Li, W.-C. and Qian, D. and Wang, G.-H. and Zhang, W.-P. and Zhang, T. and Wang, A.-Q. and Schüth, F. and Bongard, H.-J. and Lu, A.-H.
JOURNAL OF THE AMERICAN CHEMICAL SOCIETY. Volume: 133 (2011) - 2011 • 1342
Enhanced characteristics of HVOF-sprayed MCrAlY bond coats for TBC applications
Rajasekaran, B. and Mauer, G. and Vaßen, R.
JOURNAL OF THERMAL SPRAY TECHNOLOGY. Volume: 20 (2011) - 2011 • 1341
Effect of HIP parameters on the micro-structural evolution of a single crystal Ni-based superalloy
Lopez-Galilea, I. and Huth, S. and Bartsch, M. and Theisen, W.
ADVANCED MATERIALS RESEARCH. Volume: 278 (2011) - 2011 • 1340
Characterization of dielectric barrier discharge (DBD) on mouse and histological evaluation of the plasma-treated tissue
Rajasekaran, P. and Opländer, C. and Hoffmeister, D. and Bibinov, N. and Suschek, C.V. and Wandke, D. and Awakowicz, P.
PLASMA PROCESSES AND POLYMERS. Volume: 8 (2011) - 2011 • 1339
Growth of GaN based structures on focused ion beam patterned templates
Cordier, Y. and Tottereau, O. and Nguyen, L. and Ramdani, M. and Soltani, A. and Boucherit, M. and Troadec, D. and Lo, F.-Y. and Hu, Y.Y. and Ludwig, Ar. and Wieck, A.D.
PHYSICA STATUS SOLIDI (C) CURRENT TOPICS IN SOLID STATE PHYSICS. Volume: 8 (2011) - 2011 • 1338
Simulation of the external pressure influence on the micro-Structural evolution of a Single Crystal Ni-Based superalloy
Lopez-Galilea, I. and Huth, S. and Fries, S.G. and Steinbach, I. and Theisen, W.
ADVANCED MATERIALS RESEARCH. Volume: 278 (2011) - 2011 • 1337
Stabilization of an ambient-pressure collapsed tetragonal phase in CaFe2As2 and tuning of the orthorhombic-antiferromagnetic transition temperature by over 70 K via control of nanoscale precipitates
Ran, S. and Bud'Ko, S.L. and Pratt, D.K. and Kreyssig, A. and Kim, M.G. and Kramer, M.J. and Ryan, D.H. and Rowan-Weetaluktuk, W.N. and Furukawa, Y. and Roy, B. and Goldman, A.I. and Canfield, P.C.
PHYSICAL REVIEW B - CONDENSED MATTER AND MATERIALS PHYSICS. Volume: 83 (2011) - 2011 • 1336
Interface failure and adhesion measured by focused ion beam cutting of metal-polymer interfaces
Cordill, M.J. and Schmidegg, K. and Dehm, G.
PHILOSOPHICAL MAGAZINE LETTERS. Volume: 91 (2011) - 2011 • 1335
Evaluation of homoleptic guanidinate and amidinate complexes of gadolinium and dysprosium for MOCVD of rare-earth nitride thin films
Thiede, T.B. and Krasnopolski, M. and Milanov, A.P. and De Los Arcos, T. and Ney, A. and Becker, H.-W. and Rogalla, D. and Winter, J. and Devi, A. and Fischer, R.A.
CHEMISTRY OF MATERIALS. Volume: 23 (2011) - 2011 • 1334
Microstructure and adhesion of as-deposited and annealed Cu/Ti films on polyimide
Cordill, M.J. and Taylor, A. and Schalko, J. and Dehm, G.
INTERNATIONAL JOURNAL OF MATERIALS RESEARCH. Volume: 102 (2011) - 2011 • 1333
Electrical spin injection in InAs quantum dots at room temperature and adjustment of the emission wavelength for spintronic applications
Ludwig, Ar. and Roescu, R. and Rai, A.K. and Trunov, K. and Stromberg, F. and Li, M. and Soldat, H. and Ebbing, A. and Gerhardt, N.C. and Hofmann, M.R. and Wende, H. and Keune, W. and Reuter, D. and Wieck, A.D.
JOURNAL OF CRYSTAL GROWTH. Volume: 323 (2011) - 2011 • 1332
Modular high-throughput test stand for versatile screening of thin-film materials libraries
Thienhaus, S. and Hamann, S. and Ludwig, Al.
SCIENCE AND TECHNOLOGY OF ADVANCED MATERIALS. Volume: 12 (2011) - 2011 • 1331
The oxidation of tyrosine and tryptophan studied by a molecular dynamics normal hydrogen electrode
Costanzo, F. and Sulpizi, M. and Valle, R.G.D. and Sprik, M.
JOURNAL OF CHEMICAL PHYSICS. Volume: 134 (2011) - 2011 • 1330
Sterilization of heat-sensitive silicone implant material by low-pressure gas plasma
Hauser, J. and Esenwein, S.-A. and Awakowicz, P. and Steinau, H.-U. and Köller, M. and Halfmann, H.
BIOMEDICAL INSTRUMENTATION AND TECHNOLOGY. Volume: 45 (2011) - 2011 • 1329
Comparative study of hydrothermal treatment and thermal annealing effects on the properties of electrodeposited micro-columnar ZnO thin films
Lupan, O. and Pauporté, T. and Tiginyanu, I.M. and Ursaki, V.V. and Şontea, V. and Ono, L.K. and Cuenya, B.R. and Chow, L.
THIN SOLID FILMS. Volume: 519 (2011) - 2011 • 1328
High temperature elastic properties of Mg-cordierite: Experimental studies and atomistic simulations
Haussühl, E. and Vinograd, V.L. and Krenzel, T.F. and Schreuer, J. and Wilson, D.J. and Ottinger, J.
ZEITSCHRIFT FUR KRISTALLOGRAPHIE. Volume: 226 (2011) - 2011 • 1327
Exploring the void structure and activity of RUB-39 based expanded materials using the hydroconversion of decane
Tijsebaert, B. and Henry, M. and Gies, H. and Xiao, F.-S. and Zhang, W. and Bao, X. and Imai, H. and Tatsumi, T. and Müller, U. and Yilmaz, B. and Jacobs, P. and Vos, D.D.
JOURNAL OF CATALYSIS. Volume: 282 (2011) - 2011 • 1326
Shape-selective synthesis of methylamines over the RRO zeolite Al-RUB-41
Tijsebaert, B. and Yilmaz, B. and Müller, U. and Gies, H. and Zhang, W. and Bao, X. and Xiao, F.-S. and Tatsumi, T. and De Vos, D.
JOURNAL OF CATALYSIS. Volume: 278 (2011) - 2011 • 1325
A new approach to stability of singular time delay systems in the sense of non-Lyapunov delay independent conditions
Debeljkovic, D.L. and Buzurovic, I.M. and Nestorovic, T. and Popov, D.
IEEE INTERNATIONAL CONFERENCE ON CONTROL AND AUTOMATION, ICCA. Volume: (2011) - 2011 • 1324
Spatial variation of the surface state onset close to three types of surface steps on Ag(111) studied by scanning tunnelling spectroscopy
Heidorn, S. and Morgenstern, K.
NEW JOURNAL OF PHYSICS. Volume: 13 (2011) - 2011 • 1323
Theoretical modeling of growth processes, extended defects, and electronic properties of III-nitride semiconductor nanostructures
Lymperakis, L. and Abu-Farsakh, H. and Marquardt, O. and Hickel, T. and Neugebauer, J.
PHYSICA STATUS SOLIDI (B) BASIC RESEARCH. Volume: 248 (2011) - 2011 • 1322
Investigating the influence of the operating frequency on the gas phase emitter effect of dysprosium in ceramic metal halide lamps
Reinelt, J. and Westermeier, M. and Ruhrmann, C. and Bergner, A. and Luijks, G.M.J.F. and Awakowicz, P. and Mentel, J.
JOURNAL OF PHYSICS D: APPLIED PHYSICS. Volume: 44 (2011) - 2011 • 1321
Further results on stability of linear discrete time delay systems over a finite time interval: Novel delay-independent conditions
Debeljkovic, D.L. and Buzurovic, I.M. and Stojanovic, S.B. and Popov, D. and Nestorovic, T.
IEEE INTERNATIONAL CONFERENCE ON CONTROL AND AUTOMATION, ICCA. Volume: (2011) - 2011 • 1320
Grain resolved orientation changes and texture evolution in a thermally strained Al film on Si substrate
Heinz, W. and Dehm, G.
SURFACE AND COATINGS TECHNOLOGY. Volume: 206 (2011) - 2011 • 1319
Investigating the dependence of the temperature of high-intensity discharge (HID) lamp electrodes on the operating frequency by pyrometric measurements
Reinelt, J. and Westermeier, M. and Ruhrmann, C. and Bergner, A. and Awakowicz, P. and Mentel, J.
JOURNAL OF PHYSICS D: APPLIED PHYSICS. Volume: 44 (2011) - 2011 • 1318
Time delayed system stability theory in the sense of non-Lyapunov delay independent and delay dependent approach: New results
Debeljkovic, D.L. and Buzurović, I. and Nestorović, T. and Stojanovic, S.B. and Dimitrijevic, N.J. and Aleksendric, M.S.
PROCEEDINGS OF THE IEEE INTERNATIONAL CONFERENCE ON CONTROL APPLICATIONS. Volume: (2011) - 2011 • 1317
In situ scanning tunneling microscopy study of selective dissolution of Au3Cu and Cu3Au (0 0 1)
Renner, F.U. and Eckstein, G.A. and Lymperakis, L. and Dakkouri-Baldauf, A. and Rohwerder, M. and Neugebauer, J. and Stratmann, M.
ELECTROCHIMICA ACTA. Volume: 56 (2011) - 2011 • 1316
On finite and practical stability of time delayed systems: Lyapunov-Krassovski approach, delay dependent criteria
Debeljkovic, D.L.J. and Buzurovic, I.M. and Nestorovic, T. and Popov, D.
PROCEEDINGS OF THE 2011 CHINESE CONTROL AND DECISION CONFERENCE, CCDC 2011. Volume: (2011) - 2011 • 1315
First-principles investigation of the effect of carbon on the stacking fault energy of Fe-C alloys
Abbasi, A. and Dick, A. and Hickel, T. and Neugebauer, J.
ACTA MATERIALIA. Volume: 59 (2011) - 2011 • 1314
Ab initio simulation of effects of structural singularities in aerogel absorption potential
Debras, C. and Tayurskii, D. and Minisini, B. and Lysogorskiy, Y.
JOURNAL OF PHYSICS: CONFERENCE SERIES. Volume: 324 (2011) - 2011 • 1313
Atomic vapor deposition approach to In 2O 3 thin films
Hellwig, M. and Parala, H. and Cybinksa, J. and Barreca, D. and Gasparotto, A. and Niermann, B. and Becker, H.-W. and Rogalla, D. and Feydt, J. and Irsen, S. and Mudring, A.-V. and Winter, J. and Fischer, R.A. and Devi, A.
JOURNAL OF NANOSCIENCE AND NANOTECHNOLOGY. Volume: 11 (2011) - 2011 • 1312
Annealing effects on microstructure and coercive field of ferritic-martensitic ODS Eurofer steel
Renzetti, R.A. and Sandim, H.R.Z. and Sandim, M.J.R. and Santos, A.D. and Möslang, A. and Raabe, D.
MATERIALS SCIENCE AND ENGINEERING A. Volume: 528 (2011) - 2011 • 1311
Applications of an energy-dispersive pnCCD for X-ray reflectivity: Investigation of interdiffusion in Fe-Pt multilayers
Abboud, A. and Send, S. and Hartmann, R. and Strüder, L. and Savan, A. and Ludwig, Al. and Zotov, N. and Pietsch, U.
PHYSICA STATUS SOLIDI (A) APPLICATIONS AND MATERIALS SCIENCE. Volume: 208 (2011) - 2011 • 1310
Microstructure-based description of the deformation of metals: Theory and application
Helm, D. and Butz, A. and Raabe, D. and Gumbsch, P.
JOM. Volume: 63 (2011) - 2011 • 1309
Deformation mechanisms in micron-sized PST TiAl compression samples: Experiment and model
Rester, M. and Fischer, F.D. and Kirchlechner, C. and Schmoelzer, T. and Clemens, H. and Dehm, G.
ACTA MATERIALIA. Volume: 59 (2011) - 2011 • 1308
Spatially resolved simulation of a radio-frequency driven micro-atmospheric pressure plasma jet and its effluent
Hemke, T. and Wollny, A. and Gebhardt, M. and Brinkmann, R.P. and Mussenbrock, T.
JOURNAL OF PHYSICS D: APPLIED PHYSICS. Volume: 44 (2011) - 2011 • 1307
Multiple phase-transitions upon selective CO 2 adsorption in an alkyl ether functionalized metal-organic framework - An in situ X-ray diffraction study
Henke, S. and Florian Wieland, D.C. and Meilikhov, M. and Paulus, M. and Sternemann, C. and Yusenko, K. and Fischer, R.A.
CRYSTENGCOMM. Volume: 13 (2011) - 2011 • 1306
Gated channels in a honeycomb-like zinc-dicarboxylate-bipyridine framework with flexible alkyl ether side chains
Henke, S. and Fischer, R.A.
JOURNAL OF THE AMERICAN CHEMICAL SOCIETY. Volume: 133 (2011) - 2011 • 1305
Optimized orthogonal tight-binding basis: Application to iron
Madsen, G.K.H. and McEniry, E.J. and Drautz, R.
PHYSICAL REVIEW B - CONDENSED MATTER AND MATERIALS PHYSICS. Volume: 83 (2011) - 2011 • 1304
Surface reactions as carbon removal mechanism in deposition of silicon dioxide films at atmospheric pressure
Reuter, R. and Ellerweg, D. and von Keudell, A. and Benedikt, J.
APPLIED PHYSICS LETTERS. Volume: 98 (2011) - 2011 • 1303
Mechanisms of grain boundary softening and strain-rate sensitivity in deformation of ultrafine-grained metals at high temperatures
Ahmed, N. and Hartmaier, A.
ACTA MATERIALIA. Volume: 59 (2011) - 2011 • 1302
Flexibility and sorption selectivity in rigid metal-organic frameworks: The impact of ether-functionalised linkers (Chemistry - A European Journal (2010) (16))
Henke, S. and Schmid, R. and Grunwaldt, J.-D. and Fischer, R.A.
CHEMISTRY - A EUROPEAN JOURNAL. Volume: 17 (2011) - 2011 • 1301
Ultrathin metal oxidation for vacuum monitoring device applications
Mäder, S. and Haas, T. and Kunze, U. and Doll, T.
PHYSICA STATUS SOLIDI (A) APPLICATIONS AND MATERIALS SCIENCE. Volume: 208 (2011) - 2011 • 1300
RKKY interaction in the spin-density-wave phase of iron-based superconductors
Akbari, A. and Eremin, I. and Thalmeier, P.
PHYSICAL REVIEW B - CONDENSED MATTER AND MATERIALS PHYSICS. Volume: 84 (2011) - 2011 • 1299
The frontier orbitals of a push-pull azobenzene adsorbed on a metal surface in different bonding geometries investigated by scanning tunneling spectroscopy and spectroscopy mapping
Henzl, J. and Morgenstern, K.
JOURNAL OF CHEMICAL PHYSICS. Volume: 135 (2011) - 2011 • 1298
Finite-element simulation of the anti-buckling-effect of a shape memory alloy bar
Richter, F. and Kastner, O. and Eggeler, G.
JOURNAL OF MATERIALS ENGINEERING AND PERFORMANCE. Volume: 20 (2011) - 2011 • 1297
Quasiparticle interference in the heavy-fermion superconductor CeCoIn 5
Akbari, A. and Thalmeier, P. and Eremin, I.
PHYSICAL REVIEW B - CONDENSED MATTER AND MATERIALS PHYSICS. Volume: 84 (2011) - 2011 • 1296
Reaction spectroscopy of frontier orbitals
Henzl, J. and Morgenstern, K.
JOURNAL OF PHYSICAL CHEMISTRY C. Volume: 115 (2011) - 2011 • 1295
Radiaxial-fibrous calcites: A new look at an old problem
Richter, D.K. and Neuser, R.D. and Schreuer, J. and Gies, H. and Immenhauser, A.
SEDIMENTARY GEOLOGY. Volume: 239 (2011) - 2011 • 1294
Magnetic rare-earth impurity resonance bound states in iron-based superconductors
Akbari, A. and Thalmeier, P. and Eremin, I.
JOURNAL OF SUPERCONDUCTIVITY AND NOVEL MAGNETISM. Volume: 24 (2011) - 2011 • 1293
Electrons surfing on a sound wave as a platform for quantum optics with flying electrons
Hermelin, S. and Takada, S. and Yamamoto, M. and Tarucha, S. and Wieck, A.D. and Saminadayar, L. and Bäuerle, C. and Meunier, T.
NATURE. Volume: 477 (2011) - 2011 • 1292
The impact of water on CO oxidation with Au/TiO 2 catalysts: Poison or promotor? A study with an Au-TiO 2/MCM-48 model catalyst
De Toni, A. and Gies, H. and Grünert, W.
CATALYSIS LETTERS. Volume: 141 (2011) - 2011 • 1291
(1 0 0) GaAs/AlxGa1-xAs heterostructures for Zeeman spin splitting studies of hole quantum wires
Trunov, K. and Reuter, D. and Ludwig, Ar. and Chen, J.C.H. and Klochan, O. and Micolich, A.P. and Hamilton, A.R. and Wieck, A.D.
JOURNAL OF CRYSTAL GROWTH. Volume: 323 (2011) - 2011 • 1290
Field cooling-induced magnetic anisotropy in exchange biased CoO/Fe bilayer studied by ferromagneticresonance
Akdoǧan, N. and Kazan, S. and Akta, B. and Özdemir, M. and Inam, H. and Obaida, M. and Dudek, J. and Westerholt, K.
JOURNAL OF MAGNETISM AND MAGNETIC MATERIALS. Volume: 323 (2011) - 2011 • 1289
Optimal Experimental Design Strategies for Detecting Hormesis
Dette, H. and Pepelyshev, A. and Wong, W.K.
RISK ANALYSIS. Volume: 31 (2011) - 2011 • 1288
On the opening of a class of fatigue cracks due to thermo-mechanical fatigue testing of thermal barrier coatings
Hernandez, M.T. and Cojocaru, D. and Bartsch, M. and Karlsson, A.M.
COMPUTATIONAL MATERIALS SCIENCE. Volume: 50 (2011) - 2011 • 1287
Surface modification by glow discharge gasplasma treatment improves vascularization of allogenic bone implants
Ring, A. and Tilkorn, D.J. and Goertz, O. and Langer, S. and Schaffran, A. and Awakowicz, P. and Hauser, J.
JOURNAL OF ORTHOPAEDIC RESEARCH. Volume: 29 (2011) - 2011 • 1286
How to obtain structured metal deposits from diamagnetic ions in magnetic gradient fields?
Tschulik, K. and Yang, X. and Mutschke, G. and Uhlemann, M. and Eckert, K. and Sueptitz, R. and Schultz, L. and Gebert, A.
ELECTROCHEMISTRY COMMUNICATIONS. Volume: 13 (2011) - 2011 • 1285
A measure of stationarity in locally stationary processes with applications to testing
Dette, H. and Preuss, P. and Vetter, M.
JOURNAL OF THE AMERICAN STATISTICAL ASSOCIATION. Volume: 106 (2011) - 2011 • 1284
Electrodeposition of separated 3D metallic structures by pulse-reverse plating in magnetic gradient fields
Tschulik, K. and Sueptitz, R. and Uhlemann, M. and Schultz, L. and Gebert, A.
ELECTROCHIMICA ACTA. Volume: 56 (2011) - 2011 • 1283
Optimal designs for estimating the derivative in nonlinear regression
Dette, H. and Melas, V.B. and Shpilev, P.
STATISTICA SINICA. Volume: 21 (2011) - 2011 • 1282
Design of a novel Mn-based 1 GPa duplex stainless TRIP steel with 60% ductility by a reduction of austenite stability
Herrera, C. and Ponge, D. and Raabe, D.
ACTA MATERIALIA. Volume: 59 (2011) - 2011 • 1281
Effect of nodes, ellipticity, and impurities on the spin resonance in iron-based superconductors
Maiti, S. and Knolle, J. and Eremin, I. and Chubukov, A.V.
PHYSICAL REVIEW B - CONDENSED MATTER AND MATERIALS PHYSICS. Volume: 84 (2011) - 2011 • 1280
In situ analysis of three-dimensional electrolyte convection evolving during the electrodeposition of copper in magnetic gradient fields
Tschulik, K. and Cierpka, C. and Gebert, A. and Schultz, L. and Kähler, C.J. and Uhlemann, M.
ANALYTICAL CHEMISTRY. Volume: 83 (2011) - 2011 • 1279
A dislocation density-based crystal plasticity constitutive model for prismatic slip in α-titanium
Alankar, A. and Eisenlohr, P. and Raabe, D.
ACTA MATERIALIA. Volume: 59 (2011) - 2011 • 1278
Optimal design for smoothing splines
Dette, H. and Melas, V.B. and Pepelyshev, A.
ANNALS OF THE INSTITUTE OF STATISTICAL MATHEMATICS. Volume: 63 (2011) - 2011 • 1277
NMR studies of benzene mobility in metal-organic framework MOF-5
Hertel, S. and Wehring, M. and Amirjalayer, S. and Gratz, M. and Lincke, J. and Krautscheid, H. and Schmid, R. and Stallmach, F.
EPJ APPLIED PHYSICS. Volume: 55 (2011) - 2011 • 1276
Testing non-parametric hypotheses for stationary processes by estimating minimal distances
Dette, H. and Kinsvater, T. and Vetter, M.
JOURNAL OF TIME SERIES ANALYSIS. Volume: 32 (2011) - 2011 • 1275
Integrated scanning kelvin probe-scanning electrochemical microscope system: Development and first applications
Maljusch, A. and Schönberger, B. and Lindner, A. and Stratmann, M. and Rohwerder, M. and Schuhmann, W.
ANALYTICAL CHEMISTRY. Volume: 83 (2011) - 2011 • 1274
A Note on the De La Garza Phenomenon for Locally Optimal Designs
Dette, H. and Melas, V. B.
ANNALS OF STATISTICS. Volume: 39 (2011) - 2011 • 1273
Thermodynamic properties of Pt nanoparticles: Size, shape, support, and adsorbate effects
Roldan Cuenya, B. and Alcántara Ortigoza, M. and Ono, L.K. and Behafarid, F. and Mostafa, S. and Croy, J.R. and Paredis, K. and Shafai, G. and Rahman, T.S. and Li, L. and Zhang, Z. and Yang, J.C.
PHYSICAL REVIEW B - CONDENSED MATTER AND MATERIALS PHYSICS. Volume: 84 (2011) - 2011 • 1272
Orientational ordering of interstitial atoms and martensite formation in dilute Fe-based solid solutions
Udyansky, A. and Von Pezold, J. and Dick, A. and Neugebauer, J.
PHYSICAL REVIEW B - CONDENSED MATTER AND MATERIALS PHYSICS. Volume: 83 (2011) - 2011 • 1271
Estimation of additive quantile regression
Dette, H. and Scheder, R.
ANNALS OF THE INSTITUTE OF STATISTICAL MATHEMATICS. Volume: 63 (2011) - 2011 • 1270
Absolute pK a values and solvation structure of amino acids from density functional based molecular dynamics simulation
Mangold, M. and Rolland, L. and Costanzo, F. and Sprik, M. and Sulpizi, M. and Blumberger, J.
JOURNAL OF CHEMICAL THEORY AND COMPUTATION. Volume: 7 (2011) - 2011 • 1269
Optimal designs for trigonometric regression models
Dette, H. and Melas, V.B. and Shpilev, P.
JOURNAL OF STATISTICAL PLANNING AND INFERENCE. Volume: 141 (2011) - 2011 • 1268
Microfluidic emulsion separation - Simultaneous separation and sensing by multilayer nanofilm structures
Uhlmann, P. and Varnik, F. and Truman, P. and Zikos, G. and Moulin, J.-F. and Müller-Buschbaum, P. and Stamm, M.
JOURNAL OF PHYSICS CONDENSED MATTER. Volume: 23 (2011) - 2011 • 1267
Advanced Calculations for Defects in Materials: Electronic Structure Methods
Alkauskas, A. and Deàk, P. and Neugebauer, J. and Pasquarello, A. and Van de Walle, C.G.
ADVANCED CALCULATIONS FOR DEFECTS IN MATERIALS: ELECTRONIC STRUCTURE METHODS. Volume: (2011) - 2011 • 1266
Comparing Conditional Quantile Curves
Dette, H. and Wagener, J. and Volgushev, S.
SCANDINAVIAN JOURNAL OF STATISTICS. Volume: 38 (2011) - 2011 • 1265
Anodic repassivation of low energy Au-implanted ultra-thin anodic Al 2O 3
Mardare, A.I. and Melnikov, A. and Wieck, A.D. and Hassel, A.W.
PHYSICA STATUS SOLIDI (A) APPLICATIONS AND MATERIALS SCIENCE. Volume: 208 (2011) - 2011 • 1264
Hierarchical micro- and mesoporous carbide-derived carbon as a high-performance electrode material in supercapacitors
Rose, M. and Korenblit, Y. and Kockrick, E. and Borchardt, L. and Oschatz, M. and Kaskel, S. and Yushin, G.
SMALL. Volume: 7 (2011) - 2011 • 1263
Advanced Calculations for Defects in Solids - Electronic Structure Methods
Alkauskas, A. and Deák, P. and Neugebauer, J. and Pasquarello, A. and Van de Walle, C.G.
PHYSICA STATUS SOLIDI (B) BASIC RESEARCH. Volume: 248 (2011) - 2011 • 1262
Hydrodynamic fluctuations in thermostatted multiparticle collision dynamics
Híjar, H. and Sutmann, G.
PHYSICAL REVIEW E - STATISTICAL, NONLINEAR, AND SOFT MATTER PHYSICS. Volume: 83 (2011) - 2011 • 1261
Influence of heat treatment on the performance characteristics of a plastic mold steel
Hill, H. and Raab, U. and Weber, S. and Theisen, W. and Wollmann, M. and Wagner, L.
STEEL RESEARCH INTERNATIONAL. Volume: 82 (2011) - 2011 • 1260
Thermodynamic modelling of the Ag-Cu-Ti ternary system
Dezellus, O. and Arroyave, R. and Fries, S.G.
INTERNATIONAL JOURNAL OF MATERIALS RESEARCH. Volume: 102 (2011) - 2011 • 1259
The impact of processing on microstructure, single-phase properties and wear resistance of MMCs
Hill, H. and Weber, S. and Huth, S. and Niederhofer, P. and Theisen, W.
WEAR. Volume: 271 (2011) - 2011 • 1258
Development of orthogonal tight-binding models for Ti-C and Ti-N systems
Margine, E.R. and Kolmogorov, A.N. and Reese, M. and Mrovec, M. and Elsässer, C. and Meyer, B. and Drautz, R. and Pettifor, D.G.
PHYSICAL REVIEW B - CONDENSED MATTER AND MATERIALS PHYSICS. Volume: 84 (2011) - 2011 • 1257
Diffusion and phase transformation at the interface between an austenitic substrate and a thermally sprayed coating of ledeburitic cold-work tool steel
Röttger, A. and Weber, S. and Theisen, W. and Rajasekeran, B. and Vaßen, R.
STEEL RESEARCH INTERNATIONAL. Volume: 82 (2011) - 2011 • 1256
Exploring network topologies of copper paddle wheel based metal-organic frameworks with a first-principles derived force field
Amirjalayer, S. and Tafipolsky, M. and Schmid, R.
JOURNAL OF PHYSICAL CHEMISTRY C. Volume: 115 (2011) - 2011 • 1255
Investigation of corrosion and wear properties of fe based metal matrix composites consolidated by sintering and hot isostatic pressing
Hill, H. and Weber, S. and Siebert, S. and Theisen, W.
POWDER METALLURGY. Volume: 54 (2011) - 2011 • 1254
Mechanical properties of thermally sprayed Fe based coatings
Röttger, A. and Weber, S. and Theisen, W. and Rajasekeran, B. and Vassen, R.
MATERIALS SCIENCE AND TECHNOLOGY. Volume: 27 (2011) - 2011 • 1253
Parameterization of tight-binding models from density functional theory calculations
Urban, A. and Reese, M. and Mrovec, M. and Elsässer, C. and Meyer, B.
PHYSICAL REVIEW B - CONDENSED MATTER AND MATERIALS PHYSICS. Volume: 84 (2011) - 2011 • 1252
Ab initio based determination of thermodynamic properties of cementite including vibronic, magnetic, and electronic excitations
Dick, A. and Körmann, F. and Hickel, T. and Neugebauer, J.
PHYSICAL REVIEW B - CONDENSED MATTER AND MATERIALS PHYSICS. Volume: 84 (2011) - 2011 • 1251
Corrosion properties of a plastic mould steel with special focus on the processing route
Hill, H. and Huth, S. and Weber, S. and Theisen, W.
MATERIALS AND CORROSION. Volume: 62 (2011) - 2011 • 1250
All-electrical transport spectroscopy of non-equilibrium many-particle states in self-assembled quantum dots
Marquardt, B. and Geller, M. and Baxevanis, B. and Pfannkuche, D. and Wieck, A.D. and Reuter, D. and Lorke, A.
AIP CONFERENCE PROCEEDINGS. Volume: 1399 (2011) - 2011 • 1249
HT-PEM fuel cell system with integrated complex metal hydride storage tank
Urbanczyk, R. and Peil, S. and Bathen, D. and Heßke, C. and Burfeind, J. and Hauschild, K. and Felderhoff, M. and Schüth, F.
FUEL CELLS. Volume: 11 (2011) - 2011 • 1248
A regularization framework for damage-plasticity models via gradient enhancement of the free energy
Dimitrijevic, B.J. and Hackl, K.
INTERNATIONAL JOURNAL FOR NUMERICAL METHODS IN BIOMEDICAL ENGINEERING. Volume: 27 (2011) - 2011 • 1247
The influence of charged InAs quantum dots on the conductance of a two-dimensional electron gas: Mobility vs. carrier concentration
Marquardt, B. and Beckel, A. and Lorke, A. and Wieck, A.D. and Reuter, D. and Geller, M.
APPLIED PHYSICS LETTERS. Volume: 99 (2011) - 2011 • 1246
Transport spectroscopy of non-equilibrium many-particle spin states in self-assembled quantum dots
Marquardt, B. and Geller, M. and Baxevanis, B. and Pfannkuche, D. and Wieck, A.D. and Reuter, D. and Lorke, A.
NATURE COMMUNICATIONS. Volume: 2 (2011) - 2011 • 1245
Temperature dependence of low-energy phonons in magnetic nonsuperconducting TbNi2B2C
Anissimova, S. and Kreyssig, A. and Stockert, O. and Loewenhaupt, M. and Reznik, D.
PHYSICAL REVIEW B - CONDENSED MATTER AND MATERIALS PHYSICS. Volume: 84 (2011) - 2011 • 1244
A flexible, plane-wave-based formulation of continuum elasticity and multiband k·p models
Marquardt, O. and Schulz, S. and O'Reilly, E.P. and Freysoldt, C. and Boeck, S. and Hickel, T. and Neugebauer, J.
PROCEEDINGS OF THE INTERNATIONAL CONFERENCE ON NUMERICAL SIMULATION OF OPTOELECTRONIC DEVICES, NUSOD. Volume: (2011) - 2011 • 1243
Investigating the gas phase emitter effect of caesium and cerium in ceramic metal halide lamps in dependence on the operating frequency
Ruhrmann, C. and Westermeier, M. and Bergner, A. and Luijks, G.M.J.F. and Awakowicz, P. and Mentel, J.
JOURNAL OF PHYSICS D: APPLIED PHYSICS. Volume: 44 (2011) - 2011 • 1242
CO oxidation with Au/TiO2 aggregates encapsulated in the mesopores of MCM-48: Model studies on activation, deactivation and metal-support interaction
Van Den Berg, M.W.E. and De Toni, A. and Bandyopadhyay, M. and Gies, H. and Grünert, W.
APPLIED CATALYSIS A: GENERAL. Volume: 391 (2011) - 2011 • 1241
Pulsed-laser atom probe studies of a precipitation hardened maraging TRIP steel
Dmitrieva, O. and Choi, P. and Gerstl, S.S.A. and Ponge, D. and Raabe, D.
ULTRAMICROSCOPY. Volume: 111 (2011) - 2011 • 1240
Growth process, characterization, and modeling of electronic properties of coupled InAsSbP nanostructures
Marquardt, O. and Hickel, T. and Neugebauer, J. and Gambaryan, K.M. and Aroutiounian, V.M.
JOURNAL OF APPLIED PHYSICS. Volume: 110 (2011) - 2011 • 1239
Electromagnetically induced transparency in low-doped n-GaAs
Van Der Wal, C.H. and Sladkov, M. and Chaubal, A.U. and Bakker, M.P. and Onur, A.R. and Reuter, D. and Wieck, A.D.
AIP CONFERENCE PROCEEDINGS. Volume: 1399 (2011) - 2011 • 1238
Chemical gradients across phase boundaries between martensite and austenite in steel studied by atom probe tomography and simulation
Dmitrieva, O. and Ponge, D. and Inden, G. and Millán, J. and Choi, P. and Sietsma, J. and Raabe, D.
ACTA MATERIALIA. Volume: 59 (2011) - 2011 • 1237
Effect of alloying elements on hydrogen environment embrittlement of AISI type 304 austenitic stainless steel
Martin, M. and Weber, S. and Theisen, W. and Michler, T. and Naumann, J.
INTERNATIONAL JOURNAL OF HYDROGEN ENERGY. Volume: 36 (2011) - 2011 • 1236
High-pressure water intrusion investigation of pure silica RUB-41 and S-SOD zeolite materials
Saada, M.A. and Soulard, M. and Marler, B. and Gies, H. and Patarin, J.
JOURNAL OF PHYSICAL CHEMISTRY C. Volume: 115 (2011) - 2011 • 1235
Emerging and innovative processes in thermal spraying
Vardelle, A. and Vassen, R.
JOURNAL OF THERMAL SPRAY TECHNOLOGY. Volume: 20 (2011) - 2011 • 1234
Antenna impact on the gauging accuracy of industrial radar level measurements
Armbrecht, G. and Zietz, C. and Denicke, E. and Rolfes, I.
IEEE TRANSACTIONS ON MICROWAVE THEORY AND TECHNIQUES. Volume: 59 (2011) - 2011 • 1233
Construction of uricase-overproducing strains of Hansenula polymorpha and its application as biological recognition element in microbial urate biosensor
Dmytruk, K.V. and Smutok, O.V. and Dmytruk, O.V. and Schuhmann, W. and Sibirny, A.A.
BMC BIOTECHNOLOGY. Volume: 11 (2011) - 2011 • 1232
Comparison of the multi-sphere and polyhedral approach to simulate non-spherical particles within the discrete element method: Influence on temporal force evolution for multiple contacts
Höhner, D. and Wirtz, S. and Kruggel-Emden, H. and Scherer, V.
POWDER TECHNOLOGY. Volume: 208 (2011) - 2011 • 1231
Influence of machining-induced martensite on hydrogen-assisted fracture of AISI type 304 austenitic stainless steel
Martin, M. and Weber, S. and Izawa, C. and Wagner, S. and Pundt, A. and Theisen, W.
INTERNATIONAL JOURNAL OF HYDROGEN ENERGY. Volume: 36 (2011) - 2011 • 1230
Stability and dynamics of droplets on patterned substrates: Insights from experiments and lattice Boltzmann simulations
Varnik, F. and Gross, M. and Moradi, N. and Zikos, G. and Uhlmann, P. and Müller-Buschbaum, P. and Magerl, D. and Raabe, D. and Steinbach, I. and Stamm, M.
JOURNAL OF PHYSICS CONDENSED MATTER. Volume: 23 (2011) - 2011 • 1229
Scanning electrochemical microscopy for investigation of multicomponent bioelectrocatalytic films
Dobrzeniecka, A. and Zeradjanin, A. and Masa, J. and Stroka, J. and Goral, M. and Schuhmann, W. and Kulesza, P.J.
ECS TRANSACTIONS. Volume: 35 (2011) - 2011 • 1228
Intercalation in layered metal-organic frameworks: Reversible inclusion of an extended π-system
Arslan, H.K. and Shekhah, O. and Wieland, D.C.F. and Paulus, M. and Sternemann, C. and Schroer, M.A. and Tiemeyer, S. and Tolan, M. and Fischer, R.A. and Wöll, C.
JOURNAL OF THE AMERICAN CHEMICAL SOCIETY. Volume: 133 (2011) - 2011 • 1227
High-dimensional neural-network potentials for multicomponent systems: Applications to zinc oxide
Artrith, N. and Morawietz, T. and Behler, J.
PHYSICAL REVIEW B - CONDENSED MATTER AND MATERIALS PHYSICS. Volume: 83 (2011) - 2011 • 1226
Ab initio study of pressure stabilized NiTi allotropes: Pressure-induced transformations and hysteresis loops
Holec, D. and Friák, M. and Dlouhý, A. and Neugebauer, J.
PHYSICAL REVIEW B - CONDENSED MATTER AND MATERIALS PHYSICS. Volume: 84 (2011) - 2011 • 1225
Precipitation kinetics study of Al - Zr - X(Sc or Ti) alloys by phase field simulations and atom probe tomography
Masquelier, N. and Zapolsky, H. and Lefebvre, W. and Fries, S.G. and Patte, R. and Pareige, P.
SOLID STATE PHENOMENA. Volume: 172-174 (2011) - 2011 • 1224
Enhanced photoelectrochemical properties of WO3 thin films fabricated by reactive magnetron sputtering
Vidyarthi, V.S. and Hofmann, M. and Savan, A. and Sliozberg, K. and König, D. and Beranek, R. and Schuhmann, W. and Ludwig, Al.
INTERNATIONAL JOURNAL OF HYDROGEN ENERGY. Volume: 36 (2011) - 2011 • 1223
A new HRSEM approach to observe fine structures of novel nanostructured materials
Asahina, S. and Uno, S. and Suga, M. and Stevens, S.M. and Klingstedt, M. and Okano, Y. and Kudo, M. and Schüth, F. and Anderson, M.W. and Adschiri, T. and Terasaki, O.
MICROPOROUS AND MESOPOROUS MATERIALS. Volume: 146 (2011) - 2011 • 1222
A geometric characterization of optimal designs for regression models with correlated observations
Holland-Letz, T. and Dette, H. and Pepelyshev, A.
JOURNAL OF THE ROYAL STATISTICAL SOCIETY. SERIES B: STATISTICAL METHODOLOGY. Volume: 73 (2011) - 2011 • 1221
Partial oxidation of methane on Pt-supported lanthanide doped ceria-zirconia oxides: Effect of the surface/lattice oxygen mobility on catalytic performance
Sadykov, V.A. and Sazonova, N.N. and Bobin, A.S. and Muzykantov, V.S. and Gubanova, E.L. and Alikina, G.M. and Lukashevich, A.I. and Rogov, V.A. and Ermakova, E.N. and Sadovskaya, E.M. and Mezentseva, N.V. and Zevak, E.G. and Veniaminov, S.A. and Muhler, M. and Mirodatos, C. and Schuurman, Y. and Van Veen, A.C.
CATALYSIS TODAY. Volume: 169 (2011) - 2011 • 1220
Current developments and challenges in thermal barrier coatings
Mauer, G. and Vaßen, R.
SURFACE ENGINEERING. Volume: 27 (2011) - 2011 • 1219
Phase equilibria in the Fe-Nb system
Vob, S. and Palm, M. and Stein, F. and Raabe, D.
JOURNAL OF PHASE EQUILIBRIA AND DIFFUSION. Volume: 32 (2011) - 2011 • 1218
Plasma and particle temperature measurements in thermal spray: Approaches and applications
Mauer, G. and Vaßen, R. and Stöver, D.
JOURNAL OF THERMAL SPRAY TECHNOLOGY. Volume: 20 (2011) - 2011 • 1217
Improving powder injection in plasma spraying by optical diagnostics of the plasma and particle characterization
Mauer, G. and Vaßen, R. and Stöver, D. and Kirner, S. and Marqués, J.-L. and Zimmermann, S. and Forster, G. and Schein, J.
JOURNAL OF THERMAL SPRAY TECHNOLOGY. Volume: 20 (2011) - 2011 • 1216
Valence-dependent analytic bond-order potential for magnetic transition metals
Drautz, R. and Pettifor, D.G.
PHYSICAL REVIEW B - CONDENSED MATTER AND MATERIALS PHYSICS. Volume: 84 (2011) - 2011 • 1215
Short- and medium-range order in Zr80Pt20 liquids
Mauro, N.A. and Wessels, V. and Bendert, J.C. and Klein, S. and Gangopadhyay, A.K. and Kramer, M.J. and Hao, S.G. and Rustan, G.E. and Kreyssig, A. and Goldman, A.I. and Kelton, K.F.
PHYSICAL REVIEW B - CONDENSED MATTER AND MATERIALS PHYSICS. Volume: 83 (2011) - 2011 • 1214
Tight-binding simulation of transition-metal alloys
McEniry, E.J. and Madsen, G.K.H. and Drain, J.F. and Drautz, R.
JOURNAL OF PHYSICS CONDENSED MATTER. Volume: 23 (2011) - 2011 • 1213
A (S)TEM and atom probe tomography study of InGaN
Mehrtens, T. and Bley, S. and Schowalter, M. and Sebald, K. and Seyfried, M. and Gutowski, J. and Gerstl, S.S.A. and Choi, P.-P. and Raabe, D. and Rosenauer, A.
JOURNAL OF PHYSICS: CONFERENCE SERIES. Volume: 326 (2011) - 2011 • 1212
Compositional dependence of the compressive yield strength of Fe-Nb(-Al) and Co-Nb Laves phases
Voß, S. and Palm, M. and Stein, F. and Raabe, D.
MATERIALS RESEARCH SOCIETY SYMPOSIUM PROCEEDINGS. Volume: 1295 (2011) - 2011 • 1211
Lattice Boltzmann study of pattern formation in reaction-diffusion systems
Ayodele, S.G. and Varnik, F. and Raabe, D.
PHYSICAL REVIEW E - STATISTICAL, NONLINEAR, AND SOFT MATTER PHYSICS. Volume: 83 (2011) - 2011 • 1210
Columnar-structured thermal barrier coatings (TBCs) by thin film low-pressure plasma spraying (LPPS-TF)
Hospach, A. and Mauer, G. and Vaßen, R. and Stöver, D.
JOURNAL OF THERMAL SPRAY TECHNOLOGY. Volume: 20 (2011) - 2011 • 1209
Electrically driven intentionally positioned single quantum dot
Mehta, M. and Reuter, D. and Wieck, A.D. and Michaelis de Vasconcellos, S. and Zrenner, A. and Meier, C.
PHYSICA STATUS SOLIDI (C) CURRENT TOPICS IN SOLID STATE PHYSICS. Volume: 8 (2011) - 2011 • 1208
Wiring photosynthetic enzymes to electrodes
Badura, A. and Kothe, T. and Schuhmann, W. and Rögner, M.
ENERGY AND ENVIRONMENTAL SCIENCE. Volume: 4 (2011) - 2011 • 1207
First-principles study on the interaction of H interstitials with grain boundaries in α- and γ-Fe
Du, Y.A. and Ismer, L. and Rogal, J. and Hickel, T. and Neugebauer, J. and Drautz, R.
PHYSICAL REVIEW B - CONDENSED MATTER AND MATERIALS PHYSICS. Volume: 84 (2011) - 2011 • 1206
The synthesis of Nb-doped TiO2 nanoparticles by spray drying: An efficient and scalable method
Mei, B. and Sánchez, M.D. and Reinecke, T. and Kaluza, S. and Xia, W. and Muhler, M.
JOURNAL OF MATERIALS CHEMISTRY. Volume: 21 (2011) - 2011 • 1205
Separation of the inertial-ballistic signal from hot-electron thermopower in an injection-type ballistic rectifier
Salloch, D. and Wieser, U. and Kunze, U. and Hackbarth, T.
AIP CONFERENCE PROCEEDINGS. Volume: 1399 (2011) - 2011 • 1204
Photocurrent generation by photosystem 1 integrated in crosslinked redox hydrogels
Badura, A. and Guschin, D. and Kothe, T. and Kopczak, M.J. and Schuhmann, W. and Rögner, M.
ENERGY AND ENVIRONMENTAL SCIENCE. Volume: 4 (2011) - 2011 • 1203
Basic investigation of HfO2 based metal-insulator-metal diodes
Dudek, P. and Schmidt, R. and Lukosius, M. and Lupina, G. and Wenger, C. and Abrutis, A. and Albert, M. and Xu, K. and Devi, A.
THIN SOLID FILMS. Volume: 519 (2011) - 2011 • 1202
Stepwise deposition of metal organic frameworks on flexible synthetic polymer surfaces
Meilikhov, M. and Yusenko, K. and Schollmeyer, E. and Mayer, C. and Buschmann, H.-J. and Fischer, R.A.
DALTON TRANSACTIONS. Volume: 40 (2011) - 2011 • 1201
Influence of channel width on the performance of an injection-type ballistic rectifier: Carrier injection versus hot-electron thermopower
Salloch, D. and Wieser, U. and Kunze, U. and Hackbarth, T.
MICROELECTRONIC ENGINEERING. Volume: 88 (2011) - 2011 • 1200
Analysis of the particle stability in a new designed ultrasonic levitation device
Baer, S. and Andrade, M.A.B. and Esen, C. and Adamowski, J.C. and Schweiger, G. and Ostendorf, A.
REVIEW OF SCIENTIFIC INSTRUMENTS. Volume: 82 (2011) - 2011 • 1199
Creep in directionally solidified NiAl-Mo eutectics
Dudová, M. and Kuchařová, K. and Barták, T. and Bei, H. and George, E.P. and Somsen, C. and Dlouhý, A.
SCRIPTA MATERIALIA. Volume: 65 (2011) - 2011 • 1198
Electrical properties and structure of grain boundaries in n-conducting BaTiO3 ceramics
Hou, J. and Zhang, Z. and Preis, W. and Sitte, W. and Dehm, G.
JOURNAL OF THE EUROPEAN CERAMIC SOCIETY. Volume: 31 (2011) - 2011 • 1197
Liquid-crystalline elastomer microvalve for microfluidics
Sánchez-Ferrer, A. and Fischl, T. and Stubenrauch, M. and Albrecht, A. and Wurmus, H. and Hoffmann, M. and Finkelmann, H.
ADVANCED MATERIALS. Volume: 23 (2011) - 2011 • 1196
Size and size distribution of apatite crystals in sauropod fossil bones
Dumont, M. and Kostka, A. and Sander, P.M. and Borbely, A. and Kaysser-Pyzalla, A.
PALAEOGEOGRAPHY, PALAEOCLIMATOLOGY, PALAEOECOLOGY. Volume: 310 (2011) - 2011 • 1195
The relation between shear banding, microstructure and mechanical properties in Mg and Mg-Y alloys
Sandlöbes, S. and Schestakow, I. and Yi, S. and Zaefferer, S. and Chen, J. and Friák, M. and Neugebauer, J. and Raabe, D.
MATERIALS SCIENCE FORUM. Volume: 690 (2011) - 2011 • 1194
Non-invasive nano-imaging of ion implanted and activated copper in silicon
Ballout, F. and Samson, J.-S. and Schmidt, D.A. and Bründermann, E. and Mathis, Y.-L. and Gasharova, B. and Wieck, A. and Havenith, M.
JOURNAL OF APPLIED PHYSICS. Volume: 110 (2011) - 2011 • 1193
Solubility of carbon in α-iron under volumetric strain and close to the Σ5(3 1 0)[0 0 1] grain boundary: Comparison of DFT and empirical potential methods
Hristova, E. and Janisch, R. and Drautz, R. and Hartmaier, A.
COMPUTATIONAL MATERIALS SCIENCE. Volume: 50 (2011) - 2011 • 1192
Modelocked semiconductor laser system with pulse picking for variable repetition rate
Balzer, J.C. and Schlauch, T. and Hoffmann, Th. and Klehr, A. and Erbert, G. and Hofmann, M.R.
ELECTRONICS LETTERS. Volume: 47 (2011) - 2011 • 1191
Shoe box all semiconductor high power femtosecond laser system
Balzer, J.C. and Schlauch, T. and Hofmann, M.R. and Klehr, A. and Erbert, G.
2011 CONFERENCE ON LASERS AND ELECTRO-OPTICS EUROPE AND 12TH EUROPEAN QUANTUM ELECTRONICS CONFERENCE, CLEO EUROPE/EQEC 2011. Volume: (2011) - 2011 • 1190
Tuning the magnetic properties of Co nanoparticles by Pt capping
Ebbing, A. and Hellwig, O. and Agudo, L. and Eggeler, G. and Petracic, O.
PHYSICAL REVIEW B - CONDENSED MATTER AND MATERIALS PHYSICS. Volume: 84 (2011) - 2011 • 1189
Graphenated IR screen for detection of human and avian flu viruses
Banerjee, A. and Chakraborty, S. and Grebel, H.
PROCEEDINGS OF THE IEEE CONFERENCE ON NANOTECHNOLOGY. Volume: (2011) - 2011 • 1188
Tumbling of polymers in semidilute solution under shear flow
Huang, C.-C. and Sutmann, G. and Gompper, G. and Winkler, R.G.
EPL. Volume: 93 (2011) - 2011 • 1187
High-throughput characterization of Pt supported on thin film oxide material libraries applied in the oxygen reduction reaction
Schäfer, D. and Mardare, C. and Savan, A. and Sanchez, M.D. and Mei, B. and Xia, W. and Muhler, M. and Ludwig, Al. and Schuhmann, W.
ANALYTICAL CHEMISTRY. Volume: 83 (2011) - 2011 • 1186
Length-scale modulated and electrocatalytic activity enhanced nanoporous gold by doping
Wang, X. and Frenzel, J. and Wang, W. and Ji, H. and Qi, Z. and Zhang, Z. and Eggeler, G.
JOURNAL OF PHYSICAL CHEMISTRY C. Volume: 115 (2011) - 2011 • 1185
Influences of surface preparation on nanoindentation pop-in in single-crystal Mo
Wang, Z. and Bei, H. and George, E.P. and Pharr, G.M.
SCRIPTA MATERIALIA. Volume: 65 (2011) - 2011 • 1184
Reversible fcc ↔ bcc transformation in freestanding epitaxially grown Fe-Pd ferromagnetic shape memory films
Edler, T. and Hamann, S. and Ludwig, Al. and Mayr, S.G.
SCRIPTA MATERIALIA. Volume: 64 (2011) - 2011 • 1183
Determining the activation energies and slip systems for dislocation nucleation in body-centered cubic Mo and face-centered cubic Ni single crystals
Wang, L. and Bei, H. and Li, T.L. and Gao, Y.F. and George, E.P. and Nieh, T.G.
SCRIPTA MATERIALIA. Volume: 65 (2011) - 2011 • 1182
Fine-sorting one-dimensional particle-in-cell algorithm with Monte-Carlo collisions on a graphics processing unit
Mertmann, P. and Eremin, D. and Mussenbrock, T. and Brinkmann, R.P. and Awakowicz, P.
COMPUTER PHYSICS COMMUNICATIONS. Volume: 182 (2011) - 2011 • 1181
Powder metallurgy processing and compressive properties of Ti3AlC2/Al composites
Wang, W.J. and Gauthier-Brunet, V. and Bei, G.P. and Laplanche, G. and Bonneville, J. and Joulain, A. and Dubois, S.
MATERIALS SCIENCE AND ENGINEERING A. Volume: 530 (2011) - 2011 • 1180
Mechanistic studies of Fc-PNA(·DNA) surface dynamics based on the kinetics of electron-transfer processes
Hüsken, N. and Gȩbala, M. and La Mantia, F. and Schuhmann, W. and Metzler-Nolte, N.
CHEMISTRY - A EUROPEAN JOURNAL. Volume: 17 (2011) - 2011 • 1179
A facile synthesis of shape- and size-controlled α-Fe 2O3 nanoparticles through hydrothermal method
Wang, G.-H. and Li, W.-C. and Jia, K.-M. and Lu, A.-H. and Feyen, M. and Spliethoff, B. and SchÜth, F.
NANO. Volume: 6 (2011) - 2011 • 1178
Magnetic characterization of SUS316L deformed by high pressure torsion
Wang, H.C. and Umemoto, M. and Shuro, I. and Todaka, Y. and Kuo, H.H.
ADVANCED MATERIALS RESEARCH. Volume: 239-242 (2011) - 2011 • 1177
Plasma enhanced-CVD of undoped and fluorine-doped Co3O 4 nanosystems for novel gas sensors
Barreca, D. and Bekermann, D. and Comini, E. and Devi, A. and Fischer, R.A. and Gasparotto, A. and Gavagnin, M. and MacCato, C. and Sada, C. and Sberveglieri, G. and Tondello, E.
SENSORS AND ACTUATORS, B: CHEMICAL. Volume: 160 (2011) - 2011 • 1176
Lean-alloyed austenitic stainless steel with high resistance against hydrogen environment embrittlement
Weber, S. and Martin, M. and Theisen, W.
MATERIALS SCIENCE AND ENGINEERING A. Volume: 528 (2011) - 2011 • 1175
Strongly oriented Co3O4 thin films on MgO(100) and MgAl2O4(100) substrates by PE-CVD
Barreca, D. and Devi, A. and Fischer, R.A. and Bekermann, D. and Gasparotto, A. and Gavagnin, M. and MacCato, C. and Tondello, E. and Bontempi, E. and Depero, L.E. and Sada, C.
CRYSTENGCOMM. Volume: 13 (2011) - 2011 • 1174
Critical investigation of high temperature gas nitriding of a PM tool steel
Weber, S. and Theisen, W.
INTERNATIONAL JOURNAL OF MATERIALS RESEARCH. Volume: 102 (2011) - 2011 • 1173
Small-scale deposition of thin films and nanoparticles by microevaporation sources
Meyer, R. and Hamann, S. and Ehmann, M. and König, D. and Thienhaus, S. and Savan, A. and Ludwig, Al.
JOURNAL OF MICROELECTROMECHANICAL SYSTEMS. Volume: 20 (2011) - 2011 • 1172
Electrostatically trapping indirect excitons in coupled In xGa1-xAs quantum wells
Schinner, G.J. and Schubert, E. and Stallhofer, M.P. and Kotthaus, J.P. and Schuh, D. and Rai, A.K. and Reuter, D. and Wieck, A.D. and Govorov, A.O.
PHYSICAL REVIEW B - CONDENSED MATTER AND MATERIALS PHYSICS. Volume: 83 (2011) - 2011 • 1171
Passively mode-locked two section laser diode with intracavity dispersion control
Schlauch, T. and Balzer, J.C. and Hofmann, M.R. and Klehr, A. and Erbert, G. and Tränkle, G.
PROCEEDINGS OF SPIE - THE INTERNATIONAL SOCIETY FOR OPTICAL ENGINEERING. Volume: 7937 (2011) - 2011 • 1170
Anisotropic mechanical behavior of ultrafine eutectic TiFe cast under non-equilibrium conditions
Schlieter, A. and Kühn, U. and Eckert, J. and Löser, W. and Gemming, T. and Friák, M. and Neugebauer, J.
INTERMETALLICS. Volume: 19 (2011) - 2011 • 1169
Application of a biphasic representative volume element to the simulation of wave propagation through cancellous bone
Ilic, S. and Hackl, K. and Gilbert, R.P.
JOURNAL OF COMPUTATIONAL ACOUSTICS. Volume: 19 (2011) - 2011 • 1168
A novel automated electrochemical ascorbic acid assay in the 24-well microtiter plate format
Intarakamhang, S. and Leson, C. and Schuhmann, W. and Schulte, A.
ANALYTICA CHIMICA ACTA. Volume: 687 (2011) - 2011 • 1167
A density functional theory based estimation of the anharmonic contributions to the free energy of a polypeptide helix
Ismer, L. and Ireta, J. and Neugebauer, J.
JOURNAL OF CHEMICAL PHYSICS. Volume: 135 (2011) - 2011 • 1166
Thermo-mechanical behaviour of Shape Memory Polymers, e.g., Tecoflex® by 1WE method: SEM and IR analysis
Schmidt, C. and Chowdhury, A.M.S. and Neuking, K. and Eggeler, G.
JOURNAL OF POLYMER RESEARCH. Volume: 18 (2011) - 2011 • 1165
SIMS study on the surface chemistry of stainless steel AISI 304 cylindrical tensile test samples showing hydrogen embrittlement
Izawa, C. and Wagner, S. and Martin, M. and Weber, S. and Bourgeon, A. and Pargeter, R. and Michler, T. and Pundt, A.
JOURNAL OF ALLOYS AND COMPOUNDS. Volume: 509 (2011) - 2011 • 1164
Mechanical behavior of shape memory polymers by 1we method: Application to tecoflex®
Schmidt, C. and Chowdhury, A.M.S. and Neuking, K. and Eggeler, G.
JOURNAL OF THERMOPLASTIC COMPOSITE MATERIALS. Volume: 24 (2011) - 2011 • 1163
Strong temperature destabilization of free exciton recombination in a two-dimensional structures with hole gas
Jadczak, J. and Bryja, L. and Wójs, A. and Bartsch, G. and Yakovlev, D.R. and Bayer, M. and Plochocka, P. and Potemski, M. and Reuter, D. and Wieck, A.
JOURNAL OF PHYSICS: CONFERENCE SERIES. Volume: 334 (2011) - 2011 • 1162
Studies on the cycling, processing and programming of an industrially applicable shape memory polymer Tecoflex® (or TFX EG 72D)
Schmidt, C. and Chowdhury, A.M.S. and Neuking, K. and Eggeler, G.
HIGH PERFORMANCE POLYMERS. Volume: 23 (2011) - 2011 • 1161
Light driven reactions of single physisorbed azobenzenes
Bazarnik, M. and Henzl, J. and Czajka, R. and Morgenstern, K.
CHEMICAL COMMUNICATIONS. Volume: 47 (2011) - 2011 • 1160
Stress-strain behavior of shape memory polymers by 1 WE method: Application to tecoflex®
Schmidt, C. and Chowdhury, A.M.S. and Neuking, K. and Eggeler, G.
JOURNAL OF MACROMOLECULAR SCIENCE, PART A: PURE AND APPLIED CHEMISTRY. Volume: 48 (2011) - 2011 • 1159
Spectroscopic optical coherence tomography for substance identification
Jaedicke, V. and Wiethoff, H. and Agcaer, S. and Kasseck, C. and Gerhardt, N. C. and Welp, H. and Hofmann, M. R.
OPTICAL COHERENCE TOMOGRAPHY AND COHERENCE TECHNIQUES V. Volume: 8091 (2011) - 2011 • 1158
Optimizing the synthesis of cobalt-based catalysts for the selective growth of multiwalled carbon nanotubes under industrially relevant conditions
Becker, M.J. and Xia, W. and Tessonnier, J.-P. and Blume, R. and Yao, L. and Schlögl, R. and Muhler, M.
CARBON. Volume: 49 (2011) - 2011 • 1157
Malonate complexes of dysprosium: Synthesis, characterization and application for LI-MOCVD of dysprosium containing thin films
Milanov, A.P. and Seidel, R.W. and Barreca, D. and Gasparotto, A. and Winter, M. and Feydt, J. and Irsen, S. and Becker, H.-W. and Devi, A.
DALTON TRANSACTIONS. Volume: 40 (2011) - 2011 • 1156
Photoacoustic coded excitation using periodically perfect sequences
Beckmann, M.F. and Mienkina, M.P. and Schmitz, G. and Friedrich, C.-S. and Gerhardt, N.C. and Hofmann, M.R.
IEEE INTERNATIONAL ULTRASONICS SYMPOSIUM, IUS. Volume: (2011) - 2011 • 1155
Characterization of nano-sized precipitates in a Mn-based lean maraging steel by atom probe tomography
Millán, J. and Ponge, D. and Raabe, D. and Choi, P. and Dmitrieva, O.
STEEL RESEARCH INTERNATIONAL. Volume: 82 (2011) - 2011 • 1154
Atomistic processes of dislocation generation and plastic deformation during nanoindentation
Begau, C. and Hartmaier, A. and George, E.P. and Pharr, G.M.
ACTA MATERIALIA. Volume: 59 (2011) - 2011 • 1153
Quasiparticle band offsets of semiconductor heterojunctions from a generalized marker method
Mitra, C. and Lange, B. and Freysoldt, C. and Neugebauer, J.
PHYSICAL REVIEW B - CONDENSED MATTER AND MATERIALS PHYSICS. Volume: 84 (2011) - 2011 • 1152
Nanoepitaxy using micellar nanoparticles
Behafarid, F. and Roldan Cuenya, B.
NANO LETTERS. Volume: 11 (2011) - 2011 • 1151
Effect of heat-treatment on grain growth of nanocrystalline tricalcium phosphate powder synthesized via the precipitation method
Mobasherpour, I. and Salahi, E. and Manafi, S.A. and Kamachali, R.D.
MATERIALS SCIENCE- POLAND. Volume: 29 (2011) - 2011 • 1150
Neural network potential-energy surfaces in chemistry: A tool for large-scale simulations
Behler, J.
PHYSICAL CHEMISTRY CHEMICAL PHYSICS. Volume: 13 (2011) - 2011 • 1149
Atom-centered symmetry functions for constructing high-dimensional neural network potentials
Behler, J.
JOURNAL OF CHEMICAL PHYSICS. Volume: 134 (2011) - 2011 • 1148
Differential amplifier characterization using mixed-mode scattering parameters obtained from true and virtual differential measurements
Schmitz, O. and Hampel, S.K. and Rabe, H. and Reinecke, T. and Rolfes, I.
IEEE TRANSACTIONS ON MICROWAVE THEORY AND TECHNIQUES. Volume: 59 (2011) - 2011 • 1147
Knowledge-based development of a nitrate-free synthesis route for Cu/ZnO methanol synthesis catalysts via formate precursors
Behrens, M. and Kißner, S. and Girsgdies, F. and Kasatkin, I. and Hermerschmidt, F. and Mette, K. and Ruland, H. and Muhler, M. and Schlögl, R.
CHEMICAL COMMUNICATIONS. Volume: 47 (2011) - 2011 • 1146
Fastly tunable external-cavity diode-lasers controlled by electro-absorption modulators
Jähme, H. and Bülters, M. and Jäger, D. and Hofmann, M.
2011 CONFERENCE ON LASERS AND ELECTRO-OPTICS EUROPE AND 12TH EUROPEAN QUANTUM ELECTRONICS CONFERENCE, CLEO EUROPE/EQEC 2011. Volume: (2011) - 2011 • 1145
Understanding the complexity of a catalyst synthesis: Co-precipitation of mixed Cu,Zn,Al hydroxycarbonate precursors for Cu/ZnO/Al2O 3 catalysts investigated by titration experiments
Behrens, M. and Brennecke, D. and Girgsdies, F. and Kißner, S. and Trunschke, A. and Nasrudin, N. and Zakaria, S. and Idris, N.F. and Hamid, S.B.A. and Kniep, B. and Fischer, R. and Busser, W. and Muhler, M. and Schlögl, R.
APPLIED CATALYSIS A: GENERAL. Volume: 392 (2011) - 2011 • 1144
MOCVD of ZnO films from bis(ketoiminato)Zn(II) precursors: Structure, morphology and optical properties
Bekermann, D. and Ludwig, Ar. and Toader, T. and MacCato, C. and Barreca, D. and Gasparotto, A. and Bock, C. and Wieck, A.D. and Kunze, U. and Tondello, E. and Fischer, R.A. and Devi, A.
CHEMICAL VAPOR DEPOSITION. Volume: 17 (2011) - 2011 • 1143
Contact angle dependence of the velocity of sliding cylindrical drop on flat substrates
Moradi, N. and Varnik, F. and Steinbach, I.
EPL. Volume: 95 (2011) - 2011 • 1142
Integration of 3-D cell cultures in fluidic microsystems for biological screenings
Witte, H. and Stubenrauch, M. and Fröber, U. and Fischer, R. and Voges, D. and Hoffmann, M.
ENGINEERING IN LIFE SCIENCES. Volume: 11 (2011) - 2011 • 1141
p-Co3O4/n-ZnO, Obtained by PECVD, Analyzed by X-ray Photoelectron Spectroscopy
Bekermann, D. and Gasparotto, A. and Barreca, D. and Devi, A. and Fischer, R.A.
SURFACE SCIENCE SPECTRA. Volume: 18 (2011) - 2011 • 1140
Morphologies of small droplets on patterned hydrophobic substrates
Moradi, N. and Gross, M. and Varnik, F. and Zikos, G. and Steinbach, I.
MODELLING AND SIMULATION IN MATERIALS SCIENCE AND ENGINEERING. Volume: 19 (2011) - 2011 • 1139
Combined multifrequency EPR and DFT study of dangling bonds in a-Si:H
Fehr, M. and Schnegg, A. and Rech, B. and Lips, K. and Astakhov, O. and Finger, F. and Pfanner, G. and Freysoldt, C. and Neugebauer, J. and Bittl, R. and Teutloff, C.
PHYSICAL REVIEW B - CONDENSED MATTER AND MATERIALS PHYSICS. Volume: 84 (2011) - 2011 • 1138
On the interpretation of IETS spectra of a small organic molecule
Morgenstern, K.
JOURNAL OF PHYSICS CONDENSED MATTER. Volume: 23 (2011) - 2011 • 1137
The Thermophilic CotA Laccase from Bacillus subtilis: Bioelectrocatalytic Evaluation of O2 Reduction in the Direct and Mediated Electron Transfer Regime
Beneyton, T. and Beyl, Y. and Guschin, D.A. and Griffiths, A.D. and Taly, V. and Schuhmann, W.
ELECTROANALYSIS. Volume: 23 (2011) - 2011 • 1136
Switching individual molecules by light and electrons: From isomerisation to chirality flip
Morgenstern, K.
PROGRESS IN SURFACE SCIENCE. Volume: 86 (2011) - 2011 • 1135
Modification of the bilateral sagittal split osteotomy (BSSO) in a study using pig mandibles
Schoen, P. and Frotscher, M. and Eggeler, G. and Kessler, P. and Wolff, K.-D. and Boeckmann, R.
INTERNATIONAL JOURNAL OF ORAL AND MAXILLOFACIAL SURGERY. Volume: 40 (2011) - 2011 • 1134
Structural and magnetic characterization of self-assembled iron oxide nanoparticle arrays
Benitez, M.J. and Mishra, D. and Szary, P. and Badini Confalonieri, G.A. and Feyen, M. and Lu, A.H. and Agudo, L. and Eggeler, G. and Petracic, O. and Zabel, H.
JOURNAL OF PHYSICS CONDENSED MATTER. Volume: 23 (2011) - 2011 • 1133
Size effects and stochastic behavior of nanoindentation pop in
Morris, J.R. and Bei, H. and Pharr, G.M. and George, E.P.
PHYSICAL REVIEW LETTERS. Volume: 106 (2011) - 2011 • 1132
Fingerprinting the magnetic behavior of antiferromagnetic nanostructures using remanent magnetization curves
Benitez, M.J. and Petracic, O. and Tüysüz, H. and Schüth, F. and Zabel, H.
PHYSICAL REVIEW B - CONDENSED MATTER AND MATERIALS PHYSICS. Volume: 83 (2011) - 2011 • 1131
Approximation of random microstructures by periodic statistically similar representative volume elements based on lineal-path functions
Schröder, J. and Balzani, D. and Brands, D.
ARCHIVE OF APPLIED MECHANICS. Volume: 81 (2011) - 2011 • 1130
High-temperature stable, iron-based core-shell catalysts for ammonia decomposition
Feyen, M. and Weidenthaler, C. and Güttel, R. and Schlichte, K. and Holle, U. and Lu, A.-H. and Schüth, F.
CHEMISTRY - A EUROPEAN JOURNAL. Volume: 17 (2011) - 2011 • 1129
Grain boundary electrochemistry of β-type Nb-Ti alloy using a scanning droplet cell
Woldemedhin, M.T. and Raabe, D. and Hassel, A.W.
PHYSICA STATUS SOLIDI (A) APPLICATIONS AND MATERIALS SCIENCE. Volume: 208 (2011) - 2011 • 1128
Analytic many-body potential for GaAs(001) homoepitaxy: Bulk and surface properties
Fichthorn, K.A. and Tiwary, Y. and Hammerschmidt, T. and Kratzer, P. and Scheffler, M.
PHYSICAL REVIEW B - CONDENSED MATTER AND MATERIALS PHYSICS. Volume: 83 (2011) - 2011 • 1127
Co3O4 - SiO2 Nanocomposite: A very active catalyst for co oxidation with unusual catalytic behavior
Jia, C.-J. and Schwickardi, M. and Weidenthaler, C. and Schmidt, W. and Korhonen, S. and Weckhuysen, B.M. and Schüth, F.
JOURNAL OF THE AMERICAN CHEMICAL SOCIETY. Volume: 133 (2011) - 2011 • 1126
A new mixed finite element based on different approximations of the minors of deformation tensors
Schröder, J. and Wriggers, P. and Balzani, D.
COMPUTER METHODS IN APPLIED MECHANICS AND ENGINEERING. Volume: 200 (2011) - 2011 • 1125
Ignition of a microcavity plasma array
Wollny, A. and Hemke, T. and Gebhardt, M. and Brinkmann, R.P. and Mussenbrock, T.
IEEE TRANSACTIONS ON PLASMA SCIENCE. Volume: 39 (2011) - 2011 • 1124
Temperature measurements at thoriated tungsten electrodes in a model lamp and their interpretation by numerical simulation
Bergner, A. and Westermeier, M. and Ruhrmann, C. and Awakowicz, P. and Mentel, J.
JOURNAL OF PHYSICS D: APPLIED PHYSICS. Volume: 44 (2011) - 2011 • 1123
Colloidal metal nanoparticles as a component of designed catalyst
Jia, C.-J. and Schüth, F.
PHYSICAL CHEMISTRY CHEMICAL PHYSICS. Volume: 13 (2011) - 2011 • 1122
Magnetic bond-order potential for iron
Mrovec, M. and Nguyen-Manh, D. and Elsässer, C. and Gumbsch, P.
PHYSICAL REVIEW LETTERS. Volume: 106 (2011) - 2011 • 1121
Ionization wave propagation on a micro cavity plasma array
Wollny, A. and Hemke, T. and Gebhardt, M. and Peter Brinkmann, R. and Boettner, H. and Winter, J. and Schulz-Von Der Gathen, V. and Xiong, Z. and Kushner, M.J. and Mussenbrock, T.
APPLIED PHYSICS LETTERS. Volume: 99 (2011) - 2011 • 1120
Polythiophene-assisted vapor phase synthesis of carbon nanotube-supported rhodium sulfide as oxygen reduction catalyst for HCl electrolysis
Jin, C. and Nagaiah, T.C. and Xia, W. and Bron, M. and Schuhmann, W. and Muhler, M.
CHEMSUSCHEM. Volume: 4 (2011) - 2011 • 1119
Enforcing mass conservation in DPM-CFD models of dense particulate flows
Wu, C.L. and Nandakumar, K. and Berrouk, A.S. and Kruggel-Emden, H.
CHEMICAL ENGINEERING JOURNAL. Volume: 174 (2011) - 2011 • 1118
Simultaneous acquisition of impedance and gravimetric data in a cyclic potential scan for the characterization of nonstationary electrode/electrolyte interfaces
Berkes, B.B. and Maljusch, A. and Schuhmann, W. and Bondarenko, A.S.
JOURNAL OF PHYSICAL CHEMISTRY C. Volume: 115 (2011) - 2011 • 1117
Chemical compounds for energy storage
Schüth, F.
CHEMIE-INGENIEUR-TECHNIK. Volume: 83 (2011) - 2011 • 1116
Finite element simulations of poly-crystalline shape memory alloys based on a micromechanical model
Junker, P. and Hackl, K.
COMPUTATIONAL MECHANICS. Volume: 47 (2011) - 2011 • 1115
Controlled nanostructures for applications in catalysis
Schüth, F.
PHYSICAL CHEMISTRY CHEMICAL PHYSICS. Volume: 13 (2011) - 2011 • 1114
Experimental and numerical study on geometrically necessary dislocations and non-homogeneous mechanical properties of the ferrite phase in dual phase steels
Kadkhodapour, J. and Schmauder, S. and Raabe, D. and Ziaei-Rad, S. and Weber, U. and Calcagnotto, M.
ACTA MATERIALIA. Volume: 59 (2011) - 2011 • 1113
TiO 2 coating of high surface area silica gel by chemical vapor deposition of TiCl 4 in a fluidized-bed reactor
Xia, W. and Mei, B. and Sánchez, M.D. and Strunk, J. and Muhler, M.
JOURNAL OF NANOSCIENCE AND NANOTECHNOLOGY. Volume: 11 (2011) - 2011 • 1112
Dynamic plumbing system beneath volcanoes revealed by kinetic modeling, and the connection to monitoring data: An example from Mt. Etna
Kahl, M. and Chakraborty, S. and Costa, F. and Pompilio, M.
EARTH AND PLANETARY SCIENCE LETTERS. Volume: 308 (2011) - 2011 • 1111
Highly active metal-free nitrogen-containing carbon catalysts for oxygen reduction synthesized by thermal treatment of polypyridine-carbon black mixtures
Xia, W. and Masa, J. and Bron, M. and Schuhmann, W. and Muhler, M.
ELECTROCHEMISTRY COMMUNICATIONS. Volume: 13 (2011) - 2011 • 1110
Visualization and functions of surface defects on carbon nanotubes created by catalytic etching
Xia, W. and Yin, X. and Kundu, S. and Sánchez, M. and Birkner, A. and Wöll, C. and Muhler, M.
CARBON. Volume: 49 (2011) - 2011 • 1109
Impact of ceramic membranes for CO2 separation on IGCC power plant performance
Franz, J. and Scherer, V.
ENERGY PROCEDIA. Volume: 4 (2011) - 2011 • 1108
Structure and flow of droplets on solid surfaces
Müller-Buschbaum, P. and Magerl, D. and Hengstler, R. and Moulin, J.-F. and Körstgens, V. and Diethert, A. and Perlich, J. and Roth, S.V. and Burghammer, M. and Riekel, C. and Gross, M. and Varnik, F. and Uhlmann, P. and Stamm, M. and Feldkamp, J.M. and Schroer, C.G.
JOURNAL OF PHYSICS CONDENSED MATTER. Volume: 23 (2011) - 2011 • 1107
Interlayer-expanded microporous titanosilicate catalysts with functionalized hydroxyl groups
Xiao, F.-S. and Xie, B. and Zhang, H. and Wang, L. and Meng, X. and Zhang, W. and Bao, X. and Yilmaz, B. and Müller, U. and Gies, H. and Imai, H. and Tatsumi, T. and DeVos, D.
CHEMCATCHEM. Volume: 3 (2011) - 2011 • 1106
A Novel Synthesis Route for Cu/ZnO/Al 2O 3 Catalysts used in Methanol Synthesis: Combining Continuous Consecutive Precipitation with Continuous Aging of the Precipitate
Kaluza, S. and Behrens, M. and Schiefenhövel, N. and Kniep, B. and Fischer, R. and Schlögl, R. and Muhler, M.
CHEMCATCHEM. Volume: 3 (2011) - 2011 • 1105
Picosecond laser direct patterning of poly (3,4-ethylene dioxythiophene)-poly (styrene sulfonate) (PEDOT:PSS) thin films
Xiao, S. and Abreu Fernandes, S. and Esen, C. and Ostendorf, A.
JOURNAL OF LASER MICRO NANOENGINEERING. Volume: 6 (2011) - 2011 • 1104
A chloride resistant high potential oxygen reducing biocathode based on a fungal laccase incorporated into an optimized Os-complex modified redox hydrogel
Beyl, Y. and Guschin, D.A. and Shleev, S. and Schuhmann, W.
ELECTROCHEMISTRY COMMUNICATIONS. Volume: 13 (2011) - 2011 • 1103
Model catalysts for the selective oxidation of propene probed by fast redox cycling
Franzke, T. and Rosowski, F. and Muhler, M.
CHEMIE-INGENIEUR-TECHNIK. Volume: 83 (2011) - 2011 • 1102
Reply to "comment on 'Paramagnetic and ferromagnetic resonance studies on dilute magnetic semiconductors based on GaN' " [Phys. Status Solidi A 205, 1872 (2008)]
Kammermeier, T. and Ney, A. and Wieck, A.D.
PHYSICA STATUS SOLIDI (A) APPLICATIONS AND MATERIALS SCIENCE. Volume: 208 (2011) - 2011 • 1101
A broadband stacked patch antenna with enhanced antenna gain by an optimized ellipsoidal reflector for X-band applications
Schulz, C. and Baer, C. and Musch, T. and Rolfes, I.
2011 IEEE INTERNATIONAL CONFERENCE ON MICROWAVES, COMMUNICATIONS, ANTENNAS AND ELECTRONIC SYSTEMS, COMCAS 2011. Volume: (2011) - 2011 • 1100
Selective patterning of ITO on flexible PET Substrate by 1064nm picosecond Laser
Xiao, S. and Fernandes, S.A. and Ostendorf, A.
PHYSICS PROCEDIA. Volume: 12 (2011) - 2011 • 1099
Atmospheric plasma sprayed thermal barrier coatings with high segmentation crack densities: Spraying process, microstructure and thermal cycling behavior
Karger, M. and Vaßen, R. and Stöver, D.
SURFACE AND COATINGS TECHNOLOGY. Volume: 206 (2011) - 2011 • 1098
Laser selective patterning of ITO on flexible PET for organic photovoltaics
Xiao, S. and Gröger, B. and Fernandes, S.A. and Ostendorf, A.
PROCEEDINGS OF SPIE - THE INTERNATIONAL SOCIETY FOR OPTICAL ENGINEERING. Volume: 7921 (2011) - 2011 • 1097
Improvement of NiTi shape memory actuator performance through ultra-fine grained and nanocrystalline microstructures
Frenzel, J. and Burow, J.A. and Payton, E.J. and Rezanka, S. and Eggeler, G.
ADVANCED ENGINEERING MATERIALS. Volume: 13 (2011) - 2011 • 1096
Hot Direct Extrusion of Abrasion Resistant Fe-Base Metal Matrix Composites-Microstructure and Wear Properties
Karlsohn, M. and Röttger, A. and Silva, P.A. and Weber, S. and Pyzalla, R. and Reimers, W. and Theisen, W.
FRICTION, WEAR AND WEAR PROTECTION: INTERNATIONAL SYMPOSIUM ON FRICTION, WEAR AND WEAR PROTECTION 2008 AACHEN, GERMANY. Volume: (2011) - 2011 • 1095
Possible routes for synthesis of new boron-rich Fe-B and Fe1-x Crx B4 compounds
Bialon, A.F. and Hammerschmidt, T. and Drautz, R. and Shah, S. and Margine, E.R. and Kolmogorov, A.N.
APPLIED PHYSICS LETTERS. Volume: 98 (2011) - 2011 • 1094
Optical Excitations in CdSe/CdS Core-Shell Nanoparticles
Frenzel, J. and Thieme, S. and Seifert, G. and Joswig, J. O.
JOURNAL OF PHYSICAL CHEMISTRY C. Volume: 115 (2011) - 2011 • 1093
Particlehole asymmetry as a source of phase separation at the metalinsulator transition
Karnaukhov, I.N. and Eremin, I.
JOURNAL OF PHYSICS A: MATHEMATICAL AND THEORETICAL. Volume: 44 (2011) - 2011 • 1092
Silicon and magnesium diffusion in a single crystal of MgSiO3 perovskite
Xu, J. and Yamazaki, D. and Katsura, T. and Wu, X. and Remmert, P. and Yurimoto, H. and Chakraborty, S.
JOURNAL OF GEOPHYSICAL RESEARCH: SOLID EARTH. Volume: 116 (2011) - 2011 • 1091
Spectroscopic characterization of an atmospheric pressure μ-jet plasma source
Bibinov, N. and Knake, N. and Bahre, H. and Awakowicz, P. and Schulz-Von Der Gathen, V.
JOURNAL OF PHYSICS D: APPLIED PHYSICS. Volume: 44 (2011) - 2011 • 1090
Electrostatic Interactions between Charged Defects in Supercells
Freysoldt, C. and Neugebauer, J. and Van de Walle, C.G.
ADVANCED CALCULATIONS FOR DEFECTS IN MATERIALS: ELECTRONIC STRUCTURE METHODS. Volume: (2011) - 2011 • 1089
Photocatalytic activity of bulk TiO2 anatase and rutile single crystals using infrared absorption spectroscopy
Xu, M. and Gao, Y. and Moreno, E.M. and Kunst, M. and Muhler, M. and Wang, Y. and Idriss, H. and Wöll, C.
PHYSICAL REVIEW LETTERS. Volume: 106 (2011) - 2011 • 1088
Optimal designs for indirect regression
Biedermann, S. and Bissantz, N. and Dette, H. and Jones, E.
INVERSE PROBLEMS. Volume: 27 (2011) - 2011 • 1087
Electrostatic interactions between charged defects in supercells
Freysoldt, C. and Neugebauer, J. and Van de Walle, C.G.
PHYSICA STATUS SOLIDI (B) BASIC RESEARCH. Volume: 248 (2011) - 2011 • 1086
Development and characterization of novel corrosion-resistant TWIP steels
Mujica, L. and Weber, S. and Hunold, G. and Theisen, W.
STEEL RESEARCH INTERNATIONAL. Volume: 82 (2011) - 2011 • 1085
Optimal design for additive partially nonlinear models
Biedermann, S. and Dette, H. and Woods, D.C.
BIOMETRIKA. Volume: 98 (2011) - 2011 • 1084
Determining the elasticity of materials employing quantum-mechanical approaches from the electronic ground state to the limits of materials stability
Friák, M. and Hickel, T. and Körmann, F. and Udyansky, A. and Dick, A. and Von Pezold, J. and Ma, D. and Kim, O. and Counts, W.A. and Šob, M. and Gebhardt, T. and Music, D. and Schneider, J. and Raabe, D. and Neugebauer, J.
STEEL RESEARCH INTERNATIONAL. Volume: 82 (2011) - 2011 • 1083
Nucleation and precipitation kinetics of M23C6 and M2N in an Fe-Mn-Cr-C-N austenitic matrix and their relationship with the sensitization phenomenon
Mújica Roncery, L. and Weber, S. and Theisen, W.
ACTA MATERIALIA. Volume: 59 (2011) - 2011 • 1082
Making a geometrically asymmetric capacitive rf discharge electrically symmetric
Schulze, J. and Schüngel, E. and Czarnetzki, U. and Gebhardt, M. and Brinkmann, R.P. and Mussenbrock, T.
APPLIED PHYSICS LETTERS. Volume: 98 (2011) - 2011 • 1081
Methodological challenges in combining quantum-mechanical and continuum approaches for materials science applications
Friák, M. and Hickel, T. and Grabowski, B. and Lymperakis, L. and Udyansky, A. and Dick, A. and Ma, D. and Roters, F. and Zhu, L.-F. and Schlieter, A. and Kühn, U. and Ebrahimi, Z. and Lebensohn, R.A. and Holec, D. and Eckert, J. and Emmerich, H. and Raabe, D. and Neugebauer, J.
EUROPEAN PHYSICAL JOURNAL PLUS. Volume: 126 (2011) - 2011 • 1080
Influence of intermetallic precipitates and heat treatment on the mechanical properties of high-temperature corrosion resistant ferritic steels
Nabiran, N. and Weber, S. and Theisen, W.
PROCEDIA ENGINEERING. Volume: 10 (2011) - 2011 • 1079
Molecular dynamics simulation study of microstructure evolution during cyclic martensitic transformations
Kastner, O. and Eggeler, G. and Weiss, W. and Ackland, G.J.
JOURNAL OF THE MECHANICS AND PHYSICS OF SOLIDS. Volume: 59 (2011) - 2011 • 1078
Structural characteristics and catalytic performance of alumina-supported nanosized ceria-lanthana solid solutions
Katta, L. and Thrimurthulu, G. and Reddy, B.M. and Muhler, M. and Grünert, W.
CATALYSIS SCIENCE AND TECHNOLOGY. Volume: 1 (2011) - 2011 • 1077
Grout and bentonite flow around a TBM: Computational modeling and simulation-based assessment of influence on surface settlements
Nagel, F. and Meschke, G.
TUNNELLING AND UNDERGROUND SPACE TECHNOLOGY. Volume: 26 (2011) - 2011 • 1076
Local electrocatalytic induction of sol-gel deposition at Pt nanoparticles
Schwamborn, S. and Etienne, M. and Schuhmann, W.
ELECTROCHEMISTRY COMMUNICATIONS. Volume: 13 (2011) - 2011 • 1075
Quantitative photoacoustic blood oxygenation measurement of whole porcine blood samples using a multi-wavelength semiconductor laser system
Friedrich, C. S. and Mienkina, M. P. and Brenner, C. and Gerhardt, N. C. and Jorger, M. and Strauss, A. and Beckmann, M. F. and Schmitz, G. and Hofmann, M. R.
DIFFUSE OPTICAL IMAGING III. Volume: 8088 (2011) - 2011 • 1074
Modelling Shear Fracture of Hybrid CFRP/Ti Laminates with Cohesive Elements; Effects of Geometry and Material Properties
Naghipour, P. and Bartsch, M. and Hausmann, J. and Schulze, K.
SUPPLEMENTAL PROCEEDINGS: MATERIALS FABRICATION, PROPERTIES, CHARACTERIZATION, AND MODELING. Volume: 2 (2011) - 2011 • 1073
Activation/inhibition effects during the coelectrodeposition of PtAg nanoparticles: Application for ORR in alkaline media
Schwamborn, S. and Stoica, L. and Schuhmann, W.
CHEMPHYSCHEM. Volume: 12 (2011) - 2011 • 1072
Simulation and experimental validation of mixed mode delamination in multidirectional CF/PEEK laminates under fatigue loading
Naghipour, P. and Bartsch, M. and Voggenreiter, H.
INTERNATIONAL JOURNAL OF SOLIDS AND STRUCTURES. Volume: 48 (2011) - 2011 • 1071
Probing the Pt Surface for Oxygen Reduction by Insertion of Ag
Schwamborn, S. and Bron, M. and Schuhmann, W.
ELECTROANALYSIS. Volume: 23 (2011) - 2011 • 1070
Computational thermodynamics, an overview
Fries, S.G.
EPJ WEB OF CONFERENCES. Volume: 14 (2011) - 2011 • 1069
Resistively detected nuclear magnetic resonance in n- and p-type GaAs quantum point contacts
Keane, Z.K. and Godfrey, M.C. and Chen, J.C.H. and Fricke, S. and Klochan, O. and Burke, A.M. and Micolich, A.P. and Beere, H.E. and Ritchie, D.A. and Trunov, K.V. and Reuter, D. and Wieck, A.D. and Hamilton, A.R.
NANO LETTERS. Volume: 11 (2011) - 2011 • 1068
Exttrapolations from thermodynamic models
Fries, S.G.
EPJ WEB OF CONFERENCES. Volume: 14 (2011) - 2011 • 1067
Effect of shot-peening on the oxidation behaviour of boiler steels
Naraparaju, R. and Christ, H.-J. and Renner, F.U. and Kostka, A.
OXIDATION OF METALS. Volume: 76 (2011) - 2011 • 1066
Nanoindentation study of elastic anisotropy of Cu single crystals and grains in TSVs
Yeap, K.B. and Hangen, U.D. and Raabe, D. and Zschech, E.
AIP CONFERENCE PROCEEDINGS. Volume: 1378 (2011) - 2011 • 1065
Growth of epitaxial sodium-bismuth-titanate films by metal-organic chemical vapor phase deposition
Schwarzkopf, J. and Schmidbauer, M. and Duk, A. and Kwasniewski, A. and Anooz, S.B. and Wagner, G. and Devi, A. and Fornari, R.
THIN SOLID FILMS. Volume: 520 (2011) - 2011 • 1064
Al-RUB-41: A shape-selective zeolite catalyst from a layered silicate
Yilmaz, B. and Müller, U. and Tijsebaert, B. and Vos, D.D. and Xie, B. and Xiao, F.-S. and Gies, H. and Zhang, W. and Bao, X. and Imai, H. and Tatsumi, T.
CHEMICAL COMMUNICATIONS. Volume: 47 (2011) - 2011 • 1063
Achieving small structures in thin NiTi sheets for medical applications with water jet and micro machining: A comparison
Frotscher, M. and Kahleyss, F. and Simon, T. and Biermann, D. and Eggeler, G.
JOURNAL OF MATERIALS ENGINEERING AND PERFORMANCE. Volume: 20 (2011) - 2011 • 1062
Influence of Na on the structure of Bi4Ti3O 12 films deposited by liquid-delivery spin MOCVD
Schwarzkopf, J. and Dirsyte, R. and Devi, A. and Kwasniewski, A. and Schmidbauer, M. and Wagner, G. and Michling, M. and Schmeisser, D. and Fornari, R.
THIN SOLID FILMS. Volume: 519 (2011) - 2011 • 1061
Processing and characterization of braided NiTi microstents for medical applications
Frotscher, M. and Schreiber, F. and Neelakantan, L. and Gries, T. and Eggeler, G.
MATERIALWISSENSCHAFT UND WERKSTOFFTECHNIK. Volume: 42 (2011) - 2011 • 1060
Elementary deformation and damage mechanisms during fatigue of pseudoelastic NiTi microstents
Frotscher, M. and Wu, S. and Simon, T. and Somsen, C. and Dlouhy, A. and Eggeler, G.
ADVANCED ENGINEERING MATERIALS. Volume: 13 (2011) - 2011 • 1059
Liquid-phase epitaxy of multicomponent layer-based porous coordination polymer thin films of [M(L)(P)0.5] type: Importance of deposition sequence on the oriented growth
Zacher, D. and Yusenko, K. and Bétard, A. and Henke, S. and Molon, M. and Ladnorg, T. and Shekhah, O. and Schüpbach, B. and Dea Losa Arcos, T. and Krasnopolski, M. and Meilikhov, M. and Winter, J. and Terfort, A. and Wöll, C. and Fischer, R.A.
CHEMISTRY - A EUROPEAN JOURNAL. Volume: 17 (2011) - 2011 • 1058
Surface chemistry of metal-organic frameworks at the liquid-solid interface
Zacher, D. and Schmid, R. and Wöll, C. and Fischer, R.A.
ANGEWANDTE CHEMIE - INTERNATIONAL EDITION. Volume: 50 (2011) - 2011 • 1057
Nucleation mechanism for the direct graphite-to-diamond phase transition
Khaliullin, R.Z. and Eshet, H. and Kühne, T.D. and Behler, J. and Parrinello, M.
NATURE MATERIALS. Volume: 10 (2011) - 2011 • 1056
Analysis of the plastic anisotropy and pre-yielding of (γ/ α2)-phase titanium aluminide microstructures by crystal plasticity simulation
Zambaldi, C. and Roters, F. and Raabe, D.
INTERMETALLICS. Volume: 19 (2011) - 2011 • 1055
Yolk-shell gold nanoparticles as model materials for support-effect studies in heterogeneous catalysis: Au, @C and Au, @ZrO2 for CO oxidation as an example
Galeano, C. and Güttel, R. and Paul, M. and Arnal, P. and Lu, A.-H. and Schüth, F.
CHEMISTRY - A EUROPEAN JOURNAL. Volume: 17 (2011) - 2011 • 1054
High-throughput characterization of mechanical properties of Ti-Ni-Cu shape memory thin films at elevated temperature
Zarnetta, R. and Kneip, S. and Somsen, C. and Ludwig, Al.
MATERIALS SCIENCE AND ENGINEERING A. Volume: 528 (2011) - 2011 • 1053
Isolated silicon dangling bonds on a water-saturated n+-doped Si(001)-2 × 1 surface: An XPS and STM study
Gallet, J.-J. and Bournel, F. and Rochet, F. and Köhler, U. and Kubsky, S. and Silly, M.G. and Sirotti, F. and Pierucci, D.
JOURNAL OF PHYSICAL CHEMISTRY C. Volume: 115 (2011) - 2011 • 1052
Anomalous scaling in heteroepitaxial island dynamics on Ag(100)
Zaum, C. and Rieger, M. and Reuter, K. and Morgenstern, K.
PHYSICAL REVIEW LETTERS. Volume: 107 (2011) - 2011 • 1051
Recrystallization and grain growth in ultrafine-grained materials produced by high pressure torsion
Khorashadizadeh, A. and Raabe, D. and Winning, M. and Pippan, R.
ADVANCED ENGINEERING MATERIALS. Volume: 13 (2011) - 2011 • 1050
Visualization of chlorine evolution at dimensionally stable anodes by means of scanning electrochemical microscopy
Zeradjanin, A.R. and Schilling, T. and Seisel, S. and Bron, M. and Schuhmann, W.
ANALYTICAL CHEMISTRY. Volume: 83 (2011) - 2011 • 1049
Five-parameter grain boundary analysis by 3D EBSD of an ultra fine grained CuZr alloy processed by equal channel angular pressing
Khorashadizadeh, A. and Raabe, D. and Zaefferer, S. and Rohrer, G.S. and Rollett, A.D. and Winning, M.
ADVANCED ENGINEERING MATERIALS. Volume: 13 (2011) - 2011 • 1048
Theory of structural trends within 4d and 5d transition metal topologically close-packed phases
Seiser, B. and Hammerschmidt, T. and Kolmogorov, A.N. and Drautz, R. and Pettifor, D.G.
PHYSICAL REVIEW B - CONDENSED MATTER AND MATERIALS PHYSICS. Volume: 83 (2011) - 2011 • 1047
Superplastic martensitic Mn-Si-Cr-C steel with 900% elongation
Zhang, H. and Bai, B. and Raabe, D.
ACTA MATERIALIA. Volume: 59 (2011) - 2011 • 1046
Testing symmetry of a nonparametric bivariate regression function
Birke, M. and Dette, H. and Stahljans, K.
JOURNAL OF NONPARAMETRIC STATISTICS. Volume: 23 (2011) - 2011 • 1045
Work hardening in micropillar compression: In situ experiments and modeling
Kiener, D. and Guruprasad, P.J. and Keralavarma, S.M. and Dehm, G. and Benzerga, A.A.
ACTA MATERIALIA. Volume: 59 (2011) - 2011 • 1044
TCP phase predictions in Ni-based superalloys: Structure maps revisited
Seiser, B. and Drautz, R. and Pettifor, D.G.
ACTA MATERIALIA. Volume: 59 (2011) - 2011 • 1043
Phase-field simulation of diffusion couples in the Ni-Al system
Zhang, L. and Steinbach, I. and Du, Y.
INTERNATIONAL JOURNAL OF MATERIALS RESEARCH. Volume: 102 (2011) - 2011 • 1042
Review of new developments in suspension and solution precursor thermal spray processes
Killinger, A. and Gadow, R. and Mauer, G. and Guignard, A. and Vaßen, R. and Stöver, D.
JOURNAL OF THERMAL SPRAY TECHNOLOGY. Volume: 20 (2011) - 2011 • 1041
Development of graded Ni-YSZ composite coating on Alloy 690 by Pulsed Laser Deposition technique to reduce hazardous metallic nuclear waste inventory
Sengupta, P. and Rogalla, D. and Becker, H.W. and Dey, G.K. and Chakraborty, S.
JOURNAL OF HAZARDOUS MATERIALS. Volume: 192 (2011) - 2011 • 1040
Preliminary study on calcium aluminosilicate glass as a potential host matrix for radioactive 90Sr-An approach based on natural analogue study
Sengupta, P. and Fanara, S. and Chakraborty, S.
JOURNAL OF HAZARDOUS MATERIALS. Volume: 190 (2011) - 2011 • 1039
Investigations of Wear Mechanisms in Diamond Tools with Fe-Based Matrices Reinforced with WC-Co Particles
Garcia, J. and Weber, S. and Kostka, A. and Pyzalla, A. and Garcia, L.F. and Lammer, A.
FRICTION, WEAR AND WEAR PROTECTION: INTERNATIONAL SYMPOSIUM ON FRICTION, WEAR AND WEAR PROTECTION 2008 AACHEN, GERMANY. Volume: (2011) - 2011 • 1038
In-plane anisotropy of electrical resistivity in strain-detwinned SrFe 2As2
Blomberg, E.C. and Tanatar, M.A. and Kreyssig, A. and Ni, N. and Thaler, A. and Hu, R. and Bud'Ko, S.L. and Canfield, P.C. and Goldman, A.I. and Prozorov, R.
PHYSICAL REVIEW B - CONDENSED MATTER AND MATERIALS PHYSICS. Volume: 83 (2011) - 2011 • 1037
Magnetic ordering and structural distortion in Ru-doped BaFe 2As2 single crystals studied by neutron and x-ray diffraction
Kim, M.G. and Pratt, D.K. and Rustan, G.E. and Tian, W. and Zarestky, J.L. and Thaler, A. and Bud'Ko, S.L. and Canfield, P.C. and McQueeney, R.J. and Kreyssig, A. and Goldman, A.I.
PHYSICAL REVIEW B - CONDENSED MATTER AND MATERIALS PHYSICS. Volume: 83 (2011) - 2011 • 1036
Spatial dynamics of helium metastables in sheath or bulk dominated rf micro-plasma jets
Niermann, B. and Hemke, T. and Babaeva, N.Y. and Böke, M. and Kushner, M.J. and Mussenbrock, T. and Winter, J.
JOURNAL OF PHYSICS D: APPLIED PHYSICS. Volume: 44 (2011) - 2011 • 1035
Hydrogen storage properties of nanostructured MgH2/TiH 2 composite prepared by ball milling under high hydrogen pressure
Shao, H. and Felderhoff, M. and Schüth, F.
INTERNATIONAL JOURNAL OF HYDROGEN ENERGY. Volume: 36 (2011) - 2011 • 1034
F-doped Co3O4 photocatalysts for sustainable H 2 generation from water/ethanol
Gasparotto, A. and Barreca, D. and Bekermann, D. and Devi, A. and Fischer, R.A. and Fornasiero, P. and Gombac, V. and Lebedev, O.I. and MacCato, C. and Montini, T. and Van Tendeloo, G. and Tondello, E.
JOURNAL OF THE AMERICAN CHEMICAL SOCIETY. Volume: 133 (2011) - 2011 • 1033
Character of the structural and magnetic phase transitions in the parent and electron-doped BaFe2As2 compounds
Kim, M.G. and Fernandes, R.M. and Kreyssig, A. and Kim, J.W. and Thaler, A. and Bud'Ko, S.L. and Canfield, P.C. and McQueeney, R.J. and Schmalian, J. and Goldman, A.I.
PHYSICAL REVIEW B - CONDENSED MATTER AND MATERIALS PHYSICS. Volume: 83 (2011) - 2011 • 1032
Nanostructured Ti-catalyzed MgH2 for hydrogen storage
Shao, H. and Felderhoff, M. and Schüth, F. and Weidenthaler, C.
NANOTECHNOLOGY. Volume: 22 (2011) - 2011 • 1031
Martensitic transformation in rapidly solidified Heusler Ni 49Mn39Sn12 ribbons
Zheng, H. and Wu, D. and Xue, S. and Frenzel, J. and Eggeler, G. and Zhai, Q.
ACTA MATERIALIA. Volume: 59 (2011) - 2011 • 1030
Plasma processing of nanomaterials: Emerging technologies for sensing and energy applications
Gasparotto, A. and Barreca, D. and Bekermann, D. and Devi, A. and Fischer, R.A. and MacCato, C. and Tondello, E.
JOURNAL OF NANOSCIENCE AND NANOTECHNOLOGY. Volume: 11 (2011) - 2011 • 1029
Deformation resistance in the transition from coarse-grained to ultrafine-grained Cu by severe plastic deformation up to 24 passes of ECAP
Blum, W. and Li, Y.J. and Zhang, Y. and Wang, J.T.
MATERIALS SCIENCE AND ENGINEERING A. Volume: 528 (2011) - 2011 • 1028
Electronic structure of 1/6〈202̄3〉 partial dislocations in wurtzite GaN
Kioseoglou, J. and Kalesaki, E. and Lymperakis, L. and Neugebauer, J. and Komninou, Ph. and Karakostas, Th.
JOURNAL OF APPLIED PHYSICS. Volume: 109 (2011) - 2011 • 1027
Robustness and optimal use of design principles of arthropod exoskeletons studied by ab initio-based multiscale simulations
Nikolov, S. and Fabritius, H. and Petrov, M. and Friák, M. and Lymperakis, L. and Sachs, C. and Raabe, D. and Neugebauer, J.
JOURNAL OF THE MECHANICAL BEHAVIOR OF BIOMEDICAL MATERIALS. Volume: 4 (2011) - 2011 • 1026
Real-space imaging of inelastic Friedel-like surface oscillations emerging from molecular adsorbates
Gawronski, H. and Fransson, J. and Morgenstern, K.
NANO LETTERS. Volume: 11 (2011) - 2011 • 1025
Anomalous elastic behavior of relaxor ferroelectric Ca 0.28Ba 0.72Nb 2O 6:Ce studied by resonant ultrasound spectroscopy
Shekhar Pandey, C. and Schreuer, J. and Burianek, M. and Mühlberg, M.
APPLIED PHYSICS LETTERS. Volume: 99 (2011) - 2011 • 1024
PEGylated hollow mesoporous silica nanoparticles as potential drug delivery vehicles
Zhu, Y. and Fang, Y. and Borchardt, L. and Kaskel, S.
MICROPOROUS AND MESOPOROUS MATERIALS. Volume: 141 (2011) - 2011 • 1023
Amplified detection of DNA hybridization using post-labelling with a biotin-modified intercalator
Gbala, M. and Hartwich, G. and Schuhmann, W.
FARADAY DISCUSSIONS. Volume: 149 (2011) - 2011 • 1022
In situ μlaue: Instrumental setup for the deformation of micron sized samples
Kirchlechner, C. and Keckes, J. and Micha, J.-S. and Dehm, G.
ADVANCED ENGINEERING MATERIALS. Volume: 13 (2011) - 2011 • 1021
Highly dispersed MoO 3/Al 2O 3 shell-core composites synthesized by CVD of Mo(CO) 6 under atmospheric pressure
Shi, G. and Franzke, T. and Xia, W. and Sanchez, M.D. and Muhler, M.
CHEMICAL VAPOR DEPOSITION. Volume: 17 (2011) - 2011 • 1020
A new AC-SECM mode: On the way to high-resolution local impedance measurements in SECM
Gȩbala, M. and Schuhmann, W. and La Mantia, F.
ELECTROCHEMISTRY COMMUNICATIONS. Volume: 13 (2011) - 2011 • 1019
Impact of instrumental constraints and imperfections on the dislocation structure in micron-sized Cu compression pillars
Kirchlechner, C. and Keckes, J. and Motz, C. and Grosinger, W. and Kapp, M.W. and Micha, J.S. and Ulrich, O. and Dehm, G.
ACTA MATERIALIA. Volume: 59 (2011) - 2011 • 1018
Activation of carbon dioxide on ZnO nanoparticles studied by vibrational spectroscopy
Noei, H. and Wöll, C. and Muhler, M. and Wang, Y.
JOURNAL OF PHYSICAL CHEMISTRY C. Volume: 115 (2011) - 2011 • 1017
The object-oriented DFT program library S/PHI/nX
Boeck, S. and Freysoldt, C. and Dick, A. and Ismer, L. and Neugebauer, J.
COMPUTER PHYSICS COMMUNICATIONS. Volume: 182 (2011) - 2011 • 1016
Dislocation storage in single slip-oriented Cu micro-tensile samples: New insights via X-ray microdiffraction
Kirchlechner, C. and Kiener, D. and Motz, C. and Labat, S. and Vaxelaire, N. and Perroud, O. and Micha, J.-S. and Ulrich, O. and Thomas, O. and Dehm, G. and Keckes, J.
PHILOSOPHICAL MAGAZINE. Volume: 91 (2011) - 2011 • 1015
The interaction of carbon monoxide with clean and surface-modified zinc oxide nanoparticles: A UHV-FTIRS study
Noei, H. and Wöll, C. and Muhler, M. and Wang, Y.
APPLIED CATALYSIS A: GENERAL. Volume: 391 (2011) - 2011 • 1014
Electron transfer between genetically modified Hansenula polymorpha yeast cells and electrode surfaces via os-complex modified redox polymers
Shkil, H. and Schulte, A. and Guschin, D.A. and Schuhmann, W.
CHEMPHYSCHEM. Volume: 12 (2011) - 2011 • 1013
Pilot study of modification of the bilateral sagittal split osteotomy (BSSO) in pig mandibles
Böckmann, R. and Schön, P. and Frotscher, M. and Eggeler, G. and Lethaus, B. and Wolff, K.-D.
JOURNAL OF CRANIO-MAXILLOFACIAL SURGERY. Volume: 39 (2011) - 2011 • 1012
Methanol synthesis on ZnO(000(1)over-bar). III. Free energy landscapes, reaction pathways, and mechanistic insights
Kiss, J. and Frenzel, J. and Nair, N. N. and Meyer, B. and Marx, D.
JOURNAL OF CHEMICAL PHYSICS. Volume: 134 (2011) - 2011 • 1011
Edge-induced magnetoplasmon excitation in a two-dimensional electron gas under quantum Hall conditions
Notthoff, C. and Reuter, D. and Wieck, A.D. and Lorke, A.
PHYSICAL REVIEW B - CONDENSED MATTER AND MATERIALS PHYSICS. Volume: 84 (2011) - 2011 • 1010
Anomalous property evolution during annealing in HPTed SUS 304 austenitic stainless steel
Shuro, I. and Umemoto, M. and Todaka, Y. and Kuo, H.-H. and Wang, H.
MATERIALS SCIENCE FORUM. Volume: 667-669 (2011) - 2011 • 1009
The influence of additions of Al and Si on the lattice stability of fcc and hcp Fe-Mn random alloys
Gebhardt, T. and Music, D. and Ekholm, M. and Abrikosov, I.A. and Vitos, L. and Dick, A. and Hickel, T. and Neugebauer, J. and Schneider, J.M.
JOURNAL OF PHYSICS CONDENSED MATTER. Volume: 23 (2011) - 2011 • 1008
Nanoscale photoelectron ionisation detector based on lanthanum hexaboride
Zimmer, C.M. and Schubert, J. and Hamann, S. and Kunze, U. and Doll, T.
PHYSICA STATUS SOLIDI (A) APPLICATIONS AND MATERIALS SCIENCE. Volume: 208 (2011) - 2011 • 1007
Ceria/silicon carbide core-shell materials prepared by miniemulsion technique
Borchardt, L. and Oschatz, M. and Frind, R. and Kockrick, E. and Lohe, M.R. and Hauser, C.P. and Weiss, C.K. and Landfester, K. and Büchner, B. and Kaskel, S.
BEILSTEIN JOURNAL OF NANOTECHNOLOGY. Volume: 2 (2011) - 2011 • 1006
Magnetotransport properties of Cu2MnAl, Co2MnGe, and Co2MnSi Heusler alloy thin films: From nanocrystalline disordered state to long-range-ordered crystalline state
Obaida, M. and Westerholt, K. and Zabel, H.
PHYSICAL REVIEW B - CONDENSED MATTER AND MATERIALS PHYSICS. Volume: 84 (2011) - 2011 • 1005
Synthesis and hydriding/dehydriding properties of Mg2Ni-AB (AB = TiNi or TiFe) nanocomposites
Zlatanova, Z. and Spassov, T. and Eggeler, G. and Spassova, M.
INTERNATIONAL JOURNAL OF HYDROGEN ENERGY. Volume: 36 (2011) - 2011 • 1004
Interdiffusion in Fe/Pt multilayers: In situ high temperature synchrotron radiation reflectivity study
Zotov, N. and Feydt, J. and Savan, A. and Ludwig, Al. and Von Borany, J.
ADVANCED ENGINEERING MATERIALS. Volume: 13 (2011) - 2011 • 1003
Response-adaptive dose-finding under model uncertainty
Bornkamp, B. and Bretz, F. and Dette, H. and Pinheiro, J.
ANNALS OF APPLIED STATISTICS. Volume: 5 (2011) - 2011 • 1002
Time-resolved photoluminescence and optical gain of Ga(NAsP) heterostructures pseudomorphically grown on silicon (001) substrate
Gerhardt, N.C. and Koukourakis, N. and Klimasch, M. and Funke, D.A. and Hofmann, M.R. and Kunert, B. and Liebich, S. and Trusheim, D. and Zimprich, M. and Volz, K. and Stolz, W.
INTERNATIONAL CONFERENCE ON TRANSPARENT OPTICAL NETWORKS. Volume: (2011) - 2011 • 1001
Observation of the Kondo effect in a spin-32 hole quantum dot
Klochan, O. and Micolich, A.P. and Hamilton, A.R. and Trunov, K. and Reuter, D. and Wieck, A.D.
PHYSICAL REVIEW LETTERS. Volume: 107 (2011) - 2011 • 1000
Dislocation plasticity of Al film on polyimide investigated by cross-sectional in situ transmission electron microscopy straining
Oh, S.H. and Rentenberger, C. and Im, J. and Motz, C. and Kiener, D. and Karnthaler, H.-P. and Dehm, G.
SCRIPTA MATERIALIA. Volume: 65 (2011) - 2011 • 999
Ultrafast spin-induced polarization oscillations with tunable lifetime in vertical-cavity surface-emitting lasers
Gerhardt, N.C. and Li, M.Y. and Jhme, H. and Hpfner, H. and Ackemann, T. and Hofmann, M.R.
APPLIED PHYSICS LETTERS. Volume: 99 (2011) - 2011 • 998
On the stress-induced formation of R-phase in ultra-fine-grained Ni-rich NiTi shape memory alloys
Olbricht, J. and Yawny, A. and Pelegrina, J.L. and Dlouhy, A. and Eggeler, G.
METALLURGICAL AND MATERIALS TRANSACTIONS A: PHYSICAL METALLURGY AND MATERIALS SCIENCE. Volume: 42 (2011) - 2011 • 997
Microfabrication by optical tweezers
Ghadiri, R. and Weigel, T. and Esen, C. and Ostendorf, A.
PROCEEDINGS OF SPIE - THE INTERNATIONAL SOCIETY FOR OPTICAL ENGINEERING. Volume: 7921 (2011) - 2011 • 996
Magnetic resonance from the interplay of frustration and superconductivity
Knolle, J. and Eremin, I. and Schmalian, J. and Moessner, R.
PHYSICAL REVIEW B - CONDENSED MATTER AND MATERIALS PHYSICS. Volume: 84 (2011) - 2011 • 995
The role of the synthesis method in the structure formation of cobalt aluminate
Onfroy, T. and Li, W.-C. and Schüth, F. and Knözinger, H.
TOPICS IN CATALYSIS. Volume: 54 (2011) - 2011 • 994
Designing shape-memory Heusler alloys from first-principles
Siewert, M. and Gruner, M.E. and Dannenberg, A. and Chakrabarti, A. and Herper, H.C. and Wuttig, M. and Barman, S.R. and Singh, S. and Al-Zubi, A. and Hickel, T. and Neugebauer, J. and Gillessen, M. and Dronskowski, R. and Entel, P.
APPLIED PHYSICS LETTERS. Volume: 99 (2011) - 2011 • 993
Multiorbital spin susceptibility in a magnetically ordered state: Orbital versus excitonic spin density wave scenario
Knolle, J. and Eremin, I. and Moessner, R.
PHYSICAL REVIEW B - CONDENSED MATTER AND MATERIALS PHYSICS. Volume: 83 (2011) - 2011 • 992
Oxygen chemisorption, formation, and thermal stability of Pt oxides on Pt nanoparticles supported on SiO2/Si(001): Size effects
Ono, L.K. and Croy, J.R. and Heinrich, H. and Roldan Cuenya, B.
JOURNAL OF PHYSICAL CHEMISTRY C. Volume: 115 (2011) - 2011 • 991
Hot Direct Extrusion of Abrasion Resistant Fe-Base Metal Matrix Composites-Interface Characterization and Mechanical Properties of Co-Extruded Layered Structures
Silva, P.A. and Weber, S. and Karlsohn, M. and Röttger, A. and Theisen, W. and Reimers, W. and Pyzalla, A.R.
FRICTION, WEAR AND WEAR PROTECTION: INTERNATIONAL SYMPOSIUM ON FRICTION, WEAR AND WEAR PROTECTION 2008 AACHEN, GERMANY. Volume: (2011) - 2011 • 990
Influence of alkali-silica reactions on cracking in concrete pavements
Breitenbücher, R. and Sievering, C.
T AND DI CONGRESS 2011: INTEGRATED TRANSPORTATION AND DEVELOPMENT FOR A BETTER TOMORROW - PROCEEDINGS OF THE 1ST CONGRESS OF THE TRANSPORTATION AND DEVELOPMENT INSTITUTE OF ASCE. Volume: (2011) - 2011 • 989
Interlayer expansion of the layered zeolite precursor RUB-39: A universal method to synthesize functionalized microporous silicates
Gies, H. and Müller, U. and Yilmaz, B. and Tatsumi, T. and Xie, B. and Xiao, F.-S. and Xinhe Bao and Zhang, W. and De Vos, D.
CHEMISTRY OF MATERIALS. Volume: 23 (2011) - 2011 • 988
Plasma-assisted synthesis of Ag/ZnO nanocomposites: First example of photo-induced H2 production and sensing
Simon, Q. and Barreca, D. and Bekermann, D. and Gasparotto, A. and MacCato, C. and Comini, E. and Gombac, V. and Fornasiero, P. and Lebedev, O.I. and Turner, S. and Devi, A. and Fischer, R.A. and Van Tendeloo, G.
INTERNATIONAL JOURNAL OF HYDROGEN ENERGY. Volume: 36 (2011) - 2011 • 987
The evolution of laminates in finite crystal plasticity: A variational approach
Kochmann, D.M. and Hackl, K.
CONTINUUM MECHANICS AND THERMODYNAMICS. Volume: 23 (2011) - 2011 • 986
CeO2/Pt catalyst nanoparticle containing carbide-derived carbon composites by a new in situ functionalization strategy
Kockrick, E. and Borchardt, L. and Schrage, C. and Gaudillere, C. and Ziegler, C. and Freudenberg, T. and Farrusseng, D. and Eychmüller, A. and Kaskel, S.
CHEMISTRY OF MATERIALS. Volume: 23 (2011) - 2011 • 985
Semiconductor diode lasers for terahertz technology
Brenner, C. and Friedrich, C.-S. and Hofmann, M.R.
JOURNAL OF INFRARED, MILLIMETER, AND TERAHERTZ WAVES. Volume: 32 (2011) - 2011 • 984
On the formation of vacancies by edge dislocation dipole annihilation in fatigued copper
Brinckmann, S. and Sivanesapillai, R. and Hartmaier, A.
INTERNATIONAL JOURNAL OF FATIGUE. Volume: 33 (2011) - 2011 • 983
Interdigitating biocalcite dendrites form a 3-D jigsaw structure in brachiopod shells
Goetz, A.J. and Steinmetz, D.R. and Griesshaber, E. and Zaefferer, S. and Raabe, D. and Kelm, K. and Irsen, S. and Sehrbrock, A. and Schmahl, W.W.
ACTA BIOMATERIALIA. Volume: 7 (2011) - 2011 • 982
Phase transformation, structural and functional fatigue properties of Ti-Ni-Hf shape memory thin films
König, D. and Zarnetta, R. and Savan, A. and Brunken, H. and Ludwig, Al.
ACTA MATERIALIA. Volume: 59 (2011) - 2011 • 981
On the evolution of microstructure in oxygen-free high conductivity copper during thermomechanical processing using rotary swaging
Otto, F. and Frenzel, J. and Eggeler, G.
INTERNATIONAL JOURNAL OF MATERIALS RESEARCH. Volume: 102 (2011) - 2011 • 980
Role of spin quantization in determining the thermodynamic properties of magnetic transition metals
Körmann, F. and Dick, A. and Hickel, T. and Neugebauer, J.
PHYSICAL REVIEW B - CONDENSED MATTER AND MATERIALS PHYSICS. Volume: 83 (2011) - 2011 • 979
On the influence of small quantities of Bi and Sb on the evolution of microstructure during swaging and heat treatments in copper
Otto, F. and Frenzel, J. and Eggeler, G.
JOURNAL OF ALLOYS AND COMPOUNDS. Volume: 509 (2011) - 2011 • 978
Composition and orientation effects on the final recrystallization texture of coarse-grained Nb-containing AISI 430 ferritic stainless steels
Siqueira, R.P. and Sandim, H.R.Z. and Oliveira, T.R. and Raabe, D.
MATERIALS SCIENCE AND ENGINEERING A. Volume: 528 (2011) - 2011 • 977
Atomistically informed continuum model for body centered cubic iron
Koester, A. and Ma, A. and Hartmaier, A.
MATERIALS RESEARCH SOCIETY SYMPOSIUM PROCEEDINGS. Volume: 1296 (2011) - 2011 • 976
Polarization-preserving confocal microscope for optical experiments in a dilution refrigerator with high magnetic field
Sladkov, M. and Bakker, M.P. and Chaubal, A.U. and Reuter, D. and Wieck, A.D. and Van Der Wal, C.H.
REVIEW OF SCIENTIFIC INSTRUMENTS. Volume: 82 (2011) - 2011 • 975
High-energy X-ray diffraction studies of i-Sc12Zn88
Goldman, A.I. and Kreyssig, A. and Nandi, S. and Kim, M.G. and Caudle, M.L. and Canfield, P.C.
PHILOSOPHICAL MAGAZINE. Volume: 91 (2011) - 2011 • 974
Photorefractive two-wave mixing for image amplification in digital holography
Koukourakis, N. and Abdelwahab, T. and Li, M.Y. and Höpfner, H. and Lai, Y.W. and Darakis, E. and Brenner, C. and Gerhardt, N.C. and Hofmann, M.R.
OPTICS EXPRESS. Volume: 19 (2011) - 2011 • 973
High modal gain in Ga(NAsP)/(BGa)((As)P) heterostructures grown lattice matched on (001) silicon
Koukourakis, N. and Funke, D.A. and Gerhardt, N.C. and Hofmann, M.R. and Liebich, S. and Bückers, C. and Zinnkann, S. and Zimprich, M. and Beyer, A. and Chatterjee, S. and Koch, S.W. and Kunert, B. and Volz, K. and Stolz, W.
PROCEEDINGS OF SPIE - THE INTERNATIONAL SOCIETY FOR OPTICAL ENGINEERING. Volume: 7939 (2011) - 2011 • 972
Combinatorial investigation of Fe-B thin-film nanocomposites
Brunken, H. and Grochla, D. and Savan, A. and Kieschnick, M. and Meijer, J. D. and Ludwig, Al.
SCIENCE AND TECHNOLOGY OF ADVANCED MATERIALS. Volume: 12 (2011) - 2011 • 971
Mode-filtered electron injection into a waveguide interferometer
Buchholz, S.S. and Kunze, U. and Reuter, D. and Wieck, A.D. and Fischer, S.F.
APPLIED PHYSICS LETTERS. Volume: 98 (2011) - 2011 • 970
Magnetic order in GdBiPt studied by x-ray resonant magnetic scattering
Kreyssig, A. and Kim, M.G. and Kim, J.W. and Pratt, D.K. and Sauerbrei, S.M. and March, S.D. and Tesdall, G.R. and Bud'Ko, S.L. and Canfield, P.C. and McQueeney, R.J. and Goldman, A.I.
PHYSICAL REVIEW B - CONDENSED MATTER AND MATERIALS PHYSICS. Volume: 84 (2011) - 2011 • 969
Room temperature spin relaxation length in spin light-emitting diodes
Soldat, H. and Li, M. and Gerhardt, N.C. and Hofmann, M.R. and Ludwig, Ar. and Ebbing, A. and Reuter, D. and Wieck, A.D. and Stromberg, F. and Keune, W. and Wende, H.
APPLIED PHYSICS LETTERS. Volume: 99 (2011) - 2011 • 968
Simultaneous visualization of surface topography and concentration field by means of scanning electrochemical microscopy using a single electrochemical probe and impedance spectroscopy
Pähler, M. and Schuhmann, W. and Gratzl, M.
CHEMPHYSCHEM. Volume: 12 (2011) - 2011 • 967
Temperature-driven phase transitions from first principles including all relevant excitations: The fcc-to-bcc transition in Ca
Grabowski, B. and Söderlind, P. and Hickel, T. and Neugebauer, J.
PHYSICAL REVIEW B - CONDENSED MATTER AND MATERIALS PHYSICS. Volume: 84 (2011) - 2011 • 966
Particle stress in suspensions of soft objects
Krüger, T. and Varnik, F. and Raabe, D.
PHILOSOPHICAL TRANSACTIONS OF THE ROYAL SOCIETY A: MATHEMATICAL, PHYSICAL AND ENGINEERING SCIENCES. Volume: 369 (2011) - 2011 • 965
Application of AC-SECM in corrosion science: Local visualisation of inhibitor films on active metals for corrosion protection
Pähler, M. and Santana, J.J. and Schuhmann, W. and Souto, R.M.
CHEMISTRY - A EUROPEAN JOURNAL. Volume: 17 (2011) - 2011 • 964
Hierarchical microstructure of explosive joints: Example of titanium to steel cladding
Song, J. and Kostka, A. and Veehmayer, M. and Raabe, D.
MATERIALS SCIENCE AND ENGINEERING A. Volume: 528 (2011) - 2011 • 963
Testing model assumptions in functional regression models
Bücher, A. and Dette, H. and Wieczorek, G.
JOURNAL OF MULTIVARIATE ANALYSIS. Volume: 102 (2011) - 2011 • 962
Formation Energies of Point Defects at Finite Temperatures
Grabowski, B. and Hickel, T. and Neugebauer, J.
ADVANCED CALCULATIONS FOR DEFECTS IN MATERIALS: ELECTRONIC STRUCTURE METHODS. Volume: (2011) - 2011 • 961
Efficient and accurate simulations of deformable particles immersed in a fluid using a combined immersed boundary lattice Boltzmann finite element method
Krüger, T. and Varnik, F. and Raabe, D.
COMPUTERS AND MATHEMATICS WITH APPLICATIONS. Volume: 61 (2011) - 2011 • 960
High-throughput characterization of the seebeck coefficient of a-(Cr 1 - XSix)1 - yOy thin film materials libraries as verification of the extended thermopower formula
Sonntag, J. and Ziolkowski, P. and Savan, A. and Kieschnick, M. and Ludwig, Al.
JOURNAL OF PHYSICS CONDENSED MATTER. Volume: 23 (2011) - 2011 • 959
New estimators of the pickands dependence function and a test for extreme-value dependence
Bücher, A. and Dette, H. and Volgushev, S.
ANNALS OF STATISTICS. Volume: 39 (2011) - 2011 • 958
Formation energies of point defects at finite temperatures
Grabowski, B. and Hickel, T. and Neugebauer, J.
PHYSICA STATUS SOLIDI (B) BASIC RESEARCH. Volume: 248 (2011) - 2011 • 957
First-principles approach to phase stability for a ternary σ phase: Application to Cr-Ni-Re
Palumbo, M. and Abe, T. and Fries, S.G. and Pasturel, A.
PHYSICAL REVIEW B - CONDENSED MATTER AND MATERIALS PHYSICS. Volume: 83 (2011) - 2011 • 956
The effects of grain size on the phase transformation properties of annealed (Ti/Ni/W) shape memory alloy multilayers
Buenconsejo, P.J.S. and Zarnetta, R. and Ludwig, Al.
SCRIPTA MATERIALIA. Volume: 64 (2011) - 2011 • 955
The influence of the potassium promoter on the kinetics and thermodynamics of CO adsorption on a bulk iron catalyst applied in Fischer-Tropsch synthesis: A quantitative adsorption calorimetry, temperature-programmed desorption, and surface hydrogenation study
Graf, B. and Muhler, M.
PHYSICAL CHEMISTRY CHEMICAL PHYSICS. Volume: 13 (2011) - 2011 • 954
A comparative study of reaction models applied for chemical looping combustion
Kruggel-Emden, H. and Stepanek, F. and Munjiza, A.
CHEMICAL ENGINEERING RESEARCH AND DESIGN. Volume: 89 (2011) - 2011 • 953
Anomalous elastic behavior of relaxor ferroelectric Ca 0.28Ba0.72Nb2O6 single crystals
Pandey, C.S. and Schreuer, J. and Burianek, M. and Mühlberg, M.
PHYSICAL REVIEW B - CONDENSED MATTER AND MATERIALS PHYSICS. Volume: 84 (2011) - 2011 • 952
Optical control of coherent interactions between electron spins in InGaAs quantum dots
Spatzek, S. and Greilich, A. and Economou, S.E. and Varwig, S. and Schwan, A. and Yakovlev, D.R. and Reuter, D. and Wieck, A.D. and Reinecke, T.L. and Bayer, M.
PHYSICAL REVIEW LETTERS. Volume: 107 (2011) - 2011 • 951
A new prototype two-phase (TiNi)-(β-W) SMA system with tailorable thermal hysteresis
Buenconsejo, P.J.S. and Zarnetta, R. and König, D. and Savan, A. and Thienhaus, S. and Ludwig, Al.
ADVANCED FUNCTIONAL MATERIALS. Volume: 21 (2011) - 2011 • 950
Performance of integration schemes in discrete element simulations of particle systems involving consecutive contacts
Kruggel-Emden, H. and Stepanek, F. and Munjiza, A.
COMPUTERS AND CHEMICAL ENGINEERING. Volume: 35 (2011) - 2011 • 949
Correlation between dielectric properties and chemical composition of the tourmaline single crystals
Pandey, C.S. and Jodlauk, S. and Schreuer, J.
APPLIED PHYSICS LETTERS. Volume: 99 (2011) - 2011 • 948
Generation and detection of mode-locked spin coherence in (In,Ga)As/GaAs quantum dots by laser pulses of long duration
Spatzek, S. and Varwig, S. and Glazov, M.M. and Yugova, I.A. and Schwan, A. and Yakovlev, D.R. and Reuter, D. and Wieck, A.D. and Bayer, M.
PHYSICAL REVIEW B - CONDENSED MATTER AND MATERIALS PHYSICS. Volume: 84 (2011) - 2011 • 947
Heat and Mass Flow Control in an Interconnected Multiphase CFD Model for Chemical Looping Combustion
Kruggel-Emden, H. and Scherer, V.
CHEMICAL ENGINEERING AND TECHNOLOGY. Volume: 34 (2011) - 2011 • 946
A study on the role of reaction modeling in multi-phase CFD-based simulations of chemical looping combustion
Kruggel-Emden, H. and Stepanek, F. and Munjiza, A.
OIL AND GAS SCIENCE AND TECHNOLOGY. Volume: 66 (2011) - 2011 • 945
On the formation and growth of intermetallic phases during interdiffusion between low-carbon steel and aluminum alloys
Springer, H. and Kostka, A. and Payton, E.J. and Raabe, D. and Kaysser-Pyzalla, A. and Eggeler, G.
ACTA MATERIALIA. Volume: 59 (2011) - 2011 • 944
Induced magnetic Cu moments and magnetic ordering in Cu2MnAl thin films on MgO(0 0 1) observed by XMCD
Krumme, B. and Herper, H.C. and Erb, D. and Weis, C. and Antoniak, C. and Warland, A. and Westerholt, K. and Entel, P. and Wende, H.
JOURNAL OF PHYSICS D: APPLIED PHYSICS. Volume: 44 (2011) - 2011 • 943
Evolution of the structure and chemical state of Pd nanoparticles during the in situ catalytic reduction of NO with H2
Paredis, K. and Ono, L.K. and Behafarid, F. and Zhang, Z. and Yang, J.C. and Frenkel, A.I. and Cuenya, B.R.
JOURNAL OF THE AMERICAN CHEMICAL SOCIETY. Volume: 133 (2011) - 2011 • 942
Influence of intermetallic phases and Kirkendall-porosity on the mechanical properties of joints between steel and aluminium alloys
Springer, H. and Kostka, A. and dos Santos, J.F. and Raabe, D.
MATERIALS SCIENCE AND ENGINEERING A. Volume: 528 (2011) - 2011 • 941
A novel high-throughput fatigue testing method for metallic thin films
Burger, S. and Eberl, C. and Siegel, A. and Ludwig, Al. and Kraft, O.
SCIENCE AND TECHNOLOGY OF ADVANCED MATERIALS. Volume: 12 (2011) - 2011 • 940
Structure, chemical composition, and reactivity correlations during the in situ oxidation of 2-propanol
Paredis, K. and Ono, L.K. and Mostafa, S. and Li, L. and Zhang, Z. and Yang, J.C. and Barrio, L. and Frenkel, A.I. and Cuenya, B.R.
JOURNAL OF THE AMERICAN CHEMICAL SOCIETY. Volume: 133 (2011) - 2011 • 939
Easily made and handled carbon nanocones for scanning tunneling microscopy and electroanalysis
Sripirom, J. and Noor, S. and Köhler, U. and Schulte, A.
CARBON. Volume: 49 (2011) - 2011 • 938
Fatigue testing of thin films
Burger, S. and Rupp, B. and Ludwig, Al. and Kraft, O. and Eberl, C.
KEY ENGINEERING MATERIALS. Volume: 465 (2011) - 2011 • 937
Cell type-specific responses of peripheral blood mononuclear cells to silver nanoparticles
Greulich, C. and Diendorf, J. and Geßmann, J. and Simon, T. and Habijan, T. and Eggeler, G. and Schildhauer, T.A. and Epple, M. and Köller, M.
ACTA BIOMATERIALIA. Volume: 7 (2011) - 2011 • 936
Uptake and intracellular distribution of silver nanoparticles in human mesenchymal stem cells
Greulich, C. and Diendorf, J. and Simon, T. and Eggeler, G. and Epple, M. and Köller, M.
ACTA BIOMATERIALIA. Volume: 7 (2011) - 2011 • 935
Studies on synthesis and characterization of mo based in situ composite by silicothermy co-reduction process
Paul, B. and Chakraborty, S.P. and Kishor, J. and Sharma, I.G. and Suri, A.K.
METALLURGICAL AND MATERIALS TRANSACTIONS B: PROCESS METALLURGY AND MATERIALS PROCESSING SCIENCE. Volume: 42 (2011) - 2011 • 934
Sintering kinetics study of mechanically alloyed nanocrystalline Mo-30 wt.% W
Paul, B. and Jain, D. and Chakraborty, S.P. and Sharma, I.G. and Pillai, C.G.S. and Suri, A.K.
THERMOCHIMICA ACTA. Volume: 512 (2011) - 2011 • 933
Dyakonov-Perel electron spin relaxation in a wurtzite semiconductor: From the nondegenerate to the highly degenerate regime
Buß, J.H. and Rudolph, J. and Starosielec, S. and Schaefer, A. and Semond, F. and Cordier, Y. and Wieck, A.D. and Hägele, D.
PHYSICAL REVIEW B - CONDENSED MATTER AND MATERIALS PHYSICS. Volume: 84 (2011) - 2011 • 932
Long room-temperature electron spin lifetimes in bulk cubic GaN
Buß, J.H. and Rudolph, J. and Schupp, T. and As, D.J. and Lischka, K. and Hägele, D.
PROCEEDINGS OF SPIE - THE INTERNATIONAL SOCIETY FOR OPTICAL ENGINEERING. Volume: 7937 (2011) - 2011 • 931
Modelling thermal fluctuations in non-ideal fluids with the lattice Boltzmann method
Gross, M. and Adhikari, R. and Cates, M.E. and Varnik, F.
PHILOSOPHICAL TRANSACTIONS OF THE ROYAL SOCIETY A: MATHEMATICAL, PHYSICAL AND ENGINEERING SCIENCES. Volume: 369 (2011) - 2011 • 930
Langevin theory of fluctuations in the discrete Boltzmann equation
Gross, M. and Cates, M.E. and Varnik, F. and Adhikari, R.
JOURNAL OF STATISTICAL MECHANICS: THEORY AND EXPERIMENT. Volume: 2011 (2011) - 2011 • 929
Deformation and fracture mechanisms in fine- and ultrafine-grained ferrite/martensite dual-phase steels and the effect of aging
Calcagnotto, M. and Adachi, Y. and Ponge, D. and Raabe, D.
ACTA MATERIALIA. Volume: 59 (2011) - 2011 • 928
Shear stress in nonideal fluid lattice Boltzmann simulations
Gross, M. and Moradi, N. and Zikos, G. and Varnik, F.
PHYSICAL REVIEW E - STATISTICAL, NONLINEAR, AND SOFT MATTER PHYSICS. Volume: 83 (2011) - 2011 • 927
A phase-field model for incoherent martensitic transformations including plastic accommodation processes in the austenite
Kundin, J. and Raabe, D. and Emmerich, H.
JOURNAL OF THE MECHANICS AND PHYSICS OF SOLIDS. Volume: 59 (2011) - 2011 • 926
Magnetic domain patterns in Co2MnGe Heusler nanostripes
Gross, K. and Szary, P. and Petracic, O. and Brüssing, F. and Westerholt, K. and Zabel, H.
PHYSICAL REVIEW B - CONDENSED MATTER AND MATERIALS PHYSICS. Volume: 84 (2011) - 2011 • 925
Single-shot holography with colliding pulse mode-locked lasers as light source
Grosse, D. and Koukourakis, N. and Gerhardt, N.C. and Schlauch, T. and Balzer, J.C. and Klehr, A. and Erbert, G. and Tränkle, G. and Hofmann, M.R.
2011 INT. QUANTUM ELECTRON. CONF., IQEC 2011 AND CONF. LASERS AND ELECTRO-OPTICS, CLEO PACIFIC RIM 2011 INCORPORATING THE AUSTRALASIAN CONF. OPTICS, LASERS AND SPECTROSCOPY AND THE AUSTRALIAN CONF.. Volume: (2011) - 2011 • 924
Phonon-assisted exciton spin relaxation in (In,Ga)As/GaAs quantum dots
Kurtze, H. and Yakovlev, D.R. and Reuter, D. and Wieck, A.D. and Bayer, M.
PHYSICA STATUS SOLIDI (C) CURRENT TOPICS IN SOLID STATE PHYSICS. Volume: 8 (2011) - 2011 • 923
High temperature test rig for inert atmosphere miniature specimen creep testing
Peter, D. and Otto, F. and Depka, T. and Nörtershäuser, P. and Eggeler, G.
MATERIALWISSENSCHAFT UND WERKSTOFFTECHNIK. Volume: 42 (2011) - 2011 • 922
Colliding pulse mode-locked lasers as light sources for single-shot holography
Grosse, D. and Koukourakis, N. and Gerhardt, N.C. and Schlauch, T. and Balzer, J.C. and Klehr, A. and Erbert, G. and Tränkle, G. and Hofmann, M.R.
PROCEEDINGS OF SPIE - THE INTERNATIONAL SOCIETY FOR OPTICAL ENGINEERING. Volume: 8001 (2011) - 2011 • 921
X-ray photoelectron spectroscopy on implanted argon as a tool to follow local structural changes in thin films
Lahrood, A. R. and de los Arcos, T. and Prenzel, M. and von Keudell, A. and Winter, J.
THIN SOLID FILMS. Volume: 520 (2011) - 2011 • 920
High-throughput characterization of film thickness in thin film materials libraries by digital holographic microscopy
Lai, Y.W. and Krause, M. and Savan, A. and Thienhaus, S. and Koukourakis, N. and Hofmann, M.R. and Ludwig, Al.
SCIENCE AND TECHNOLOGY OF ADVANCED MATERIALS. Volume: 12 (2011) - 2011 • 919
Phase-field modelling of microstructure evolution in solids: Perspectives and challenges
Steinbach, I. and Shchyglo, O.
CURRENT OPINION IN SOLID STATE AND MATERIALS SCIENCE. Volume: 15 (2011) - 2011 • 918
First-principles study of the influence of (110)-oriented strain on the ferroelectric properties of rutile TiO2
Grünebohm, A. and Ederer, C. and Entel, P.
PHYSICAL REVIEW B - CONDENSED MATTER AND MATERIALS PHYSICS. Volume: 84 (2011) - 2011 • 917
High-throughput characterization of stresses in thin film materials libraries using Si cantilever array wafers and digital holographic microscopy
Lai, Y.W. and Hamann, S. and Ehmann, M. and Ludwig, Al.
REVIEW OF SCIENTIFIC INSTRUMENTS. Volume: 82 (2011) - 2011 • 916
Strain-induced effects on the electronic structure and N K-edge ELNES of wurtzite A1N and AlxGa1-xN
Petrov, M. and Holec, D. and Lymperakis, L. and Neugebauer, J. and Humphreys, C.J.
JOURNAL OF PHYSICS: CONFERENCE SERIES. Volume: 326 (2011) - 2011 • 915
Surface texturing by laser cladding
Grueninger, A. and Hustedt, M. and Herzog, D. and Huse, M. and Kracht, D. and Haferkamp, H. and Ostendorf, A.
JOURNAL OF LASER APPLICATIONS. Volume: 23 (2011) - 2011 • 914
Construction and performance of fully numerical optimum atomic basis sets
Lange, B. and Freysoldt, C. and Neugebauer, J.
PHYSICAL REVIEW B - CONDENSED MATTER AND MATERIALS PHYSICS. Volume: 84 (2011) - 2011 • 913
Ab initio study of electron paramagnetic resonance hyperfine structure of the silicon dangling bond: Role of the local environment
Pfanner, G. and Freysoldt, C. and Neugebauer, J.
PHYSICAL REVIEW B - CONDENSED MATTER AND MATERIALS PHYSICS. Volume: 83 (2011) - 2011 • 912
Plasma and optical thin film technologies
Stenzel, O. and Wilbrandt, S. and Kaiser, N. and Schmitz, C. and Turowski, M. and Ristau, D. and Awakowicz, P. and Brinkmann, R.P. and Musch, T. and Rolfes, I. and Steffen, H. and Foest, R. and Ohl, A. and Köhler, T. and Dolgonos, G. and Frauenheim, T.
PROCEEDINGS OF SPIE - THE INTERNATIONAL SOCIETY FOR OPTICAL ENGINEERING. Volume: 8168 (2011) - 2011 • 911
Micro-shear deformation of pure copper
Pfetzing-Micklich, J. and Brinckmann, S. and Dey, S.R. and Otto, F. and Hartmaier, A. and Eggeler, G.
MATERIALWISSENSCHAFT UND WERKSTOFFTECHNIK. Volume: 42 (2011) - 2011 • 910
The collagen fibril architecture in the lamina cribrosa and peripapillary sclera predicted by a computational remodeling approach
Grytz, R. and Meschke, G. and Jonas, J.B.
BIOMECHANICS AND MODELING IN MECHANOBIOLOGY. Volume: 10 (2011) - 2011 • 909
The multipole resonance probe: Characterization of a prototype
Lapke, M. and Oberrath, J. and Schulz, C. and Storch, R. and Styrnoll, T. and Zietz, C. and Awakowicz, P. and Brinkmann, R.P. and Musch, T. and Mussenbrock, T. and Rolfes, I.
PLASMA SOURCES SCIENCE AND TECHNOLOGY. Volume: 20 (2011) - 2011 • 908
Scanning transmission electron microscope observations of defects in as-grown and pre-strained Mo alloy fibers
Phani, P.S. and Johanns, K.E. and Duscher, G. and Gali, A. and George, E.P. and Pharr, G.M.
ACTA MATERIALIA. Volume: 59 (2011) - 2011 • 907
Effect of fermi surface nesting on resonant spin excitations in Ba 1-xKxFe2As2
Castellan, J.-P. and Rosenkranz, S. and Goremychkin, E.A. and Chung, D.Y. and Todorov, I.S. and Kanatzidis, M.G. and Eremin, I. and Knolle, J. and Chubukov, A.V. and Maiti, S. and Norman, M.R. and Weber, F. and Claus, H. and Guidi, T. and Bewley, R.I. and Osborn, R.
PHYSICAL REVIEW LETTERS. Volume: 107 (2011) - 2011 • 906
Impedimetric detection of hairpin ribozyme activity
Piekielska, K. and Gebala, M. and Gwiazda, S. and Müller, S. and Schuhmann, W.
ELECTROANALYSIS. Volume: 23 (2011) - 2011 • 905
Towards the quantized magnetic confinement in quantum wires
Cerchez, M. and Tarasov, A. and Hugger, S. and Xu, H. and Heinzel, T. and Zozoulenko, I.V. and Gasser-Szerer, U. and Reuter, D. and Wieck, A.D.
AIP CONFERENCE PROCEEDINGS. Volume: 1399 (2011) - 2011 • 904
Solid particle erosion of thermal spray and physical vapour deposition thermal barrier coatings
Cernuschi, F. and Lorenzoni, L. and Capelli, S. and Guardamagna, C. and Karger, M. and Vaßen, R. and von Niessen, K. and Markocsan, N. and Menuey, J. and Giolli, C.
WEAR. Volume: 271 (2011) - 2011 • 903
Microstructure evolution and mechanical properties of an intermetallic Ti-43.5Al-4Nb-1Mo-0.1B alloy after ageing below the eutectoid temperature
Cha, L. and Clemens, H. and Dehm, G.
INTERNATIONAL JOURNAL OF MATERIALS RESEARCH. Volume: 102 (2011) - 2011 • 902
In-situ TEM heating study of the γ lamellae formation inside the α2 matrix of a Ti-45Al-7.5Nb alloy
Cha, L. and Clemens, H. and Dehm, G. and Zhang, Z.
ADVANCED MATERIALS RESEARCH. Volume: 146-147 (2011) - 2011 • 901
Activity improvement of gold yolk-shell catalysts for CO oxidation by doping with TiO2
Güttel, R. and Paul, M. and Schüth, F.
CATALYSIS SCIENCE AND TECHNOLOGY. Volume: 1 (2011) - 2011 • 900
Studies on the development of TZM alloy by aluminothermic coreduction process and formation of protective coating over the alloy by plasma spray technique
Chakraborty, S.P.
INTERNATIONAL JOURNAL OF REFRACTORY METALS AND HARD MATERIALS. Volume: 29 (2011) - 2011 • 899
On the effect of superimposed external stresses on the nucleation and growth of Ni 4Ti 3 particles: A parametric phase field study
Guo, W. and Steinbach, I. and Somsen, C. and Eggeler, G.
ACTA MATERIALIA. Volume: 59 (2011) - 2011 • 898
Discrete element analysis of experiments on mixing and stoking of monodisperse spheres on a grate
Sudbrock, F. and Simsek, E. and Rickelt, S. and Wirtz, S. and Scherer, V.
POWDER TECHNOLOGY. Volume: 208 (2011) - 2011 • 897
Thermodynamics and molecular dynamics investigation of a possible new critical size for surface and inner cohesive energy of Al nanoparticles
Chamaani, A. and Marzbanrad, E. and Rahimipour, M. R. and Yaghmaee, M. S. and Aghaei, A. and Kamachali, R. D. and Behnamian, Y.
JOURNAL OF NANOPARTICLE RESEARCH. Volume: 13 (2011) - 2011 • 896
An analytical study of the static state of multi-junctions in a multi-phase field model
Guo, W. and Spatschek, R. and Steinbach, I.
PHYSICA D: NONLINEAR PHENOMENA. Volume: 240 (2011) - 2011 • 895
Effect of high gradient magnetic fields on the anodic behaviour and localized corrosion of iron in sulphuric acid solutions
Sueptitz, R. and Tschulik, K. and Uhlemann, M. and Schultz, L. and Gebert, A.
CORROSION SCIENCE. Volume: 53 (2011) - 2011 • 894
Effect of magnetization state on the corrosion behaviour of NdFeB permanent magnets
Sueptitz, R. and Tschulik, K. and Uhlemann, M. and Katter, M. and Schultz, L. and Gebert, A.
CORROSION SCIENCE. Volume: 53 (2011) - 2011 • 893
Magnetic field effects on the active dissolution of iron
Sueptitz, R. and Tschulik, K. and Uhlemann, M. and Schultz, L. and Gebert, A.
ELECTROCHIMICA ACTA. Volume: 56 (2011) - 2011 • 892
Dynamic nuclear spin resonance in n-GaAs
Chen, Y.S. and Reuter, D. and Wieck, A.D. and Bacher, G.
PHYSICAL REVIEW LETTERS. Volume: 107 (2011) - 2011 • 891
Formation of silicon grass: Nanomasking by carbon clusters in cyclic deep reactive ion etching
Leopold, S. and Kremin, C. and Ulbrich, A. and Krischok, S. and Hoffmann, M.
JOURNAL OF VACUUM SCIENCE AND TECHNOLOGY B:NANOTECHNOLOGY AND MICROELECTRONICS. Volume: 29 (2011) - 2011 • 890
Optically detected nuclear magnetic resonance in n-GaAs using an on-chip microcoil
Chen, Y.S. and Huang, J. and Reuter, D. and Ludwig, Ar. and Wieck, A.D. and Bacher, G.
APPLIED PHYSICS LETTERS. Volume: 98 (2011) - 2011 • 889
3D assembly of semiconductor and metal nanocrystals: Hybrid CdTe/Au structures with controlled content
Lesnyak, V. and Wolf, A. and Dubavik, A. and Borchardt, L. and Voitekhovich, S.V. and Gaponik, N. and Kaskel, S. and Eychmüller, A.
JOURNAL OF THE AMERICAN CHEMICAL SOCIETY. Volume: 133 (2011) - 2011 • 888
Modelling of dendritic growth in ternary alloy solidification with melt convection
Sun, D.-K. and Zhu, M.-F. and Dai, T. and Cao, W.-S. and Chen, S.-L. and Raabe, D. and Hong, C.-P.
INTERNATIONAL JOURNAL OF CAST METALS RESEARCH. Volume: 24 (2011) - 2011 • 887
Manipulation of nuclear spin dynamics in n-GaAs using an on-chip microcoil
Chen, Y.S. and Huang, J. and Ludwig, Ar. and Reuter, D. and Wieck, A.D. and Bacher, G.
JOURNAL OF APPLIED PHYSICS. Volume: 109 (2011) - 2011 • 886
Amorphous carbon film deposition on the inner surface of tubes using atmospheric pressure pulsed filamentary plasma source
Pothiraja, R. and Bibinov, N. and Awakowicz, P.
JOURNAL OF PHYSICS D: APPLIED PHYSICS. Volume: 44 (2011) - 2011 • 885
Lattice Boltzmann modeling of dendritic growth in forced and natural convection
Sun, D.K. and Zhu, M.F. and Pan, S.Y. and Yang, C.R. and Raabe, D.
COMPUTERS AND MATHEMATICS WITH APPLICATIONS. Volume: 61 (2011) - 2011 • 884
Dislocation-vacancy interactions in tungsten
Chen, Z.M. and Mrovec, M. and Gumbsch, P.
MODELLING AND SIMULATION IN MATERIALS SCIENCE AND ENGINEERING. Volume: 19 (2011) - 2011 • 883
Indentation Schmid factor and orientation dependence of nanoindentation pop-in behavior of NiAl single crystals
Li, T.L. and Gao, Y.F. and Bei, H. and George, E.P.
JOURNAL OF THE MECHANICS AND PHYSICS OF SOLIDS. Volume: 59 (2011) - 2011 • 882
Pollen characterization and identification by elastically scattered light
Surbek, M. and Esen, C. and Schweiger, G. and Ostendorf, A.
JOURNAL OF BIOPHOTONICS. Volume: 4 (2011) - 2011 • 881
Dislocation and twin substructure evolution during strain hardening of an Fe-22 wt.% Mn-0.6 wt.% C TWIP steel observed by electron channeling contrast imaging
Gutierrez-Urrutia, I. and Raabe, D.
ACTA MATERIALIA. Volume: 59 (2011) - 2011 • 880
Birefringence and spin controlled ultrafast polarization oscillations in vertical-cavity surface-emitting lasers
Li, M.Y. and Jaehme, H. and Soldat, H. and Gerhardt, N.C. and Hofmann, M.R. and Ackemann, T.
2011 CONFERENCE ON LASERS AND ELECTRO-OPTICS EUROPE AND 12TH EUROPEAN QUANTUM ELECTRONICS CONFERENCE, CLEO EUROPE/EQEC 2011. Volume: (2011) - 2011 • 879
Resonant nuclear spin pumping in (In,Ga)As quantum dots
Cherbunin, R.V. and Flisinski, K. and Gerlovin, I.Y. and Ignatiev, I.V. and Kuznetsova, M.S. and Petrov, M.Y. and Yakovlev, D.R. and Reuter, D. and Wieck, A.D. and Bayer, M.
PHYSICAL REVIEW B - CONDENSED MATTER AND MATERIALS PHYSICS. Volume: 84 (2011) - 2011 • 878
Spin induced gigahertz polarization oscillations in vertical-cavity surface-emitting laser devices
Li, M.Y. and Jaehme, H. and Soldat, H. and Gerhardt, N.C. and Hofmann, M.R. and Ackemann, T.
PROCEEDINGS OF SPIE - THE INTERNATIONAL SOCIETY FOR OPTICAL ENGINEERING. Volume: 7952 (2011) - 2011 • 877
Incommensurate spin-density wave order in electron-doped BaFe 2As2 superconductors
Pratt, D.K. and Kim, M.G. and Kreyssig, A. and Lee, Y.B. and Tucker, G.S. and Thaler, A. and Tian, W. and Zarestky, J.L. and Bud'Ko, S.L. and Canfield, P.C. and Harmon, B.N. and Goldman, A.I. and McQueeney, R.J.
PHYSICAL REVIEW LETTERS. Volume: 106 (2011) - 2011 • 876
Comparative atom probe study of Cu(In,Ga)Se 2 thin-film solar cells deposited on soda-lime glass and mild steel substrates
Choi, P.-P. and Cojocaru-Mirédin, O. and Wuerz, R. and Raabe, D.
JOURNAL OF APPLIED PHYSICS. Volume: 110 (2011) - 2011 • 875
Can human mesenchymal stem cells survive on a NiTi implant material subjected to cyclic loading?
Habijan, T. and Glogowski, T. and Kühn, S. and Pohl, M. and Wittsiepe, J. and Greulich, C. and Eggeler, G. and Schildhauer, T.A. and Köller, M.
ACTA BIOMATERIALIA. Volume: 7 (2011) - 2011 • 874
Simulation of fracture in heterogeneous elastic materials with cohesive zone models
Prechtel, M. and Ronda, P.L. and Janisch, R. and Hartmaier, A. and Leugering, G. and Steinmann, P. and Stingl, M.
INTERNATIONAL JOURNAL OF FRACTURE. Volume: 168 (2011) - 2011 • 873
Characterization of oxidation and reduction of a platinum-rhodium alloy by atom-probe tomography
Li, T. and Marquis, E.A. and Bagot, P.A.J. and Tsang, S.C. and Smith, G.D.W.
CATALYSIS TODAY. Volume: 175 (2011) - 2011 • 872
Thermal stability of TiAIN/CrN multilayer coatings studied by atom probe tomography
Choi, P.-P. and Povstugar, I. and Ahn, J.-P. and Kostka, A. and Raabe, D.
ULTRAMICROSCOPY. Volume: 111 (2011) - 2011 • 871
Atomic-scale mechanisms of deformation-induced cementite decomposition in pearlite
Li, Y.J. and Choi, P. and Borchers, C. and Westerkamp, S. and Goto, S. and Raabe, D. and Kirchheim, R.
ACTA MATERIALIA. Volume: 59 (2011) - 2011 • 870
Comparative studies on thermomechanical behavior of veriflex, a shape memory polymer, for a low strain (m = 70%): Laser experiments
Chowdhury, A.M.S. and Schmidt, C. and Neuking, K. and Eggeler, G.
JOURNAL OF MACROMOLECULAR SCIENCE, PART A: PURE AND APPLIED CHEMISTRY. Volume: 48 (2011) - 2011 • 869
Variational modeling of shape memory alloys - An overview
Hackl, K. and Junker, P. and Heinen, R.
INTERNATIONAL JOURNAL OF MATERIALS RESEARCH. Volume: 102 (2011) - 2011 • 868
Atom probe tomography characterization of heavily cold drawn pearlitic steel wire
Lia, Y.J. and Choi, P. and Borchers, C. and Chen, Y.Z. and Goto, S. and Raabe, D. and Kirchheim, R.
ULTRAMICROSCOPY. Volume: 111 (2011) - 2011 • 867
Magnetization distribution in the tetragonal Ba(Fe1-xCo x)2As2, x=0.066 probed by polarized neutron diffraction
Prokeš, K. and Gukasov, A. and Argyriou, D.N. and Bud'ko, S.L. and Canfield, P.C. and Kreyssig, A. and Goldman, A.I.
EPL. Volume: 93 (2011) - 2011 • 866
A study on the principle of maximum dissipation for coupled and non-coupled non-isothermal processes in materials
Hackl, K. and Fischer, F.D. and Svoboda, J.
PROCEEDINGS OF THE ROYAL SOCIETY A: MATHEMATICAL, PHYSICAL AND ENGINEERING SCIENCES. Volume: 467 (2011) - 2011 • 865
Relation between superconductivity and tetragonal phase stabilized by uniaxial pressure in CaFe2As2
Prokeš, K. and Kreyssig, A. and Ouladdiaf, B. and Pratt, D.K. and Ni, N. and Bud'Ko, S.L. and Canfield, P.C. and McQueeney, R.J. and Goldman, A.I. and Argyriou, D.N.
JOURNAL OF PHYSICS: CONFERENCE SERIES. Volume: 273 (2011) - 2011 • 864
A study on the principle of maximum dissipation for coupled and non-coupled non-isothermal processes in materials (vol 467, pg 1186, 2010)
Hackl, K. and Fischer, F. D. and Svoboda, J.
PROCEEDINGS OF THE ROYAL SOCIETY A-MATHEMATICAL PHYSICAL AND ENGINEERING SCIENCES. Volume: 467 (2011) - 2011 • 863
Phase transformations and functional properties of NiTi alloy with ultrafine-grained structure
Prokofiev, E. and Burow, J. and Frenzel, J. and Gunderov, D. and Eggeler, G. and Valiev, R.
MATERIALS SCIENCE FORUM. Volume: 667-669 (2011) - 2011 • 862
3D3C velocity field measurements during the electrochemical copper deposition by long-range microscopic astigmatism PTV
Cierpka, C. and Tschulik, K. and Segura, R. and Uhlemann, M. and Kähler, C.J.
TECHNISCHES MESSEN. Volume: 78 (2011) - 2011 • 861
Biological effects of nitric oxide generated by an atmospheric pressure gas-plasma on human skin cells
Liebmann, J. and Scherer, J. and Bibinov, N. and Rajasekaran, P. and Kovacs, R. and Gesche, R. and Awakowicz, P. and Kolb-Bachofen, V.
NITRIC OXIDE - BIOLOGY AND CHEMISTRY. Volume: 24 (2011) - 2011 • 860
On bootstrapping L2-type statistics in inverse regression models with convolution-type operators
Proksch, K. and Bissantz, N. and Dette, H.
AIP CONFERENCE PROCEEDINGS. Volume: 1389 (2011) - 2011 • 859
The effect of temperature and strain rate on the periodic cracking of amorphous AlxOy films on Cu
Taylor, A.A. and Edlmayr, V. and Cordill, M.J. and Dehm, G.
SURFACE AND COATINGS TECHNOLOGY. Volume: 206 (2011) - 2011 • 858
Ab initio study of the modification of elastic properties of α-iron by hydrostatic strain and by hydrogen interstitials
Psiachos, D. and Hammerschmidt, T. and Drautz, R.
ACTA MATERIALIA. Volume: 59 (2011) - 2011 • 857
The effect of film thickness variations in periodic cracking: Analysis and experiments
Taylor, A.A. and Edlmayr, V. and Cordill, M.J. and Dehm, G.
SURFACE AND COATINGS TECHNOLOGY. Volume: 206 (2011) - 2011 • 856
Quantum fluids in nanoporous media-Effects of the confinement and fractal geometry
Tayurskii, D. and Lysogorskiy, Y.
CHINESE SCIENCE BULLETIN. Volume: 56 (2011) - 2011 • 855
Stress distributions in plasma-sprayed thermal barrier coatings under thermal cycling in a temperature gradient
Limarga, A.M. and Vaßen, R. and Clarke, D.R.
JOURNAL OF APPLIED MECHANICS, TRANSACTIONS ASME. Volume: 78 (2011) - 2011 • 854
Hydrogen production from formic acid decomposition at room temperature using a Ag-Pd core-shell nanocatalyst
Tedsree, K. and Li, T. and Jones, S. and Chan, C.W.A. and Yu, K.M.K. and Bagot, P.A.J. and Marquis, E.A. and Smith, G.D.W. and Tsang, S.C.E.
NATURE NANOTECHNOLOGY. Volume: 6 (2011) - 2011 • 853
From electrons to materials
Hammerschmidt, T. and Madsen, G.K.H. and Rogal, J. and Drautz, R.
PHYSICA STATUS SOLIDI (B) BASIC RESEARCH. Volume: 248 (2011) - 2011 • 852
Effect of boron on the fracture behavior and grain boundary chemistry of Ni3Fe
Liu, Y. and Liu, C.T. and Heatherly, L. and George, E.P.
SCRIPTA MATERIALIA. Volume: 64 (2011) - 2011 • 851
Physical metallurgy and properties of β-solidifying TiAl based alloys
Clemens, H. and Schmoelzer, T. and Schloffer, M. and Schwaighofer, E. and Mayer, S. and Dehm, G.
MATERIALS RESEARCH SOCIETY SYMPOSIUM PROCEEDINGS. Volume: 1295 (2011) - 2011 • 850
Many-body enhanced nonlinear conductance resonance in quantum channels
Han, J.E. and Fischer, S.F. and Buchholz, S.S. and Kunze, U. and Reuter, D. and Wieck, A.D. and Bird, J.P.
PHYSICAL REVIEW B - CONDENSED MATTER AND MATERIALS PHYSICS. Volume: 84 (2011) - 2011 • 849
High-pressure CO adsorption on Cu-based catalysts: Zn-induced formation of strongly bound CO monitored by ATR-IR spectroscopy
Liu, Z. and Rittermeier, A. and Becker, M. and Kähler, K. and Löffler, E. and Muhler, M.
LANGMUIR. Volume: 27 (2011) - 2011 • 848
Atomic-scale characterization of the CdS/CuInSe2 interface in thin-film solar cells
Cojocaru-Miŕdin, O. and Choi, P. and Wuerz, R. and Raabe, D.
APPLIED PHYSICS LETTERS. Volume: 98 (2011) - 2011 • 847
Dislocation interactions and low-angle grain boundary strengthening
Liu, B. and Raabe, D. and Eisenlohr, P. and Roters, F. and Arsenlis, A. and Hommes, G.
ACTA MATERIALIA. Volume: 59 (2011) - 2011 • 846
Electrochemical behaviour of the anticancer dacarbazine-cu2+complex and its analytical applications
Temerk, Y.M. and Kamal, M.M. and Ibrahim, M.S. and Ibrahim, H. and Schuhmann, W.
ELECTROANALYSIS. Volume: 23 (2011) - 2011 • 845
Characterization of grain boundaries in Cu(In,Ga)Se 2 films using atom-probe tomography
Cojocaru-Mirédin, O. and Choi, P.-P. and Abou-Ras, D. and Schmidt, S.S. and Caballero, R. and Raabe, D.
IEEE JOURNAL OF PHOTOVOLTAICS. Volume: 1 (2011) - 2011 • 844
Atomic-scale distribution of impurities in cuinse2-based thin-film solar cells
Cojocaru-Miredin, O. and Choi, P. and Wuerz, R. and Raabe, D.
ULTRAMICROSCOPY. Volume: 111 (2011) - 2011 • 843
Structural, optical, and magnetic properties of Ho-implanted GaN thin films
Lo, F.-Y. and Guo, J.-Y. and Ney, V. and Ney, A. and Chern, M.-Y. and Melnikov, A. and Pezzagna, S. and Reuter, D. and Wieck, A.D. and Massies, J.
JOURNAL OF PHYSICS: CONFERENCE SERIES. Volume: 266 (2011) - 2010 • 842
A voltage-tunable in-plane diode in a two-dimensional-electron system
Ganczarczyk, A. and Voßen, S. and Geller, M. and Lorke, A. and Reuter, D. and Wieck, A.D.
PHYSICA E: LOW-DIMENSIONAL SYSTEMS AND NANOSTRUCTURES. Volume: 42 (2010) - 2010 • 841
Electrocrystallisation of metallic films under the influence of an external homogeneous magnetic field - Early stages of the layer growth
Koza, J.A. and Mogi, I. and Tschulik, K. and Uhlemann, M. and Mickel, C. and Gebert, A. and Schultz, L.
ELECTROCHIMICA ACTA. Volume: 55 (2010) - 2010 • 840
Impact of magnetic field gradients on the free corrosion of iron
Sueptitz, R. and Tschulik, K. and Uhlemann, M. and Gebert, A. and Schultz, L.
ELECTROCHIMICA ACTA. Volume: 55 (2010) - 2010 • 839
Design and characterization of novel wear resistant multilayer CVD coatings with improved adhesion between Al2O3 and Ti(C,N)
Garcia, J. and Pitonak, R. and Weißenbacher, R. and Köpf, A. and Soldera, F. and Suarez, S. and Miguel, F. and Pinto, H. and Kostka, A. and Mücklich, F.
ADVANCED ENGINEERING MATERIALS. Volume: 12 (2010) - 2010 • 838
Microstructure and texture evolution in dual-phase steels: Competition between recovery, recrystallization, and phase transformation
Peranio, N. and Li, Y.J. and Roters, F. and Raabe, D.
MATERIALS SCIENCE AND ENGINEERING A. Volume: 527 (2010) - 2010 • 837
Real-space investigation of non-adiabatic CO2 synthesis
Gawronski, H. and Mehlhorn, M. and Morgenstern, K.
ANGEWANDTE CHEMIE - INTERNATIONAL EDITION. Volume: 49 (2010) - 2010 • 836
Acidity constants from DFT-based molecular dynamics simulations
Sulpizi, M. and Sprik, M.
JOURNAL OF PHYSICS CONDENSED MATTER. Volume: 22 (2010) - 2010 • 835
How dislocation substructures evolve during long-term creep of a 12% Cr tempered martensitic ferritic steel
Pešička, J. and Aghajani, A. and Somsen, C. and Hartmaier, A. and Eggeler, G.
SCRIPTA MATERIALIA. Volume: 62 (2010) - 2010 • 834
Controlled orientation of DNA in a binary SAM as a key for the successful determination of DNA hybridization by means of electrochemical impedance spectroscopy
Gebala, M. and Schuhmann, W.
CHEMPHYSCHEM. Volume: 11 (2010) - 2010 • 833
Analysis of local microstructure after shear creep deformation of a fine-grained duplex γ-TiAl alloy
Peter, D. and Viswanathan, G.B. and Dlouhy, A. and Eggeler, G.
ACTA MATERIALIA. Volume: 58 (2010) - 2010 • 832
Zero field magnetic phase transitions and anomalous low temperature upturn in resistivity of single crystalline [formula omitted]
Sung, N.H. and Kreyssig, A. and Kim, H. and Tanatar, M.A. and Rhyee, J.S. and Kang, B.Y. and Kim, M.G. and Kim, J.Y. and Canfield, P.C. and Prozorov, R. and Goldman, A.I. and Cho, B.K.
JOURNAL OF APPLIED PHYSICS. Volume: 107 (2010) - 2010 • 831
A biotinylated intercalator for selective post-labeling of double-stranded DNA as a basis for high-sensitive DNA assays
Gebala, M. and Stoica, L. and Guschin, D. and Stratmann, L. and Hartwich, G. and Schuhmann, W.
ELECTROCHEMISTRY COMMUNICATIONS. Volume: 12 (2010) - 2010 • 830
L21-ordered Fe-Al-Ti alloys
Krein, R. and Friak, M. and Neugebauer, J. and Palm, M. and Heilmaier, M.
INTERMETALLICS. Volume: 18 (2010) - 2010 • 829
Microstructural characterization of lamellar features in TiAl by FIB imaging
Peter, D. and Eggeler, G. and Wagner, M.F.-X.
ADVANCED ENGINEERING MATERIALS. Volume: 12 (2010) - 2010 • 828
A two-dimensional electron gas as a sensitive detector for time-resolved tunneling measurements on self-assembled quantum dots
Geller, M. and Marquardt, B. and Lorke, A. and Reuter, D. and Wieck, A.D.
NANOSCALE RESEARCH LETTERS. Volume: 5 (2010) - 2010 • 827
Phase shifts and phase π jumps in four-terminal waveguide aharonov-bohm interferometers
Kreisbeck, C. and Kramer, T. and Buchholz, S.S. and Fischer, S.F. and Kunze, U. and Reuter, D. and Wieck, A.D.
PHYSICAL REVIEW B - CONDENSED MATTER AND MATERIALS PHYSICS. Volume: 82 (2010) - 2010 • 826
Particle based simulations of complex systems with MP2C: Hydrodynamics and electrostatics
Sutmann, G. and Westphal, L. and Bolten, M.
AIP CONFERENCE PROCEEDINGS. Volume: 1281 (2010) - 2010 • 825
Suppression of antiferromagnetic order and orthorhombic distortion in superconducting Ba ( Fe0.961 Rh0.039 )2As 2
Kreyssig, A. and Kim, M.G. and Nandi, S. and Pratt, D.K. and Tian, W. and Zarestky, J.L. and Ni, N. and Thaler, A. and Bud'Ko, S.L. and Canfield, P.C. and McQueeney, R.J. and Goldman, A.I.
PHYSICAL REVIEW B - CONDENSED MATTER AND MATERIALS PHYSICS. Volume: 81 (2010) - 2010 • 824
Nanoscale engineering and optical addressing of single spins in diamond
Pezzagna, S. and Wildanger, D. and Mazarov, P. and Wieck, A.D. and Sarov, Y. and Rangelow, I. and Naydenov, B. and Jelezko, F. and Hell, S.W. and Meijer, J.
SMALL. Volume: 6 (2010) - 2010 • 823
Crystal orientation effects in scratch testing with a spherical Indenter
Swadener, J.G. and Bögershausen, H. and Sander, B. and Raabe, D.
JOURNAL OF MATERIALS RESEARCH. Volume: 25 (2010) - 2010 • 822
Second-order convergence of the deviatoric stress tensor in the standard Bhatnagar-Gross-Krook lattice Boltzmann method
Krüger, T. and Varnik, F. and Raabe, D.
PHYSICAL REVIEW E - STATISTICAL, NONLINEAR, AND SOFT MATTER PHYSICS. Volume: 82 (2010) - 2010 • 821
Nanoindentation of a pseudoelastic NiTiFe shape memory alloy
Pfetzing-Micklich, J. and Wagner, M.F.-X. and Zarnetta, R. and Frenzel, J. and Eggeler, G. and Markaki, A.E. and Wheeler, J. and Clyne, T.W.
ADVANCED ENGINEERING MATERIALS. Volume: 12 (2010) - 2010 • 820
Ultrafast circular polarization oscillations in spin-polarized vertical-cavity surface-emitting laser devices
Gerhardt, N. C. and Li, M. and Jaehme, H. and Soldat, H. and Hofmann, M. R. and Ackemann, T.
PHYSICS AND SIMULATION OF OPTOELECTRONIC DEVICES XVIII. Volume: 7597 (2010) - 2010 • 819
First-principles-derived force field for copper paddle-wheel-based metal-organic frameworks
Tafipolsky, M. and Amirjalayer, S. and Schmid, R.
JOURNAL OF PHYSICAL CHEMISTRY C. Volume: 114 (2010) - 2010 • 818
Self-assembled quantum dots in a liquid-crystal-tunable microdisk resonator
Piegdon, K.A. and Offer, M. and Lorke, A. and Urbanski, M. and Hoischen, A. and Kitzerow, H.-S. and Declair, S. and Frstner, J. and Meier, T. and Reuter, D. and Wieck, A.D. and Meier, C.
PHYSICA E: LOW-DIMENSIONAL SYSTEMS AND NANOSTRUCTURES. Volume: 42 (2010) - 2010 • 817
Atomistic theoretical models for nanoporous hybrid materials
Tafipolsky, M. and Amirjalayer, S. and Schmid, R.
MICROPOROUS AND MESOPOROUS MATERIALS. Volume: 129 (2010) - 2010 • 816
Development and testing of an interconnected multiphase CFD-model for chemical looping combustion
Kruggel-Emden, H. and Rickelt, S. and Stepanek, F. and Munjiza, A.
CHEMICAL ENGINEERING SCIENCE. Volume: 65 (2010) - 2010 • 815
Tuning quantum-dot based photonic devices with liquid crystals
Piegdon, K. A. and Declair, S. and Forstner, J. and Meier, T. and Matthias, H. and Urbanski, M. and Kitzerow, H. S. and Reuter, D. and Wieck, A. D. and Lorke, A. and Meier, C.
OPTICS EXPRESS. Volume: 18 (2010) - 2010 • 814
Uniaxial-strain mechanical detwinning of CaFe2As2 and BaFe2As2 crystals: Optical and transport study
Tanatar, M.A. and Blomberg, E.C. and Kreyssig, A. and Kim, M.G. and Ni, N. and Thaler, A. and Bud'Ko, S.L. and Canfield, P.C. and Goldman, A.I. and Mazin, I.I. and Prozorov, R.
PHYSICAL REVIEW B - CONDENSED MATTER AND MATERIALS PHYSICS. Volume: 81 (2010) - 2010 • 813
Discrete element methods for large scale particle/fluid simulations
Kruggel-Emden, H. and Stepanek, F. and Munjiza, A.
AMERICAN SOCIETY OF MECHANICAL ENGINEERS, PRESSURE VESSELS AND PIPING DIVISION (PUBLICATION) PVP. Volume: 4 (2010) - 2010 • 812
A study on adjusted contact force laws for accelerated large scale discrete element simulations
Kruggel-Emden, H. and Stepanek, F. and Munjiza, A.
PARTICUOLOGY. Volume: 8 (2010) - 2010 • 811
Quantized magnetic confinement in quantum wires
Tarasov, A. and Hugger, S. and Xu, H. and Cerchez, M. and Heinzel, T. and Zozoulenko, I.V. and Gasser-Szerer, U. and Reuter, D. and Wieck, A.D.
PHYSICAL REVIEW LETTERS. Volume: 104 (2010) - 2010 • 810
Comparison of bismuth emitting liquid metal ion sources
Bischoff, L. and Pilz, W. and Mazarov, P. and Wieck, A.D.
APPLIED PHYSICS A: MATERIALS SCIENCE AND PROCESSING. Volume: 99 (2010) - 2010 • 809
Optically based manufacturing with polymer particles
Ghadiri, R. and Surbek, M. and Esen, C. and Ostendorf, A.
PHYSICS PROCEDIA. Volume: 5 (2010) - 2010 • 808
Microplasticity phenomena in thermomechanically strained nickel thin films
Taylor, A.A. and Oh, S.H. and Dehm, G.
JOURNAL OF MATERIALS SCIENCE. Volume: 45 (2010) - 2010 • 807
Magnetoelastic and structural properties of azurite Cu3(CO 3)2(OH)2 from neutron scattering and muon spin rotation
Gibson, M.C.R. and Rule, K.C. and Wolter, A.U.B. and Hoffmann, J.-U. and Prokhnenko, O. and Tennant, D.A. and Gerischer, S. and Kraken, M. and Litterst, F.J. and Süllow, S. and Schreuer, J. and Luetkens, H. and Brühl, A. and Wolf, B. and Lang, M.
PHYSICAL REVIEW B - CONDENSED MATTER AND MATERIALS PHYSICS. Volume: 81 (2010) - 2010 • 806
Nonextensive entropy of quantum liquid in fractal dimension space
Tayurskii, D.A. and Lysogorskii, Y.V.
JOURNAL OF LOW TEMPERATURE PHYSICS. Volume: 158 (2010) - 2010 • 805
Correlation-induced single-flux-quantum penetration in quantum rings
Giesbers, A.J.M. and Zeitler, U. and Katsnelson, M.I. and Reuter, D. and Wieck, A.D. and Biasiol, G. and Sorba, L. and Maan, J.C.
NATURE PHYSICS. Volume: 6 (2010) - 2010 • 804
Effect of pump-probe detuning on the Faraday rotation and ellipticity signals of mode-locked spins in (In,Ga)As/GaAs quantum dots
Glazov, M.M. and Yugova, I.A. and Spatzek, S. and Schwan, A. and Varwig, S. and Yakovlev, D.R. and Reuter, D. and Wieck, A.D. and Bayer, M.
PHYSICAL REVIEW B - CONDENSED MATTER AND MATERIALS PHYSICS. Volume: 82 (2010) - 2010 • 803
Equivalent circuit of silicon diodes subjected to high-fluence electron irradiation
Poklonski, N.A. and Gorbachuk, N.I. and Shpakovski, S.V. and Wieck, A.
TECHNICAL PHYSICS. Volume: 55 (2010) - 2010 • 802
Spinel-Type Cobalt-Manganese-Based Mixed Oxide as Sacrificial Catalyst for the High-Yield Production of Homogeneous Carbon Nanotubes
Tessonnier, J.-P. and Becker, M. and Xia, W. and Girgsdies, F. and Blume, R. and Yao, L. and Su, D.S. and Muhler, M. and Schlögl, R.
CHEMCATCHEM. Volume: 2 (2010) - 2010 • 801
A coupled fluid dynamic-discrete element simulation of heat and mass transfer in a lime shaft kiln
Bluhm-Drenhaus, T. and Simsek, E. and Wirtz, S. and Scherer, V.
CHEMICAL ENGINEERING SCIENCE. Volume: 65 (2010) - 2010 • 800
Impedance and barrier capacitance of silicon diodes implanted with high-energy Xe ions
Poklonski, N.A. and Gorbachuk, N.I. and Shpakovski, S.V. and Filipenia, V.A. and Lastovskii, S.B. and Skuratov, V.A. and Wieck, A. and Markevich, V.P.
MICROELECTRONICS RELIABILITY. Volume: 50 (2010) - 2010 • 799
Influence of radiation defects on electrical losses in silicon diodes irradiated with electrons
Poklonski, N.A. and Gorbachuk, N.I. and Shpakovski, S.V. and Lastovskii, S.B. and Wieck, A.
SEMICONDUCTORS. Volume: 44 (2010) - 2010 • 798
Non-thermal atmospheric pressure HF plasma source: Generation of nitric oxide and ozone for bio-medical applications
Kühn, S. and Bibinov, N. and Gesche, R. and Awakowicz, P.
PLASMA SOURCES SCIENCE AND TECHNOLOGY. Volume: 19 (2010) - 2010 • 797
Carbon-stabilized mesoporous MoS2 - Structural and surface characterization with spectroscopic and catalytic tools
Polyakov, M. and Poisot, M. and Van Den Berg, M.W.E. and Drescher, T. and Lotnyk, A. and Kienle, L. and Bensch, W. and Muhler, M. and Grünert, W.
CATALYSIS COMMUNICATIONS. Volume: 12 (2010) - 2010 • 796
Modelling of hot ductility during solidification of steel grades in continuous casting - Part II
Böttger, B. and Stratemeier, S. and Subasic, E. and Göhler, K. and Steinbach, I. and Senk, D.
ADVANCED ENGINEERING MATERIALS. Volume: 12 (2010) - 2010 • 795
Influence of the ball milling conditions on the preparation of rare earth aluminum hydrides
Pommerin, A. and Felderhoff, M. and Schüth, F. and Weidenthaler, C.
SCRIPTA MATERIALIA. Volume: 63 (2010) - 2010 • 794
Al-matrix composite materials reinforced by Al-Cu-Fe particles
Bonneville, J. and Laplanche, G. and Joulain, A. and Gauthier-Brunet, V. and Dubois, S.
JOURNAL OF PHYSICS: CONFERENCE SERIES. Volume: 240 (2010) - 2010 • 793
Direct synthesis of pure complex aluminium hydrides by cryomilling
Pommerin, A. and Weidenthaler, C. and Schüth, F. and Felderhoff, M.
SCRIPTA MATERIALIA. Volume: 62 (2010) - 2010 • 792
Feedback effect on spin excitations in Ce-based unconventional superconductors
Thalmeier, P. and Akbari, A. and Eremin, I.
PHYSICA C: SUPERCONDUCTIVITY AND ITS APPLICATIONS. Volume: 470 (2010) - 2010 • 791
Ordered mesoporous boron carbide based materials via precursor nanocasting
Borchardt, L. and Kockrick, E. and Wollmann, P. and Kaskel, S. and Guron, M.M. and Sneddon, L.G. and Geiger, D.
CHEMISTRY OF MATERIALS. Volume: 22 (2010) - 2010 • 790
Pulsed corona plasma source characterization for film deposition on the inner surface of tubes
Pothiraja, R. and Bibinov, N. and Awakowicz, P.
JOURNAL OF PHYSICS D: APPLIED PHYSICS. Volume: 43 (2010) - 2010 • 789
Feedback spin exciton formation in unconventional superconductors
Thalmeier, P. and Eremin, I. and Akbari, A. and Fulde, P.
JOURNAL OF SUPERCONDUCTIVITY AND NOVEL MAGNETISM. Volume: 23 (2010) - 2010 • 788
Electrochemical synthesis of core-shell catalysts for electrocatalytic applications
Kulp, C. and Chen, X. and Puschhof, A. and Schwamborn, S. and Somsen, C. and Schuhmann, W. and Bron, M.
CHEMPHYSCHEM. Volume: 11 (2010) - 2010 • 787
Electroluminescence from silicon nanoparticles fabricated from the gas phase
Theis, J. and Geller, M. and Lorke, A. and Wiggers, H. and Wieck, A. and Meier, C.
NANOTECHNOLOGY. Volume: 21 (2010) - 2010 • 786
The formation of methane over iron catalysts applied in Fischer-Tropsch synthesis: A transient and steady state kinetic study
Graf, B. and Schulte, H. and Muhler, M.
JOURNAL OF CATALYSIS. Volume: 276 (2010) - 2010 • 785
The formation of nitrogen-containing functional groups on carbon nanotube surfaces: A quantitative XPS and TPD study
Kundu, S. and Xia, W. and Busser, W. and Becker, M. and Schmidt, D.A. and Havenith, M. and Muhler, M.
PHYSICAL CHEMISTRY CHEMICAL PHYSICS. Volume: 12 (2010) - 2010 • 784
Dispersion of the superconducting spin resonance in underdoped and antiferromagnetic BaFe2 As2
Pratt, D.K. and Kreyssig, A. and Nandi, S. and Ni, N. and Thaler, A. and Lumsden, M.D. and Tian, W. and Zarestky, J.L. and Bud'Ko, S.L. and Canfield, P.C. and Goldman, A.I. and McQueeney, R.J.
PHYSICAL REVIEW B - CONDENSED MATTER AND MATERIALS PHYSICS. Volume: 81 (2010) - 2010 • 783
Time resolved optical emission spectroscopy of an HPPMS coating process
Theiss, S. and Bibinov, N. and Bagcivan, N. and Ewering, M. and Awakowicz, P. and Bobzin, K.
JOURNAL OF PHYSICS D-APPLIED PHYSICS. Volume: 43 (2010) - 2010 • 782
Ion-enhanced oxidation of aluminum as a fundamental surface process during target poisoning in reactive magnetron sputtering
Kuschel, T. and von Keudell, A.
JOURNAL OF APPLIED PHYSICS. Volume: 107 (2010) - 2010 • 781
Evidence from neutron diffraction for superconductivity in the stabilized tetragonal phase of CaFe2 As2 under uniaxial pressure
Prokeš, K. and Kreyssig, A. and Ouladdiaf, B. and Pratt, D.K. and Ni, N. and Bud'Ko, S.L. and Canfield, P.C. and McQueeney, R.J. and Argyriou, D.N. and Goldman, A.I.
PHYSICAL REVIEW B - CONDENSED MATTER AND MATERIALS PHYSICS. Volume: 81 (2010) - 2010 • 780
Suppression of Ni4Ti3 precipitation by grain size refinement in Ni-rich NiTi shape memory alloys
Prokofiev, E.A. and Burow, J.A. and Payton, E.J. and Zarnetta, R. and Frenzel, J. and Gunderov, D.V. and Valiev, R.Z. and Eggeler, G.
ADVANCED ENGINEERING MATERIALS. Volume: 12 (2010) - 2010 • 779
Liquid injection MOCVD grown binary oxides and ternary rare-earth oxide as alternate gate-oxides for logic devices
Thomas, R. and Ehrhart, P. and Waser, R. and Schubert, J. and Devi, A. and Katiyar, R.S.
ECS TRANSACTIONS. Volume: 33 (2010) - 2010 • 778
Interplay of Fe and Nd magnetism in NdFeAsO single crystals
Tian, W. and Ratcliff, W. and Kim, M.G. and Yan, J.-Q. and Kienzle, P.A. and Huang, Q. and Jensen, B. and Dennis, K.W. and McCallum, R.W. and Lograsso, T.A. and McQueeney, R.J. and Goldman, A.I. and Lynn, J.W. and Kreyssig, A.
PHYSICAL REVIEW B - CONDENSED MATTER AND MATERIALS PHYSICS. Volume: 82 (2010) - 2010 • 777
Small droplets on superhydrophobic substrates
Gross, M. and Varnik, F. and Raabe, D. and Steinbach, I.
PHYSICAL REVIEW E - STATISTICAL, NONLINEAR, AND SOFT MATTER PHYSICS. Volume: 81 (2010) - 2010 • 776
Thermal fluctuations in the lattice Boltzmann method for nonideal fluids
Gross, M. and Adhikari, R. and Cates, M.E. and Varnik, F.
PHYSICAL REVIEW E - STATISTICAL, NONLINEAR, AND SOFT MATTER PHYSICS. Volume: 82 (2010) - 2010 • 775
Metallic composites processed via extreme deformation: Toward the limits of strength in bulk materials
Raabe, D. and Choi, P. P. and Li, Y. J. and Kostka, A. and Sauvage, X. and Lecouturier, F. and Hono, K. and Kirchheim, R. and Pippan, R. and Embury, D.
MRS BULLETIN. Volume: 35 (2010) - 2010 • 774
A comparative study of (Fe, Fe3Si)/GaAs and Heusler/MgO for spintronics applications
Grünebohm, A. and Siewert, M. and Herper, H.C. and Gruner, M.E. and Entel, P.
JOURNAL OF PHYSICS: CONFERENCE SERIES. Volume: 200 (2010) - 2010 • 773
Integrity of micro-hotplates during high-temperature operation monitored by digital holographic microscopy
Lai, Y.W. and Koukourakis, N. and Gerhardt, N.C. and Hofmann, M.R. and Meyer, R. and Hamann, S. and Ehmann, M. and Hackl, K. and Darakis, E. and Ludwig, Al.
JOURNAL OF MICROELECTROMECHANICAL SYSTEMS. Volume: 19 (2010) - 2010 • 772
Synthesis of hollow metallic particles via ultrasonic treatment of a metal emulsion
Raabe, D. and Hessling, D.
SCRIPTA MATERIALIA. Volume: 62 (2010) - 2010 • 771
Compact diode-laser-based system for continuous-wave and quasi-time-domain terahertz spectroscopy
Brenner, C. and Hofmann, M. and Scheller, M. and Shakfa, M.K. and Koch, M. and Mayorga, I.C. and Klehr, A. and Erbert, G. and Tränkle, G.
OPTICS LETTERS. Volume: 35 (2010) - 2010 • 770
Practical considerations for optimal designs in clinical dose finding studies
Bretz, F. and Dette, H. and Pinheiro, J.C.
STATISTICS IN MEDICINE. Volume: 29 (2010) - 2010 • 769
Native and hydrogen-containing point defects in Mg3 N 2: A density functional theory study
Lange, B. and Freysoldt, C. and Neugebauer, J.
PHYSICAL REVIEW B - CONDENSED MATTER AND MATERIALS PHYSICS. Volume: 81 (2010) - 2010 • 768
Axial-torsional thermomechanical fatigue of a near-γ TiAl-alloy
Brookes, S.P. and Kühn, H.-J. and Skrotzki, B. and Klingelhöffer, H. and Sievert, R. and Pfetzing, J. and Peter, D. and Eggeler, G.
MATERIALS SCIENCE AND ENGINEERING A. Volume: 527 (2010) - 2010 • 767
In situ TEM study of microplasticity and Bauschinger effect in nanocrystalline metals
Rajagopalan, J. and Rentenberger, C. and Peter Karnthaler, H. and Dehm, G. and Saif, M.T.A.
ACTA MATERIALIA. Volume: 58 (2010) - 2010 • 766
Filamentary and homogeneous modes of dielectric barrier discharge (DBD) in air: Investigation through plasma characterization and simulation of surface irradiation
Rajasekaran, P. and Mertmann, P. and Bibinov, N. and Wandke, D. and Viöl, W. and Awakowicz, P.
PLASMA PROCESSES AND POLYMERS. Volume: 7 (2010) - 2010 • 765
A computational remodeling approach to predict the physiological architecture of the collagen fibril network in corneo-scleral shells
Grytz, R. and Meschke, G.
BIOMECHANICS AND MODELING IN MECHANOBIOLOGY. Volume: 9 (2010) - 2010 • 764
Synthesis and brittle-to-ductile transition of the ω-Al0.7Cu0.2Fe0.1 tetragonal phase
Laplanche, G. and Joulain, A. and Bonneville, J. and Gauthier-Brunet, V. and Dubois, S.
MATERIALS SCIENCE AND ENGINEERING A. Volume: 527 (2010) - 2010 • 763
Thick tool steel coatings using HVOF spraying for wear resistance applications
Rajasekaran, B. and Mauer, G. and Vaßen, R. and Röttger, A. and Weber, S. and Theisen, W.
SURFACE AND COATINGS TECHNOLOGY. Volume: 205 (2010) - 2010 • 762
Growth of single-crystal mesoporous carbons with im 3̄ m symmetry
Gu, D. and Bongard, H. and Meng, Y. and Miyasaka, K. and Terasaki, O. and Zhang, F. and Deng, Y. and Wu, Z. and Feng, D. and Fang, Y. and Tu, B. and Schüth, F. and Zhao, D.
CHEMISTRY OF MATERIALS. Volume: 22 (2010) - 2010 • 761
Microstructural and mechanical study of an Al matrix composite reinforced by Al-Cu-Fe Icosahedral particles
Laplanche, G. and Joulain, A. and Bonneville, J. and Gauthier-Brunet, V. and Dubois, S. and El Kabir, T.
JOURNAL OF MATERIALS RESEARCH. Volume: 25 (2010) - 2010 • 760
Development of cold work tool steel based-MMC coating using HVOF spraying and its HIP densification behaviour
Rajasekaran, B. and Mauer, G. and Vaßen, R. and Röttger, A. and Weber, S. and Theisen, W.
SURFACE AND COATINGS TECHNOLOGY. Volume: 204 (2010) - 2010 • 759
An aqueous emulsion route to synthesize mesoporous carbon vesicles and their nanocomposites
Gu, D. and Bongard, H. and Deng, Y. and Feng, D. and Wu, Z. and Fang, Y. and Mao, J. and Tu, B. and Schüth, F. and Zhao, D.
ADVANCED MATERIALS. Volume: 22 (2010) - 2010 • 758
Microstructures and mechanical properties of Al-base composite materials reinforced by Al-Cu-Fe particles
Laplanche, G. and Joulain, A. and Bonneville, J. and Schaller, R. and El Kabir, T.
JOURNAL OF ALLOYS AND COMPOUNDS. Volume: 493 (2010) - 2010 • 757
Coating of high-alloyed, ledeburitic cold work tool steel applied by HVOF spraying
Rajasekaran, B. and Mauer, G. and Vassen, R. and Röttger, A. and Weber, S. and Theisen, W.
JOURNAL OF THERMAL SPRAY TECHNOLOGY. Volume: 19 (2010) - 2010 • 756
Substrate size-selective catalysis with zeolite-encapsulated gold nanoparticles
Laursen, A.B. and Højholt, K.T. and Lundegaard, L.F. and Simonsen, S.B. and Helveg, S. and Schüth, F. and Paul, M. and Grunwaldt, J.-D. and Kegnœs, S. and Christensen, C.H. and Egeblad, K.
ANGEWANDTE CHEMIE - INTERNATIONAL EDITION. Volume: 49 (2010) - 2010 • 755
Downscaling of defect-passivated Gd2O3 thin films on p-Si(0 0 1) wafers grown by H2O-assisted atomic layer deposition
Ranjith, R. and Laha, A. and Bugiel, E. and Osten, H.J. and Xu, K. and Milanov, A.P. and Devi, A.
SEMICONDUCTOR SCIENCE AND TECHNOLOGY. Volume: 25 (2010) - 2010 • 754
Microstructure and magnetic properties of FeCo/Ti thin film multilayers annealed in nitrogen
Brunken, H. and Somsen, C. and Savan, A. and Ludwig, Al.
THIN SOLID FILMS. Volume: 519 (2010) - 2010 • 753
Ex-post size control of high-temperature-stable yolk-shell Au,@ZrO 2 catalysts
Güttel, R. and Paul, M. and Schüth, F.
CHEMICAL COMMUNICATIONS. Volume: 46 (2010) - 2010 • 752
Where Does the Lithium Go? - A Study of the Precipitates in the Stir Zone of a Friction Stir Weld in a Li-containing 2xxx Series Al Alloy
Rao, J.C. and Payton, E.J. and Somsen, C. and Neuking, K. and Eggeler, G. and Kostka, A. and Dos Santos, J.F.
ADVANCED ENGINEERING MATERIALS. Volume: 12 (2010) - 2010 • 751
Creep properties beyond 1100°C and microstructure of Co-Re-Cr alloys
Brunner, M. and Hüttner, R. and Bölitz, M.-C. and Völkl, R. and Mukherji, D. and Rösler, J. and Depka, T. and Somsen, C. and Eggeler, G. and Glatzel, U.
MATERIALS SCIENCE AND ENGINEERING A. Volume: 528 (2010) - 2010 • 750
Discharge cavitation during microwave electrochemistry at micrometre-sized electrodes
Rassaei, L. and Nebel, M. and Rees, N.V. and Compton, R.G. and Schuhmann, W. and Marken, F.
CHEMICAL COMMUNICATIONS. Volume: 46 (2010) - 2010 • 749
Random block matrices generalizing the classical Jacobi and Laguerre ensembles
Guhlich, M. and Nagel, J. and Dette, H.
JOURNAL OF MULTIVARIATE ANALYSIS. Volume: 101 (2010) - 2010 • 748
Nonlinear reaction coordinate analysis in the reweighted path ensemble
Lechner, W. and Rogal, J. and Juraszek, J. and Ensing, B. and Bolhuis, P.G.
JOURNAL OF CHEMICAL PHYSICS. Volume: 133 (2010) - 2010 • 747
Multi-phase field study of the equilibrium state of multi-junctions
Guo, W. and Steinbach, I.
INTERNATIONAL JOURNAL OF MATERIALS RESEARCH. Volume: 101 (2010) - 2010 • 746
The exoskeleton of the American lobster- From texture to anisotropic properties
Raue, L. and Klein, H. and Raabe, D.
SOLID STATE PHENOMENA. Volume: 160 (2010) - 2010 • 745
Control of the transmission phase in an asymmetric four-terminal Aharonov-Bohm interferometer
Buchholz, S.S. and Fischer, S.F. and Kunze, U. and Bell, M. and Reuter, D. and Wieck, A.D.
PHYSICAL REVIEW B - CONDENSED MATTER AND MATERIALS PHYSICS. Volume: 82 (2010) - 2010 • 744
Aharonov-Bohm oscillation phase shift in a multi-terminal asymmetric quantum ring
Buchholz, S.S. and Fischer, S.F. and Kunze, U. and Reuter, D. and Wieck, A.D.
PHYSICA E: LOW-DIMENSIONAL SYSTEMS AND NANOSTRUCTURES. Volume: 42 (2010) - 2010 • 743
A note on bootstrap approximations for the empirical copula process
Bücher, A. and Dette, H.
STATISTICS AND PROBABILITY LETTERS. Volume: 80 (2010) - 2010 • 742
"Artificial atoms" in magnetic fields: Wave-function shaping and phase-sensitive tunneling
Lei, W. and Notthoff, C. and Peng, J. and Reuter, D. and Wieck, A. and Bester, G. and Lorke, A.
PHYSICAL REVIEW LETTERS. Volume: 105 (2010) - 2010 • 741
Elimination of Biological Contaminations from Surfaces by Plasma Discharges: Chemical Sputtering
Rauscher, H. and Kylian, O. and Benedikt, J. and von Keudell, A. and Rossi, F.
CHEMPHYSCHEM. Volume: 11 (2010) - 2010 • 740
Some comments on goodness-of-fit tests for the parametric form of the copula based on L2-distances
Bücher, A. and Dette, H.
JOURNAL OF MULTIVARIATE ANALYSIS. Volume: 101 (2010) - 2010 • 739
Electronic structure of self-assembled InGaAs/GaAs quantum rings studied by capacitance-voltage spectroscopy
Lei, W. and Notthoff, C. and Lorke, A. and Reuter, D. and Wieck, A.D.
APPLIED PHYSICS LETTERS. Volume: 96 (2010) - 2010 • 738
Studies regarding the homogeneity range of the zirconium phosphide telluride Zr2+δPTe2
Tschulik, K. and Hoffmann, S. and Fokwa, B.P.T. and Gilleßen, M. and Schmidt, P.
SOLID STATE SCIENCES. Volume: 12 (2010) - 2010 • 737
Electrochemically induced formation of surface-attached temperature-responsive hydrogels. amperometric glucose sensors with tunable sensor characteristics
Bünsow, J. and Enzenberg, A. and Pohl, K. and Schuhmann, W. and Johannsmann, D.
ELECTROANALYSIS. Volume: 22 (2010) - 2010 • 736
Alkene epoxidation with mesoporous materials assembled from TS-1 seeds - Is there a hierarchical pore system?
Reichinger, M. and Schmidt, W. and Berg, M.W.E.v.d. and Aerts, A. and Martens, J.A. and Kirschhock, C.E.A. and Gies, H. and Grünert, W.
JOURNAL OF CATALYSIS. Volume: 269 (2010) - 2010 • 735
Magnetoelectrochemical surface structuring electrodeposition of structured metallic layers in magnetic gradient fields
Tschulik, K. and Koza, J.A. and Uhlemann, M. and Gebert, A. and Schultz, L.
ECS TRANSACTIONS. Volume: 25 (2010) - 2010 • 734
Influence of heat treatment and microstructure on the tensile pseudoelastic response of an Ni-rich NiTi shape memory alloy
Bujoreanu, L.-G. and Young, M.L. and Gollerthan, S. and Somsen, C. and Eggeler, G.
INTERNATIONAL JOURNAL OF MATERIALS RESEARCH. Volume: 101 (2010) - 2010 • 733
Studies on the patterning effect of copper deposits in magnetic gradient fields
Tschulik, K. and Sueptitz, R. and Koza, J. and Uhlemann, M. and Mutschke, G. and Weier, T. and Gebert, A. and Schultz, L.
ELECTROCHIMICA ACTA. Volume: 56 (2010) - 2010 • 732
Nonlinear behaviour of the resonance fluorescence from excitons in quantum wells
Burau, G.K.G. and Manzke, G. and Kieseling, F. and Stolz, H. and Reuter, D. and Wieck, A.
JOURNAL OF PHYSICS: CONFERENCE SERIES. Volume: 210 (2010) - 2010 • 731
EBSD characterization of pure iron deformed by ECAE
Renzetti, R.A. and Sandim, M.J.R. and Sandim, H.R.Z. and Hartwig, K.T. and Bernardi, H.H. and Raabe, D.
MATERIALS SCIENCE FORUM. Volume: 638-642 (2010) - 2010 • 730
Correlation of phase transformations and magnetic properties in annealed epitaxial Fe-Pd magnetic shape memory alloy films
Buschbeck, J. and Hamann, S. and Ludwig, Al. and Holzapfel, B. and Schultz, L. and Fähler, S.
JOURNAL OF APPLIED PHYSICS. Volume: 107 (2010) - 2010 • 729
Long room-temperature electron spin lifetimes in highly doped cubic GaN
Buß, J.H. and Rudolph, J. and Schupp, T. and As, D.J. and Lischka, K. and Hägele, D.
APPLIED PHYSICS LETTERS. Volume: 97 (2010) - 2010 • 728
Redox electrodeposition polymers: Adaptation of the redox potential of polymer-bound Os complexes for bioanalytical applications
Guschin, D.A. and Castillo, J. and Dimcheva, N. and Schuhmann, W.
ANALYTICAL AND BIOANALYTICAL CHEMISTRY. Volume: 398 (2010) - 2010 • 727
Birefringence controlled room-temperature picosecond spin dynamics close to the threshold of vertical-cavity surface-emitting laser devices
Li, M.Y. and Jähme, H. and Soldat, H. and Gerhardt, N.C. and Hofmann, M.R. and Ackemann, T.
APPLIED PHYSICS LETTERS. Volume: 97 (2010) - 2010 • 726
Temperature dependence of electron spin relaxation in bulk GaN
Buß, J.H. and Rudolph, J. and Natali, F. and Semond, F. and Hägele, D.
PHYSICAL REVIEW B - CONDENSED MATTER AND MATERIALS PHYSICS. Volume: 81 (2010) - 2010 • 725
Study of internal stresses in a TWIP steel analyzing transient and permanent softening during reverse shear tests
Gutierrez-Urrutia, I. and Del Valle, J.A. and Zaefferer, S. and Raabe, D.
JOURNAL OF MATERIALS SCIENCE. Volume: 45 (2010) - 2010 • 724
The effect of grain size and grain orientation on deformation twinning in a Fe-22wt.% Mn-0.6wt.% C TWIP steel
Gutierrez-Urrutia, I. and Zaefferer, S. and Raabe, D.
MATERIALS SCIENCE AND ENGINEERING A. Volume: 527 (2010) - 2010 • 723
Ethylenediamine-anchored gold nanoparticles on multi-walled carbon nanotubes: Synthesis and characterization
Li, N. and Xu, Q. and Zhou, M. and Xia, W. and Chen, X. and Bron, M. and Schuhmann, W. and Muhler, M.
ELECTROCHEMISTRY COMMUNICATIONS. Volume: 12 (2010) - 2010 • 722
Capacitance-voltage spectroscopy on InAs quantum dot valence band states in tilted magnetic fields
Reuter, D. and Roescu, R. and Zeitler, U. and Maan, J.C. and Wieck, A.D.
JOURNAL OF PHYSICS: CONFERENCE SERIES. Volume: 244 (2010) - 2010 • 721
Effect of grain refinement to 1μm on strength and toughness of dual-phase steels
Calcagnotto, M. and Ponge, D. and Raabe, D.
MATERIALS SCIENCE AND ENGINEERING A. Volume: 527 (2010) - 2010 • 720
Orientation gradients and geometrically necessary dislocations in ultrafine grained dual-phase steels studied by 2D and 3D EBSD
Calcagnotto, M. and Ponge, D. and Demir, E. and Raabe, D.
MATERIALS SCIENCE AND ENGINEERING A. Volume: 527 (2010) - 2010 • 719
All-nitrogen coordinated amidinato/imido complexes of molybdenum and tungsten: Syntheses and characterization
Gwildies, V. and Thiede, T.B. and Amirjalayer, S. and Alsamman, L. and Devi, A. and Fischer, R.A.
INORGANIC CHEMISTRY. Volume: 49 (2010) - 2010 • 718
Solution growth of a binary icosahedral quasicrystal of Sc12 Zn88
Canfield, P.C. and Caudle, M.L. and Ho, C.-S. and Kreyssig, A. and Nandi, S. and Kim, M.G. and Lin, X. and Kracher, A. and Dennis, K.W. and McCallum, R.W. and Goldman, A.I.
PHYSICAL REVIEW B - CONDENSED MATTER AND MATERIALS PHYSICS. Volume: 81 (2010) - 2010 • 717
Interplay of magnetic and structural transitions in iron-based pnictide superconductors
Cano, A. and Civelli, M. and Eremin, I. and Paul, I.
PHYSICAL REVIEW B - CONDENSED MATTER AND MATERIALS PHYSICS. Volume: 82 (2010) - 2010 • 716
An integrated catalytic approach to fermentable sugars from cellulose
Rinaldi, R. and Engel, P. and Büchs, J. and Spiess, A.C. and Schüth, F.
CHEMSUSCHEM. Volume: 3 (2010) - 2010 • 715
In situ study of the polar ZnO(0001)-Zn surface in alkaline electrolytes
Valtiner, M. and Torrelles, X. and Pareek, A. and Borodin, S. and Gies, H. and Grundmeier, G.
JOURNAL OF PHYSICAL CHEMISTRY C. Volume: 114 (2010) - 2010 • 714
Focused ion beam/scanning electron microscopy tomography and conventional transmission electron microscopy assessment of Ni4Ti3 morphology in compression-aged Ni-rich Ni-Ti single crystals
Cao, S. and Somsen, C. and Croitoru, M. and Schryvers, D. and Eggeler, G.
SCRIPTA MATERIALIA. Volume: 62 (2010) - 2010 • 713
Synthesis and characterization of lamellar and fibre-reinforced NiAl-Mo and NiAl-Cr
Haenschke, T. and Gali, A. and Heilmaier, M. and Krüger, M. and Bei, H. and George, E.P.
JOURNAL OF PHYSICS: CONFERENCE SERIES. Volume: 240 (2010) - 2010 • 712
Comparison of finite element and fast Fourier transform crystal plasticity solvers for texture prediction
Liu, B. and Raabe, D. and Roters, F. and Eisenlohr, P. and Lebensohn, R.A.
MODELLING AND SIMULATION IN MATERIALS SCIENCE AND ENGINEERING. Volume: 18 (2010) - 2010 • 711
Which controls the depolymerization of cellulose in ionic liquids: The solid acid catalyst or cellulose?
Rinaldi, R. and Meine, N. and vom Stein, J. and Palkovits, R. and Schüth, F.
CHEMSUSCHEM. Volume: 3 (2010) - 2010 • 710
Hydrogen adsorption on polar ZnO(0001)-Zn: Extending equilibrium surface phase diagrams to kinetically stabilized structures
Valtiner, M. and Todorova, M. and Neugebauer, J.
PHYSICAL REVIEW B - CONDENSED MATTER AND MATERIALS PHYSICS. Volume: 82 (2010) - 2010 • 709
Effect of strain hardening on texture development in cold rolled Al-Mg alloy
Liu, W.C. and Man, C.-S. and Raabe, D.
MATERIALS SCIENCE AND ENGINEERING A. Volume: 527 (2010) - 2010 • 708
Enhanced neovascularization of dermis substitutes via low-pressure plasma-mediated surface activation
Ring, A. and Langer, S. and Schaffran, A. and Stricker, I. and Awakowicz, P. and Steinau, H.-U. and Hauser, J.
BURNS. Volume: 36 (2010) - 2010 • 707
A review of crystallographic textures in chemical vapor-deposited diamond films
Liu, T. and Raabe, D. and Mao, W.-M.
SIGNAL, IMAGE AND VIDEO PROCESSING. Volume: 4 (2010) - 2010 • 706
Optical probing of spin dynamics of two-dimensional and bulk electrons in a GaAs/AlGaAs heterojunction system
Rizo, P.J. and Pugzlys, A. and Slachter, A. and Denega, S.Z. and Reuter, D. and Wieck, A.D. and Van Loosdrecht, P.H.M. and Van Der Wal, C.H.
NEW JOURNAL OF PHYSICS. Volume: 12 (2010) - 2010 • 705
Signatures of nonadiabatic O2 dissociation at Al(111): First-principles fewest-switches study
Carbogno, C. and Behler, J. and Reuter, K. and Groß, A.
PHYSICAL REVIEW B - CONDENSED MATTER AND MATERIALS PHYSICS. Volume: 81 (2010) - 2010 • 704
From glycerol to allyl alcohol: Iron oxide catalyzed dehydration and consecutive hydrogen transfer
Liu, Y. and Tüysüz, H. and Jia, C.-J. and Schwickardi, M. and Rinaldi, R. and Lu, A.-H. and Schmidt, W. and Schüth, F.
CHEMICAL COMMUNICATIONS. Volume: 46 (2010) - 2010 • 703
Suspension plasma spraying: Process characteristics and applications
Vaßen, R. and Kaner, H. and Mauer, G. and Stöver, D.
JOURNAL OF THERMAL SPRAY TECHNOLOGY. Volume: 19 (2010) - 2010 • 702
Highly active iron oxide supported gold catalysts for CO oxidation: How small must the gold nanoparticles be?
Liu, Y. and Jia, C.-N. and Yamasaki, J. and Terasaki, O. and Schüth, F.
ANGEWANDTE CHEMIE - INTERNATIONAL EDITION. Volume: 49 (2010) - 2010 • 701
Functionally graded thermal barrier coatings with improved reflectivity and high-temperature capability
Vassen, R. and Kassner, H. and Stuke, A. and Mack, D.E. and Jarligo, M.O. and Stöver, D.
MATERIALS SCIENCE FORUM. Volume: 631-632 (2010) - 2010 • 700
Structural characterization of a Cu/MgO(001) interface using C S-corrected HRTEM
Cazottes, S. and Zhang, Z.L. and Daniel, R. and Chawla, J.S. and Gall, D. and Dehm, G.
THIN SOLID FILMS. Volume: 519 (2010) - 2010 • 699
Overview on advanced thermal barrier coatings
Vaßen, R. and Jarligo, M.O. and Steinke, T. and Mack, D.E. and Stöver, D.
SURFACE AND COATINGS TECHNOLOGY. Volume: 205 (2010) - 2010 • 698
Diffusion coefficients in olivine, wadsleyite and ringwoodite
Chakraborty, S.
REVIEWS IN MINERALOGY AND GEOCHEMISTRY. Volume: 72 (2010) - 2010 • 697
The reweighted path ensemble
Rogal, J. and Lechner, W. and Juraszek, J. and Ensing, B. and Bolhuis, P.G.
JOURNAL OF CHEMICAL PHYSICS. Volume: 133 (2010) - 2010 • 696
First-row transition metal catalyzed reduction of carbonyl functionalities: A mechanistic perspective
Chakraborty, S. and Guan, H.
DALTON TRANSACTIONS. Volume: 39 (2010) - 2010 • 695
Thermodynamic properties of cementite (Fe3 C)
Hallstedt, B. and Djurovic, D. and von Appen, J. and Dronskowski, R. and Dick, A. and Körmann, F. and Hickel, T. and Neugebauer, J.
CALPHAD: COMPUTER COUPLING OF PHASE DIAGRAMS AND THERMOCHEMISTRY. Volume: 34 (2010) - 2010 • 694
On the efficiency of biased sampling of the multiple state path ensemble
Rogal, J. and Bolhuis, P.G.
JOURNAL OF CHEMICAL PHYSICS. Volume: 133 (2010) - 2010 • 693
An efficient nickel catalyst for the reduction of carbon dioxide with a borane
Chakraborty, S. and Zhang, J. and Krause, J.A. and Guan, H.
JOURNAL OF THE AMERICAN CHEMICAL SOCIETY. Volume: 132 (2010) - 2010 • 692
The ferromagnetic shape memory system Fe-Pd-Cu
Hamann, S. and Gruner, M.E. and Irsen, S. and Buschbeck, J. and Bechtold, C. and Kock, I. and Mayr, S.G. and Savan, A. and Thienhaus, S. and Quandt, E. and Fähler, S. and Entel, P. and Ludwig, Al.
ACTA MATERIALIA. Volume: 58 (2010) - 2010 • 691
Spatially and size selective synthesis of Fe-based nanoparticles on ordered mesoporous supports as highly active and stable catalysts for ammonia decomposition
Lu, A.-H. and Nitz, J.-J. and Comotti, M. and Weidenthaler, C. and Schlichte, K. and Lehmann, C.W. and Terasaki, O. and Schüth, F.
JOURNAL OF THE AMERICAN CHEMICAL SOCIETY. Volume: 132 (2010) - 2010 • 690
Phytochrome as Molecular Machine: Revealing Chromophore Action during the Pfr -> Pr Photoconversion by Magic-Angle Spinning NMR Spectroscopy
Rohmer, T. and Lang, C. and Bongards, C. and Gupta, K. B. S. S. and Neugebauer, J. and Hughes, J. and Gartner, W. and Matysik, J.
JOURNAL OF THE AMERICAN CHEMICAL SOCIETY. Volume: 132 (2010) - 2010 • 689
Co-adsorption processes, kinetics and quantum mechanical modelling of nanofilm semiconductor gas sensors
Velasco-Vélez, J.-J. and Kunze, U. and Haas, T. and Doll, T.
PHYSICA STATUS SOLIDI (A) APPLICATIONS AND MATERIALS SCIENCE. Volume: 207 (2010) - 2010 • 688
Easy synthesis of hollow polymer, carbon, and graphitized microspheres
Lu, A.-H. and Li, W.-C. and Hao, G.-P. and Spliethoff, B. and Bongard, H.-J. and Schaack, B.B. and Schüth, F.
ANGEWANDTE CHEMIE - INTERNATIONAL EDITION. Volume: 49 (2010) - 2010 • 687
Anomalous lattice dynamics and thermal properties of supported size- and shape-selected Pt nanoparticles
Roldan Cuenya, B. and Frenkel, A.I. and Mostafa, S. and Behafarid, F. and Croy, J.R. and Ono, L.K. and Wang, Q.
PHYSICAL REVIEW B - CONDENSED MATTER AND MATERIALS PHYSICS. Volume: 82 (2010) - 2010 • 686
Unusual disorder effects in superconducting LaFeAs1-δ O0.9 F0.1 as revealed by 75As NMR spectroscopy
Hammerath, F. and Drechsler, S.-L. and Grafe, H.-J. and Lang, G. and Fuchs, G. and Behr, G. and Eremin, I. and Korshunov, M.M. and Büchner, B.
PHYSICAL REVIEW B - CONDENSED MATTER AND MATERIALS PHYSICS. Volume: 81 (2010) - 2010 • 685
Solving the structure of size-selected Pt nanocatalysts synthesized by inverse micelle encapsulation
Roldan Cuenya, B. and Croy, J.R. and Mostafa, S. and Behafarid, F. and Li, L. and Zhang, Z. and Yang, J.C. and Wang, Q. and Frenkel, A.I.
JOURNAL OF THE AMERICAN CHEMICAL SOCIETY. Volume: 132 (2010) - 2010 • 684
Theoretical investigation of the Pt3Al ground state
Chauke, H.R. and Minisini, B. and Drautz, R. and Nguyen-Manh, D. and Ngoepe, P.E. and Pettifor, D.G.
INTERMETALLICS. Volume: 18 (2010) - 2010 • 683
Development of Mn-Cr-(C-N) corrosion resistant twinning induced plasticity steels: Thermodynamic and diffusion calculations, production, and characterization
Roncery, L.M. and Weber, S. and Theisen, W.
METALLURGICAL AND MATERIALS TRANSACTIONS A: PHYSICAL METALLURGY AND MATERIALS SCIENCE. Volume: 41 (2010) - 2010 • 682
Enhanced sequential carrier capture into individual quantum dots and quantum posts controlled by surface acoustic waves
Völk, S. and Schülein, F.J.R. and Knall, F. and Reuter, D. and Wieck, A.D. and Truong, T.A. and Kim, H. and Petroff, P.M. and Wixforth, A. and Krenner, H.J.
NANO LETTERS. Volume: 10 (2010) - 2010 • 681
Inductorless low-voltage and low-power wideband mixer for multistandard receivers
Hampel, S.K. and Schmitz, O. and Tiebout, M. and Rolfes, I.
IEEE TRANSACTIONS ON MICROWAVE THEORY AND TECHNIQUES. Volume: 58 (2010) - 2010 • 680
On the reticular construction concept of covalent organic frameworks
Lukose, B. and Kuc, A. and Frenzel, J. and Heine, T.
BEILSTEIN JOURNAL OF NANOTECHNOLOGY. Volume: 1 (2010) - 2010 • 679
Synthesis and characterization of ZnO nanowires for nanosensor applications
Lupan, O. and Emelchenko, G.A. and Ursaki, V.V. and Chai, G. and Redkin, A.N. and Gruzintsev, A.N. and Tiginyanu, I.M. and Chow, L. and Ono, L.K. and Roldan Cuenya, B. and Heinrich, H. and Yakimov, E.E.
MATERIALS RESEARCH BULLETIN. Volume: 45 (2010) - 2010 • 678
Synthesis and characterization of ag- or sb-doped zno nanorods by a facile hydrothermal route
Lupan, O. and Chow, L. and Ono, L.K. and Cuenya, B.R. and Chai, G. and Khallaf, H. and Park, S. and Schulte, A.
JOURNAL OF PHYSICAL CHEMISTRY C. Volume: 114 (2010) - 2010 • 677
Inactivation of bacteria and biomolecules by low-pressure plasma discharges
Von Keudell, A. and Awakowicz, P. and Benedikt, J. and Raballand, V. and Yanguas-Gil, A. and Opretzka, J. and Flötgen, C. and Reuter, R. and Byelykh, L. and Halfmann, H. and Stapelmann, K. and Denis, B. and Wunderlich, J. and Muranyi, P. and Rossi, F. and Kylián, O. and Hasiwa, N. and Ruiz, A. and Rauscher, H. and Sirghi, L. and Comoy, E. and Dehen, C. and Challier, L. and Deslys, J.P.
PLASMA PROCESSES AND POLYMERS. Volume: 7 (2010) - 2010 • 676
Fabrication and characterisation of an induced ambipolar device on AlGaAs/GaAs Heterostructures
Chen, J.C.H. and Klochan, O. and Micolich, A.P. and Hamilton, A.R. and Das Gupta, K. and Sfigakis, F. and Ritchie, D.A. and Trunov, K.V. and Reuter, D. and Wieck, A.D.
CONFERENCE ON OPTOELECTRONIC AND MICROELECTRONIC MATERIALS AND DEVICES, PROCEEDINGS, COMMAD. Volume: (2010) - 2010 • 675
Effects of annealing on properties of ZnO thin films prepared by electrochemical deposition in chloride medium
Lupan, O. and Pauporté, T. and Chow, L. and Viana, B. and Pellé, F. and Ono, L.K. and Roldan Cuenya, B. and Heinrich, H.
APPLIED SURFACE SCIENCE. Volume: 256 (2010) - 2010 • 674
Generation and performance of special quasirandom structures for studying the elastic properties of random alloys: Application to Al-Ti
Von Pezold, J. and Dick, A. and Friák, M. and Neugebauer, J.
PHYSICAL REVIEW B - CONDENSED MATTER AND MATERIALS PHYSICS. Volume: 81 (2010) - 2010 • 673
Observation of orientation- and k-dependent zeeman spin-splitting in hole quantum wires on (100)-oriented AlGaAs/GaAs heterostructures
Chen, J.C.H. and Klochan, O. and Micolich, A.P. and Hamilton, A.R. and Martin, T.P. and Ho, L.H. and Zülicke, U. and Reuter, D. and Wieck, A.D.
NEW JOURNAL OF PHYSICS. Volume: 12 (2010) - 2010 • 672
Ballistic induced hole quantum wires fabricated on a (1 0 0)-oriented AlGaAs/GaAs heterostructure
Chen, J.H. and Klochan, O. and Micolich, A.P. and Hamilton, A.R. and Reuter, D. and Wieck, A.D.
PHYSICA E: LOW-DIMENSIONAL SYSTEMS AND NANOSTRUCTURES. Volume: 42 (2010) - 2010 • 671
Synthesis of defect-free single-phase bars of high-melting Laves phases through modified cold crucible levitation melting
Voß, S. and Stein, F. and Palm, M. and Raabe, D.
MATERIALS SCIENCE AND ENGINEERING A. Volume: 527 (2010) - 2010 • 670
Time-resolved Hanle effect in (In,Ga)As/GaAs quantum dots
Cherbunin, R.V. and Verbin, S.Yu. and Flisinski, K. and Gerlovin, I.Ya. and Ignatiev, I.V. and Vishnevsky, D.V. and Reuter, D. and Wieck, A.D. and Yakovlev, D.R. and Bayer, M.
JOURNAL OF PHYSICS: CONFERENCE SERIES. Volume: 245 (2010) - 2010 • 669
Plasma mediated collagen-I-coating of metal implant materials to improve biocompatibility
Hauser, J. and Koeller, M. and Bensch, S. and Halfmann, H. and Awakowicz, P. and Steinau, H.-U. and Esenwein, S.
JOURNAL OF BIOMEDICAL MATERIALS RESEARCH - PART A. Volume: 94 (2010) - 2010 • 668
Crystal Plasticity Finite Element Methods: In Materials Science and Engineering
Roters, F. and Eisenlohr, P. and Bieler, T.R. and Raabe, D.
CRYSTAL PLASTICITY FINITE ELEMENT METHODS: IN MATERIALS SCIENCE AND ENGINEERING. Volume: (2010) - 2010 • 667
Can insulating the gates lead us to stable modulation-doped hole quantum devices?
Waddington, D. and Burke, A.M. and Fricke, S. and Tan, H.H. and Jagadish, C. and Hamilton, A.R. and Trunov, K. and Reuter, D. and Wieck, A.D. and Micolich, A.P.
CONFERENCE ON OPTOELECTRONIC AND MICROELECTRONIC MATERIALS AND DEVICES, PROCEEDINGS, COMMAD. Volume: (2010) - 2010 • 666
Optimization of mesh-based anodes for direct methanol fuel cells
Chetty, R. and Scott, K. and Kundu, S. and Muhler, M.
JOURNAL OF FUEL CELL SCIENCE AND TECHNOLOGY. Volume: 7 (2010) - 2010 • 665
Nanofilm metal layers as vacuum quality sensors
Mader, S. and Haas, T. and Kunze, U. and Doll, T.
PROCEDIA ENGINEERING. Volume: 5 (2010) - 2010 • 664
Overview of constitutive laws, kinematics, homogenization and multiscale methods in crystal plasticity finite-element modeling: Theory, experiments, applications
Roters, F. and Eisenlohr, P. and Hantcherli, L. and Tjahjanto, D.D. and Bieler, T.R. and Raabe, D.
ACTA MATERIALIA. Volume: 58 (2010) - 2010 • 663
Self-energy effects and electron-phonon coupling in Fe-As superconductors
Choi, K.-Y. and Lemmens, P. and Eremin, I. and Zwicknagl, G. and Berger, H. and Sun, G.L. and Sun, D.L. and Lin, C.T.
JOURNAL OF PHYSICS CONDENSED MATTER. Volume: 22 (2010) - 2010 • 662
Angle-resolved specific heat in iron-based superconductors: The case for a nodeless extended s-wave gap
Chubukov, A.V. and Eremin, I.
PHYSICAL REVIEW B - CONDENSED MATTER AND MATERIALS PHYSICS. Volume: 82 (2010) - 2010 • 661
A micromechanical model for polycrystalline shape memory alloys - Formulation and numerical validation
Heinen, R. and Hackl, K.
IUTAM BOOKSERIES. Volume: 21 (2010) - 2010 • 660
Effect of low-temperature precipitation on the transformation characteristics of Ni-rich NiTi shape memory alloys during thermal cycling
Wagner, M.F.-X. and Dey, S.R. and Gugel, H. and Frenzel, J. and Somsen, C. and Eggeler, G.
INTERMETALLICS. Volume: 18 (2010) - 2010 • 659
Investigation of the fatigue behavior of Al thin films with different microstructure
Heinz, W. and Pippan, R. and Dehm, G.
MATERIALS SCIENCE AND ENGINEERING A. Volume: 527 (2010) - 2010 • 658
Real time monitoring of micro and nano particles, blood phantoms in situ by optical micro resonance methods
Saetchnikov, V.A. and Tcheriavskaia, E.A. and Schweiger, G. and Ostendorf, A.
CONFERENCE PROCEEDINGS - 5TH INTERNATIONAL CONFERENCE ON ADVANCED OPTOELECTRONICS AND LASERS, CAOL' 2010. Volume: (2010) - 2010 • 657
Magnetic coupling mechanisms in particle/thin film composite systems
Confalonieri, G.A.B. and Szary, P. and Mishra, D. and Benitez, M.J. and Feyen, M. and Lu, A.H. and Agudo, L. and Eggeler, G. and Petracic, O. and Zabel, H.
BEILSTEIN JOURNAL OF NANOTECHNOLOGY. Volume: 1 (2010) - 2010 • 656
Pt-Ag catalysts as cathode material for oxygen-depolarized electrodes in hydrochloric acid electrolysis
Maljusch, A. and Nagaiah, T.C. and Schwamborn, S. and Bron, M. and Schuhmann, W.
ANALYTICAL CHEMISTRY. Volume: 82 (2010) - 2010 • 655
Optical micro resonance based sensor schemes for detection and identification of nano particles and biological agents in situ
Saetchnikov, V.A. and Tcherniavskaia, E.A. and Schweiger, G. and Ostendorf, A.
PROCEEDINGS OF SPIE - THE INTERNATIONAL SOCIETY FOR OPTICAL ENGINEERING. Volume: 7712 (2010) - 2010 • 654
Ultrasonic investigation on the distorted diamond chain compound Azurite
Cong, P.T. and Wolf, B. and Tutsch, U. and Remović-Langer, K. and Schreuer, J. and Süllow, S. and Lang, M.
JOURNAL OF PHYSICS: CONFERENCE SERIES. Volume: 200 (2010) - 2010 • 653
Flexibility and Sorption Selectivity in Rigid Metal-Organic Frameworks: The Impact of Ether-Functionalised Linkers
Henke, S. and Schmid, R. and Grunwaldt, J. D. and Fischer, R. A.
CHEMISTRY-A EUROPEAN JOURNAL. Volume: 16 (2010) - 2010 • 652
Isomerization of an azobenzene derivative on a thin insulating layer by inelastically tunneling electrons
Safiei, A. and Henzl, J. and Morgenstern, K.
PHYSICAL REVIEW LETTERS. Volume: 104 (2010) - 2010 • 651
Adhesion energies of Cr thin films on polyimide determined from buckling: Experiment and model
Cordill, M.J. and Fischer, F.D. and Rammerstorfer, F.G. and Dehm, G.
ACTA MATERIALIA. Volume: 58 (2010) - 2010 • 650
Fracture and delamination of chromium thin films on polymer substrates
Cordill, M.J. and Taylor, A. and Schalko, J. and Dehm, G.
METALLURGICAL AND MATERIALS TRANSACTIONS A: PHYSICAL METALLURGY AND MATERIALS SCIENCE. Volume: 41 (2010) - 2010 • 649
Combinatorial investigation of Hf-Ta thin films and their anodic oxides
Mardare, A.I. and Ludwig, Al. and Savan, A. and Wieck, A.D. and Hassel, A.W.
ELECTROCHIMICA ACTA. Volume: 55 (2010) - 2010 • 648
The time scales of magma mixing and mingling involving primitive melts and melt-mush interaction at mid-ocean ridges
Costa, F. and Coogan, L.A. and Chakraborty, S.
CONTRIBUTIONS TO MINERALOGY AND PETROLOGY. Volume: 159 (2010) - 2010 • 647
An electron induced two-dimensional switch made of azobenzene derivatives anchored in supramolecular assemblies
Henzl, J. and Morgenstern, K.
PHYSICAL CHEMISTRY CHEMICAL PHYSICS. Volume: 12 (2010) - 2010 • 646
A 2D electron gas for studies on tunneling dynamics and charge storage in self-assembled quantum dots
Marquardt, B. and Moujib, H. and Lorke, A. and Reuter, D. and Wieck, A.D. and Geller, M.
LECTURE NOTES OF THE INSTITUTE FOR COMPUTER SCIENCES, SOCIAL-INFORMATICS AND TELECOMMUNICATIONS ENGINEERING. Volume: 36 LNICST (2010) - 2010 • 645
Using Ab initio calculations in designing bcc MgLi-X alloys for ultra-lightweight applications
Counts, W.A. and Friák, M. and Raabe, D. and Neugebauer, J.
ADVANCED ENGINEERING MATERIALS. Volume: 12 (2010) - 2010 • 644
A two-dimensional electron gas as a sensitive detector to observe the charge carrier dynamics of self-assembled QDs
Marquardt, B. and Geller, M. and Lorke, A. and Reuter, D. and Wieck, A.D.
PHYSICA E: LOW-DIMENSIONAL SYSTEMS AND NANOSTRUCTURES. Volume: 42 (2010) - 2010 • 643
Ab Initio guided design of bcc ternary Mg-Li-X (X=Ca, Al, Si, Zn, Cu) alloys for ultra-lightweight applications
Counts, W.A. and Friák, M. and Raabe, D. and Neugebauer, J.
ADVANCED ENGINEERING MATERIALS. Volume: 12 (2010) - 2010 • 642
Plane-wave implementation of the real-space k ṡ p formalism and continuum elasticity theory
Marquardt, O. and Boeck, S. and Freysoldt, C. and Hickel, T. and Neugebauer, J.
COMPUTER PHYSICS COMMUNICATIONS. Volume: 181 (2010) - 2010 • 641
Synthesis, characterization and structure analysis of UZM-22, a MEI-type zeolite framework structure
Wang, G. and Marler, B. and Gies, H. and Fyfe, C.A. and Sidhu, P. and Yilmaz, B. and Müller, U.
MICROPOROUS AND MESOPOROUS MATERIALS. Volume: 132 (2010) - 2010 • 640
Spin Noise of Electrons and Holes in Self-Assembled Quantum Dots
Crooker, S.A. and Brandt, J. and Sandfort, C. and Greilich, A. and Yakovlev, D.R. and Reuter, D. and Wieck, A.D. and Bayer, M.
PHYSICAL REVIEW LETTERS. Volume: 104 (2010) - 2010 • 639
Comprehensive investigations of the supersolidus liquid-phase sintering of two plastic mold steels
Hill, H. and Weber, S. and Siebert, S. and Huth, S. and Theisen, W.
METALLURGICAL AND MATERIALS TRANSACTIONS A: PHYSICAL METALLURGY AND MATERIALS SCIENCE. Volume: 41 (2010) - 2010 • 638
Nanostructures in p-GaAs with improved tunability
Csontos, M. and Komijani, Y. and Shorubalko, I. and Ensslin, K. and Reuter, D. and Wieck, A.D.
APPLIED PHYSICS LETTERS. Volume: 97 (2010) - 2010 • 637
Synthesis and catalytic properties of metal nanoparticles: Size, shape, support, composition, and oxidation state effects
Cuenya, B.R.
THIN SOLID FILMS. Volume: 518 (2010) - 2010 • 636
Microstructure and wear properties of novel sintered cold work steel and related particle reinforced composite materials
Weber, S. and Li, J.R. and Theisen, W.
MATERIALS SCIENCE AND TECHNOLOGY. Volume: 26 (2010) - 2010 • 635
EBSD study of substructure and texture formation in dual-phase steel sheets for semi-finished products
Masimov, M. and Peranio, N. and Springub, B. and Roters, F. and Raabe, D.
SOLID STATE PHENOMENA. Volume: 160 (2010) - 2010 • 634
Efficient injection-type ballistic rectification in Si/SiGe cross junctions
Salloch, D. and Wieser, U. and Kunze, U. and Hackbarth, T.
PHYSICA E: LOW-DIMENSIONAL SYSTEMS AND NANOSTRUCTURES. Volume: 42 (2010) - 2010 • 633
Theoretical investigation of {110} generalized stacking faults and their relation to dislocation behavior in perovskite oxides
Hirel, P. and Marton, P. and Mrovec, M. and Elsässer, C.
ACTA MATERIALIA. Volume: 58 (2010) - 2010 • 632
Ionothermal synthesis and crystal structure of a new layered nickel(II) diphosphate, DRM-1
Wei, Y. and Gies, H. and Tian, Z. and Marler, B. and Xu, Y. and Wang, L. and Ma, H. and Pei, R. and Li, K. and Wang, B.
INORGANIC CHEMISTRY COMMUNICATIONS. Volume: 13 (2010) - 2010 • 631
Engineered Tungsten Oxy-Nitride Thin Film Materials for Photocatalytical Water Splitting Fabricated by MOCVD
Cwik, S. and Milanov, A. P. and Gwildies, V. and Thiede, T. B. and Vidyarthi, V. S. and Savan, A. and Meyer, R. and Becker, H. W. and Rogalla, D. and Ludwig, Al. and Fischer, R. A. and Devi, A.
ADVANCED ORGANIC AND INORGANIC MATERIALS FOR ELECTROCHEMICAL POWER SOURCES. Volume: 28 (2010) - 2010 • 630
Annealing behavior of ferritic-martensitic 9%Cr-ODS-Eurofer steel
Sandim, H.R.Z. and Renzetti, R.A. and Padilha, A.F. and Raabe, D. and Klimenkov, M. and Lindau, R. and Möslang, A.
MATERIALS SCIENCE AND ENGINEERING A. Volume: 527 (2010) - 2010 • 629
Ionothermal synthesis of an aluminophosphate molecular sieve with 20-ring pore openings
Wei, Y. and Tian, Z. and Gies, H. and Xu, R. and Ma, H. and Pei, R. and Zhang, W. and Xu, Y. and Wang, L. and Li, K. and Wang, B. and Wen, G. and Lin, L.
ANGEWANDTE CHEMIE - INTERNATIONAL EDITION. Volume: 49 (2010) - 2010 • 628
Micron-sized fracture experiments on amorphous SiOx films and SiOx/SiNx multi-layers
Matoy, K. and Schönherr, H. and Detzel, T. and Dehm, G.
THIN SOLID FILMS. Volume: 518 (2010) - 2010 • 627
Electron backscatter diffraction study of Nb3Sn superconducting multifilamentary wire
Sandim, M.J.R. and Sandim, H.R.Z. and Zaefferer, S. and Raabe, D. and Awaji, S. and Watanabe, K.
SCRIPTA MATERIALIA. Volume: 62 (2010) - 2010 • 626
Thin and dense ceramic coatings by plasma spraying at very low pressure
Mauer, G. and Vaßen, R. and Stöver, D.
JOURNAL OF THERMAL SPRAY TECHNOLOGY. Volume: 19 (2010) - 2010 • 625
Process diagnostics in suspension plasma spraying
Mauer, G. and Guignard, A. and Vaßen, R. and Stöver, D.
SURFACE AND COATINGS TECHNOLOGY. Volume: 205 (2010) - 2010 • 624
Localization events and microstructural evolution in ultra-fine grained NiTi shape memory alloys during thermo-mechanical loading
Schaefer, A. and Wagner, M.F.-X. and Pelegrina, J.L. and Olbricht, J. and Eggeler, G.
ADVANCED ENGINEERING MATERIALS. Volume: 12 (2010) - 2010 • 623
A hybrid modeling concept for ultra low cycle fatigue of metallic structures based on micropore damage and unit cell models
Hommel, J.-H. and Meschke, G.
INTERNATIONAL JOURNAL OF FATIGUE. Volume: 32 (2010) - 2010 • 622
High resolution spectroscopy with a microparticle array sensor
Weigel, T. and Nett, R. and Schweiger, G. and Ostendorf, A.
PROCEEDINGS OF SPIE - THE INTERNATIONAL SOCIETY FOR OPTICAL ENGINEERING. Volume: 7726 (2010) - 2010 • 621
The influence of the surface state onto the distance distribution of single molecules and small molecular clusters
Mehlhorn, M. and Simic-Milosevic, V. and Jaksch, S. and Scheier, P. and Morgenstern, K.
SURFACE SCIENCE. Volume: 604 (2010) - 2010 • 620
A new approach to the stability of time-delay systems in the sense of non-Lyapunov delay-independent and delay-dependent criteria
Debeljkovic, D.Lj. and Nestorovic, T. and Buzurovic, I.M. and Dimitrijevic, N.J.
SIISY 2010 - 8TH IEEE INTERNATIONAL SYMPOSIUM ON INTELLIGENT SYSTEMS AND INFORMATICS. Volume: (2010) - 2010 • 619
Diffusion and dimer formation of CO molecules induced by femtosecond laser pulses
Mehlhorn, M. and Gawronski, H. and Morgenstern, K.
PHYSICAL REVIEW LETTERS. Volume: 104 (2010) - 2010 • 618
Influence of the growth conditions of epitaxial graphene on the film topography and the electron transport properties
Weingart, S. and Bock, C. and Kunze, U. and Emtsev, K.V. and Seyller, Th. and Ley, L.
PHYSICA E: LOW-DIMENSIONAL SYSTEMS AND NANOSTRUCTURES. Volume: 42 (2010) - 2010 • 617
An intentionally positioned (In,Ga)As quantum dot in a micron sized light emitting diode
Mehta, M. and Reuter, D. and Wieck, A.D. and Michaelis De Vasconcellos, S. and Zrenner, A. and Meier, C.
APPLIED PHYSICS LETTERS. Volume: 97 (2010) - 2010 • 616
Formation, binding, and stability of O-Ag-CO2-Ag-O compounds on Ag(100) investigated by low temperature scanning tunneling microscopy and manipulation
Hsieh, M.-F. and Li, H.-D. and Lin, D.-S. and Morgenstern, K.
JOURNAL OF PHYSICAL CHEMISTRY C. Volume: 114 (2010) - 2010 • 615
Intentionally positioned self-assembled InAs quantum dots in an electroluminescent pin junction diode
Mehta, M. and Reuter, D. and Melnikov, A. and Wieck, A.D. and Michaelis De Vasconcellos, S. and Baumgarten, T. and Zrenner, A. and Meier, C.
PHYSICA E: LOW-DIMENSIONAL SYSTEMS AND NANOSTRUCTURES. Volume: 42 (2010) - 2010 • 614
Determination of dissociative fragment-adsorbate interaction energy during chemisorption of the diatomic molecule HCI on Si(100)
Hsieh, M.-F. and Cheng, J.-Y. and Yang, J.-C. and Lin, D.-S. and Morgenstern, K. and Pai, W.-W.
PHYSICAL REVIEW B - CONDENSED MATTER AND MATERIALS PHYSICS. Volume: 81 (2010) - 2010 • 613
Development of a method to determine Burgers vectors from atomistic data
Hua, J. and Hartmaier, A.
JOURNAL OF PHYSICS: CONFERENCE SERIES. Volume: 240 (2010) - 2010 • 612
Particle methods on multicore architectures: Experiences and future plans
Schiller, A. and Sutmann, G. and Martinell, L. and Bellens, P. and Badia, R.
AIP CONFERENCE PROCEEDINGS. Volume: 1281 (2010) - 2010 • 611
Determining Burgers vectors and geometrically necessary dislocation densities from atomistic data
Hua, J. and Hartmaier, A.
MODELLING AND SIMULATION IN MATERIALS SCIENCE AND ENGINEERING. Volume: 18 (2010) - 2010 • 610
Carbon nanotubes modified with electrodeposited metal porphyrins and phenanthrolines for electrocatalytic applications
Schilling, T. and Okunola, A. and Masa, J. and Schuhmann, W. and Bron, M.
ELECTROCHIMICA ACTA. Volume: 55 (2010) - 2010 • 609
Measurement of Ba-densities close to the electrode in an HPS-lamp by broadband absorption spectroscopy with a UHP-lamp
Westermeier, M. and Reinelt, J. and Luijks, G. and Mentel, J. and Awakowicz, P.
JOURNAL OF PHYSICS D: APPLIED PHYSICS. Volume: 43 (2010) - 2010 • 608
Mechanical and microstructural single-crystal Bauschinger effects: Observation of reversible plasticity in copper during bending
Demir, E. and Raabe, D.
ACTA MATERIALIA. Volume: 58 (2010) - 2010 • 607
Disparate tendency of stress evolution of thin and thick electroplated cu films at room temperature
Huang, R. and Robl, W. and Dehm, G. and Ceric, H. and Detzel, T.
PROCEEDINGS OF THE INTERNATIONAL SYMPOSIUM ON THE PHYSICAL AND FAILURE ANALYSIS OF INTEGRATED CIRCUITS, IPFA. Volume: (2010) - 2010 • 606
Stearate-Based Cu Colloids in Methanol Synthesis: Structural Changes Driven by Strong Metal-Support Interactions
Schimpf, S. and Rittermeier, A. and Zhang, X. and Li, Z.-A. and Spasova, M. and van den Berg, M.W.E. and Farle, M. and Wang, Y. and Fischer, R.A. and Muhler, M.
CHEMCATCHEM. Volume: 2 (2010) - 2010 • 605
Bending of single crystal microcantilever beams of cube orientation: Finite element model and experiments
Demir, E. and Roters, F. and Raabe, D.
JOURNAL OF THE MECHANICS AND PHYSICS OF SOLIDS. Volume: 58 (2010) - 2010 • 604
Stress, sheet resistance, and microstructure evolution of electroplated cu films during self-annealing
Huang, R. and Robl, W. and Ceric, H. and Detzel, T. and Dehm, G.
IEEE TRANSACTIONS ON DEVICE AND MATERIALS RELIABILITY. Volume: 10 (2010) - 2010 • 603
Femtosecond passively modelocked diode laser with intracavity dispersion management
Schlauch, T. and Balzer, J.C. and Klehr, A. and Erbert, G. and Tränkle, G. and Hofmann, M.R.
OPTICS EXPRESS. Volume: 18 (2010) - 2010 • 602
The mechanical size effect as a mean-field breakdown phenomenon: Example of microscale single crystal beam bending
Demir, E. and Raabe, D. and Roters, F.
ACTA MATERIALIA. Volume: 58 (2010) - 2010 • 601
Semidilute polymer solutions at equilibrium and under shear flow
Huang, C.-C. and Winkler, R.G. and Sutmann, G. and Gompper, G.
MACROMOLECULES. Volume: 43 (2010) - 2010 • 600
Support effects in the Au-catalyzed CO oxidation - Correlation between activity, oxygen storage capacity, and support reducibility
Widmann, D. and Liu, Y. and Schüth, F. and Behm, R.J.
JOURNAL OF CATALYSIS. Volume: 276 (2010) - 2010 • 599
Cell-level canonical sampling by velocity scaling for multiparticle collision dynamics simulations
Huang, C.C. and Chatterji, A. and Sutmann, G. and Gompper, G. and Winkler, R.G.
JOURNAL OF COMPUTATIONAL PHYSICS. Volume: 229 (2010) - 2010 • 598
Experimental and computer simulation determination of the structural changes occurring through the liquid-glass transition in Cu-Zr alloys
Mendelev, M.I. and Kramer, M.J. and Ott, R.T. and Sordelet, D.J. and Besser, M.F. and Kreyssig, A. and Goldman, A.I. and Wessels, V. and Sahu, K.K. and Kelton, K.F. and Hyers, R.W. and Canepari, S. and Rogers, J.R.
PHILOSOPHICAL MAGAZINE. Volume: 90 (2010) - 2010 • 597
Suppressed spin dephasing for two-dimensional and bulk electrons in GaAs wires due to engineered cancellation of spin-orbit interaction terms
Denega, S.Z. and Last, T. and Liu, J. and Slachter, A. and Rizo, P.J. and Van Loosdrecht, P.H.M. and Van Wees, B.J. and Reuter, D. and Wieck, A.D. and Van Der Wal, C.H.
PHYSICAL REVIEW B - CONDENSED MATTER AND MATERIALS PHYSICS. Volume: 81 (2010) - 2010 • 596
Precise radar filling level measurements in Still pipes
Denicke, E. and Armbrecht, G. and Rolfes, I.
TECHNISCHES MESSEN. Volume: 77 (2010) - 2010 • 595
Accessing ultrashort reaction times in particle formation with SAXS experiments: ZnS precipitation on the microsecond time scale
Schmidt, W. and Bussian, P. and Lindén, M. and Amenitsch, H. and Agren, P. and Tiemann, M. and Schüth, F.
JOURNAL OF THE AMERICAN CHEMICAL SOCIETY. Volume: 132 (2010) - 2010 • 594
Radar distance measurements in circular waveguides involving intermodal dispersion effects
Denicke, E. and Armbrecht, G. and Rolfes, I.
INTERNATIONAL JOURNAL OF MICROWAVE AND WIRELESS TECHNOLOGIES. Volume: 2 (2010) - 2010 • 593
Full-wave rectification based upon hot-electron thermopower
Wiemann, M. and Wieser, U. and Kunze, U. and Reuter, D. and Wieck, A.D.
APPLIED PHYSICS LETTERS. Volume: 97 (2010) - 2010 • 592
NPUA: A new approach for the analysis of computer experiments
Dette, H. and Pepelyshev, A.
CHEMOMETRICS AND INTELLIGENT LABORATORY SYSTEMS. Volume: 104 (2010) - 2010 • 591
Some comments on quasi-birth-and-death processes and matrix measures
Dette, H. and Reuther, B.
JOURNAL OF PROBABILITY AND STATISTICS. Volume: (2010) - 2010 • 590
A single-electrode, dual-potential ferrocene-PNA biosensor for the detection of DNA
Hüsken, N. and Gȩbala, M. and Schuhmann, W. and Metzler-Nolte, N.
CHEMBIOCHEM. Volume: 11 (2010) - 2010 • 589
Determination of argon resonance line emission in an ICP hitting a biological sample
Mertmann, P. and Bibinov, N. and Halfmann, H. and Awakowicz, P.
PLASMA SOURCES SCIENCE AND TECHNOLOGY. Volume: 19 (2010) - 2010 • 588
Optimal designs for estimating the slope of a regression
Dette, H. and Melas, V.B. and Pepelyshev, A.
STATISTICS. Volume: 44 (2010) - 2010 • 587
Shearforce-based constant-distance scanning electrochemical microscopy as fabrication tool for needle-type carbon-fiber nanoelectrodes
Hussien, E.M. and Schuhmann, W. and Schulte, A.
ANALYTICAL CHEMISTRY. Volume: 82 (2010) - 2010 • 586
Minimal area 65nm CMOS amplifier for Ultra-Wideband transmitter applications
Schmitz, O. and Hampel, S.K. and Mertens, K. and Tiebout, M. and Rolfes, I.
2010 IEEE INTERNATIONAL CONFERENCE ON ULTRA-WIDEBAND, ICUWB2010 - PROCEEDINGS. Volume: 1 (2010) - 2010 • 585
Fast, physically-based algorithms for online calculations of texture and anisotropy during fabrication of steel sheets
Winning, M. and Raabe, D.
ADVANCED ENGINEERING MATERIALS. Volume: 12 (2010) - 2010 • 584
Generalized latin hypercube design for computer experiments
Dette, H. and Pepelyshev, A.
TECHNOMETRICS. Volume: 52 (2010) - 2010 • 583
Body effect up- and down-conversion mixer circuits for low-voltage ultra-wideband operation
Schmitz, O. and Hampel, S.K. and Orlob, C. and Tiebout, M. and Rolfes, I.
ANALOG INTEGRATED CIRCUITS AND SIGNAL PROCESSING. Volume: 64 (2010) - 2010 • 582
A Web-Based Tool for Finding Optimal Designs for the Michaelis-Menten Model and an Overview
Dette, H. and Kiss, C. and Wong, W. K.
STATISTICS IN BIOPHARMACEUTICAL RESEARCH. Volume: 2 (2010) - 2010 • 581
Experimental evaluation of photoacoustic coded excitation using unipolar golay codes
Mienkina, M.P. and Friedrich, C.-S. and Gerhardt, N.C. and Wilkening, W.G. and Hofmann, M.R. and Schmitz, G.
IEEE TRANSACTIONS ON ULTRASONICS, FERROELECTRICS, AND FREQUENCY CONTROL. Volume: 57 (2010) - 2010 • 580
Optimal designs for discriminating between dose-response models in toxicology studies
Dette, H. and Pepelyshev, A. and Shpilev, P. and Wong, W.K.
BERNOULLI. Volume: 16 (2010) - 2010 • 579
Multispectral photoacoustic coded excitation imaging using unipolar orthogonal Golay codes
Mienkina, M.P. and Friedrich, C.-S. and Gerhardt, N.C. and Beckmann, M.F. and Schiffner, M.F. and Hofmann, M.R. and Schmitz, G.
OPTICS EXPRESS. Volume: 18 (2010) - 2010 • 578
Optimal designs for random effect models with correlated errors with applications in population pharmacokinetics
Dette, H. and Pepelyshev, A. and Holland-Letz, T.
ANNALS OF APPLIED STATISTICS. Volume: 4 (2010) - 2010 • 577
Anodic oxides on a beta type Nb-Ti alloy and their characterization by electrochemical impedance spectroscopy
Woldemedhin, M.T. and Raabe, D. and Hassel, A.W.
PHYSICA STATUS SOLIDI (A) APPLICATIONS AND MATERIALS SCIENCE. Volume: 207 (2010) - 2010 • 576
A robust test for homoscedasticity in nonparametric regression
Dette, H. and Marchlewski, M.
JOURNAL OF NONPARAMETRIC STATISTICS. Volume: 22 (2010) - 2010 • 575
Solution-Precipitation Creep - Modeling and Extended FE Implementation
Ilic, S. and Hackl, K.
IUTAM SYMPOSIUM ON VARIATIONAL CONCEPTS WITH APPLICATIONS TO THE MECHANICS OF MATERIALS. Volume: 21 (2010) - 2010 • 574
Growth of crystalline Gd2O3 thin films with a high-quality interface on Si(100) by low-temperature H2O-assisted atomic layer deposition
Milanov, A.P. and Xu, K. and Laha, A. and Bugiel, E. and Ranjith, R. and Schwendt, D. and Osten, H.J. and Parala, H. and Fischer, R.A. and Devi, A.
JOURNAL OF THE AMERICAN CHEMICAL SOCIETY. Volume: 132 (2010) - 2010 • 573
A note on all-bias designs with applications in spline regression models
Dette, H. and Melas, V.B.
JOURNAL OF STATISTICAL PLANNING AND INFERENCE. Volume: 140 (2010) - 2010 • 572
Application of the multiscale FEM to the modeling of cancellous bone
Ilic, S. and Hackl, K. and Gilbert, R.
BIOMECHANICS AND MODELING IN MECHANOBIOLOGY. Volume: 9 (2010) - 2010 • 571
Random block matrices and matrix orthogonal polynomials
Dette, H. and Reuther, B.
JOURNAL OF THEORETICAL PROBABILITY. Volume: 23 (2010) - 2010 • 570
Theory of multiband superconductivity in Spin-density-wave metals
Ismer, J.-P. and Eremin, I. and Rossi, E. and Morr, D.K. and Blumberg, G.
PHYSICAL REVIEW LETTERS. Volume: 105 (2010) - 2010 • 569
Roughness-gradient-induced spontaneous motion of droplets on hydrophobic surfaces: A lattice Boltzmann study
Moradi, N. and Varnik, F. and Steinbach, I.
EPL. Volume: 89 (2010) - 2010 • 568
Optimal designs for the emax, log-linear and exponential models
Dette, H. and Kiss, C. and Bevanda, M. and Bretz, F.
BIOMETRIKA. Volume: 97 (2010) - 2010 • 567
Ab initio study of the solubility and kinetics of hydrogen in austenitic high Mn steels
Ismer, L. and Hickel, T. and Neugebauer, J.
PHYSICAL REVIEW B - CONDENSED MATTER AND MATERIALS PHYSICS. Volume: 81 (2010) - 2010 • 566
Single molecule manipulation
Morgenstern, K.
SURFACE AND INTERFACE ANALYSIS. Volume: 42 (2010) - 2010 • 565
A finite sample comparison of nonparametric estimates of the effective dose in quantal bioassay
Dette, H. and Scheder, R.
JOURNAL OF STATISTICAL COMPUTATION AND SIMULATION. Volume: 80 (2010) - 2010 • 564
The observation of exciton-cyclotron resonance in photoluminescence spectra of a two dimensional hole gas
Jadczak, J. and Bryja, L. and Pochocka, P. and Wójs, A. and Misiewicz, J. and Maude, D. and Potemski, M. and Reuter, D. and Wieck, A.
JOURNAL OF PHYSICS: CONFERENCE SERIES. Volume: 210 (2010) - 2010 • 563
Matrix measures on the unit circle, moment spaces, orthogonal polynomials and the Geronimus relations
Dette, H. and Wagener, J.
LINEAR ALGEBRA AND ITS APPLICATIONS. Volume: 432 (2010) - 2010 • 562
Rejoinder: A general approach to D-optimal designs for weighted univariate polynomial regression models
Dette, H. and Trampisch, M.
JOURNAL OF THE KOREAN STATISTICAL SOCIETY. Volume: 39 (2010) - 2010 • 561
Gas-phase synthesis of gradient catalyst libraries consisting of nanoparticles supported on high surface area porous substrates
Xia, W. and Mei, B. and Muhler, M.
NANOSCIENCE AND NANOTECHNOLOGY LETTERS. Volume: 2 (2010) - 2010 • 560
A general approach to D-optimal designs for weighted univariate polynomial regression models
Dette, H. and Trampisch, M.
JOURNAL OF THE KOREAN STATISTICAL SOCIETY. Volume: 39 (2010) - 2010 • 559
Growth and characterization of ti-ta-o thin films on si substrates by liquid injection MOCVD for high-k applications from modified titanium and tantalum precursors
Devi, A. and Hellwig, M. and Barreca, D. and Parala, H. and Thomas, R. and Becker, H.-W. and Katiyar, R.S. and Fischer, R.A. and Tondello, E.
CHEMICAL VAPOR DEPOSITION. Volume: 16 (2010) - 2010 • 558
Synthesis and structure analysis of RUB-50, an LEV-type aluminosilicate zeolite
Yamamoto, K. and Ikeda, T. and Onodera, M. and Muramatsu, A. and Mizukami, F. and Wang, Y. and Gies, H.
MICROPOROUS AND MESOPOROUS MATERIALS. Volume: 128 (2010) - 2010 • 557
Cyclic loading behavior of micro-sized polycrystalline copper wires
Yang, B. and Motz, C. and Grosinger, W. and Dehm, G.
PROCEDIA ENGINEERING. Volume: 2 (2010) - 2010 • 556
Paramagnetic spin correlations in CaFe2As2 single crystals
Diallo, S.O. and Pratt, D.K. and Fernandes, R.M. and Tian, W. and Zarestky, J.L. and Lumsden, M. and Perring, T.G. and Broholm, C.L. and Ni, N. and Bud'Ko, S.L. and Canfield, P.C. and Li, H.-F. and Vaknin, D. and Kreyssig, A. and Goldman, A.I. and McQueeney, R.J.
PHYSICAL REVIEW B - CONDENSED MATTER AND MATERIALS PHYSICS. Volume: 81 (2010) - 2010 • 555
Ab initio tensile tests of Al bulk crystals and grain boundaries: Universality of mechanical behavior
Janisch, R. and Ahmed, N. and Hartmaier, A.
PHYSICAL REVIEW B - CONDENSED MATTER AND MATERIALS PHYSICS. Volume: 81 (2010) - 2010 • 554
Overview of porous materials
Schüth, F.
CHEMIE-INGENIEUR-TECHNIK. Volume: 82 (2010) - 2010 • 553
Effects of Ti, Zr, and Hf on the phase stability of Mo_ss + Mo3Si + Mo5SiB2 alloys at 1600 °C
Yang, Y. and Bei, H. and Chen, S. and George, E.P. and Tiley, J. and Chang, Y.A.
ACTA MATERIALIA. Volume: 58 (2010) - 2010 • 552
Kein Ausbau erneuerbarer Energien ohne chemische Technik
Schüth, F. and Wagemann, K.
CHEMIE-INGENIEUR-TECHNIK. Volume: 82 (2010) - 2010 • 551
One year for energy
Schüth, F.
NACHRICHTEN AUS DER CHEMIE. Volume: 58 (2010) - 2010 • 550
Atmospheric plasma spraying of high melting temperature complex perovskites for TBC application
Jarligo, M.O. and MacK, D.E. and Mauer, G. and Vaßen, R. and Stöver, D.
JOURNAL OF THERMAL SPRAY TECHNOLOGY. Volume: 19 (2010) - 2010 • 549
Shape-dependent catalytic properties of Pt nanoparticles
Mostafa, S. and Behafarid, F. and Croy, J.R. and Ono, L.K. and Li, L. and Yang, J.C. and Frenkel, A.I. and Cuenya, B.R.
JOURNAL OF THE AMERICAN CHEMICAL SOCIETY. Volume: 132 (2010) - 2010 • 548
Paving the way to new energy systems: The key role of the chemical sciences
Schüth, F.
CHEMSUSCHEM. Volume: 3 (2010) - 2010 • 547
Investigation of the internal substructure of microbands in a deformed copper single crystal: Experiments and dislocation dynamics simulation
Dmitrieva, O. and Svirina, J.V. and Demir, E. and Raabe, D.
MODELLING AND SIMULATION IN MATERIALS SCIENCE AND ENGINEERING. Volume: 18 (2010) - 2010 • 546
Diffusion of Li in olivine. Part I: Experimental observations and a multi species diffusion model
Dohmen, R. and Kasemann, S.A. and Coogan, L. and Chakraborty, S.
GEOCHIMICA ET COSMOCHIMICA ACTA. Volume: 74 (2010) - 2010 • 545
Atomistic simulations of lattice defects in tungsten
Mrovec, M. and Elsässer, C. and Gumbsch, P.
INTERNATIONAL JOURNAL OF REFRACTORY METALS AND HARD MATERIALS. Volume: 28 (2010) - 2010 • 544
Phase volume fractions and strain measurements in an ultrafine-grained NiTi shape-memory alloy during tensile loading
Young, M.L. and Wagner, M.F.-X. and Frenzel, J. and Schmahl, W.W. and Eggeler, G.
ACTA MATERIALIA. Volume: 58 (2010) - 2010 • 543
Step-by-step growth of highly oriented and continuous seeding layers of [Cu2(ndc)2(dabco)] on bare oxide and nitride substrates
Yusenko, K. and Meilikhov, M. and Zacher, D. and Wieland, F. and Sternemann, C. and Stammer, X. and Ladnorg, T. and Wöll, C. and Fischer, R.A.
CRYSTENGCOMM. Volume: 12 (2010) - 2010 • 542
Measurement based channel model for large concert halls
Dortmund, S. and Schmidt, A. and Rolfes, I.
2010 IEEE INTERNATIONAL SYMPOSIUM ON ANTENNAS AND PROPAGATION AND CNC-USNC/URSI RADIO SCIENCE MEETING - LEADING THE WAVE, AP-S/URSI 2010. Volume: (2010) - 2010 • 541
An ultrafine nanoporous bimetallic Ag-Pd alloy with superior catalytic activity
Ji, H. and Frenzel, J. and Qi, Z. and Wang, X. and Zhao, C. and Zhang, Z. and Eggeler, G.
CRYSTENGCOMM. Volume: 12 (2010) - 2010 • 540
Towards a high potential biocathode based on direct bioelectrochemistry between horseradish peroxidase and hierarchically structured carbon nanotubes
Jia, W. and Schwamborn, S. and Jin, C. and Xia, W. and Muhler, M. and Schuhmann, W. and Stoica, L.
PHYSICAL CHEMISTRY CHEMICAL PHYSICS. Volume: 12 (2010) - 2010 • 539
Small gold particles supported on MgFe2O4 nanocrystals as novel catalyst for CO oxidation
Jia, C.-J. and Liu, Y. and Schwickardi, M. and Weidenthaler, C. and Spliethoff, B. and Schmidt, W. and Schüth, F.
APPLIED CATALYSIS A: GENERAL. Volume: 386 (2010) - 2010 • 538
Scanning electrochemical microscopy in neuroscience
Schulte, A. and Nebel, M. and Schuhmann, W.
ANNUAL REVIEW OF ANALYTICAL CHEMISTRY. Volume: 3 (2010) - 2010 • 537
Crystal plasticity modelling and experiments for deriving microstructure-property relationships in γ-TiAl based alloys
Zambaldi, C. and Raabe, D.
JOURNAL OF PHYSICS: CONFERENCE SERIES. Volume: 240 (2010) - 2010 • 536
In-plane gate transistors implanted with different channel geometries by focussed ion beam in positive mode pattern definition technique
Draghici, M. and Diaconescu, D. and Melnikov, A. and Wieck, A.D.
PHYSICA STATUS SOLIDI (A) APPLICATIONS AND MATERIALS SCIENCE. Volume: 207 (2010) - 2010 • 535
Small-sized HZSM-5 zeolite as highly active catalyst for gas phase dehydration of glycerol to acrolein
Jia, C.-J. and Liu, Y. and Schmidt, W. and Lu, A.-H. and Schüth, F.
JOURNAL OF CATALYSIS. Volume: 269 (2010) - 2010 • 534
Microstructure and mechanical properties of laser-welded joints of TWIP and TRIP steels
Mujica, L. and Weber, S. and Pinto, H. and Thomy, C. and Vollertsen, F.
MATERIALS SCIENCE AND ENGINEERING A. Volume: 527 (2010) - 2010 • 533
The multipole resonance probe: Realization of an optimized radio-frequency plasma probe based on active plasma resonance spectroscopy
Schulz, C. and Lapke, M. and Oberrath, J. and Storch, R. and Styrnoll, T. and Zietz, C. and Awakowicz, P. and Brinkmann, R.P. and Musch, T. and Mussenbrock, T. and Rolfes, I.
MECAP'10, 1ST MIDDLE EAST CONFERENCE ON ANTENNAS AND PROPAGATION. Volume: (2010) - 2010 • 532
Plastic anisotropy of γ-TiAl revealed by axisymmetric indentation
Zambaldi, C. and Raabe, D.
ACTA MATERIALIA. Volume: 58 (2010) - 2010 • 531
Thermal-gradient testing of thermal barrier coatings under simultaneous attack by molten glassy deposits and its mitigation
Drexler, J.M. and Aygun, A. and Li, D. and Vaßen, R. and Steinke, T. and Padture, N.P.
SURFACE AND COATINGS TECHNOLOGY. Volume: 204 (2010) - 2010 • 530
Very low temperature CO oxidation over colloidally deposited gold nanoparticles on Mg(OH)2 and MgO
Jia, C.-N. and Liu, Y. and Bongard, H. and Schüth, F.
JOURNAL OF THE AMERICAN CHEMICAL SOCIETY. Volume: 132 (2010) - 2010 • 529
Tuning low-temperature physical properties of CeNiGe3 by magnetic field
Mun, E.D. and Bud'Ko, S.L. and Kreyssig, A. and Canfield, P.C.
PHYSICAL REVIEW B - CONDENSED MATTER AND MATERIALS PHYSICS. Volume: 82 (2010) - 2010 • 528
Identification of quaternary shape memory alloys with near-zero thermal hysteresis and unprecedented functional stability
Zarnetta, R. and Takahashi, R. and Young, M.L. and Savan, A. and Furuya, Y. and Thienhaus, S. and Maaß, B. and Rahim, M. and Frenzel, J. and Brunken, H. and Chu, Y.S. and Srivastava, V. and James, R.D. and Takeuchi, I. and Eggeler, G. and Ludwig, Al.
ADVANCED FUNCTIONAL MATERIALS. Volume: 20 (2010) - 2010 • 527
Design of a bioelectrocatalytic electrode interface for oxygen reduction in biofuel cells based on a specifically adapted Os-complex containing redox polymer with entrapped Trametes hirsuta laccase
Ackermann, Y. and Guschin, D.A. and Eckhard, K. and Shleev, S. and Schuhmann, W.
ELECTROCHEMISTRY COMMUNICATIONS. Volume: 12 (2010) - 2010 • 526
Identification of optimized Ti-Ni-Cu shape memory alloy compositions for high-frequency thin film microactuator applications
Zarnetta, R. and Ehmann, M. and Savan, A. and Ludwig, Al.
SMART MATERIALS AND STRUCTURES. Volume: 19 (2010) - 2010 • 525
A two-dimensional dislocation dynamics model of the plastic deformation of polycrystalline metals
Ahmed, N. and Hartmaier, A.
JOURNAL OF THE MECHANICS AND PHYSICS OF SOLIDS. Volume: 58 (2010) - 2010 • 524
Carbon nanotube-supported sulfided Rh catalysts for the oxygen reduction reaction
Jin, C. and Xia, W. and Guo, J. and Nagaiah, T.C. and Bron, M. and Schuhmann, W. and Muhler, M.
STUDIES IN SURFACE SCIENCE AND CATALYSIS. Volume: 175 (2010) - 2010 • 523
Investigation of strain-induced martensitic transformation in metastable austenite using nanoindentation
Ahn, T.-H. and Oh, C.-S. and Kim, D.H. and Oh, K.H. and Bei, H. and George, E.P. and Han, H.N.
SCRIPTA MATERIALIA. Volume: 63 (2010) - 2010 • 522
Metal-free and electrocatalytically active nitrogen-doped carbon nanotubes synthesized by coating with polyaniline
Jin, C. and Nagaiah, T.C. and Xia, W. and Spliethoff, B. and Wang, S. and Bron, M. and Schuhmann, W. and Muhler, M.
NANOSCALE. Volume: 2 (2010) - 2010 • 521
On the action of magnetic gradient forces in micro-structured copper deposition
Mutschke, G. and Tschulik, K. and Weier, T. and Uhlemann, M. and Bund, A. and Fröhlich, J.
ELECTROCHIMICA ACTA. Volume: 55 (2010) - 2010 • 520
Quasiparticle interference in iron-based superconductors
Akbari, A. and Knolle, J. and Eremin, I. and Moessner, R.
PHYSICAL REVIEW B - CONDENSED MATTER AND MATERIALS PHYSICS. Volume: 82 (2010) - 2010 • 519
On the characterization of recrystallized fraction using electron backscatter diffraction: A direct comparison to local hardness in an IF steel using nanoindentation
Dziaszyk, S. and Payton, E.J. and Friedel, F. and Marx, V. and Eggeler, G.
MATERIALS SCIENCE AND ENGINEERING A. Volume: 527 (2010) - 2010 • 518
Rh-RhSx nanoparticles grafted on functionalized carbon nanotubes as catalyst for the oxygen reduction reaction
Jin, C. and Xia, W. and Nagaiah, T.C. and Guo, J. and Chen, X. and Li, N. and Bron, M. and Schuhmann, W. and Muhler, M.
JOURNAL OF MATERIALS CHEMISTRY. Volume: 20 (2010) - 2010 • 517
Magnetic impurity resonance states and symmetry of the superconducting order parameter in iron-based superconductors
Akbari, A. and Eremin, I. and Thalmeier, P.
PHYSICAL REVIEW B - CONDENSED MATTER AND MATERIALS PHYSICS. Volume: 81 (2010) - 2010 • 516
Modeling size effects on fracture toughness by dislocation dynamics
Zeng, X.H. and Hartmaier, A.
ACTA MATERIALIA. Volume: 58 (2010) - 2010 • 515
Epitaxial growth and interfacial magnetism of spin aligner for remanent spin injection: [Fe/Tb]n /Fe/MgO/GaAs -light emitting diode as a prototype system
Schuster, E. and Brand, R.A. and Stromberg, F. and Lo, F.-Y. and Ludwig, Ar. and Reuter, D. and Wieck, A.D. and Hövel, S. and Gerhardt, N.C. and Hofmann, M.R. and Wende, H. and Keune, W.
JOURNAL OF APPLIED PHYSICS. Volume: 108 (2010) - 2010 • 514
Unveiling the atomic and electronic structure of the VN/MgO interface
Zhang, Z. and Rashkova, B. and Dehm, G. and Lazar, P. and Redinger, J. and Podloucky, R.
PHYSICAL REVIEW B - CONDENSED MATTER AND MATERIALS PHYSICS. Volume: 82 (2010) - 2010 • 513
Numerical investigation of room-temperature deformation behavior of a duplex type γtiAl alloy using a multi-scale modeling approach
Kabir, M.R. and Chernova, L. and Bartsch, M.
ACTA MATERIALIA. Volume: 58 (2010) - 2010 • 512
Nitrogen-doped carbon nanotubes as a cathode catalyst for the oxygen reduction reaction in alkaline medium
Nagaiah, T.C. and Kundu, S. and Bron, M. and Muhler, M. and Schuhmann, W.
ELECTROCHEMISTRY COMMUNICATIONS. Volume: 12 (2010) - 2010 • 511
Probing the reactivity of ZnO and Au/ZnO nanoparticles by methanol adsorption: A TPD and DRIFTS study
Kähler, K. and Holz, M.C. and Rohe, M. and Strunk, J. and Muhler, M.
CHEMPHYSCHEM. Volume: 11 (2010) - 2010 • 510
Patterned CNT arrays for the evaluation of oxygen reduction activity by SECM
Schwamborn, S. and Stoica, L. and Chen, X. and Xia, W. and Kundu, S. and Muhler, M. and Schuhmann, W.
CHEMPHYSCHEM. Volume: 11 (2010) - 2010 • 509
An elasto-plastic three phase model for partially saturated soil for the finite element simulation of compressed air support in tunnelling
Nagel, F. and Meschke, G.
INTERNATIONAL JOURNAL FOR NUMERICAL AND ANALYTICAL METHODS IN GEOMECHANICS. Volume: 34 (2010) - 2010 • 508
Ancient technology/novel nanomaterials: Casting titanium carbide nanowires
Zhang, Z. and Wang, Y. and Frenzel, J.
CRYSTENGCOMM. Volume: 12 (2010) - 2010 • 507
Load modulation for efficiency enhancement of inverse class-D power amplifiers
El Din, M.G. and Geek, B. and Rolfes, I. and Eul, H.
MECAP'10, 1ST MIDDLE EAST CONFERENCE ON ANTENNAS AND PROPAGATION. Volume: (2010) - 2010 • 506
Depositions of SrRuO3 thin films on oxide substrates with liquid-delivery spin MOCVD
Schwarzkopf, J. and Dirsyte, R. and Devi, A. and Schmidbauer, M. and Wagner, G. and Fornari, R.
THIN SOLID FILMS. Volume: 518 (2010) - 2010 • 505
A novel continuous approach for the synthesis and characterization of pure and mixed metal oxide systems applied in heterogeneous catalysis
Kaluza, S. and Muhler, M.
STUDIES IN SURFACE SCIENCE AND CATALYSIS. Volume: 175 (2010) - 2010 • 504
Effect of fiber angle orientation and stacking sequence on mixed mode fracture toughness of carbon fiber reinforced plastics: Numerical and experimental investigations
Naghipour, P. and Bartsch, M. and Chernova, L. and Hausmann, J. and Voggenreiter, H.
MATERIALS SCIENCE AND ENGINEERING A. Volume: 527 (2010) - 2010 • 503
Atomic mobilities and diffusivities in the fcc, L12 and B2 phases of the Ni-Al system
Zhang, L. and Du, Y. and Chen, Q. and Steinbach, I. and Huang, B.
INTERNATIONAL JOURNAL OF MATERIALS RESEARCH. Volume: 101 (2010) - 2010 • 502
Multiscale simulations on the grain growth process in nanostructured materials
Kamachali, R.D. and Hua, J. and Steinbach, I. and Hartmaier, A.
INTERNATIONAL JOURNAL OF MATERIALS RESEARCH. Volume: 101 (2010) - 2010 • 501
Anomalous suppression of the orthorhombic lattice distortion in superconducting Ba(Fe1-xCox)2As2 single crystals
Nandi, S. and Kim, M.G. and Kreyssig, A. and Fernandes, R.M. and Pratt, D.K. and Thaler, A. and Ni, N. and Bud'Ko, S.L. and Canfield, P.C. and Schmalian, J. and McQueeney, R.J. and Goldman, A.I.
PHYSICAL REVIEW LETTERS. Volume: 104 (2010) - 2010 • 500
Diffusivities of an Al-Fe-Ni melt and their effects on the microstructure during solidification
Zhang, L. and Du, Y. and Steinbach, I. and Chen, Q. and Huang, B.
ACTA MATERIALIA. Volume: 58 (2010) - 2010 • 499
Characterization of the effluent of a He/O-2 microscale atmospheric pressure plasma jet by quantitative molecular beam mass spectrometry
Ellerweg, D. and Benedikt, J. and von Keudell, A. and Knake, N. and Schulz-von der Gathen, V.
NEW JOURNAL OF PHYSICS. Volume: 12 (2010) - 2010 • 498
First-principles study of the thermodynamics of hydrogen-vacancy interaction in fcc iron
Nazarov, R. and Hickel, T. and Neugebauer, J.
PHYSICAL REVIEW B - CONDENSED MATTER AND MATERIALS PHYSICS. Volume: 82 (2010) - 2010 • 497
Modeling of hot ductility during solidification of steel grades in continuous casting - Part I
Senk, D. and Stratemeier, S. and Böttger, B. and Göhler, K. and Steinbach, I.
ADVANCED ENGINEERING MATERIALS. Volume: 12 (2010) - 2010 • 496
Structure-Function Correlations for Ru/CNT in the Catalytic Decomposition of Ammonia
Zheng, W. Q. and Zhang, J. and Zhu, B. and Blume, R. and Zhang, Y. L. and Schlichte, K. and Schlogl, R. and Schüth, F. and Su, D. S.
CHEMSUSCHEM. Volume: 3 (2010) - 2010 • 495
Ab initio study of thermodynamic, structural, and elastic properties of Mg-substituted crystalline calcite
Elstnerová, P. and Friák, M. and Fabritius, H.O. and Lymperakis, L. and Hickel, T. and Petrov, M. and Nikolov, S. and Raabe, D. and Ziegler, A. and Hild, S. and Neugebauer, J.
ACTA BIOMATERIALIA. Volume: 6 (2010) - 2010 • 494
Local reactivity of diamond-like carbon modified PTFE membranes used in SO2 sensors
Nebel, M. and Neugebauer, S. and Kiesele, H. and Schuhmann, W.
ELECTROCHIMICA ACTA. Volume: 55 (2010) - 2010 • 493
A new approach to optimal design for linear models with correlated observations
Zhigljavsky, A. and Dette, H. and Pepelyshev, A.
JOURNAL OF THE AMERICAN STATISTICAL ASSOCIATION. Volume: 105 (2010) - 2010 • 492
Substance identification by depth resolved spectroscopic pattern reconstruction in frequency domain optical coherence tomography
Kasseck, C. and Jaedicke, V. and Gerhardt, N.C. and Welp, H. and Hofmann, M.R.
OPTICS COMMUNICATIONS. Volume: 283 (2010) - 2010 • 491
4D shearforce-based constant-distance mode scanning electrochemical microscopy
Nebel, M. and Eckhard, K. and Erichsen, T. and Schulte, A. and Schuhmann, W.
ANALYTICAL CHEMISTRY. Volume: 82 (2010) - 2010 • 490
Effect of Ni4Ti3 precipitation on martensitic transformation in Ti-Ni
Zhou, N. and Shen, C. and Wagner, M.F.-X. and Eggeler, G. and Mills, M.J. and Wang, Y.
ACTA MATERIALIA. Volume: 58 (2010) - 2010 • 489
Incommensurate itinerant antiferromagnetic excitations and spin resonance in the FeTe0.6Se0.4 superconductor
Argyriou, D.N. and Hiess, A. and Akbari, A. and Eremin, I. and Korshunov, M.M. and Hu, J. and Qian, B. and Mao, Z. and Qiu, Y. and Broholm, C. and Bao, W.
PHYSICAL REVIEW B - CONDENSED MATTER AND MATERIALS PHYSICS. Volume: 81 (2010) - 2010 • 488
Comparison of optical coherence tomography, microcomputed tomography, and histology at a three-dimensionally imaged trabecular bone sample
Kasseck, C. and Kratz, M. and Torcasio, A. and Gerhardt, N.C. and Van Lenthe, G.H. and Gambichler, T. and Hoffmann, K. and Jones, D.B. and Hofmann, M.R.
JOURNAL OF BIOMEDICAL OPTICS. Volume: 15 (2010) - 2010 • 487
An in situ tensile tester for studying electrochemical repassivation behavior: Fabrication and challenges
Neelakantan, L. and Schönberger, B. and Eggeler, G. and Hassel, A.W.
REVIEW OF SCIENTIFIC INSTRUMENTS. Volume: 81 (2010) - 2010 • 486
Frequency domain optical coherence tomography with subsequent depth resolved spectroscopic image analysis
Kasseck, C. and Jaedicke, V. and Gerhardt, N.C. and Welp, H. and Hofmann, M.R.
PROGRESS IN BIOMEDICAL OPTICS AND IMAGING - PROCEEDINGS OF SPIE. Volume: 7554 (2010) - 2010 • 485
Surface chemistry and topographical changes of an electropolished NiTi shape memory alloy
Neelakantan, L. and Valtiner, M. and Eggeler, G. and Hassel, A. W.
PHYSICA STATUS SOLIDI A-APPLICATIONS AND MATERIALS SCIENCE. Volume: 207 (2010) - 2010 • 484
Influence of crystal anisotropy on elastic deformation and onset of plasticity in nanoindentation: A simulational study
Ziegenhain, G. and Urbassek, H.M. and Hartmaier, A.
JOURNAL OF APPLIED PHYSICS. Volume: 107 (2010) - 2010 • 483
Molecular dynamics simulations of the shape memory effect in a chain of Lennard-Jones crystals
Kastner, O. and Eggeler, G.
MULTIDISCIPLINE MODELING IN MATERIALS AND STRUCTURES. Volume: 6 (2010) - 2010 • 482
The influence of the relative phase between the driving voltages on electron heating in asymmetric dual frequency capacitive discharges
Ziegler, D. and Trieschmann, J. and Mussenbrock, T. and Brinkmann, R.P. and Schulze, J. and Czarnetzki, U. and Semmler, E. and Awakowicz, P. and O'Connell, D. and Gans, T.
PLASMA SOURCES SCIENCE AND TECHNOLOGY. Volume: 19 (2010) - 2010 • 481
Thin films of the Heusler alloys Cu2MnAl and Co2MnSi: Recovery of ferromagnetism via solid-state crystallization from the x-ray amorphous state
Erb, D. and Nowak, G. and Westerholt, K. and Zabel, H.
JOURNAL OF PHYSICS D: APPLIED PHYSICS. Volume: 43 (2010) - 2010 • 480
Magnetic degeneracy and hidden metallicity of the spin-density-wave state in ferropnictides
Eremin, I. and Chubukov, A.V.
PHYSICAL REVIEW B - CONDENSED MATTER AND MATERIALS PHYSICS. Volume: 81 (2010) - 2010 • 479
A Physicist's Perspective on "Views on Macroscopic Kinetics of Plasma Polymerisation"
von Keudell, A. and Benedikt, J.
PLASMA PROCESSES AND POLYMERS. Volume: 7 (2010) - 2010 • 478
Raman spectroscopy on single levitated particles
Esen, C. and Šurbek, M. and Baer, S. and Ostendorf, A.
CHEMIE-INGENIEUR-TECHNIK. Volume: 82 (2010) - 2010 • 477
Graphite-diamond phase coexistence study employing a neural-network mapping of the ab initio potential energy surface
Khaliullin, R.Z. and Eshet, H. and Kühne, T.D. and Behler, J. and Parrinello, M.
PHYSICAL REVIEW B - CONDENSED MATTER AND MATERIALS PHYSICS. Volume: 81 (2010) - 2010 • 476
Ab initio quality neural-network potential for sodium
Eshet, H. and Khaliullin, R.Z. and Kühne, T.D. and Behler, J. and Parrinello, M.
PHYSICAL REVIEW B - CONDENSED MATTER AND MATERIALS PHYSICS. Volume: 81 (2010) - 2010 • 475
Effects of focused ion beam milling and pre-straining on the microstructure of directionally solidified molybdenum pillars: A Laue diffraction analysis
Zimmermann, J. and Van Petegem, S. and Bei, H. and Grolimund, D. and George, E.P. and Van Swygenhoven, H.
SCRIPTA MATERIALIA. Volume: 62 (2010) - 2010 • 474
Pair breaking by nonmagnetic impurities in the noncentrosymmetric superconductor CePt3 Si
Nicklas, M. and Steglich, F. and Knolle, J. and Eremin, I. and Lackner, R. and Bauer, E.
PHYSICAL REVIEW B - CONDENSED MATTER AND MATERIALS PHYSICS. Volume: 81 (2010) - 2010 • 473
Effects of annealing on the microstructure and the mechanical properties of EB-PVD thermal barrier coatings
Zotov, N. and Bartsch, M. and Chernova, L. and Schmidt, D.A. and Havenith, M. and Eggeler, G.
SURFACE AND COATINGS TECHNOLOGY. Volume: 205 (2010) - 2010 • 472
Effects of annealing time on the structural and magnetic properties of L10 FePt thin films
Zotov, N. and Hiergeist, R. and Savan, A. and Ludwig, Al.
THIN SOLID FILMS. Volume: 518 (2010) - 2010 • 471
A novel method to measure diffusion coefficients in porous metal-organic frameworks
Zybaylo, O. and Shekhah, O. and Wang, H. and Tafipolsky, M. and Schmid, R. and Johannsmann, D. and Wöll, C.
PHYSICAL CHEMISTRY CHEMICAL PHYSICS. Volume: 12 (2010) - 2010 • 470
Acoustic driven flow and lattice Boltzmann simulations to study cell adhesion in biofunctionalized μ-fluidic channels with complex geometry
Fallah, M.A. and Myles, V.M. and Krüger, T. and Sritharan, K. and Wixforth, A. and Varnik, F. and Schneider, S.W. and Schneider, M.F.
BIOMICROFLUIDICS. Volume: 4 (2010) - 2010 • 469
Antiferromagnetic ordering in the absence of structural distortion in Ba (Fe1-x Mnx )2 As2
Kim, M.G. and Kreyssig, A. and Thaler, A. and Pratt, D.K. and Tian, W. and Zarestky, J.L. and Green, M.A. and Bud'Ko, S.L. and Canfield, P.C. and McQueeney, R.J. and Goldman, A.I.
PHYSICAL REVIEW B - CONDENSED MATTER AND MATERIALS PHYSICS. Volume: 82 (2010) - 2010 • 468
On the multiplication of dislocations during martensitic transformations in NiTi shape memory alloys
Simon, T. and Kröger, A. and Somsen, C. and Dlouhy, A. and Eggeler, G.
ACTA MATERIALIA. Volume: 58 (2010) - 2010 • 467
Commensurate antiferromagnetic ordering in Ba(Fe1-x Co x )2As2 determined by x-ray resonant magnetic scattering at the Fe K edge
Kim, M.G. and Kreyssig, A. and Lee, Y.B. and Kim, J.W. and Pratt, D.K. and Thaler, A. and Bud'Ko, S.L. and Canfield, P.C. and Harmon, B.N. and McQueeney, R.J. and Goldman, A.I.
PHYSICAL REVIEW B - CONDENSED MATTER AND MATERIALS PHYSICS. Volume: 82 (2010) - 2010 • 466
Quantum coherence at low temperatures in mesoscopic systems: Effect of disorder
Niimi, Y. and Baines, Y. and Capron, T. and Mailly, D. and Lo, F.-Y. and Wieck, A.D. and Meunier, T. and Saminadayar, L. and Bäuerle, C.
PHYSICAL REVIEW B - CONDENSED MATTER AND MATERIALS PHYSICS. Volume: 81 (2010) - 2010 • 465
Fundamental aspects of substrate biasing: ion velocity distributions and nonlinear effects
Baloniak, T. and Reuter, R. and von Keudell, A.
JOURNAL OF PHYSICS D-APPLIED PHYSICS. Volume: 43 (2010) - 2010 • 464
Revealing the design principles of high-performance biological composites using Ab initio and multiscale simulations: The example of lobster cuticle
Nikolov, S. and Petrov, M. and Lymperakis, L. and Friák, M. and Sachs, C. and Fabritius, H.-O. and Raabe, D. and Neugebauer, J.
ADVANCED MATERIALS. Volume: 22 (2010) - 2010 • 463
Electromagnetically induced transparency with an ensemble of donor-bound electron spins in a semiconductor
Sladkov, M. and Chaubal, A.U. and Bakker, M.P. and Onur, A.R. and Reuter, D. and Wieck, A.D. and Van Der Wal, C.H.
PHYSICAL REVIEW B - CONDENSED MATTER AND MATERIALS PHYSICS. Volume: 82 (2010) - 2010 • 462
Calibration of a miniaturized retarding field analyzer for low-temperature plasmas: geometrical transparency and collisional effects
Baloniak, T. and Reuter, R. and Flotgen, C. and von Keudell, A.
JOURNAL OF PHYSICS D-APPLIED PHYSICS. Volume: 43 (2010) - 2010 • 461
Unconventional pairing in the iron arsenide superconductors
Fernandes, R.M. and Pratt, D.K. and Tian, W. and Zarestky, J. and Kreyssig, A. and Nandi, S. and Kim, M.G. and Thaler, A. and Ni, N. and Canfield, P.C. and McQueeney, R.J. and Schmalian, J. and Goldman, A.I.
PHYSICAL REVIEW B - CONDENSED MATTER AND MATERIALS PHYSICS. Volume: 81 (2010) - 2010 • 460
Interfacial Cu/ZnO contact by selective photodeposition of copper onto the surface of small ZnO nanoparticles in non-aqueous colloidal solution
Sliem, M.A. and Hikov, T. and Li, Z.-A. and Spasova, M. and Farle, M. and Schmidt, D.A. and Havenith-Newen, M. and Fischer, R.A.
PHYSICAL CHEMISTRY CHEMICAL PHYSICS. Volume: 12 (2010) - 2010 • 459
FE 2-simulation of microheterogeneous steels based on statistically similar RVEs
Balzani, D. and Schröder, J. and Brands, D.
IUTAM BOOKSERIES. Volume: 21 (2010) - 2010 • 458
Hydrogen Loading of Oxide Powder Particles: A Transmission IR Study for the Case of Zinc Oxide
Noei, H. and Qiu, H. and Wang, Y. and Muhler, M. and Wöll, C.
CHEMPHYSCHEM. Volume: 11 (2010) - 2010 • 457
On the mechanical modeling of anisotropic biological soft tissue and iterative parallel solution strategies
Balzani, D. and Brands, D. and Klawonn, A. and Rheinbach, O. and Schröder, J.
ARCHIVE OF APPLIED MECHANICS. Volume: 80 (2010) - 2010 • 456
Regioselectively controlled synthesis of colloidal mushroom nanostructures and their hollow derivatives
Feyen, M. and Weidenthaler, C. and Schüth, F. and Lu, A.-H.
JOURNAL OF THE AMERICAN CHEMICAL SOCIETY. Volume: 132 (2010) - 2010 • 455
Synthesis of structurally stable colloidal composites as magnetically recyclable acid catalysts
Feyen, M. and Weidenthaler, C. and Schüth, F. and Lu, A.-H.
CHEMISTRY OF MATERIALS. Volume: 22 (2010) - 2010 • 454
A microelectrochemical sensing system for the determination of Epstein-Barr virus antibodies
Bandilla, M. and Zimdars, A. and Neugebauer, S. and Motz, M. and Schuhmann, W. and Hartwich, G.
ANALYTICAL AND BIOANALYTICAL CHEMISTRY. Volume: 398 (2010) - 2010 • 453
3D x-ray microprobe investigation of local dislocation densities and elastic strain gradients in a NiAl-Mo composite and exposed Mo micropillars as a function of prestrain
Barabash, R.I. and Bei, H. and Gao, Y. and Ice, G.E. and George, E.P.
JOURNAL OF MATERIALS RESEARCH. Volume: 25 (2010) - 2010 • 452
Can local hot spots induce α2/γ lamellae during incomplete massive transformation of γ-TiAl alloys?
Fischer, F.D. and Cha, L. and Dehm, G. and Clemens, H.
INTERMETALLICS. Volume: 18 (2010) - 2010 • 451
Study of nanometer-scaled lamellar microstructure in a Ti-45Al-7.5Nb alloy - Experiments and modeling
Fischer, F.D. and Waitz, T. and Scheu, Ch. and Cha, L. and Dehm, G. and Antretter, T. and Clemens, H.
INTERMETALLICS. Volume: 18 (2010) - 2010 • 450
Biocompatibility of nanoactuators: Stem cell growth on laser-generated nickel-titanium shape memory alloy nanoparticles
Barcikowski, S. and Hahn, A. and Guggenheim, M. and Reimers, K. and Ostendorf, A.
JOURNAL OF NANOPARTICLE RESEARCH. Volume: 12 (2010) - 2010 • 449
Temperature sensing by using whispering gallery modes with hollow core fibers
Özel, B. and Nett, R. and Weigel, T. and Schweiger, G. and Ostendorf, A.
MEASUREMENT SCIENCE AND TECHNOLOGY. Volume: 21 (2010) - 2010 • 448
Novel insight into the alignment and structural ordering of supported ZnO nanorods
Barreca, D. and Bekermann, D. and Devi, A. and Fischer, R.A. and Gasparotto, A. and MacCato, C. and Tondello, E. and Rossi, M. and Orlanducci, S. and Terranova, M.L.
CHEMICAL PHYSICS LETTERS. Volume: 500 (2010) - 2010 • 447
Variational concepts with applications to microstructural evolution
Fischer, F.D. and Svoboda, J. and Hackl, K.
IUTAM BOOKSERIES. Volume: 21 (2010) - 2010 • 446
Urchin-like ZnO nanorod arrays for gas sensing applications
Barreca, D. and Bekermann, D. and Comini, E. and Devi, A. and Fischer, R.A. and Gasparotto, A. and MacCato, C. and Sada, C. and Sberveglieri, G. and Tondello, E.
CRYSTENGCOMM. Volume: 12 (2010) - 2010 • 445
1D ZnO nano-assemblies by Plasma-CVD as chemical sensors for flammable and toxic gases
Barreca, D. and Bekermann, D. and Comini, E. and Devi, A. and Fischer, R.A. and Gasparotto, A. and MacCato, C. and Sberveglieri, G. and Tondello, E.
SENSORS AND ACTUATORS, B: CHEMICAL. Volume: 149 (2010) - 2010 • 444
Optically detected magnetic resonance at the quadrupole-split nuclear states in (In,Ga)As/GaAs quantum dots
Flisinski, K. and Gerlovin, I.Ya. and Ignatiev, I.V. and Petrov, M.Yu. and Verbin, S.Yu. and Yakovlev, D.R. and Reuter, D. and Wieck, A.D. and Bayer, M.
PHYSICAL REVIEW B - CONDENSED MATTER AND MATERIALS PHYSICS. Volume: 82 (2010) - 2010 • 443
Formation and thermal stability of platinum oxides on size-selected platinum nanoparticles: Support effects
Ono, L.K. and Yuan, B. and Heinrich, H. and Roldan Cuenya, B.
JOURNAL OF PHYSICAL CHEMISTRY C. Volume: 114 (2010) - 2010 • 442
Epitaxially stabilized TiN/(Ti,Fe,Co)N multilayer thin films in (pseudo-)fcc crystal structure by sequential magnetron sputter deposition
Klever, C. and Seemann, K. and Stüber, M. and Ulrich, S. and Brunken, H. and Ludwig, Al. and Leiste, H.
JOURNAL OF PHYSICS D: APPLIED PHYSICS. Volume: 43 (2010) - 2010 • 441
Evaluation of factors influencing deep cryogenic treatment that affect the properties of tool steels
Oppenkowski, A. and Weber, S. and Theisen, W.
JOURNAL OF MATERIALS PROCESSING TECHNOLOGY. Volume: 210 (2010) - 2010 • 440
Spin dynamics of electrons and holes in InGaAs/GaAs quantum wells at millikelvin temperatures
Fokina, L.V. and Yugova, I.A. and Yakovlev, D.R. and Glazov, M.M. and Akimov, I.A. and Greilich, A. and Reuter, D. and Wieck, A.D. and Bayer, M.
PHYSICAL REVIEW B - CONDENSED MATTER AND MATERIALS PHYSICS. Volume: 81 (2010) - 2010 • 439
A cubic ordered, mesoporous carbide-derived carbon for gas and energy storage applications
Oschatz, M. and Kockrick, E. and Rose, M. and Borchardt, L. and Klein, N. and Senkovska, I. and Freudenberg, T. and Korenblit, Y. and Yushin, G. and Kaskel, S.
CARBON. Volume: 48 (2010) - 2010 • 438
Thermal expansion of RFeAsO (R=La,Ce,Pr,Sm,Gd)
Klingeler, R. and Wang, L. and Köhler, U. and Behr, G. and Hess, C. and Büchner, B.
JOURNAL OF PHYSICS: CONFERENCE SERIES. Volume: 200 (2010) - 2010 • 437
Artificial single variant martensite in freestanding Fe70Pd 30 films obtained by coherent epitaxial growth
Bechtold, C. and Buschbeck, J. and Lotnyk, A. and Erkartal, B. and Hamann, S. and Zamponi, C. and Schultz, L. and Ludwig, Al. and Kienle, L. and Fähler, S. and Quandt, E.
ADVANCED MATERIALS. Volume: 22 (2010) - 2010 • 436
Micro- and nano-parts generated by laser-based solid freeform fabrication
Ostendorf, A. and Neumeister, A. and Dudziak, S. and Passinger, S. and Stampfl, J.
ADVANCES IN LASER MATERIALS PROCESSING: TECHNOLOGY, RESEARCH AND APPLICATION. Volume: (2010) - 2010 • 435
Three types of bulk impurity induced interference patterns on the (100) and (111) faces of Ne- and Ar-doped silver
Sprodowski, C. and Morgenstern, K.
PHYSICAL REVIEW B - CONDENSED MATTER AND MATERIALS PHYSICS. Volume: 82 (2010) - 2010 • 434
An evaluation of CO2 and H2 selective polymeric membranes for CO2 separation in IGCC processes
Franz, J. and Scherer, V.
JOURNAL OF MEMBRANE SCIENCE. Volume: 359 (2010) - 2010 • 433
Dissociation of oxygen on Ag(100) induced by inelastic electron tunneling
Sprodowski, C. and Mehlhorn, M. and Morgenstern, K.
JOURNAL OF PHYSICS CONDENSED MATTER. Volume: 22 (2010) - 2010 • 432
The synthesis of highly loaded Cu/Al2O3 and Cu/Zno/Al2O3 catalysts by the two-step CVD of Cu IIdiethylamino-2-propoxide in a fluidized-bed reactor
Becker, M. and D'Alnoncourt, R.N. and Kähler, K. and Sekulic, J. and Fischer, R.A. and Muhler, M.
CHEMICAL VAPOR DEPOSITION. Volume: 16 (2010) - 2010 • 431
Evidence for triplet superconductivity in Josephson junctions with barriers of the ferromagnetic Heusler alloy Cu2 MnAl
Sprungmann, D. and Westerholt, K. and Zabel, H. and Weides, M. and Kohlstedt, H.
PHYSICAL REVIEW B - CONDENSED MATTER AND MATERIALS PHYSICS. Volume: 82 (2010) - 2010 • 430
Monospectral photoacoustic imaging using Legendre sequences
Beckmann, M.F. and Mienkina, M.P. and Schmitz, G. and Friedrich, C.-S. and Gerhardt, N.C. and Hofmann, M.R.
PROCEEDINGS - IEEE ULTRASONICS SYMPOSIUM. Volume: (2010) - 2010 • 429
The Potential of Microstructural Optimization in Metal/Oxide Catalysts: Higher Intrinsic Activity of Copper by Partial Embedding of Copper Nanoparticles
Behrens, M. and Furche, A. and Kasatkin, I. and Trunschke, A. and Busser, W. and Muhler, M. and Kniep, B. and Fischer, R. and Schlögl, R.
CHEMCATCHEM. Volume: 2 (2010) - 2010 • 428
High-throughput parallel-I/O using sionlib for mesoscopic particle dynamics simulations on massively parallel computers
Freche, J. and Frings, W. and Sutmann, G.
ADVANCES IN PARALLEL COMPUTING. Volume: 19 (2010) - 2010 • 427
Quasiparticle interference in the spin-density wave phase of iron-based superconductors
Knolle, J. and Eremin, I. and Akbari, A. and Moessner, R.
PHYSICAL REVIEW LETTERS. Volume: 104 (2010) - 2010 • 426
Theory of itinerant magnetic excitations in the spin-density-wave phase of iron-based superconductors
Knolle, J. and Eremin, I. and Chubukov, A.V. and Moessner, R.
PHYSICAL REVIEW B - CONDENSED MATTER AND MATERIALS PHYSICS. Volume: 81 (2010) - 2010 • 425
Orientation dependence of local lattice rotations at precipitates: Example of κ-Fe3AlC carbides in a Fe3Al-based alloy
Kobayashi, S. and Zambaldi, C. and Raabe, D.
ACTA MATERIALIA. Volume: 58 (2010) - 2010 • 424
Formation, stability and crystal structure of the r phase in Mo-Re-Si alloys
Bei, H. and Yang, Y. and Viswanathan, G.B. and Rawn, C.J. and George, E.P. and Tiley, J. and Chang, Y.A.
ACTA MATERIALIA. Volume: 58 (2010) - 2010 • 423
Influence of Ni on martensitic phase transformations in NiTi shape memory alloys
Frenzel, J. and George, E.P. and Dlouhy, A. and Somsen, C. and Wagner, M.F.-X. and Eggeler, G.
ACTA MATERIALIA. Volume: 58 (2010) - 2010 • 422
Specimen size effects on Zr-based bulk metallic glasses investigated by uniaxial compression and spherical nanoindentation
Bei, H. and Lu, Z.P. and Shim, S. and Chen, G. and George, E.P.
METALLURGICAL AND MATERIALS TRANSACTIONS A: PHYSICAL METALLURGY AND MATERIALS SCIENCE. Volume: 41 (2010) - 2010 • 421
Ab initio study of the anomalous volume-composition dependence in Fe-Al alloys
Friák, M. and Neugebauer, J.
INTERMETALLICS. Volume: 18 (2010) - 2010 • 420
Time-Continuous Evolution of Microstructures in Finite Plasticity
Kochmann, D. M. and Hackl, K.
IUTAM SYMPOSIUM ON VARIATIONAL CONCEPTS WITH APPLICATIONS TO THE MECHANICS OF MATERIALS. Volume: 21 (2010) - 2010 • 419
Two-dimensional higher order noise spectroscopy up to radio frequencies
Starosielec, S. and Fainblat, R. and Rudolph, J. and Hägele, D.
REVIEW OF SCIENTIFIC INSTRUMENTS. Volume: 81 (2010) - 2010 • 418
Combined ab initio and experimental study of structural and elastic properties of Fe3Al-based ternaries
Friák, M. and Deges, J. and Krein, R. and Frommeyer, G. and Neugebauer, J.
INTERMETALLICS. Volume: 18 (2010) - 2010 • 417
Development and characterization of Fe70Pd30 ferromagnetic shape memory splats
Kock, I. and Hamann, S. and Brunken, H. and Edler, T. and Mayr, S.G. and Ludwig, Al.
INTERMETALLICS. Volume: 18 (2010) - 2010 • 416
ZnO nanorod arrays by plasma-enhanced CVD for light-activated functional applications
Bekermann, D. and Gasparotto, A. and Barreca, D. and Devi, A. and Fischer, R.A. and Kete, M. and Štangar, U.L. and Lebedev, O.I. and Maccato, C. and Tondello, E. and van Tendeloo, G.
CHEMPHYSCHEM. Volume: 11 (2010) - 2010 • 415
Oxidation of 2-propanol by peroxo titanium complexes: A combined experimental and theoretical study
Friese, D.H. and Hättig, C. and Rohe, M. and Merz, K. and Rittermeier, A. and Muhler, M.
JOURNAL OF PHYSICAL CHEMISTRY C. Volume: 114 (2010) - 2010 • 414
Ordered mesoporous carbide derived carbons for high pressure gas storage
Kockrick, E. and Schrage, C. and Borchardt, L. and Klein, N. and Rose, M. and Senkovska, I. and Kaskel, S.
CARBON. Volume: 48 (2010) - 2010 • 413
Highly oriented ZnO nanorod arrays by a novel plasma chemical vapor deposition process
Bekermann, D. and Gasparotto, A. and Barreca, D. and Bovo, L. and Devi, A. and Fischer, R.A. and Lebedev, O.I. and MacCato, C. and Tondello, E. and Van Tendeloo, G.
CRYSTAL GROWTH AND DESIGN. Volume: 10 (2010) - 2010 • 412
Recent Developments and Challenges in Shape Memory Technology
Frotscher, M. and Eggeler, G.
NANOTECHNOLOGICAL BASIS FOR ADVANCED SENSORS. Volume: (2010) - 2010 • 411
Rescaled Monte Carlo approach for magnetic systems: Ab initio thermodynamics of bcc iron
Körmann, F. and Dick, A. and Hickel, T. and Neugebauer, J.
PHYSICAL REVIEW B - CONDENSED MATTER AND MATERIALS PHYSICS. Volume: 81 (2010) - 2010 • 410
Phase-field model with plastic flow for grain growth in nanocrystalline material
Steinbach, I. and Song, X. and Hartmaier, A.
PHILOSOPHICAL MAGAZINE. Volume: 90 (2010) - 2010 • 409
Volatile, monomeric, and fluorine-free precursors for the metal organic chemical vapor deposition of zinc oxide
Bekermann, D. and Rogalla, D. and Becker, H.-W. and Winter, M. and Fischer, R.A. and Devi, A.
EUROPEAN JOURNAL OF INORGANIC CHEMISTRY. Volume: (2010) - 2010 • 408
A novel test approach for plasma-sprayed coatings tested simultaneously under CMAS and thermal gradient cycling conditions
Steinke, T. and Sebold, D. and Mack, D.E. and Vaßen, R. and Stöver, D.
SURFACE AND COATINGS TECHNOLOGY. Volume: 205 (2010) - 2010 • 407
Structural, static and dynamic magnetic properties of Co2MnGe thin films on a sapphire a-plane substrate
Belmeguenai, M. and Zighem, F. and Chauveau, T. and Faurie, D. and Roussigń, Y. and Ch́rif, S.M. and Moch, P. and Westerholt, K. and Monod, P.
JOURNAL OF APPLIED PHYSICS. Volume: 108 (2010) - 2010 • 406
Cross-metathesis as a versatile tool for reversible surface modifications
Palkovits, R. and Arlt, D. and Stepowska, H. and Schüth, F.
MICROPOROUS AND MESOPOROUS MATERIALS. Volume: 132 (2010) - 2010 • 405
Process design and monitoring for plasma sprayed abradable coatings
Steinke, T. and Mauer, G. and Vaßen, R. and Stöver, D. and Roth-Fagaraseanu, D. and Hancock, M.
JOURNAL OF THERMAL SPRAY TECHNOLOGY. Volume: 19 (2010) - 2010 • 404
Development of molecular and solid catalysts for the direct low-temperature oxidation of methane to methanol
Palkovits, R. and von Malotki, C. and Baumgarten, M. and Müllen, K. and Baltes, C. and Antonietti, M. and Kuhn, P. and Weber, J. and Thomas, A. and Schüth, F.
CHEMSUSCHEM. Volume: 3 (2010) - 2010 • 403
Efficient and reliable finite element techniques for phase field models
Stiemer, M. and Grosse-Wohrmann, A. and Gladkov, S. and Svendsen, B. and Spatschek, R. and Steinbach, I.
INTERNATIONAL JOURNAL OF MATERIALS RESEARCH. Volume: 101 (2010) - 2010 • 402
Phase resolved optical emission spectroscopy of coaxial microplasma jet operated with He and Ar
Benedikt, J. and Hofmann, S. and Knake, N. and Bottner, H. and Reuter, R. and von Keudell, A. and Schulz-von der Gathen, V.
EUROPEAN PHYSICAL JOURNAL D. Volume: 60 (2010) - 2010 • 401
New superconducting and semiconducting Fe-B compounds predicted with an Ab initio evolutionary search
Kolmogorov, A.N. and Shah, S. and Margine, E.R. and Bialon, A.F. and Hammerschmidt, T. and Drautz, R.
PHYSICAL REVIEW LETTERS. Volume: 105 (2010) - 2010 • 400
Influence of the microstructure of gold-zirconia yolk-shell catalysts on the CO oxidation activity
Pandey, A.D. and Güttel, R. and Leoni, M. and Schüth, F. and Weidenthaler, C.
JOURNAL OF PHYSICAL CHEMISTRY C. Volume: 114 (2010) - 2010 • 399
Evidence for localization and 0.7 anomaly in hole quantum point contacts
Komijani, Y. and Csontos, M. and Shorubalko, I. and Ihn, T. and Ensslin, K. and Meir, Y. and Reuter, D. and Wieck, A.D.
EPL. Volume: 91 (2010) - 2010 • 398
Micro- to nanostructured devices for the characterization of scaling effects in shape-memory thin films
König, D. and Ehmann, M. and Thienhaus, S. and Ludwig, Al.
JOURNAL OF MICROELECTROMECHANICAL SYSTEMS. Volume: 19 (2010) - 2010 • 397
High-rate electrochemical capacitors based on ordered mesoporous silicon carbide-derived carbon
Korenblit, Y. and Rose, M. and Kockrick, E. and Borchardt, L. and Kvit, A. and Kaskel, S. and Yushin, G.
ACS NANO. Volume: 4 (2010) - 2010 • 396
Configurational forces and couples in fracture mechanics accounting for microstructures and dissipation
Stumpf, H. and Makowski, J. and Hackl, K.
INTERNATIONAL JOURNAL OF SOLIDS AND STRUCTURES. Volume: 47 (2010) - 2010 • 395
Effects of boron on the microstructure and thermal stability of directionally solidified NiAl-Mo eutectic
Gali, A. and Bei, H. and George, E.P.
ACTA MATERIALIA. Volume: 58 (2010) - 2010 • 394
Effects of antenna characteristics and placements on a vehicle-to-vehicle channel scenario
Kornek, D. and Schack, M. and Slottke, E. and Klemp, O. and Rolfes, I. and Kürner, T.
2010 IEEE INTERNATIONAL CONFERENCE ON COMMUNICATIONS WORKSHOPS, ICC 2010. Volume: (2010) - 2010 • 393
Coupled DEM-CFD simulation of pneumatically conveyed granular media
Sturm, M. and Wirtz, S. and Scherer, V. and Denecke, J.
CHEMICAL ENGINEERING AND TECHNOLOGY. Volume: 33 (2010) - 2010 • 392
Coupled Discrete Element (DEM) - Continuous Fluid (CFD) method for the application of pneumatic conveyed granular media
Sturm, M. and Wirtz, S. and Scherer, V. and Denecke, J.
AMERICAN SOCIETY OF MECHANICAL ENGINEERS, PRESSURE VESSELS AND PIPING DIVISION (PUBLICATION) PVP. Volume: 4 (2010) - 2010 • 391
An experimental analysis on mixing and stoking of monodisperse spheres on a grate
Sudbrock, F. and Simsek, E. and Wirtz, S. and Scherer, V.
POWDER TECHNOLOGY. Volume: 198 (2010) - 2009 • 390
Electro catalysis in fuel cells and electrolysis units: Carbon-nano tubes-based catalysts and novel study methods
Bron, M. and Xia, W. and Chen, X. and Jin, C. and Kundu, S. and Nagaiah, T.L. and Chetty, R. and Schilling, T. and Li, N. and Schuhmann, W. and Muhler, M.
CHEMIE-INGENIEUR-TECHNIK. Volume: 81 (2009) - 2009 • 389
Visualization of the local catalytic activity of electrodeposited Pt-Ag catalysts for oxygen reduction by means of SECM
Nagaiah, T.C. and Maljusch, A. and Chen, X. and Bron, M. and Schuhmann, W.
CHEMPHYSCHEM. Volume: 10 (2009) - 2009 • 388
Multi-axial thermo-mechanical fatigue of a near-gamma TiAI-alloy
Brookes, S.P. and Kühn, H.-J. and Skrotzki, B. and Klingelhöffer, H. and Sievert, R. and Pfetzing, J. and Peter, D. and Eggeler, G.
ADVANCED MATERIALS RESEARCH. Volume: 59 (2009) - 2009 • 387
Fracture simulation of CFRP laminates in mixed mode bending
Naghipour, P. and Schneider, J. and Bartsch, M. and Hausmann, J. and Voggenreiter, H.
ENGINEERING FRACTURE MECHANICS. Volume: 76 (2009) - 2009 • 386
Odorant-dependent generation of nitric oxide in mammalian olfactory sensory neurons
Brunert, D. and Kurtenbach, S. and Isik, S. and Benecke, H. and Gisselmann, G. and Schuhmann, W. and Hatt, H. and Wetzel, C.H.
PLOS ONE. Volume: 4 (2009) - 2009 • 385
Enhanced thermal stability and nanoparticle-mediated surface patterning: Pt/ TiO2 (110)
Naitabdi, A. and Behafarid, F. and Cuenya, B.R.
APPLIED PHYSICS LETTERS. Volume: 94 (2009) - 2009 • 384
Signature of singlet-triplet crossing in PL in GaAs QW's
Bryja, L. and Wójs, A. and Jadczak, J. and Misiewicz, J. and Płochocka, P. and Maude, D. and Potemski, M. and Reuter, D. and Wieck, A.
AIP CONFERENCE PROCEEDINGS. Volume: 1199 (2009) - 2009 • 383
Thermal stability and segregation processes in self-assembled size-selected au xfe 1-x nanoparticles deposited on tio 2(110): composition effects
Naitabdi, A. and Ono, L.K. and Behafarid, F. and Cuenya, B.R.
JOURNAL OF PHYSICAL CHEMISTRY C. Volume: 113 (2009) - 2009 • 382
Magnetic ordering in EuRh2 As2 studied by x-ray resonant magnetic scattering
Nandi, S. and Kreyssig, A. and Lee, Y. and Singh, Y. and Kim, J.W. and Johnston, D.C. and Harmon, B.N. and Goldman, A.I.
PHYSICAL REVIEW B - CONDENSED MATTER AND MATERIALS PHYSICS. Volume: 79 (2009) - 2009 • 381
Anisotropic electron spin relaxation in bulk GaN
Bu, J.H. and Rudolph, J. and Natali, F. and Semond, F. and Hägele, D.
APPLIED PHYSICS LETTERS. Volume: 95 (2009) - 2009 • 380
Crystal structure of MCM-22 (MWW) and Its delaminated zeolite ITQ-2 from high-resolution powder X-Ray diffraction data: An analysis using rietveld technique and atomic pair distribution function
Narkhede, V.V. and Gies, H.
CHEMISTRY OF MATERIALS. Volume: 21 (2009) - 2009 • 379
Magnetic-field reentrant wave function hybridization in stacked one-dimensional electron systems
Buchholz, S.S. and Zapp, P.S. and Fischer, S.F. and Kunze, U. and Schuh, D. and Abstreiter, G.
AIP CONFERENCE PROCEEDINGS. Volume: 1199 (2009) - 2009 • 378
Pt nanoparticles inside the mesopores of TiO2-MCM-48: Synthesis, characterization and catalytic activity for CO oxidation
Narkhede, V.V. and De Toni, A. and Narkhede, V.S. and Reichinger, M. and Birkner, A. and Niemantsverdriet, J.W. and Grünert, W. and Gies, H.
JOURNAL OF MATERIALS SCIENCE. Volume: 44 (2009) - 2009 • 377
Au/TiO2 catalysts encapsulated in the mesopores of siliceous MCM-48 - Reproducible synthesis, structural characterization and activity for CO oxidation
Narkhede, V.S. and Toni, A.D. and Narkhede, V.V. and Guraya, M. and (Hans) Niemantsverdriet, J.W. and van den Berg, M.W.E. and Grünert, W. and Gies, H.
MICROPOROUS AND MESOPOROUS MATERIALS. Volume: 118 (2009) - 2009 • 376
Nonlocal Aharonov-Bohm conductance oscillations in an asymmetric quantum ring
Buchholz, S.S. and Fischer, S.F. and Kunze, U. and Reuter, D. and Wieck, A.D.
APPLIED PHYSICS LETTERS. Volume: 94 (2009) - 2009 • 375
Selective surface oxidation and nitridation of NiTi shape memory alloys by reduction annealing
Neelakantan, L. and Swaminathan, S. and Spiegel, M. and Eggeler, G. and Hassel, A.W.
CORROSION SCIENCE. Volume: 51 (2009) - 2009 • 374
Structural phase transition in Ba (Fe0.973 Cr0.027) 2 As2 single crystals
Bud'ko, S.L. and Nandi, S. and Ni, N. and Thaler, A. and Kreyssig, A. and Kracher, A. and Yan, J.-Q. and Goldman, A.I. and Canfield, P.C.
PHYSICAL REVIEW B - CONDENSED MATTER AND MATERIALS PHYSICS. Volume: 80 (2009) - 2009 • 373
Reaction mechanisms of thiamin diphosphate enzymes: Defining states of ionization and tautomerization of the cofactor at individual steps
Nemeria, N.S. and Chakraborty, S. and Balakrishnan, A. and Jordan, F.
FEBS JOURNAL. Volume: 276 (2009) - 2009 • 372
Vertical modulator array for fast intra-cavity wavelength control
Bülters, M. and Breede, M. and Hofmann, M. and Jäger, D.
CLEO/EUROPE - EQEC 2009 - EUROPEAN CONFERENCE ON LASERS AND ELECTRO-OPTICS AND THE EUROPEAN QUANTUM ELECTRONICS CONFERENCE. Volume: (2009) - 2009 • 371
Nanosecond switching and wavelength tuning of external-cavity laser diode using a reflective electroabsorption modulator
Bülters, M. and Breede, M. and Hofmann, M. and Jäger, D.
IEEE PHOTONICS TECHNOLOGY LETTERS. Volume: 21 (2009) - 2009 • 370
Rigid molecule approximation in memory function-based models for molecular liquids: Application to liquid water
Calandrini, V. and Sutmann, G. and Deriu, A. and Kneller, G.R.
ZEITSCHRIFT FUR PHYSIKALISCHE CHEMIE. Volume: 223 (2009) - 2009 • 369
Optimization of an electrochemical DNA assay by using a 48-electrode array and redox amplification studies by means of scanning electrochemical microscopy
Neugebauer, S. and Zimdars, A. and Liepold, P. and Gebala, M. and Schuhmann, W. and Hartwich, G.
CHEMBIOCHEM. Volume: 10 (2009) - 2009 • 368
Structural, magnetic and superconducting phase transitions in CaFe2As2 under ambient and applied pressure
Canfield, P.C. and Bud'ko, S.L. and Ni, N. and Kreyssig, A. and Goldman, A.I. and McQueeney, R.J. and Torikachvili, M.S. and Argyriou, D.N. and Luke, G. and Yu, W.
PHYSICA C: SUPERCONDUCTIVITY AND ITS APPLICATIONS. Volume: 469 (2009) - 2009 • 367
Combinatorial polymer synthesis as a tool in biosensor and biofuel cell development and optimization
Ngounou, B. and Guschin, D.A. and Castillo, J. and Schuhmann, W.
ECS TRANSACTIONS. Volume: 19 (2009) - 2009 • 366
The use of flat punch indentation to determine the viscoelastic properties in the time and frequency domains of a soft layer bonded to a rigid substrate
Cao, Y. and Ma, D. and Raabe, D.
ACTA BIOMATERIALIA. Volume: 5 (2009) - 2009 • 365
Single-crystal growth, crystallography, magnetic susceptibility, heat capacity, and thermal expansion of the antiferromagnetic S=1 chain compound CaV2 O4
Niazi, A. and Bud'Ko, S.L. and Schlagel, D.L. and Yan, J.Q. and Lograsso, T.A. and Kreyssig, A. and Das, S. and Nandi, S. and Goldman, A.I. and Honecker, A. and McCallum, R.W. and Reehuis, M. and Pieper, O. and Lake, B. and Johnston, D.C.
PHYSICAL REVIEW B - CONDENSED MATTER AND MATERIALS PHYSICS. Volume: 79 (2009) - 2009 • 364
Magnetic barrier in a two-dimensional hole gas
Cerchez, M. and Heinzel, T. and Reuter, D. and Wieck, A.D.
SUPERLATTICES AND MICROSTRUCTURES. Volume: 46 (2009) - 2009 • 363
Effect of disorder on the quantum coherence in mesoscopic wires
Niimi, Y. and Baines, Y. and Capron, T. and Mailly, D. and Lo, F.-Y. and Wieck, A.D. and Meunier, T. and Saminadayar, L. and Bäuerle, C.
PHYSICAL REVIEW LETTERS. Volume: 102 (2009) - 2009 • 362
Thermoelectric power of correlated compounds
Oeschler, N. and Hartmann, S. and Köhler, U. and Deppe, M. and Sun, P. and Steglich, F.
NATO SCIENCE FOR PEACE AND SECURITY SERIES B: PHYSICS AND BIOPHYSICS. Volume: (2009) - 2009 • 361
Detection and time course of formation of major thiamin diphosphate-bound covalent intermediates derived from a chromophoric substrate analogue on benzoylformate decarboxylase
Chakraborty, S. and Nemeria, N.S. and Balakrishnan, A. and Brandt, G.S. and Kneen, M.M. and Yep, A. and McLeish, M.J. and Kenyon, G.L. and Petsko, G.A. and Ringe, D. and Jordan, F.
BIOCHEMISTRY. Volume: 48 (2009) - 2009 • 360
In situ observation of dislocation nucleation and escape in a submicrometre aluminium single crystal
Oh, S.H. and Legros, M. and Kiener, D. and Dehm, G.
NATURE MATERIALS. Volume: 8 (2009) - 2009 • 359
Hydrosilylation of aldehydes and ketones catalyzed by Nickel PCP-Píncer hydride complexes
Chakraborty, S. and Krause, J.A. and Guan, H.
ORGANOMETALLICS. Volume: 28 (2009) - 2009 • 358
Visualization of local electrocatalytic activity of metalloporphyrins towards oxygen reduction by means of redox competition scanning electrochemical microscopy (RC-SECM)
Okunola, A.O. and Nagaiah, T.C. and Chen, X. and Eckhard, K. and Schuhmann, W. and Bron, M.
ELECTROCHIMICA ACTA. Volume: 54 (2009) - 2009 • 357
Cooper-pair formation by anharmonic rattling modes in the β-pyrochlore superconductor Kos2O6
Chang, J. and Eremin, I. and Thalmeier, P.
NEW JOURNAL OF PHYSICS. Volume: 11 (2009) - 2009 • 356
Electrocatalytic reduction of oxygen at electropolymerized films of metalloporphyrins deposited onto multi-walled carbon nanotubes
Okunola, A. and Kowalewska, B. and Bron, M. and Kulesza, P.J. and Schuhmann, W.
ELECTROCHIMICA ACTA. Volume: 54 (2009) - 2009 • 355
Enhanced plasticity in a Zr-based bulk metallic glass composite with in situ formed intermetallic phases
Chen, G. and Bei, H. and Cao, Y. and Gali, A. and Liu, C.T. and George, E.P.
APPLIED PHYSICS LETTERS. Volume: 95 (2009) - 2009 • 354
Mechanical and microstructural observations during compression creep of a short fiber reinforced AlMg metal matrix composite
Olbricht, J. and Yawny, A. and Young, M.L. and Eggeler, G.
MATERIALS SCIENCE AND ENGINEERING A. Volume: 510-511 (2009) - 2009 • 353
Influence of vibrational entropy on structural stability of Nb-Si and Mo-Si systems at elevated temperatures
Chen, Y. and Hammerschmidt, T. and Pettifor, D.G. and Shang, J.-X. and Zhang, Y.
ACTA MATERIALIA. Volume: 57 (2009) - 2009 • 352
Surface chemistry of carbon-templated mesoporous aluminas
Onfroy, T. and Li, W.-C. and Schüth, F. and Knözinger, H.
PHYSICAL CHEMISTRY CHEMICAL PHYSICS. Volume: 11 (2009) - 2009 • 351
Electrocatalysis on gold nanostructures: Is the {1 1 0} facet more active than the {1 1 1} facet?
Chen, Y. and Schuhmann, W. and Hassel, A.W.
ELECTROCHEMISTRY COMMUNICATIONS. Volume: 11 (2009) - 2009 • 350
Laser
Ostendorf, A. and Hundertmark, H.
TECHNOLOGY GUIDE: PRINCIPLES - APPLICATIONS - TRENDS. Volume: (2009) - 2009 • 349
Electrocatalytic activity of spots of electrodeposited noble-metal catalysts on carbon nanotubes modified glassy carbon
Chen, X. and Eckhard, K. and Zhou, M. and Bron, M. and Schuhmann, W.
ANALYTICAL CHEMISTRY. Volume: 81 (2009) - 2009 • 348
Reversible immobilization of a molecular catalyst and challenges of catalyst characterization
Palkovits, R. and Arlt, D. and Stepowska, H. and Schüth, F.
CHEMISTRY - A EUROPEAN JOURNAL. Volume: 15 (2009) - 2009 • 347
Fabrication of single crystalline gold nanobelts
Chen, Y. and Somsen, C. and Milenkovic, S. and Hassel, A.W.
JOURNAL OF MATERIALS CHEMISTRY. Volume: 19 (2009) - 2009 • 346
Solid catalysts for the selective low-temperature oxidation of methane to methanol
Palkovits, R. and Antonietti, M. and Kuhn, P. and Thomas, A. and Schüth, F.
ANGEWANDTE CHEMIE - INTERNATIONAL EDITION. Volume: 48 (2009) - 2009 • 345
Redox potentials and pKa for benzoquinone from density functional theory based molecular dynamics
Cheng, J. and Sulpizi, M. and Sprik, M.
JOURNAL OF CHEMICAL PHYSICS. Volume: 131 (2009) - 2009 • 344
Crosslinked TS-1 as stable catalyst for the Beckmann rearrangement of cyclohexanone oxime
Palkovits, R. and Schmidt, W. and Ilhan, Y. and Erdem-Şenatalar, A. and Schüth, F.
MICROPOROUS AND MESOPOROUS MATERIALS. Volume: 117 (2009) - 2009 • 343
Dynamics of the nuclear spin polarization by optically oriented electrons in a (In,Ga)As/GaAs quantum dot ensemble
Cherbunin, R.V. and Verbin, S.Yu. and Auer, T. and Yakovlev, D.R. and Reuter, D. and Wieck, A.D. and Gerlovin, I.Ya. and Ignatiev, I.V. and Vishnevsky, D.V. and Bayer, M.
PHYSICAL REVIEW B - CONDENSED MATTER AND MATERIALS PHYSICS. Volume: 80 (2009) - 2009 • 342
Competitive adsorption of glycine and water on the fluorapatite (100) surface
Pareek, A. and Torrelles, X. and Angermund, K. and Rius, J. and Magdans, U. and Gies, H.
LANGMUIR. Volume: 25 (2009) - 2009 • 341
PtRu nanoparticles supported on nitrogen-doped multiwalled carbon nanotubes as catalyst for methanol electrooxidation
Chetty, R. and Kundu, S. and Xia, W. and Bron, M. and Schuhmann, W. and Chirila, V. and Brandl, W. and Reinecke, T. and Muhler, M.
ELECTROCHIMICA ACTA. Volume: 54 (2009) - 2009 • 340
Effect of reduction temperature on the preparation and characterization of Pt-Ru nanoparticles on multiwalled carbon nanotubes
Chetty, R. and Xia, W. and Kundu, S. and Bron, M. and Reinecke, T. and Schuhmann, W. and Muhler, M.
LANGMUIR. Volume: 25 (2009) - 2009 • 339
Mechanochemical synthesis of ternary potassium transition metal chlorides
Pawelke, R.H. and Felderhoff, M. and Weidenthaler, C. and Bogdanovíc, B. and Schüth, F.
ZEITSCHRIFT FUR ANORGANISCHE UND ALLGEMEINE CHEMIE. Volume: 635 (2009) - 2009 • 338
Theory of Raman response of a superconductor with extended s -wave symmetry: Application to the iron pnictides
Chubukov, A.V. and Eremin, I. and Korshunov, M.M.
PHYSICAL REVIEW B - CONDENSED MATTER AND MATERIALS PHYSICS. Volume: 79 (2009) - 2009 • 337
Grain-boundary sliding in a TiAl alloy with fine-grained duplex microstructure during 750 °C creep
Peter, D. and Viswanathan, G.B. and Wagner, M.F.-X. and Eggeler, G.
MATERIALS SCIENCE AND ENGINEERING A. Volume: 510-511 (2009) - 2009 • 336
Structural stability of intermetallic phases in the Sn-Ti system
Colinet, C. and Tedenac, J.-C. and Fries, S.G.
CALPHAD: COMPUTER COUPLING OF PHASE DIAGRAMS AND THERMOCHEMISTRY. Volume: 33 (2009) - 2009 • 335
Microstructural anisotropy, uniaxial and biaxial creep behavior of Ti-45Al-5Nb-0.2B-0.2C
Peter, D. and Pfetzing, J. and Wagner, M.F.-X. and Eggeler, G.
MATERIALS SCIENCE AND ENGINEERING A. Volume: 510-511 (2009) - 2009 • 334
The role of C2H4 for the acetylene chemistry in particle forming Ar/He/C2H2 plasmas studied via quantitative mass spectrometry
Consoli, A. and Benedikt, J. and Von Keudell, A.
PLASMA SOURCES SCIENCE AND TECHNOLOGY. Volume: 18 (2009) - 2009 • 333
Using ab initio calculations in designing bcc Mg-Li alloys for ultra-lightweight applications
Counts, W.A. and Friák, M. and Raabe, D. and Neugebauer, J.
ACTA MATERIALIA. Volume: 57 (2009) - 2009 • 332
Size-selected pt nanoparticles synthesized via micelle encapsulation: Effect of pretreatment and oxidation state on the activity for methanol decomposition and oxidation
Croy, J.R. and Mostafa, S. and Heinrich, H. and Cuenya, B.R.
CATALYSIS LETTERS. Volume: 131 (2009) - 2009 • 331
Nanoindentation of pseudoelastic NiTi shape memory alloys: Thermomechanical and microstructural aspects
Pfetzing, J. and Schaefer, A. and Somsen, C. and Wagner, M.F.-X.
INTERNATIONAL JOURNAL OF MATERIALS RESEARCH. Volume: 100 (2009) - 2009 • 330
Absence of structural correlations of magnetic defects in the heavy-fermion compound LiV2 O4
Das, S. and Kreyssig, A. and Nandi, S. and Goldman, A.I. and Johnston, D.C.
PHYSICAL REVIEW B - CONDENSED MATTER AND MATERIALS PHYSICS. Volume: 80 (2009) - 2009 • 329
Kinetics of reverse resistance recovery of silicon diodes: The role of the distance the metallurgical p+n-junction-defect layer formed by 250 MeV krypton implantation
Poklonski, N.A. and Gorbachuk, N.I. and Shpakovski, S.V. and Filipenia, V.A. and Skuratov, V.A. and Wieck, A.
PHYSICA B: CONDENSED MATTER. Volume: 404 (2009) - 2009 • 328
Petrology of an inverted Barrovian sequence of metapelites in Sikkim Himalaya, India: Constraints on the tectonics of inversion
Dasgupta, S. and Chakraborty, S. and Neogi, S.
AMERICAN JOURNAL OF SCIENCE. Volume: 309 (2009) - 2009 • 327
Hafnium carbamates and ureates: New class of precursors for low-temperature growth of HfO2 thin films
Pothiraja, R. and Milanov, A.P. and Barreca, D. and Gasparotto, A. and Becker, H.-W. and Winter, M. and Fischer, R.A. and Devi, A.
CHEMICAL COMMUNICATIONS. Volume: (2009) - 2009 • 326
Microstructure characterization of tool steel claddings Co-extruded on low alloyed steel substrates
Da Souza e Silva, P.A. and Weber, S. and Kostka, A. and Pyzalla, A.R.
ADVANCED ENGINEERING MATERIALS. Volume: 11 (2009) - 2009 • 325
Monomeric malonate precursors for the MOCVD of HfO2 and ZrO 2 thin films
Pothiraja, R. and Milanov, A. and Parala, H. and Winter, M. and Fischer, R.A. and Devi, A.
DALTON TRANSACTIONS. Volume: (2009) - 2009 • 324
Coexistence of competing antiferromagnetic and superconducting phases in the underdoped Ba(Fe0.953Co0.047)2As2 compound using X-ray and neutron scattering techniques
Pratt, D.K. and Tian, W. and Kreyssig, A. and Zarestky, J.L. and Nandi, S. and Ni, N. and Bud'Ko, S.L. and Canfield, P.C. and Goldman, A.I. and McQueeney, R.J.
PHYSICAL REVIEW LETTERS. Volume: 103 (2009) - 2009 • 323
Can micro-compression testing provide stress-strain data for thin films?. A comparative study using Cu, VN, TiN and W coatings
Dehm, G. and Wörgötter, H.P. and Cazottes, S. and Purswani, J.M. and Gall, D. and Mitterer, C. and Kiener, D.
THIN SOLID FILMS. Volume: 518 (2009) - 2009 • 322
Suppression of antiferromagnetic spin fluctuations in the collapsed phase of CaFe2 As2
Pratt, D.K. and Zhao, Y. and Kimber, S.A.J. and Hiess, A. and Argyriou, D.N. and Broholm, C. and Kreyssig, A. and Nandi, S. and Bud'Ko, S.L. and Ni, N. and Canfield, P.C. and McQueeney, R.J. and Goldman, A.I.
PHYSICAL REVIEW B - CONDENSED MATTER AND MATERIALS PHYSICS. Volume: 79 (2009) - 2009 • 321
Miniaturized single-crystalline fcc metals deformed in tension: New insights in size-dependent plasticity
Dehm, G.
PROGRESS IN MATERIALS SCIENCE. Volume: 54 (2009) - 2009 • 320
Intrinsic pinning on structural domains in underdoped single crystals of Ba (Fe1-x Cox) 2 As2
Prozorov, R. and Tanatar, M.A. and Ni, N. and Kreyssig, A. and Nandi, S. and Bud'Ko, S.L. and Goldman, A.I. and Canfield, P.C.
PHYSICAL REVIEW B - CONDENSED MATTER AND MATERIALS PHYSICS. Volume: 80 (2009) - 2009 • 319
Silicon oxide permeation barrier coating and plasma sterilization of PET bottles and foils
Deilmann, M. and Mann, H.H. and Steves, S. and Bibinov, N. and Awakowicz, P.
PLASMA PROCESSES AND POLYMERS. Volume: 6 (2009) - 2009 • 318
Investigation of the indentation size effect through the measurement of the geometrically necessary dislocations beneath small indents of different depths using EBSD tomography
Demir, E. and Raabe, D. and Zaafarani, N. and Zaefferer, S.
ACTA MATERIALIA. Volume: 57 (2009) - 2009 • 317
Microstructures of Co-Re-Cr, Mo-Si and Mo-Si-B high-temperature alloys
Depka, T. and Somsen, C. and Eggeler, G. and Mukherji, D. and Rösler, J. and Krüger, M. and Saage, H. and Heilmaier, M.
MATERIALS SCIENCE AND ENGINEERING A. Volume: 510-511 (2009) - 2009 • 316
Mechanical alloying and amorphization in Cu-Nb-Ag in situ composite wires studied by transmission electron microscopy and atom probe tomography
Raabe, D. and Ohsaki, S. and Hono, K.
ACTA MATERIALIA. Volume: 57 (2009) - 2009 • 315
Some asymptotic properties of the spectrum of the jacobi ensemble
Dette, H. and Nagel, J.
SIAM JOURNAL ON MATHEMATICAL ANALYSIS. Volume: 41 (2009) - 2009 • 314
Designing ultrahigh strength steels with good ductility by combining transformation induced plasticity and martensite aging
Raabe, D. and Ponge, D. and Dmitrieva, O. and Sander, B.
ADVANCED ENGINEERING MATERIALS. Volume: 11 (2009) - 2009 • 313
A simple test for the parametric form of the variance function in nonparametric regression
Dette, H. and Hetzler, B.
ANNALS OF THE INSTITUTE OF STATISTICAL MATHEMATICS. Volume: 61 (2009) - 2009 • 312
Nanoprecipitate-hardened 1.5 GPa steels with unexpected high ductility
Raabe, D. and Ponge, D. and Dmitrieva, O. and Sander, B.
SCRIPTA MATERIALIA. Volume: 60 (2009) - 2009 • 311
A geometric characterization of c-optimal designs for heteroscedastic regression
Dette, H. and Holland-Letz, T.
ANNALS OF STATISTICS. Volume: 37 (2009) - 2009 • 310
Influence of nitrogen doping on growth rate and texture evolution of chemical vapor deposition diamond films
Raabe, D. and Liu, T.
APPLIED PHYSICS LETTERS. Volume: 94 (2009) - 2009 • 309
Goodness-of-fit tests for multiplicative models with dependent data
Dette, H. and Pardo-FernÁndez, J.C. and Keilegom, I.V.
SCANDINAVIAN JOURNAL OF STATISTICS. Volume: 36 (2009) - 2009 • 308
Deposition of silicon dioxide films using an atmospheric pressure microplasma jet
Raballand, V. and Benedikt, J. and Hoffmann, S. and Zimmermann, M. and Von Keudell, A.
JOURNAL OF APPLIED PHYSICS. Volume: 105 (2009) - 2009 • 307
DBD plasma source operated in single-filamentary mode for therapeutic use in dermatology
Rajasekaran, P. and Mertmann, P. and Bibinov, N. and Wandke, D. and Viöl, W. and Awakowicz, P.
JOURNAL OF PHYSICS D: APPLIED PHYSICS. Volume: 42 (2009) - 2009 • 306
Bootstrapping frequency domain tests in multivariate time series with an application to comparing spectral densities
Dette, H. and Paparoditis, E.
JOURNAL OF THE ROYAL STATISTICAL SOCIETY. SERIES B: STATISTICAL METHODOLOGY. Volume: 71 (2009) - 2009 • 305
Deformation twinning in Ni-Mn-Ga micropillars with 10M martensite
Reinhold, M. and Kiener, D. and Knowlton, W.B. and Dehm, G. and Müllner, P.
JOURNAL OF APPLIED PHYSICS. Volume: 106 (2009) - 2009 • 304
Optimal discrimination designs
Dette, H. and Titoff, S.
ANNALS OF STATISTICS. Volume: 37 (2009) - 2009 • 303
Implementation of the Müller-Achenbach-Seelecke model for shape memory alloys in ABAQUS
Richter, F. and Kastner, O. and Eggeler, G.
JOURNAL OF MATERIALS ENGINEERING AND PERFORMANCE. Volume: 18 (2009) - 2009 • 302
Khmaladze transformation of integrated variance processes with applications to goodness-of-fit testing
Dette, H. and Hetzler, B.
MATHEMATICAL METHODS OF STATISTICS. Volume: 18 (2009) - 2009 • 301
On the estimation of a monotone conditional variance in nonparametric regression
Dette, H. and Pilz, K.
ANNALS OF THE INSTITUTE OF STATISTICAL MATHEMATICS. Volume: 61 (2009) - 2009 • 300
Acid hydrolysis of cellulose as the entry point into biorefinery schemes
Rinaldi, R. and Schüth, F.
CHEMSUSCHEM. Volume: 2 (2009) - 2009 • 299
A bootstrap test for the comparison of nonlinear time series
Dette, H. and Weißbach, R.
COMPUTATIONAL STATISTICS AND DATA ANALYSIS. Volume: 53 (2009) - 2009 • 298
Design of solid catalysts for the conversion of biomass
Rinaldi, R. and Schüth, F.
ENERGY AND ENVIRONMENTAL SCIENCE. Volume: 2 (2009) - 2009 • 297
Optimal desings for dose-finding experiments in toxicity studies
Dette, H. and Pepelyshev, A. and Wong, W.K.
BERNOULLI. Volume: 15 (2009) - 2009 • 296
Testing for a constant coefficient of variation in nonparametric regression
Dette, H. and Wieczorek, G.
JOURNAL OF STATISTICAL THEORY AND PRACTICE. Volume: 3 (2009) - 2009 • 295
The influence of body mass index, age, implants, and dental restorations on image quality of cone beam computed tomography
Ritter, L. and Mischkowski, R.A. and Neugebauer, J. and Dreiseidler, T. and Scheer, M. and Keeve, E. and Zöller, J.E.
ORAL SURGERY, ORAL MEDICINE, ORAL PATHOLOGY, ORAL RADIOLOGY AND ENDODONTOLOGY. Volume: 108 (2009) - 2009 • 294
Asymptotic optimal designs under long-range dependence error structure
Dette, H. and Leonenko, N. and Pepelyshev, A. and Zhigljavsky, A.
BERNOULLI. Volume: 15 (2009) - 2009 • 293
The formation of colloidal copper nanoparticles stabilized by zinc stearate: One-pot single-step synthesis and characterization of the core-shell particles
Rittermeier, A. and Miao, S. and Schröter, M.K. and Zhang, X. and Van Den Berg, M.W.E. and Kundu, S. and Wang, Y. and Schimpf, S. and Löffler, E. and Fischer, R.A. and Muhler, M.
PHYSICAL CHEMISTRY CHEMICAL PHYSICS. Volume: 11 (2009) - 2009 • 292
Robust Designs in Noninferiority Three-Arm Clinical Trials With Presence of Heteroscedasticity
Dette, H. and Trampisch, M. and Hothorn, L. A.
STATISTICS IN BIOPHARMACEUTICAL RESEARCH. Volume: 1 (2009) - 2009 • 291
Structure and phonon density of states of supported size-selected F 57 eAu nanoclusters: A nuclear resonant inelastic x-ray scattering study
Roldan Cuenya, B. and Ono, L.K. and Croy, J.R. and Naitabdi, A. and Heinrich, H. and Zhao, J. and Alp, E.E. and Sturhahn, W. and Keune, W.
APPLIED PHYSICS LETTERS. Volume: 95 (2009) - 2009 • 290
Itinerant magnetic excitations in antiferromagnetic CaFe2As2
Diallo, S.O. and Antropov, V.P. and Perring, T.G. and Broholm, C. and Pulikkotil, J.J. and Ni, N. and Bud'Ko, S.L. and Canfield, P.C. and Kreyssig, A. and Goldman, A.I. and McQueeney, R.J.
PHYSICAL REVIEW LETTERS. Volume: 102 (2009) - 2009 • 289
Phonon density of states of self-assembled isolated Fe-rich Fe-Pt alloy nanoclusters
Roldan Cuenya, B. and Croy, J.R. and Ono, L.K. and Naitabdi, A. and Heinrich, H. and Keune, W. and Zhao, J. and Sturhahn, W. and Alp, E.E. and Hu, M.
PHYSICAL REVIEW B - CONDENSED MATTER AND MATERIALS PHYSICS. Volume: 80 (2009) - 2009 • 288
The effect of disorder on the concentration-dependence of stacking fault energies in fe1-xmnx - A first principles study
Dick, A. and Hickel, T. and Neugebauer, J.
STEEL RESEARCH INTERNATIONAL. Volume: 80 (2009) - 2009 • 287
Ductilization of Mo-Si solid solutions manufactured by powder metallurgy
Saage, H. and Krüger, M. and Sturm, D. and Heilmaier, M. and Schneibel, J.H. and George, E. and Heatherly, L. and Somsen, C. and Eggeler, G. and Yang, Y.
ACTA MATERIALIA. Volume: 57 (2009) - 2009 • 286
Development of an in situ ellipsometer for deposition and erosion studies at the first wall of a tokamak
Dittmar, T. and Kreter, A. and Von Keudell, A.
NUCLEAR FUSION. Volume: 49 (2009) - 2009 • 285
Surface of Ti-Ni alloys after their preparation
Saldan, I. and Frenzel, J. and Shekhah, O. and Chelmowski, R. and Birkner, A. and Wöll, Ch.
JOURNAL OF ALLOYS AND COMPOUNDS. Volume: 470 (2009) - 2009 • 284
Lamination microstructure in shear deformed copper single crystals
Dmitrieva, O. and Dondl, P.W. and Müller, S. and Raabe, D.
ACTA MATERIALIA. Volume: 57 (2009) - 2009 • 283
Spin screening effect in superconductor/ferromagnet thin film heterostructures studied using nuclear magnetic resonance
Salikhov, R.I. and Garif'Yanov, N.N. and Garifullin, I.A. and Tagirov, L.R. and Westerholt, K. and Zabel, H.
PHYSICAL REVIEW B - CONDENSED MATTER AND MATERIALS PHYSICS. Volume: 80 (2009) - 2009 • 282
Experimental observation of the spin screening effect in superconductor/ferromagnet thin film heterostructures
Salikhov, R.I. and Garifullin, I.A. and Garif'Yanov, N.N. and Tagirov, L.R. and Theis-Bröhl, K. and Westerholt, K. and Zabel, H.
PHYSICAL REVIEW LETTERS. Volume: 102 (2009) - 2009 • 281
Electron-phonon interaction and phonon renormalization in the lamellar cobaltate Na x CoO 2
Donkov, A. and Eremin, I. and Knolle, J. and Korshunov, M.M.
JOURNAL OF SUPERCONDUCTIVITY AND NOVEL MAGNETISM. Volume: 22 (2009) - 2009 • 280
Thermopower-enhanced efficiency of Si/SiGe ballistic rectifiers
Salloch, D. and Wieser, U. and Kunze, U. and Hackbarth, T.
APPLIED PHYSICS LETTERS. Volume: 94 (2009) - 2009 • 279
Metallurgy and weld performance in friction stir welding
Dos Santos, J.F. and Olea, C.A.W. and Coelho, R.S. and Kostka, A. and Paglia, C.S. and Ghidini, T. and Donne, C.D.
FRICTION STIR WELDING: FROM BASICS TO APPLICATIONS. Volume: (2009) - 2009 • 278
Laser-assisted micro-forming process with miniaturised structures in sapphire dies
Samm, K. and Terzi, M. and Ostendorf, A. and Wulfsberg, J.
APPLIED SURFACE SCIENCE. Volume: 255 (2009) - 2009 • 277
Excitation wavelength independent sensitized Er3+ concentration in as-deposited and low temperature annealed Si-rich SiO2 films
Savchyn, O. and Todi, R.M. and Coffey, K.R. and Ono, L.K. and Cuenya, B.R. and Kik, P.G.
APPLIED PHYSICS LETTERS. Volume: 95 (2009) - 2009 • 276
Electropolishing of a Nickel-Titanium-Copper shape memory alloy in Methanolic Sulfuric acid
Drensler, S. and Neelakantan, L. and Somsen, C. and Eggeler, G. and Hassel, A.W.
ELECTROCHEMICAL AND SOLID-STATE LETTERS. Volume: 12 (2009) - 2009 • 275
Nucleation of ITQ-21 studied by ESI-MS
Schaack, B.B. and Schrader, W. and Corma, A. and Scḧuth, F.
CHEMISTRY OF MATERIALS. Volume: 21 (2009) - 2009 • 274
Anomalous photoresponse of GaN x-ray Schottky detectors
Duboz, J.-Y. and Beaumont, B. and Reverchon, J.-L. and Wieck, A.D.
JOURNAL OF APPLIED PHYSICS. Volume: 105 (2009) - 2009 • 273
Structural insight into germanium-containing silicate species by electrospray ionization mass spectrometry (ESI-MS) and ESI-MS/MS
Schaack, B.B. and Schrader, W. and Schüth, F.
JOURNAL OF PHYSICAL CHEMISTRY B. Volume: 113 (2009) - 2009 • 272
The EU programme for modelling radiation effects in fusion reactor materials: An overview of recent advances and future goals
Dudarev, S.L. and Boutard, J.-L. and Lässer, R. and Caturla, M.J. and Derlet, P.M. and Fivel, M. and Fu, C.-C. and Lavrentiev, M.Y. and Malerba, L. and Mrovec, M. and Nguyen-Manh, D. and Nordlund, K. and Perlado, M. and Schäublin, R. and Van Swygenhoven, H. and Terentyev, D. and Wallenius, J. and Weygand, D. and Willaime, F.
JOURNAL OF NUCLEAR MATERIALS. Volume: 386-388 (2009) - 2009 • 271
How are heteroelements (ga and ge) incorporated in silicate oligomers?
Schaack, B.B. and Schrader, W. and Schüth, F.
CHEMISTRY - A EUROPEAN JOURNAL. Volume: 15 (2009) - 2009 • 270
Intraoral molluscum contagiosum imitating a squamous-cell carcinoma in an immunocompetent person - case report and review of the literature
Scherer, P. and Fries, J. and Mischkowski, R.A. and Neugebauer, J. and Scheer, M. and Zöller, J.E.
INTERNATIONAL JOURNAL OF ORAL AND MAXILLOFACIAL SURGERY. Volume: 38 (2009) - 2009 • 269
Chemical and physical solutions for hydrogen storage
Eberle, U. and Felderhoff, M. and Schüth, F.
ANGEWANDTE CHEMIE - INTERNATIONAL EDITION. Volume: 48 (2009) - 2009 • 268
Determination of optimum imaging conditions in AC-SECM using the mathematical distance between approach curves displayed in the impedance domain
Eckhard, K. and Schuhmann, W. and Maciejewska, M.
ELECTROCHIMICA ACTA. Volume: 54 (2009) - 2009 • 267
Methanol Catalysts
Schimpf, S. and Muhler, M.
SYNTHESIS OF SOLID CATALYSTS. Volume: (2009) - 2009 • 266
Novel experimentation techniques to study damage accumulation and microstructural instability during creep of super alloy single crystals at high temperatures (>1000°C)
Eggeler, G.
MATERIALS SCIENCE AND TECHNOLOGY. Volume: 25 (2009) - 2009 • 265
High-resolution transmission electron microscopy and electron backscatter diffraction in nanoscaled ferritic and ferritic-martensitic oxide dispersion strengthened-steels
Eiselt, Ch.Ch. and Klimenkov, M. and Lindau, R. and Möslang, A. and Sandim, H.R.Z. and Padilha, A.F. and Raabe, D.
JOURNAL OF NUCLEAR MATERIALS. Volume: 385 (2009) - 2009 • 264
Challenges in hydrogen storage
Schüth, F.
EUROPEAN PHYSICAL JOURNAL: SPECIAL TOPICS. Volume: 176 (2009) - 2009 • 263
Texture prediction from a novel grain cluster-based homogenization scheme
Eisenlohr, P. and Tjahjanto, D.D. and Hochrainer, T. and Roters, F. and Raabe, D.
INTERNATIONAL JOURNAL OF MATERIAL FORMING. Volume: 2 (2009) - 2009 • 262
Comparison of texture evolution in fcc metals predicted by various grain cluster homogenization schemes
Eisenlohr, P. and Tjahjanto, D.D. and Hochrainer, T. and Roters, F. and Raabe, D.
INTERNATIONAL JOURNAL OF MATERIALS RESEARCH. Volume: 100 (2009) - 2009 • 261
Photoluminescence upconversion in GaAs quantum wells
Eshlaghi, S. and Worthoff, W. and Wieck, A.D. and Suter, D.
PROCEEDINGS OF SPIE - THE INTERNATIONAL SOCIETY FOR OPTICAL ENGINEERING. Volume: 7228 (2009) - 2009 • 260
Vortex imaging in Co-doped BaFe2As2
Eskildsen, M.R. and Vinnikov, L.Ya. and Veshchunov, I.S. and Artemova, T.M. and Blasius, T.D. and Densmore, J.M. and Dewhurst, C.D. and Ni, N. and Kreyssig, A. and Bud'ko, S.L. and Canfield, P.C. and Goldman, A.I.
PHYSICA C: SUPERCONDUCTIVITY AND ITS APPLICATIONS. Volume: 469 (2009) - 2009 • 259
Local modulation of the redox state of p-nitrothiophenol self-assembled monolayers using the direct mode of scanning electrochemical microscopy
Schwamborn, S. and Stoica, L. and Neugebauer, S. and Reda, T. and Schmidt, H.-L. and Schuhmann, W.
CHEMPHYSCHEM. Volume: 10 (2009) - 2009 • 258
Vortices in superconducting Ba(Fe 0.93Co 0.07) 2As 2 studied via small-angle neutron scattering and Bitter decoration
Eskildsen, M.R. and Vinnikov, L.Ya. and Blasius, T.D. and Veshchunov, I.S. and Artemova, T.M. and Densmore, J.M. and Dewhurst, C.D. and Ni, N. and Kreyssig, A. and Bud'ko, S.L. and Canfield, P.C. and Goldman, A.I.
PHYSICAL REVIEW B - CONDENSED MATTER AND MATERIALS PHYSICS. Volume: 79 (2009) - 2009 • 257
Effects of focused ion beam milling on the compressive behavior of directionally solidified micropillars and the nanoindentation response of an electropolished surface
Shim, S. and Bei, H. and Miller, M.K. and Pharr, G.M. and George, E.P.
ACTA MATERIALIA. Volume: 57 (2009) - 2009 • 256
Influence of structural principles on the mechanics of a biological fiber-based composite material with hierarchical organization: The exoskeleton of the lobster homarus americanus
Fabritius, H.-O. and Sachs, C. and Triguero, P.R. and Raabe, D.
ADVANCED MATERIALS. Volume: 21 (2009) - 2009 • 255
Si and O diffusion in (Mg,Fe)2SiO4 wadsleyite and ringwoodite and its implications for the rheology of the mantle transition zone
Shimojuku, A. and Kubo, T. and Ohtani, E. and Nakamura, T. and Okazaki, R. and Dohmen, R. and Chakraborty, S.
EARTH AND PLANETARY SCIENCE LETTERS. Volume: 284 (2009) - 2009 • 254
Bioelectrochemical detection of L-lactate respiration using genetically modified Hansenula polymorpha yeast cells overexpressing flavocytochrome b2
Shkil, H. and Stoica, L. and Dmytruk, K. and Smutok, O. and Gonchar, M. and Sibirny, A. and Schuhmann, W.
BIOELECTROCHEMISTRY. Volume: 76 (2009) - 2009 • 253
Lateral electron tunnelling spectroscopy in etched GaAs/AlGaAs-based nanostructures
Fischer, S.F. and Deborde, J.L. and Kunze, U. and Reuter, D. and Wieck, A.D.
JOURNAL OF PHYSICS: CONFERENCE SERIES. Volume: 193 (2009) - 2009 • 252
Adsorption orientation and STM imaging of meta-benzyne resolved by scanning tunnelling microscopy and ab initio calculations
Simic-Milosevic, V. and Bocquet, M.-L. and Morgenstern, K.
SURFACE SCIENCE. Volume: 603 (2009) - 2009 • 251
Direct minimization technique for metals in density functional theory
Freysoldt, C. and Boeck, S. and Neugebauer, J.
PHYSICAL REVIEW B - CONDENSED MATTER AND MATERIALS PHYSICS. Volume: 79 (2009) - 2009 • 250
Bending a bond within an individual adsorbed molecule
Simic-Milosevic, V. and Morgenstern, K.
JOURNAL OF THE AMERICAN CHEMICAL SOCIETY. Volume: 131 (2009) - 2009 • 249
Fully Ab initio finite-size corrections for charged-defect supercell calculations
Freysoldt, C. and Neugebauer, J. and Van De Walle, C.G.
PHYSICAL REVIEW LETTERS. Volume: 102 (2009) - 2009 • 248
Magnetic order in BaMn2 As2 from neutron diffraction measurements
Singh, Y. and Green, M.A. and Huang, Q. and Kreyssig, A. and McQueeney, R.J. and Johnston, D.C. and Goldman, A.I.
PHYSICAL REVIEW B - CONDENSED MATTER AND MATERIALS PHYSICS. Volume: 80 (2009) - 2009 • 247
Josephson tunnel junctions with ferromagnetic Fe0.75Co 0.25 barriers
Sprungmann, D. and Westerholt, K. and Zabel, H. and Weides, M. and Kohlstedt, H.
JOURNAL OF PHYSICS D: APPLIED PHYSICS. Volume: 42 (2009) - 2009 • 246
Compact semiconductor laser sources for photoacoustic imaging
Friedrich, C.-S. and Wawreczko, M.-C. and Mienkina, M.P. and Gerhardt, N.C. and Schmitz, G. and Hofmann, M.R.
PROGRESS IN BIOMEDICAL OPTICS AND IMAGING - PROCEEDINGS OF SPIE. Volume: 7177 (2009) - 2009 • 245
THz sources and detectors based on diode lasers
Friedrich, C.-S. and Brenner, C. and Hoffmann, S. and Schlauch, T. and Klehr, A. and Erbert, G. and Tränkle, G. and Jördens, C. and Salhi, M. and Koch, M. and Hofmann, M.R.
PROCEEDINGS OF SPIE - THE INTERNATIONAL SOCIETY FOR OPTICAL ENGINEERING. Volume: 7197 (2009) - 2009 • 244
Lifetime-oriented structural design concepts
Stangenberg, F. and Breitenbücher, R. and Bruhns, O.T. and Hartmann, D. and Höffer, R. and Kuhl, D. and Meschke, G.
LIFETIME-ORIENTED STRUCTURAL DESIGN CONCEPTS. Volume: (2009) - 2009 • 243
Upgrading CALPHAD to microstructure simulation: The phase-field method
Fries, S.G. and Boettger, B. and Eiken, J. and Steinbach, I.
INTERNATIONAL JOURNAL OF MATERIALS RESEARCH. Volume: 100 (2009) - 2009 • 242
Phase-field models in materials science
Steinbach, I.
MODELLING AND SIMULATION IN MATERIALS SCIENCE AND ENGINEERING. Volume: 17 (2009) - 2009 • 241
Pattern formation in constrained dendritic growth with solutal buoyancy
Steinbach, I.
ACTA MATERIALIA. Volume: 57 (2009) - 2009 • 240
Microstructure and structural fatigue of ultra-fine grained NiTi-stents
Frotscher, M. and Nörtershäuser, P. and Somsen, C. and Neuking, K. and Böckmann, R. and Eggeler, G.
MATERIALS SCIENCE AND ENGINEERING A. Volume: 503 (2009) - 2009 • 239
Membrane-Less Biofuel Cell Based on Cellobiose Dehydrogenase (Anode)/Laccase (Cathode) Wired via Specific Os-Redox Polymers
Stoica, L. and Dimcheva, N. and Ackermann, Y. and Karnicka, K. and Guschin, D.A. and Kulesza, P.J. and Rogalski, J. and Haltrich, D. and Ludwig, R. and Gorton, L. and Schuhmann, W.
FUEL CELLS. Volume: 9 (2009) - 2009 • 238
Characterization of the mechanical properties of ultra-fine grained NiTiCr-wires
Frotscher, M. and Burow, J. and Schön, P. and Neuking, K. and Böckmann, R. and Eggeler, G.
MATERIALWISSENSCHAFT UND WERKSTOFFTECHNIK. Volume: 40 (2009) - 2009 • 237
Catalytic hydrogenations of tolane and stilbene with Ti- and Ce-doped complex metal hydrides
Streukens, G. and Schüth, F.
JOURNAL OF ALLOYS AND COMPOUNDS. Volume: 474 (2009) - 2009 • 236
Influence of fatigue on the nanohardness of NiTiCr-wires
Frotscher, M. and Young, M. L. and Bei, H. and George, E. P. and Neuking, K. and Eggeler, G.
ESOMAT 2009 - 8TH EUROPEAN SYMPOSIUM ON MARTENSITIC TRANSFORMATIONS. Volume: (2009) - 2009 • 235
A practical guide for optimal designs of experiments in the Monod model
Strigul, N. and Dette, H. and Melas, V.B.
ENVIRONMENTAL MODELLING AND SOFTWARE. Volume: 24 (2009) - 2009 • 234
Modelling and analysis of piezoelectric smart structures for vibration and noise control
Gabbert, U. and Lefèvre, J. and Laugwitz, F. and Nestorović, T.
INTERNATIONAL JOURNAL OF APPLIED ELECTROMAGNETICS AND MECHANICS. Volume: 31 (2009) - 2009 • 233
The surface chemistry of ZnO nanoparticles applied as heterogeneous catalysts in methanol synthesis
Strunk, J. and Kähler, K. and Xia, X. and Muhler, M.
SURFACE SCIENCE. Volume: 603 (2009) - 2009 • 232
Dendritic solidification in undercooled Ni-Zr-Al melts: Experiments and modeling
Galenko, P.K. and Reutzel, S. and Herlach, D.M. and Fries, S.G. and Steinbach, I. and Apel, M.
ACTA MATERIALIA. Volume: 57 (2009) - 2009 • 231
Au/ZnO as catalyst for methanol synthesis: The role of oxygen vacancies
Strunk, J. and Kähler, K. and Xia, X. and Comotti, M. and Schüth, F. and Reinecke, T. and Muhler, M.
APPLIED CATALYSIS A: GENERAL. Volume: 359 (2009) - 2009 • 230
Thermal stability of Cr-Cr3Si eutectic microstructures
Gali, A. and Bei, H. and George, E.P.
ACTA MATERIALIA. Volume: 57 (2009) - 2009 • 229
Dynamical evolution of fracture process region in ductile materials
Stumpf, H. and Makowski, J. and Hackl, K.
INTERNATIONAL JOURNAL OF PLASTICITY. Volume: 25 (2009) - 2009 • 228
Transverse ballistic rectification in density-modulated 2D-systems
Ganczarczyk, A. and Notthoff, C. and Geller, M. and Lorke, A. and Reuter, D. and Wieck, A.D.
AIP CONFERENCE PROCEEDINGS. Volume: 1199 (2009) - 2009 • 227
Effect of exposure conditions on the oxidation of MCrAlY-bondcoats and lifetime of thermal barrier coatings
Subanovic, M. and Song, P. and Wessel, E. and Vassen, R. and Naumenko, D. and Singheiser, L. and Quadakkers, W.J.
SURFACE AND COATINGS TECHNOLOGY. Volume: 204 (2009) - 2009 • 226
Tunability of the piezoelectric fields in strained III-V semiconductors
Garg, R. and Hüe, A. and Haxha, V. and Migliorato, M.A. and Hammerschmidt, T. and Srivastava, G.P.
APPLIED PHYSICS LETTERS. Volume: 95 (2009) - 2009 • 225
Lattice Boltzmann modeling of dendritic growth in a forced melt convection
Sun, D. and Zhu, M. and Pan, S. and Raabe, D.
ACTA MATERIALIA. Volume: 57 (2009) - 2009 • 224
Strain dependence of piezoelectric coefficients for pseudomorphically grown semiconductors
Garg, R. and Haxha, V. and Migliorato, M.A. and Hue, A. and Srivastava, G.P. and Hammerschmidt, T.
MICROELECTRONICS JOURNAL. Volume: 40 (2009) - 2009 • 223
An assessment of the entire Al-Fe system including D03 ordering
Sundman, B. and Ohnuma, I. and Dupin, N. and Kattner, U.R. and Fries, S.G.
ACTA MATERIALIA. Volume: 57 (2009) - 2009 • 222
Innovative M(hfa)2·TMEDA (M=Cu, Co) precursors for the CVD of copper-cobalt oxides: An integrated theoretical and experimental approach
Gasparotto, A. and Barreca, D. and Devi, A. and Fischer, R.A. and Fois, E. and Gamba, A. and Maccato, C. and Seraglia, R. and Tabacchi, G. and Tondello, E.
ECS TRANSACTIONS. Volume: 25 (2009) - 2009 • 221
On the effect of micrograin crystallography on creep of FeCr alloys
Tak, K.-G. and Schulz, U. and Eggeler, G.
MATERIALS SCIENCE AND ENGINEERING A. Volume: 510-511 (2009) - 2009 • 220
Label-free detection of DNA hybridization in presence of intercalators using electrochemical impedance spectroscopy
Gebala, M. and Stoica, L. and Neugebauer, S. and Schuhmann, W.
ELECTROANALYSIS. Volume: 21 (2009) - 2009 • 219
Direct imaging of the structural domains in the iron pnictides A Fe2 As2 (A=Ca,Sr,Ba)
Tanatar, M.A. and Kreyssig, A. and Nandi, S. and Ni, N. and Bud'Ko, S.L. and Canfield, P.C. and Goldman, A.I. and Prozorov, R.
PHYSICAL REVIEW B - CONDENSED MATTER AND MATERIALS PHYSICS. Volume: 79 (2009) - 2009 • 218
Y-rich oxide distribution in plasma sprayed MCrAlY-coatings studied by SEM with a cathodoluminescence detector and Raman spectroscopy
Gil, A. and Naumenko, D. and Vassen, R. and Toscano, J. and Subanovic, M. and Singheiser, L. and Quadakkers, W.J.
SURFACE AND COATINGS TECHNOLOGY. Volume: 204 (2009) - 2009 • 217
Single-crystal neutron diffraction study of short-range magnetic correlations in Tb5 Ge4
Tian, W. and Kreyssig, A. and Zarestky, J.L. and Tan, L. and Nandi, S. and Goldman, A.I. and Lograsso, T.A. and Schlagel, D.L. and Gschneidner, K.A. and Pecharsky, V.K. and McQueeney, R.J.
PHYSICAL REVIEW B - CONDENSED MATTER AND MATERIALS PHYSICS. Volume: 80 (2009) - 2009 • 216
Influence of additives on the global mechanical behavior and the microscopic strain localization in wood reinforced polypropylene composites during tensile deformation investigated using digital image correlation
Godara, A. and Raabe, D. and Bergmann, I. and Putz, R. and Müller, U.
COMPOSITES SCIENCE AND TECHNOLOGY. Volume: 69 (2009) - 2009 • 215
Nanoparticle silicalite-1 crystallization from clear solutions: Nucleation
Tokay, B. and Somer, M. and Erdem-Şenatalar, A. and Schüth, F. and Thompson, R.W.
MICROPOROUS AND MESOPOROUS MATERIALS. Volume: 118 (2009) - 2009 • 214
Comment on "dipolar excitations at the LIII X-ray absorption edges of the heavy rare-earth metals"
Goldman, A.I. and Harmon, B.N. and Lee, Y.B. and Kreyssig, A.
PHYSICAL REVIEW LETTERS. Volume: 102 (2009) - 2009 • 213
Peri-implant bone organization under immediate loading conditions: Collagen fiber orientation and mineral density analyses in the minipig model
Traini, T. and Neugebauer, J. and Thams, U. and Zöller, J.E. and Caputi, S. and Piattelli, A.
CLINICAL IMPLANT DENTISTRY AND RELATED RESEARCH. Volume: 11 (2009) - 2009 • 212
Lattice collapse and quenching of magnetism in CaFe2As 2 under pressure: A single-crystal neutron and x-ray diffraction investigation
Goldman, A.I. and Kreyssig, A. and Prokeš, K. and Pratt, D.K. and Argyriou, D.N. and Lynn, J.W. and Nandi, S. and Kimber, S.A.J. and Chen, Y. and Lee, Y.B. and Samolyuk, G. and Leão, J.B. and Poulton, S.J. and Bud'ko, S.L. and Ni, N. and Canfield, P.C. and Harmon, B.N. and McQueeney, R.J.
PHYSICAL REVIEW B - CONDENSED MATTER AND MATERIALS PHYSICS. Volume: 79 (2009) - 2009 • 211
Effects of well-defined magnetic field gradients on the electrodeposition of copper and bismuth
Tschulik, K. and Koza, J.A. and Uhlemann, M. and Gebert, A. and Schultz, L.
ELECTROCHEMISTRY COMMUNICATIONS. Volume: 11 (2009) - 2009 • 210
Direct physical evidence for the back-transformation of stress-induced martensite in the vicinity of cracks in pseudoelastic NiTi shape memory alloys
Gollerthan, S. and Young, M.L. and Neuking, K. and Ramamurty, U. and Eggeler, G.
ACTA MATERIALIA. Volume: 57 (2009) - 2009 • 209
Chemistry and physical properties of the phosphide telluride Zr 2PTe2
Tschulik, K. and Ruck, M. and Binnewies, M. and Milke, E. and Hoffmann, S. and Schnelle, W. and Fokwa, B.P.T. and Gilleßen, M. and Schmidt, P.
EUROPEAN JOURNAL OF INORGANIC CHEMISTRY. Volume: (2009) - 2009 • 208
Fracture mechanics and microstructure in NiTi shape memory alloys
Gollerthan, S. and Young, M.L. and Baruj, A. and Frenzel, J. and Schmahl, W.W. and Eggeler, G.
ACTA MATERIALIA. Volume: 57 (2009) - 2009 • 207
Highly diluted copper in a silica matrix as active catalyst for propylene oxidation to acrolein
Tüysüz, H. and Llamas Galilea, J. and Schüth, F.
CATALYSIS LETTERS. Volume: 131 (2009) - 2009 • 206
Unconventional london penetration depth in single-crystal Ba(Fe0.93Co0.07)2As2 superconductors
Gordon, R.T. and Ni, N. and Martin, C. and Tanatar, M.A. and Vannette, M.D. and Kim, H. and Samolyuk, G.D. and Schmalian, J. and Nandi, S. and Kreyssig, A. and Goldman, A.I. and Yan, J.Q. and Bud'Ko, S.L. and Canfield, P.C. and Prozorov, R.
PHYSICAL REVIEW LETTERS. Volume: 102 (2009) - 2009 • 205
Interplay between long-range elastic and short-range chemical interactions in Fe-C martensite formation
Udyansky, A. and Von Pezold, J. and Bugaev, V.N. and Friák, M. and Neugebauer, J.
PHYSICAL REVIEW B - CONDENSED MATTER AND MATERIALS PHYSICS. Volume: 79 (2009) - 2009 • 204
Ab initio up to the melting point: Anharmonicity and vacancies in aluminum
Grabowski, B. and Ismer, L. and Hickel, T. and Neugebauer, J.
PHYSICAL REVIEW B - CONDENSED MATTER AND MATERIALS PHYSICS. Volume: 79 (2009) - 2009 • 203
Understanding the phase transitions of the Ni2MnGa magnetic shape memory system from first principles
Uijttewaal, M.A. and Hickel, T. and Neugebauer, J. and Gruner, M.E. and Entel, P.
PHYSICAL REVIEW LETTERS. Volume: 102 (2009) - 2009 • 202
A way to a single frequency precession of an inhomogeneous ensemble of electron spins in InGaAs quantum dots
Greilich, A. and Spatzek, S. and Efros, Al.L. and Yugova, I.A. and Yakovlev, D.R. and Reuter, D. and Wieck, A.D. and Bayer, M.
PHYSICA STATUS SOLIDI (C) CURRENT TOPICS IN SOLID STATE PHYSICS. Volume: 6 (2009) - 2009 • 201
Structural characterization and magnetic profile of annealed CoFeBMgO multilayers
Vadalá, M. and Zhernenkov, K. and Wolff, M. and Toperverg, B.P. and Westerholt, K. and Zabel, H. and Wisniowski, P. and Cardoso, S. and Freitas, P.P.
JOURNAL OF APPLIED PHYSICS. Volume: 105 (2009) - 2009 • 200
Collective single-mode precession of electron spins in an ensemble of singly charged (In,Ga)As/GaAs quantum dots
Greilich, A. and Spatzek, S. and Yugova, I.A. and Akimov, I.A. and Yakovlev, D.R. and Efros, Al.L. and Reuter, D. and Wieck, A.D. and Bayer, M.
PHYSICAL REVIEW B - CONDENSED MATTER AND MATERIALS PHYSICS. Volume: 79 (2009) - 2009 • 199
Temperature stabilized surface reconstructions at polar ZnO(0001)
Valtiner, M. and Todorova, M. and Grundmeier, G. and Neugebauer, J.
PHYSICAL REVIEW LETTERS. Volume: 103 (2009) - 2009 • 198
Dynamical changes in the Cu-ZnO x interaction observed in a model methanol synthesis catalyst
Van Den Berg, M.W.E. and Polarz, S. and Tkachenko, O.P. and Kähler, K. and Muhler, M. and Grünert, W.
CATALYSIS LETTERS. Volume: 128 (2009) - 2009 • 197
Multiscale modeling of polymers at interfaces
Varnik, F. and Binder, K.
INTERNATIONAL JOURNAL OF MATERIALS RESEARCH. Volume: 100 (2009) - 2009 • 196
Fall and rise of small droplets on rough hydrophobic substrates
Gross, M. and Varnik, F. and Raabe, D.
EUROPHYSICS LETTERS. Volume: 88 (2009) - 2009 • 195
Suspension plasma spraying: Process development and applications
Vaßen, R. and Kaßner, H. and Mauer, G. and Stöver, D.
PROCEEDINGS OF THE INTERNATIONAL THERMAL SPRAY CONFERENCE. Volume: (2009) - 2009 • 194
Elementary transformation and deformation processes and the cyclic stability of NiTi and NiTiCu shape memory spring actuators
Grossmann, Ch. and Frenzel, J. and Sampath, V. and Depka, T. and Eggeler, G.
METALLURGICAL AND MATERIALS TRANSACTIONS A: PHYSICAL METALLURGY AND MATERIALS SCIENCE. Volume: 40 (2009) - 2009 • 193
Suspension plasma spraying of TiO2 for the manufacture of photovoltaic cells
Vaßen, R. and Yi, Z. and Kaßner, H. and Stöver, D.
SURFACE AND COATINGS TECHNOLOGY. Volume: 203 (2009) - 2009 • 192
Recent developments in the field of thermal barrier coatings
Vassen, R. and Stuke, A. and Stöver, D.
JOURNAL OF THERMAL SPRAY TECHNOLOGY. Volume: 18 (2009) - 2009 • 191
Constitutive modeling of crimped collagen fibrils in soft tissues
Grytz, R. and Meschke, G.
JOURNAL OF THE MECHANICAL BEHAVIOR OF BIOMEDICAL MATERIALS. Volume: 2 (2009) - 2009 • 190
Evaluation of the crystallographic orientation relationships between FCC and BCC phases in TRIP steels
Verbeken, K. and Barbé, L. and Raabe, D.
ISIJ INTERNATIONAL. Volume: 49 (2009) - 2009 • 189
Identification of ε martensite in a Fe-based shape memory alloy by means of EBSD
Verbeken, K. and Van Caenegem, N. and Raabe, D.
MICRON. Volume: 40 (2009) - 2009 • 188
Visualization of electrocatalytic activity of microstructured metal hexacyanoferrates by means of redox competition mode of scanning electrochemical microscopy (RC-SECM)
Guadagnini, L. and Maljusch, A. and Chen, X. and Neugebauer, S. and Tonelli, D. and Schuhmann, W.
ELECTROCHIMICA ACTA. Volume: 54 (2009) - 2009 • 187
Electron-nuclear spin polarization dynamics in InGaAs quantum dots
Verbin, S.Yu. and Cherbunin, R.V. and Ignatiev, I.V. and Auer, T. and Yakovlev, D.R. and Bayer, M. and Reuter, D. and Wieck, A.D.
AIP CONFERENCE PROCEEDINGS. Volume: 1199 (2009) - 2009 • 186
Influence of the indenter tip geometry and environment on the indentation modulus of enamel
Guidoni, G.M. and He, L.H. and Schöberl, T. and Jäger, I. and Dehm, G. and Swain, M.V.
JOURNAL OF MATERIALS RESEARCH. Volume: 24 (2009) - 2009 • 185
Chirality change of chloronitrobenzene on au(111) induced by inelastic electron tunneling
Violeta, S.-M. and Meyer, J. and Morgenstern, K.
ANGEWANDTE CHEMIE - INTERNATIONAL EDITION. Volume: 48 (2009) - 2009 • 184
Bistable thin-film shape memory actuators for applications in tactile displays
Vitushinsky, R. and Schmitz, S. and Ludwig, Al.
JOURNAL OF MICROELECTROMECHANICAL SYSTEMS. Volume: 18 (2009) - 2009 • 183
Constitutive modelling of the anisotropic creep behaviour of nickel-base single crystal superalloys
Vladimirov, I.N. and Reese, S. and Eggeler, G.
INTERNATIONAL JOURNAL OF MECHANICAL SCIENCES. Volume: 51 (2009) - 2009 • 182
Electrodeposition polymers as immobilization matrices in amperometric biosensors: Improved polymer synthesis and biosensor fabrication
Guschin, D.A. and Shkil, H. and Schuhmann, W.
ANALYTICAL AND BIOANALYTICAL CHEMISTRY. Volume: 395 (2009) - 2009 • 181
Conversion of bound excitons to free excitons by surface acoustic waves
Völk, S. and Wixforth, A. and Reuter, D. and Wieck, A.D. and Ebbecke, J.
PHYSICAL REVIEW B - CONDENSED MATTER AND MATERIALS PHYSICS. Volume: 80 (2009) - 2009 • 180
Electron channeling contrast imaging of twins and dislocations in twinning-induced plasticity steels under controlled diffraction conditions in a scanning electron microscope
Gutierrez-Urrutia, I. and Zaefferer, S. and Raabe, D.
SCRIPTA MATERIALIA. Volume: 61 (2009) - 2009 • 179
Towards bose-einstein condensation of semiconductor excitons: The biexciton polarization effect
Hägele, D. and Pfalz, S. and Oestreich, M.
PHYSICAL REVIEW LETTERS. Volume: 103 (2009) - 2009 • 178
Thin film growth from a low pressure plasma excited in a supersonic expanding gas jet
Wachtendorf, C. and Herweg, C. and Daeuber, M. and Benedikt, J. and Von Keudell, A.
JOURNAL OF PHYSICS D: APPLIED PHYSICS. Volume: 42 (2009) - 2009 • 177
Atomistic modelling of materials with bond-order potentials
Hammerschmidt, T. and Drautz, R. and Pettifor, D.G.
INTERNATIONAL JOURNAL OF MATERIALS RESEARCH. Volume: 100 (2009) - 2009 • 176
Thermal expansion of LaFeAsO1-x Fx: Evidence for high-temperature fluctuations
Wang, L. and Köhler, U. and Leps, N. and Kondrat, A. and Nale, M. and Gasparini, A. and De Visser, A. and Behr, G. and Hess, C. and Klingeler, R. and Büchner, B.
PHYSICAL REVIEW B - CONDENSED MATTER AND MATERIALS PHYSICS. Volume: 80 (2009) - 2009 • 175
Experimental and ab initio structural studies of liquid Zr2 Ni
Hao, S.G. and Kramer, M.J. and Wang, C.Z. and Ho, K.M. and Nandi, S. and Kreyssig, A. and Goldman, A.I. and Wessels, V. and Sahu, K.K. and Kelton, K.F. and Hyers, R.W. and Canepari, S.M. and Rogers, J.R.
PHYSICAL REVIEW B - CONDENSED MATTER AND MATERIALS PHYSICS. Volume: 79 (2009) - 2009 • 174
A new dual-purpose ultrahigh vacuum infrared spectroscopy apparatus optimized for grazing-incidence reflection as well as for transmission geometries
Wang, Y. and Glenz, A. and Muhler, M. and Wöll, Ch.
REVIEW OF SCIENTIFIC INSTRUMENTS. Volume: 80 (2009) - 2009 • 173
Diffusion in diamond. II. High-pressure-temperature experiments
Harte, B. and Taniguchi, T. and Chakraborty, S.
MINERALOGICAL MAGAZINE. Volume: 73 (2009) - 2009 • 172
Shape and size controlled α-Fe2O3 nanoparticles as supports for gold-catalysts: Synthesis and influence of support shape and size on catalytic performance
Wang, G.-H. and Li, W.-C. and Jia, K.-M. and Spliethoff, B. and Schüth, F. and Lu, A.-H.
APPLIED CATALYSIS A: GENERAL. Volume: 364 (2009) - 2009 • 171
Enhanced cell adhesion to silicone implant material through plasma surface modification
Hauser, J. and Zietlow, J. and Köller, M. and Esenwein, S.A. and Halfmann, H. and Awakowicz, P. and Steinau, H.U.
JOURNAL OF MATERIALS SCIENCE: MATERIALS IN MEDICINE. Volume: 20 (2009) - 2009 • 170
Quantitative simulations of microstructure evolution in single crystal superalloys during solution heat treatment
Warnken, N. and Ma, D. and Drevermann, A. and Steinbach, I. and Fries, S.G.
INTERNATIONAL HEAT TREATMENT AND SURFACE ENGINEERING. Volume: 3 (2009) - 2009 • 169
Surface modification of metal implant materials by low-pressure plasma treatment
Hauser, J. and Krüger, C.D. and Halfmann, H. and Awakowicz, P. and Köller, M. and Esenwein, S.A.
BIOMEDIZINISCHE TECHNIK. Volume: 54 (2009) - 2009 • 168
Phase-field modelling of as-cast microstructure evolution in nickel-based superalloys
Warnken, N. and Ma, D. and Drevermann, A. and Reed, R.C. and Fries, S.G. and Steinbach, I.
ACTA MATERIALIA. Volume: 57 (2009) - 2009 • 167
Crystal structure of catena-(tris(μ-betaine-O,O′)manganese(II)) tetrabromomanganate, [Mn(C5H11NO2)3] [MnBr4]
Haussiihl, E. and Wiehl, L. and Schreuer, J.
ZEITSCHRIFT FUR KRISTALLOGRAPHIE - NEW CRYSTAL STRUCTURES. Volume: 224 (2009) - 2009 • 166
Crystal structure of trisbetaine manganese(II) dibromide dihydrate, (C 5H11NO2)3 · MnBr2 · 2H2O
Haussühl, E. and Wiehl, L. and Schreuer, J.
ZEITSCHRIFT FUR KRISTALLOGRAPHIE - NEW CRYSTAL STRUCTURES. Volume: 224 (2009) - 2009 • 165
Complex rare-earth aluminum hydrides: Mechanochemical preparation, crystal structure and potential for hydrogen storage
Weidenthaler, C. and Pommerin, A. and Felderhoff, M. and Sun, W. and Wolverton, C. and Bogdanović, B. and Schüth, F.
JOURNAL OF THE AMERICAN CHEMICAL SOCIETY. Volume: 131 (2009) - 2009 • 164
Microstructural aspects of crack nucleation during cyclic loading of AA7075-T651
Weiland, H. and Nardiello, J. and Zaefferer, S. and Cheong, S. and Papazian, J. and Raabe, D.
ENGINEERING FRACTURE MECHANICS. Volume: 76 (2009) - 2009 • 163
Metallic materials for structural applications beyond nickel-based superalloys
Heilmaier, M. and Krüger, M. and Saage, H. and Rösler, J. and Mukherji, D. and Glatzel, U. and Völkl, R. and Hüttner, R. and Eggeler, G. and Somsen, C. and Depka, T. and Christ, H.-J. and Gorr, B. and Burk, S.
JOM. Volume: 61 (2009) - 2009 • 162
Low-temperature ballistic transport in nanoscale epitaxial graphene cross junctions
Weingart, S. and Bock, C. and Kunze, U. and Speck, F. and Seyller, Th. and Ley, L.
APPLIED PHYSICS LETTERS. Volume: 95 (2009) - 2009 • 161
Microstructural evolution during multiaxial deformation of pseudoelastic NiTi studied by first-principles-based micromechanical modeling
Heinen, R. and Hackl, K. and Windl, W. and Wagner, M.F.-X.
ACTA MATERIALIA. Volume: 57 (2009) - 2009 • 160
Inversion-asymmetry-induced spin splitting observed in the quantum oscillatory magnetization of a two-dimensional electron system
Wilde, M.A. and Reuter, D. and Heyn, Ch. and Wieck, A.D. and Grundler, D.
PHYSICAL REVIEW B - CONDENSED MATTER AND MATERIALS PHYSICS. Volume: 79 (2009) - 2009 • 159
MOCVD of gallium oxide thin films using homoleptic gallium complexes: Precursor evaluation and thin film characterisation
Hellwig, M. and Xu, K. and Barreca, D. and Gasparotto, A. and Niermann, B. and Winter, J. and Becker, H.W. and Rogalla, D. and Fischer, R.A. and Devi, A.
ECS TRANSACTIONS. Volume: 25 (2009) - 2009 • 158
Novel gallium complexes with malonic diester anions as molecular precursors for the MOCVD of ga203 thin films
Hellwig, M. and Ke, X. and Barreca, D. and Gasparotto, A. and Winter, M. and Tondello, E. and Fischer, R.A. and Devi, A.
EUROPEAN JOURNAL OF INORGANIC CHEMISTRY. Volume: (2009) - 2009 • 157
Thermodynamics and kinetics of the adsorption of carbon monoxide on supported gold catalysts probed by static adsorption microcalorimetry: The role of the support
Xia, X. and Strunk, J. and Busser, W. and Comotti, M. and Schüth, F. and Muhler, M.
JOURNAL OF PHYSICAL CHEMISTRY C. Volume: 113 (2009) - 2009 • 156
On TGO creep and the initiation of a class of fatigue cracks in thermal barrier coatings
Hernandez, M.T. and Karlsson, A.M. and Bartsch, M.
SURFACE AND COATINGS TECHNOLOGY. Volume: 203 (2009) - 2009 • 155
A highly efficient gas-phase route for the oxygen functionalization of carbon nanotubes based on nitric acid vapor
Xia, W. and Jin, C. and Kundu, S. and Muhler, M.
CARBON. Volume: 47 (2009) - 2009 • 154
Steel design from fully parameter-free ab initio computer simulations
Hickel, T. and Dick, A. and Grabowski, B. and Körmann, F. and Neugebauer, J.
STEEL RESEARCH INTERNATIONAL. Volume: 80 (2009) - 2009 • 153
Iron impregnation on the amorphous shell of vapor grown carbon fibers and the catalytic growth of secondary nanofibers
Xia, W. and Bitter, J.H. and Su, D. and Qian, J. and Muhler, M.
DIAMOND AND RELATED MATERIALS. Volume: 18 (2009) - 2009 • 152
Laser processing in solar cell production
Xiao, S. and Ostendorf, A.
ZHONGGUO JIGUANG/CHINESE JOURNAL OF LASERS. Volume: 36 (2009) - 2009 • 151
Spin-controlled optoelectronic devices
Hövel, S. and Gerhardt, N.C. and Hofmann, M.R. and Lo, F.-Y. and Reuter, D. and Wieck, A.D. and Schuster, E. and Wende, H. and Keune, W.
PHYSICA STATUS SOLIDI (C) CURRENT TOPICS IN SOLID STATE PHYSICS. Volume: 6 (2009) - 2009 • 150
Tuning the thermal properties of hafnium precursors by tailoring the ligands
Xu, K. and Milanov, A.P. and Devi, A.
ECS TRANSACTIONS. Volume: 25 (2009) - 2009 • 149
Coherent spin dynamics in nanopatterned ferromagnet-semiconductor hybrids at room-temperature
Hohage, P.E. and Nannen, J. and Wahle, M. and Fischer, S.F. and Kunze, U. and Reuter, D. and Wieck, A.D. and Bacher, G.
AIP CONFERENCE PROCEEDINGS. Volume: 1199 (2009) - 2009 • 148
On stresses induced in a thermal barrier coating due to indentation testing
Yan, J. and Karlsson, A.M. and Bartsch, M. and Chen, X.
COMPUTATIONAL MATERIALS SCIENCE. Volume: 44 (2009) - 2009 • 147
Fe-Mg interdiffusion in wadsleyite: The role of pressure, temperature and composition and the magnitude of jump in diffusion rates at the 410 km discontinuity
Holzapfel, C. and Chakraborty, S. and Rubie, D.C. and Frost, D.J.
PHYSICS OF THE EARTH AND PLANETARY INTERIORS. Volume: 172 (2009) - 2009 • 146
Flux growth at ambient pressure of millimeter-sized single crystals of LaFeAsO, LaFeAsO1-x Fx, and LaFe1-x Co x AsO
Yan, J.-Q. and Nandi, S. and Zarestky, J.L. and Tian, W. and Kreyssig, A. and Jensen, B. and Kracher, A. and Dennis, K.W. and McQueeney, R.J. and Goldman, A.I. and McCallum, R.W. and Lograsso, T.A.
APPLIED PHYSICS LETTERS. Volume: 95 (2009) - 2009 • 145
Hard repulsive barrier in hot adatom motion during dissociative adsorption of oxygen on Ag(100)
Hsieh, M.-F. and Lin, D.-S. and Gawronski, H. and Morgenstern, K.
JOURNAL OF CHEMICAL PHYSICS. Volume: 131 (2009) - 2009 • 144
Stress-controlled fatigue behaviour of micro-sized polycrystalline copper wires
Yang, B. and Motz, C. and Grosinger, W. and Dehm, G.
MATERIALS SCIENCE AND ENGINEERING A. Volume: 515 (2009) - 2009 • 143
Controlled normal/shear loading and shear fracture in bulk metallic glasses
Hsueh, C.H. and Bei, H. and Liu, C.T. and George, E.P. and Becher, P.F.
INTERMETALLICS. Volume: 17 (2009) - 2009 • 142
FMR studies of half metallic ferromagnetic thin films Co 2MnSn and Co 2MnGe
Yilgin, R. and Kazan, S. and Rameev, B. and Aktas, B. and Westerholt, K.
JOURNAL OF PHYSICS: CONFERENCE SERIES. Volume: 153 (2009) - 2009 • 141
Temperature-dependent electron Landé g factor and the interband matrix element of GaAs
Hübner, J. and Döhrmann, S. and Hägele, D. and Oestreich, M.
PHYSICAL REVIEW B - CONDENSED MATTER AND MATERIALS PHYSICS. Volume: 79 (2009) - 2009 • 140
Long-term hole spin memory in the resonantly amplified spin coherence of InGaAs/GaAs quantum well electrons
Yugova, I.A. and Sokolova, A.A. and Yakovlev, D.R. and Greilich, A. and Reuter, D. and Wieck, A.D. and Bayer, M.
PHYSICAL REVIEW LETTERS. Volume: 102 (2009) - 2009 • 139
Modeling of dislocation patterns of small- and high-angle grain boundaries in aluminum
Zahn, D. and Tlatlik, H. and Raabe, D.
COMPUTATIONAL MATERIALS SCIENCE. Volume: 46 (2009) - 2009 • 138
R-phase formation in Ti39Ni45Cu16 shape memory thin films and bulk alloys discovered by combinatorial methods
Zarnetta, R. and König, D. and Zamponi, C. and Aghajani, A. and Frenzel, J. and Eggeler, G. and Ludwig, Al.
ACTA MATERIALIA. Volume: 57 (2009) - 2009 • 137
Influence of precipitates on the thermal hysteresis of Ti-Ni-Pd shape memory thin films
Zarnetta, R. and Zelaya, E. and Eggeler, G. and Ludwig, Al.
SCRIPTA MATERIALIA. Volume: 60 (2009) - 2009 • 136
Specific features of spin, charge, and orbital ordering in cobaltites
Ivanova, N.B. and Ovchinnikov, S.G. and Korshunov, M.M. and Eremin, I.M. and Kazak, N.V.
PHYSICS-USPEKHI. Volume: 52 (2009) - 2009 • 135
Reagentless enzymatic sensors based on carbon-paste electrodes containing ruthenium mediators for the on-line determination of glycerol
Ivanova, E.V. and Schuhmann, W. and Ryabov, A.D.
JOURNAL OF ANALYTICAL CHEMISTRY. Volume: 64 (2009) - 2009 • 134
Generalized fabrication of nanoporous metals (Au, Pd, Pt, Ag, and Cu) through chemical dealloying
Zhang, Z. and Wang, Y. and Qi, Z. and Zhang, W. and Qin, J. and Frenzel, J.
JOURNAL OF PHYSICAL CHEMISTRY C. Volume: 113 (2009) - 2009 • 133
Interstitial impurities at grain boundaries in metals: insight from atomistic calculations
Janisch, R. and Elsässer, C.
INTERNATIONAL JOURNAL OF MATERIALS RESEARCH. Volume: 100 (2009) - 2009 • 132
Design of amphiphilic ABC triblock copolymer for templating synthesis of large-pore ordered mesoporous carbons with tunable pore wall thickness
Zhang, J. and Deng, Y. and Wei, J. and Sun, Z. and Gu, D. and Bongard, H. and Liu, C. and Wu, H. and Tu, B. and Schüth, F. and Zhao, D.
CHEMISTRY OF MATERIALS. Volume: 21 (2009) - 2009 • 131
Atmospheric plasma spraying of high melting temperature complex perovskites for TBC application
Jarligo, M.O. and Mack, D.E. and Mauer, G. and Vaßen, R. and Stöver, D.
PROCEEDINGS OF THE INTERNATIONAL THERMAL SPRAY CONFERENCE. Volume: (2009) - 2009 • 130
Fabrication and characterization of nanoporous gold composites through chemical dealloying of two phase Al-Au alloys
Zhang, Z. and Wang, Y. and Qi, Z. and Somsen, C. and Wang, X. and Zhao, C.
JOURNAL OF MATERIALS CHEMISTRY. Volume: 19 (2009) - 2009 • 129
Application of plasma-sprayed complex perovskites as thermal barrier coatings
Jarligo, M.O. and Mack, D.E. and Vassen, R. and Stöver, D.
JOURNAL OF THERMAL SPRAY TECHNOLOGY. Volume: 18 (2009) - 2009 • 128
Nanoindentation of Ti50Ni48Fe2 and Ti 50Ni40Cu10 shape memory alloys
Zheng, H. and Pfetzing, J. and Frenzel, J. and Eggeler, G.
INTERNATIONAL JOURNAL OF MATERIALS RESEARCH. Volume: 100 (2009) - 2009 • 127
Vapor phase synthesis of Pt nanoparticles on carbon nanotube-active carbon hierarchical composites
Jin, C. and Holz, M. and Xia, W. and Mei, B. and Kundu, S. and Muhler, M.
ECS TRANSACTIONS. Volume: 25 (2009) - 2009 • 126
Pair vs many-body potentials: Influence on elastic and plastic behavior in nanoindentation of fcc metals
Ziegenhain, G. and Hartmaier, A. and Urbassek, H.M.
JOURNAL OF THE MECHANICS AND PHYSICS OF SOLIDS. Volume: 57 (2009) - 2009 • 125
On the role of the thermal treatment of sulfided Rh/CNT catalysts applied in the oxygen reduction reaction
Jin, C. and Xia, W. and Nagaiah, T.C. and Guo, J. and Chen, X. and Bron, M. and Schuhmann, W. and Muhler, M.
ELECTROCHIMICA ACTA. Volume: 54 (2009) - 2009 • 124
UV LED Photo Electron Ionisation for MS and IMS
Zimmer, C. and Medyanyk, K. and Schönhense, G. and Schubert, J. and Kunze, U. and Doll, T.
PROCEDIA CHEMISTRY. Volume: 1 (2009) - 2009 • 123
On the numerical simulation of material inhomogeneities due to martensitic phase transformations in poly-crystals
Junker, P. and Hackl, K.
ESOMAT 2009 - 8TH EUROPEAN SYMPOSIUM ON MARTENSITIC TRANSFORMATIONS. Volume: (2009) - 2009 • 122
Thermal barrier coating systems - analysis of nanoindentation curves
Zotov, N. and Bartsch, M. and Eggeler, G.
SURFACE AND COATINGS TECHNOLOGY. Volume: 203 (2009) - 2009 • 121
Transient terahertz spectroscopy of excitons and unbound carriers in quasi-two-dimensional electron-hole gases
Kaindl, R.A. and Hägele, D. and Carnahan, M.A. and Chemla, D.S.
PHYSICAL REVIEW B - CONDENSED MATTER AND MATERIALS PHYSICS. Volume: 79 (2009) - 2009 • 120
On the precipitation mechanism and the role of the post-precipitation steps during the synthesis of binary ZnO-Al2O3 composites with high specific surface area
Kaluza, S. and Muhler, M.
JOURNAL OF MATERIALS CHEMISTRY. Volume: 19 (2009) - 2009 • 119
On the role of aging, washing, and drying in the synthesis of polycrystalline zinc oxide by precipitation: Combining fast continuous mixing, spray drying and freeze drying to unravel the solid-state transformations of the precipitate
Kaluza, S. and Muhler, M.
CATALYSIS LETTERS. Volume: 129 (2009) - 2009 • 118
Microstructure and thermal cycling behavior of atmospheric plasma sprayed thermal barrier coatings with high segmentation crack densities
Karger, M. and Vaßen, R. and Stöver, D.
PROCEEDINGS OF THE INTERNATIONAL THERMAL SPRAY CONFERENCE. Volume: (2009) - 2009 • 117
Three-dimensional bone imaging: Optical coherence tomography versus micro computer tomography
Kasseck, C. and Kratz, M. and Torcasio, A. and Gerhardt, N.C. and Van Lenthe, G.H. and Gambichler, T. and Hoffmann, K. and Jones, D.B. and Hofmann, M.R.
PROGRESS IN BIOMEDICAL OPTICS AND IMAGING - PROCEEDINGS OF SPIE. Volume: 7372 (2009) - 2009 • 116
Multivariant formulation of the thermomechanically coupled Müller-Achenbach-Seelecke-model for shape memory alloys
Kastner, O. and Richter, F. and Eggeler, G.
PROCEEDINGS OF THE ASME CONFERENCE ON SMART MATERIALS, ADAPTIVE STRUCTURES AND INTELLIGENT SYSTEMS 2009, SMASIS2009. Volume: 1 (2009) - 2009 • 115
Numerical determination of heat distribution and castability simulations of as cast Mg-Al alloys
Khan, S.S. and Hort, N. and Eiken, J. and Steinbach, I. and Schmauder, S.
ADVANCED ENGINEERING MATERIALS. Volume: 11 (2009) - 2009 • 114
Overview on established and novel FIB based miniaturized mechanical testing using in-situ SEM
Kiener, D. and Motz, C. and Dehm, G. and Pippan, R.
INTERNATIONAL JOURNAL OF MATERIALS RESEARCH. Volume: 100 (2009) - 2009 • 113
Micro-compression testing: A critical discussion of experimental constraints
Kiener, D. and Motz, C. and Dehm, G.
MATERIALS SCIENCE AND ENGINEERING A. Volume: 505 (2009) - 2009 • 112
On the importance of sample compliance in uniaxial microtesting
Kiener, D. and Grosinger, W. and Dehm, G.
SCRIPTA MATERIALIA. Volume: 60 (2009) - 2009 • 111
Similarities between structural distortions underpressure and chemical doping in superconducting BaFe 2 As 2
Kimber, S.A.J. and Kreyssig, A. and Zhang, Y.-Z. and Jeschke, H.O. and Valentí, R. and Yokaichiya, F. and Colombier, E. and Yan, J. and Hansen, T.C. and Chatterji, T. and Mcqueeney, R.J. and Canfield, P.C. and Goldman, A.I. and Argyriou, D.N.
NATURE MATERIALS. Volume: 8 (2009) - 2009 • 110
Multifunctional FeCo/TiN multilayer thin films with combined magnetic and protective properties
Klever, C. and Stüber, M. and Leiste, H. and Nold, E. and Seemann, K. and Ulrich, S. and Brunken, H. and Ludwig, Al. and Thede, C. and Quandt, E.
ADVANCED ENGINEERING MATERIALS. Volume: 11 (2009) - 2009 • 109
Field dependence of the thermopower of CeNiSn
Köhler, U. and Sun, P. and Oeschler, N. and Takabatake, T. and Paschen, S. and Steglich, F.
JOURNAL OF PHYSICS: CONFERENCE SERIES. Volume: 150 (2009) - 2009 • 108
Pressure dependence of the Curie temperature in bcc iron studied by ab initio simulations
Körmann, F. and Dick, A. and Hickel, T. and Neugebauer, J.
PHYSICAL REVIEW B - CONDENSED MATTER AND MATERIALS PHYSICS. Volume: 79 (2009) - 2009 • 107
Nonanalytic spin susceptibility of a fermi liquid: The case of Fe-based pnictides
Korshunov, M.M. and Eremin, I. and Efremov, D.V. and Maslov, D.L. and Chubukov, A.V.
PHYSICAL REVIEW LETTERS. Volume: 102 (2009) - 2009 • 106
Microstructure of friction stir welding of aluminium alloy to magnesium alloy
Kostka, A. and Coelho, R.S. and dos Santos, J. and Pyzalla, A.R.
SCRIPTA MATERIALIA. Volume: 60 (2009) - 2009 • 105
Single-shot holography for depth resolved three dimensional imaging
Koukourakis, N. and Kasseck, C. and Rytz, D. and Gerhardt, N.C. and Hofmann, M.R.
OPTICS EXPRESS. Volume: 17 (2009) - 2009 • 104
Depth resolved holographic imaging with variable depth resolution using spectrally tunable diode laser
Koukourakis, N. and Breede, M. and Gerhardt, N.C. and Hofmann, M. and Köber, S. and Salvador, M. and Meerholz, K.
ELECTRONICS LETTERS. Volume: 45 (2009) - 2009 • 103
An integrated atmospheric microwave plasma source
Kovacs, R. and Bibinov, N. and Awakowicz, P. and Porteanu, H.-E. and Kühn, S. and Gesche, R.
PLASMA PROCESSES AND POLYMERS. Volume: 6 (2009) - 2009 • 102
Virtual material testing for stamping simulations based on polycrystal plasticity
Kraska, M. and Doig, M. and Tikhomirov, D. and Raabe, D. and Roters, F.
COMPUTATIONAL MATERIALS SCIENCE. Volume: 46 (2009) - 2009 • 101
Atomic processes in molecular beam epitaxy on strained InAs(137): A density-functional theory study
Kratzer, P. and Hammerschmidt, T.
PHYSICAL REVIEW B - CONDENSED MATTER AND MATERIALS PHYSICS. Volume: 80 (2009) - 2009 • 100
Ordered mesoporous carbide derived carbons: Novel materials for catalysis and adsorption
Krawiec, P. and Kockrick, E. and Borchardt, L. and Geiger, D. and Corma, A. and Kaskel, S.
JOURNAL OF PHYSICAL CHEMISTRY C. Volume: 113 (2009) - 2009 • 99
Probing fractal magnetic domains on multiple length scales in Nd2Fe14B
Kreyssig, A. and Prozorov, R. and Dewhurst, C.D. and Canfield, P.C. and McCallum, R.W. and Goldman, A.I.
PHYSICAL REVIEW LETTERS. Volume: 102 (2009) - 2009 • 98
Large scale morphology of ZnO(1 0over(1, ̄) 0)-surfaces
Kroll, M. and Kuschel, T. and Löber, T. and Köhler, U.
SURFACE SCIENCE. Volume: 603 (2009) - 2009 • 97
Shear stress in lattice Boltzmann simulations
Krüger, T. and Varnik, F. and Raabe, D.
PHYSICAL REVIEW E - STATISTICAL, NONLINEAR, AND SOFT MATTER PHYSICS. Volume: 79 (2009) - 2009 • 96
Characterization of DBD plasma source for biomedical applications
Kuchenbecker, M. and Bibinov, N. and Kaemlimg, A. and Wandke, D. and Awakowicz, P. and Viöl, W.
JOURNAL OF PHYSICS D: APPLIED PHYSICS. Volume: 42 (2009) - 2009 • 95
Electrocatalytic activity and stability of nitrogen-containing carbon nanotubes in the oxygen reduction reaction
Kundu, S. and Nagaiah, T.C. and Xia, W. and Wang, Y. and Van Dommele, S. and Bitter, J.H. and Santa, M. and Grundmeier, G. and Bron, M. and Schuhmann, W. and Muhler, M.
JOURNAL OF PHYSICAL CHEMISTRY C. Volume: 113 (2009) - 2009 • 94
Laser operation of the III/V compound material Ga(NAsP) grown lattice matched on (001) Si substrate
Kunert, B. and Liebich, S. and Zinnkann, S. and Neméth, I. and Fritz, R. and Volz, K. and Stolz, W. and Lange, C. and Koester, N.S. and Franzbach, D.J. and Chatterjee, S. and Ruhle, W.W. and Gerhardt, N.C. and Koukourakis, N. and Hofmann, M.
DEVICE RESEARCH CONFERENCE - CONFERENCE DIGEST, DRC. Volume: (2009) - 2009 • 93
Lasing of the III/V compound semiconductor Ga(NAsP) integrated lattice-matched to Si substrate
Kunert, B. and Liebich, S. and Zimprich, M. and Zinnkann, S. and Fritz, R. and Volz, K. and Stolz, W. and Lange, C. and Köster, N.S. and Chatterjee, S. and Rühle, W.W. and Gerhardt, N.C. and Koukourakis, N. and Hofmann, M.
IEEE INTERNATIONAL CONFERENCE ON GROUP IV PHOTONICS GFP. Volume: (2009) - 2009 • 92
Carrier relaxation dynamics in self-assembled semiconductor quantum dots
Kurtze, H. and Seebeck, J. and Gartner, P. and Yakovlev, D.R. and Reuter, D. and Wieck, A.D. and Bayer, M. and Jahnke, F.
PHYSICAL REVIEW B - CONDENSED MATTER AND MATERIALS PHYSICS. Volume: 80 (2009) - 2009 • 91
Removal of model proteins using beams of argon ions, oxygen atoms and molecules: Mimicking the action of low-pressure Ar/O2 ICP discharges
Kylián, O. and Benedikt, J. and Sirghi, L. and Reuter, R. and Rauscher, H. and Von Keudell, A. and Rossi, F.
PLASMA PROCESSES AND POLYMERS. Volume: 6 (2009) - 2009 • 90
Gain characteristics and lasing of Ga(NAsP) multi-quantum well structures
Lange, C. and Chatterjee, S. and Kunert, B. and Volz, K. and Stolz, W. and Rühle, W.W. and Gerhardt, N.C. and Hofmann, M.R.
PHYSICA STATUS SOLIDI (C) CURRENT TOPICS IN SOLID STATE PHYSICS. Volume: 6 (2009) - 2009 • 89
Imaging biocatalytic activity of enzyme-polymer spots by means of combined scanning electrochemical microscopy/electrogenerated chemiluminescence
Lei, R. and Stratmann, L. and Schäfer, D. and Erichsen, T. and Neugebauer, S. and Li, N. and Schuhmann, W.
ANALYTICAL CHEMISTRY. Volume: 81 (2009) - 2009 • 88
Electron energy structure of self-assembled In(Ga)As nanostructures probed by capacitance-voltage spectroscopy and one-dimensional numerical simulation
Lei, W. and Notthoff, C. and Offer, M. and Meier, C. and Lorke, A. and Jagadish, C. and Wieck, A.D.
JOURNAL OF MATERIALS RESEARCH. Volume: 24 (2009) - 2009 • 87
Detection of covalent and noncovalent intermediates in the polymerization reaction catalyzed by a C149S class III polyhydroxybutyrate synthase
Li, P. and Chakraborty, S. and Stubbe, J.
BIOCHEMISTRY. Volume: 48 (2009) - 2009 • 86
High porosity and high-strength porous NiTi shape memory alloys with controllable pore characteristics
Li, D.S. and Zhang, Y.P. and Eggeler, G. and Zhang, X.P.
JOURNAL OF ALLOYS AND COMPOUNDS. Volume: 470 (2009) - 2009 • 85
Magnetic and lattice coupling in single-crystal SrFe2 As2: A neutron scattering study
Li, H. and Tian, W. and Zarestky, J.L. and Kreyssig, A. and Ni, N. and Bud'Ko, S.L. and Canfield, P.C. and Goldman, A.I. and McQueeney, R.J. and Vaknin, D.
PHYSICAL REVIEW B - CONDENSED MATTER AND MATERIALS PHYSICS. Volume: 80 (2009) - 2009 • 84
Synthesis and catalytic performance of pd nanoparticle/functionalized cnf composites by a two-step chemical vapor deposition of pd(allyl)(Cp) Precursor
Liang, C. and Xia, W. and Van Den Berg, M. and Wang, Y. and Soltani-Ahmadi, H. and Schlüter, O. and Fischer, R.A. and Muhler, M.
CHEMISTRY OF MATERIALS. Volume: 21 (2009) - 2009 • 83
Enhancing nitrogen solubility in GaAs and InAs by surface kinetics: An ab initio study
Abu-Farsakh, H. and Neugebauer, J.
PHYSICAL REVIEW B - CONDENSED MATTER AND MATERIALS PHYSICS. Volume: 79 (2009) - 2009 • 82
Effect of the titania morphology on the Au/TiO2-catalyzed aerobic epoxidation of stilbene
Lignier, P. and Comotti, M. and Schüth, F. and Rousset, J.-L. and Caps, V.
CATALYSIS TODAY. Volume: 141 (2009) - 2009 • 81
The electron attachment energy of the aqueous hydroxyl radical predicted from the detachment energy of the aqueous hydroxide anion
Adriaanse, C. and Sulpizi, M. and VandeVondele, J. and Sprik, M.
JOURNAL OF THE AMERICAN CHEMICAL SOCIETY. Volume: 131 (2009) - 2009 • 80
Wide band gap semiconductor nanostructures for optoelectronic applications
Lischka, K. and Waag, A. and Mariette, H. and Neugebauer, J.
MICROELECTRONICS JOURNAL. Volume: 40 (2009) - 2009 • 79
On the formation and growth of Mo-rich Laves phase particles during long-term creep of a 12% chromium tempered martensite ferritic steel
Aghajani, A. and Richter, F. and Somsen, C. and Fries, S.G. and Steinbach, I. and Eggeler, G.
SCRIPTA MATERIALIA. Volume: 61 (2009) - 2009 • 78
Microtexture and grain boundaries in freestanding CVD diamond films: Growth and twinning mechanisms
Liu, T. and Raabe, D. and Mao, W. and Zaefferer, S.
ADVANCED FUNCTIONAL MATERIALS. Volume: 19 (2009) - 2009 • 77
On the effect of long-term creep on the microstructure of a 12% chromium tempered martensite ferritic steel
Aghajani, A. and Somsen, C. and Eggeler, G.
ACTA MATERIALIA. Volume: 57 (2009) - 2009 • 76
Residual stress analysis in chemical-vapor-deposition diamond films
Liu, T. and Pinto, H. and Brito, P. and Sales, L.A. and Raabe, D.
APPLIED PHYSICS LETTERS. Volume: 94 (2009) - 2009 • 75
Microstructural evolution in T24, a modified 2(1/4)Cr-1Mo steel during creep after different heat treatments
Aghajani, A. and Somsen, C. and Pesicka, J. and Bendick, W. and Hahn, B. and Eggeler, G.
MATERIALS SCIENCE AND ENGINEERING A. Volume: 510-511 (2009) - 2009 • 74
Comparison of single- and multiobjective design of experiment in combinatorial chemistry for the selective dehydrogenation of propane
Llamas-Galilea, J. and Gobin, O.C. and Schüth, F.
JOURNAL OF COMBINATORIAL CHEMISTRY. Volume: 11 (2009) - 2009 • 73
Theory of the bound state of 4f excitations and magnetic resonance in unconventional superconductors
Akbari, A. and Eremin, I. and Thalmeier, P. and Fulde, P.
PHYSICAL REVIEW B - CONDENSED MATTER AND MATERIALS PHYSICS. Volume: 80 (2009) - 2009 • 72
High-temperature ferromagnetism in Co-implanted TiO2 rutile
Akdogan, N. and Nefedov, A. and Zabel, H. and Westerholt, K. and Becker, H.-W. and Somsen, C. and Gök, A. and Bashir, A. and Khaibullin, R. and Tagirov, L.
JOURNAL OF PHYSICS D: APPLIED PHYSICS. Volume: 42 (2009) - 2009 • 71
Dose dependence of ferromagnetism in Co-implanted ZnO
Akdogan, N. and Zabel, H. and Nefedov, A. and Westerholt, K. and Becker, H.-W. and Gök, S. and Khaibullin, R. and Tagirov, L.
JOURNAL OF APPLIED PHYSICS. Volume: 105 (2009) - 2009 • 70
Nanostructured zinc oxide films synthesized by successive chemical solution deposition for gas sensor applications
Lupan, O. and Chow, L. and Shishiyanu, S. and Monaico, E. and Shishiyanu, T. and Şontea, V. and Roldan Cuenya, B. and Naitabdi, A. and Park, S. and Schulte, A.
MATERIALS RESEARCH BULLETIN. Volume: 44 (2009) - 2009 • 69
Theory of dynamic spin susceptibility in terms of the t-J-V model: Comparison with neutron scattering data for Pr0.88LaCe 0.12CuO4 - X and La2 - X Sr x CuO4
Andreev, A.I. and Eremin, I.M. and Eremin, M.V.
JOURNAL OF EXPERIMENTAL AND THEORETICAL PHYSICS. Volume: 108 (2009) - 2009 • 68
Atomistic calculations on interfaces: Bridging the length and time scales : Al review
Lymperakis, L. and Friák, M. and Neugebauer, J.
EUROPEAN PHYSICAL JOURNAL: SPECIAL TOPICS. Volume: 177 (2009) - 2009 • 67
Grain growth simulations including particle pinning using the multiphase-field concept
Apel, M. and Böttger, B. and Rudnizki, J. and Schaffnit, P. and Steinbach, I.
ISIJ INTERNATIONAL. Volume: 49 (2009) - 2009 • 66
Large anisotropic adatom kinetics on nonpolar GaN surfaces: Consequences for surface morphologies and nanowire growth
Lymperakis, L. and Neugebauer, J.
PHYSICAL REVIEW B - CONDENSED MATTER AND MATERIALS PHYSICS. Volume: 79 (2009) - 2009 • 65
Virtual dilatometer curves and effective Young's modulus of a 3D multiphase structure calculated by the phase-field method
Apel, M. and Benke, S. and Steinbach, I.
COMPUTATIONAL MATERIALS SCIENCE. Volume: 45 (2009) - 2009 • 64
Dendritic Solidification in the Diffuse Regime and under the Influence of Buoyancy-Driven Melt Convection
Apel, M. and Steinbach, I.
PHASE TRANSFORMATIONS IN MULTICOMPONENT MELTS. Volume: (2009) - 2009 • 63
Smaller is stronger: The effect of strain hardening
Maaß, R. and Van Petegem, S. and Ma, D. and Zimmermann, J. and Grolimund, D. and Roters, F. and Van Swygenhoven, H. and Raabe, D.
ACTA MATERIALIA. Volume: 57 (2009) - 2009 • 62
Microstructural evolution during deformation of tin dioxide nanoparticles in a comminution process
Armstrong, P. and Knieke, C. and Mackovic, M. and Frank, G. and Hartmaier, A. and Göken, M. and Peukert, W.
ACTA MATERIALIA. Volume: 57 (2009) - 2009 • 61
Thermally oxidized Mn-Co thin films as protective coatings for SOFC interconnects
Mardare, C.C. and Spiegel, M. and Savan, A. and Ludwig, Al.
JOURNAL OF THE ELECTROCHEMICAL SOCIETY. Volume: 156 (2009) - 2009 • 60
CO ppb sensors based on monodispersed SnOx:Pd mixed nanoparticle layers: Insight into dual conductance response
Aruna, I. and Kruis, F.E. and Kundu, S. and Muhler, M. and Theissmann, R. and Spasova, M.
JOURNAL OF APPLIED PHYSICS. Volume: 105 (2009) - 2009 • 59
High-throughput synthesis and characterization of anodic oxides on Nb-Ti alloys
Mardare, A.I. and Savan, A. and Ludwig, Al. and Wieck, A.D. and Hassel, A.W.
ELECTROCHIMICA ACTA. Volume: 54 (2009) - 2009 • 58
High-throughput study of the anodic oxidation of Hf-Ti thin films
Mardare, A.I. and Ludwig, Al. and Savan, A. and Wieck, A.D. and Hassel, A.W.
ELECTROCHIMICA ACTA. Volume: 54 (2009) - 2009 • 57
Measurement of the Knight field and local nuclear dipole-dipole field in an InGaAs/GaAs quantum dot ensemble
Auer, T. and Oulton, R. and Bauschulte, A. and Yakovlev, D.R. and Bayer, M. and Verbin, S.Yu. and Cherbunin, R.V. and Reuter, D. and Wieck, A.D.
PHYSICAL REVIEW B - CONDENSED MATTER AND MATERIALS PHYSICS. Volume: 80 (2009) - 2009 • 56
A combinatorial passivation study of Ta-Ti alloys
Mardare, A.I. and Savan, A. and Ludwig, Al. and Wieck, A.D. and Hassel, A.W.
CORROSION SCIENCE. Volume: 51 (2009) - 2009 • 55
Biological stimulation of the human skin applying healthpromoting light and plasma sources
Awakowicz, P. and Bibinov, N. and Born, M. and Busse, B. and Gesche, R. and Helmke, A. and Kaemling, A. and Kolb-Bachofen, V. and Kovacs, R. and Kuehn, S. and Liebmann, J. and Mertens, N. and Niemann, U. and Oplaender, C. and Porteanu, H.-E. and Scherer, J. and Suschek, C. and Vioel, W. and Wandke, D.
CONTRIBUTIONS TO PLASMA PHYSICS. Volume: 49 (2009) - 2009 • 54
Gold nanoparticles partially embedded in ultrathin anodic alumina films
Mardare, A.I. and Borodin, S. and Wieck, A.D. and Rohwerder, M. and Hassel, A.W.
JOURNAL OF PHYSICAL CHEMISTRY C. Volume: 113 (2009) - 2009 • 53
Effect of aspect ratio on transverse diffusive broadening: A lattice Boltzmann study
Ayodele, S.G. and Varnik, F. and Raabe, D.
PHYSICAL REVIEW E - STATISTICAL, NONLINEAR, AND SOFT MATTER PHYSICS. Volume: 80 (2009) - 2009 • 52
Polarization-induced charge carrier separation in polar and nonpolar grown GaN quantum dots
Marquardt, O. and Hickel, T. and Neugebauer, J.
JOURNAL OF APPLIED PHYSICS. Volume: 106 (2009) - 2009 • 51
Transverse diffusive mixing of solutes in pressure driven microchannels : a Lattice Boltzmann study of the scaling laws
Ayodele, S. and Varnik, F. and Raabe, D.
HOUILLE BLANCHE-REVUE INTERNATIONALE DE L EAU. Volume: (2009) - 2009 • 50
Using a two-dimensional electron gas to study nonequilibrium tunneling dynamics and charge storage in self-assembled quantum dots
Marquardt, B. and Geller, M. and Lorke, A. and Reuter, D. and Wieck, A.D.
APPLIED PHYSICS LETTERS. Volume: 95 (2009) - 2009 • 49
Conductance features in point contact Andreev reflection spectra
Baltz, V. and Naylor, A.D. and Seemann, K.M. and Elder, W. and Sheen, S. and Westerholt, K. and Zabel, H. and Burnell, G. and Marrows, C.H. and Hickey, B.J.
JOURNAL OF PHYSICS CONDENSED MATTER. Volume: 21 (2009) - 2009 • 48
An integrated experimental and theoretical investigation on Cu(hfa) 2·TMEDA: Structure, bonding and reactivity
Bandoli, G. and Barreca, D. and Gasparotto, A. and Seraglia, R. and Tondello, E. and Devi, A. and Fischer, R.A. and Winter, M. and Fois, E. and Gamba, A. and Tabacchi, G.
PHYSICAL CHEMISTRY CHEMICAL PHYSICS. Volume: 11 (2009) - 2009 • 47
Nonexponential London penetration depth of FeAs-based superconducting RFeAsO0.9F0.1 (R=La, Nd) single crystals
Martin, C. and Tillman, M.E. and Kim, H. and Tanatar, M.A. and Kim, S.K. and Kreyssig, A. and Gordon, R.T. and Vannette, M.D. and Nandi, S. and Kogan, V.G. and Bud'Ko, S.L. and Canfield, P.C. and Goldman, A.I. and Prozorov, R.
PHYSICAL REVIEW LETTERS. Volume: 102 (2009) - 2009 • 46
A cobalt(ll) hexafluoroacetylacetonate ethylenediamine complex as a CVD molecular source of cobalt oxide nanostructures
Bandoli, G. and Barreca, D. and Gasparotto, A. and Maccato, C. and Seraglia, R. and Tondello, E. and Devi, A. and Fischer, R.A. and Winter, M.
INORGANIC CHEMISTRY. Volume: 48 (2009) - 2009 • 45
Molecular staples on Si(001)-2 × 1: Dual-head primary amines
Mathieu, C. and Bai, X. and Gallet, J.-J. and Bournel, F. and Carniato, S. and Rochet, F. and Magnano, E. and Bondino, F. and Funke, R. and Köhler, U. and Kubsky, S.
JOURNAL OF PHYSICAL CHEMISTRY C. Volume: 113 (2009) - 2009 • 44
Influence of micro-blasting on the microstructure and residual stresses of CVD κ-Al2O3 coatings
Barbatti, C. and Garcia, J. and Pitonak, R. and Pinto, H. and Kostka, A. and Di Prinzio, A. and Staia, M.H. and Pyzalla, A.R.
SURFACE AND COATINGS TECHNOLOGY. Volume: 203 (2009) - 2009 • 43
Interface fracture properties of thin films studied by using the micro-cantilever deflection technique
Matoy, K. and Detzel, T. and Müller, M. and Motz, C. and Dehm, G.
SURFACE AND COATINGS TECHNOLOGY. Volume: 204 (2009) - 2009 • 42
A micromechanical model for martensitic phase-transformations in shape-memory alloys based on energy-relaxation
Bartel, T. and Hackl, K.
ZAMM ZEITSCHRIFT FUR ANGEWANDTE MATHEMATIK UND MECHANIK. Volume: 89 (2009) - 2009 • 41
A comparative micro-cantilever study of the mechanical behavior of silicon based passivation films
Matoy, K. and Schönherr, H. and Detzel, T. and Schöberl, T. and Pippan, R. and Motz, C. and Dehm, G.
THIN SOLID FILMS. Volume: 518 (2009) - 2009 • 40
Anisotropic and field-specific higher order spatial discretization methods for multiphase durability analyses
Becker, C. and Jox, S. and Meschke, G.
COMPUTERS AND STRUCTURES. Volume: 87 (2009) - 2009 • 39
Thin and dense ceramic coatings by plasma spraying at very low pressure
Mauer, G. and Vaßen, R. and Stöver, D.
PROCEEDINGS OF THE INTERNATIONAL THERMAL SPRAY CONFERENCE. Volume: (2009) - 2009 • 38
Zinc malonate based precursors for MOCVD of ZnO
Bekermann, D. and Pilard, D. and Fischer, R.A. and Devi, A.
ECS TRANSACTIONS. Volume: 25 (2009) - 2009 • 37
Atmospheric plasma spraying of yttria-stabilized zirconia coatings with specific porosity
Mauer, G. and Vaßen, R. and Stöver, D.
SURFACE AND COATINGS TECHNOLOGY. Volume: 204 (2009) - 2009 • 36
Investigation of niobium nitride and oxy-nitride films grown by MOCVD
Bekermann, D. and Barreca, D. and Gasparotto, A. and Becker, H.W. and Fischer, R.A. and Devi, A.
SURFACE AND COATINGS TECHNOLOGY. Volume: 204 (2009) - 2009 • 35
Alloy liquid metal ion source for carbon focused ion beams
Mazarov, P. and Wieck, A.D. and Bischoff, L. and Pilz, W.
JOURNAL OF VACUUM SCIENCE AND TECHNOLOGY B: MICROELECTRONICS AND NANOMETER STRUCTURES. Volume: 27 (2009) - 2009 • 34
Anisotropy and dynamic properties of Co2MnGe Heusler thin films
Belmeguenai, M. and Zighem, F. and Woltersdorf, G. and Roussigné, Y. and Chérif, S.M. and Westerholt, K. and Bayreuther, G.
JOURNAL OF MAGNETISM AND MAGNETIC MATERIALS. Volume: 321 (2009) - 2009 • 33
In situ high energy x-ray synchrotron diffraction study of the synthesis and stoichiometry of LaFeAsO and LaFeAs O1-xFy
McCallum, R.W. and Yan, J.-Q. and Rustan, G.E. and Mun, E.D. and Singh, Y. and Das, S. and Nath, R. and Bud'Ko, S.L. and Dennis, K.W. and Johnston, D.C. and Canfield, P.C. and Kramer, M.J. and Kreyssig, A. and Lograsso, T.A. and Goldman, A.I.
JOURNAL OF APPLIED PHYSICS. Volume: 105 (2009) - 2009 • 32
Microstrip line ferromagnetic resonance and Brillouin light scattering investigations of magnetic properties of Co2MnGe Heusler thin films
Belmeguenai, M. and Zighem, F. and Roussigné, Y. and Chérif, S.-M. and Moch, P. and Westerholt, K. and Woltersdorf, G. and Bayreuther, G.
PHYSICAL REVIEW B - CONDENSED MATTER AND MATERIALS PHYSICS. Volume: 79 (2009) - 2009 • 31
Height analysis of amorphous and crystalline ice structures on Cu(111) in scanning tunneling microscopy
Mehlhorn, M. and Morgenstern, K.
NEW JOURNAL OF PHYSICS. Volume: 11 (2009) - 2009 • 30
Molecular beam sampling system with very high beam-to-background ratio: The rotating skimmer concept
Benedikt, J. and Ellerweg, D. and Von Keudell, A.
REVIEW OF SCIENTIFIC INSTRUMENTS. Volume: 80 (2009) - 2009 • 29
Local investigation of femtosecond laser induced dynamics of water nanoclusters on Cu(111)
Mehlhorn, M. and Carrasco, J. and Michaelides, A. and Morgenstern, K.
PHYSICAL REVIEW LETTERS. Volume: 103 (2009) - 2009 • 28
Decoupling of magnetic core and shell contributions in antiferromagnetic Co3O4 nanostructures
Benitez, M.J. and Petracic, O. and Tüysüz, H. and Schüth, F. and Zabel, H.
EUROPHYSICS LETTERS. Volume: 88 (2009) - 2009 • 27
A Study of the influence of composition on the microstructural properties of ZnO/Al2O3 mixed oxides
Miao, S. and D'Alnoncourt, R.N. and Reinecke, T. and Kasatkin, I. and Behrens, M. and Schlögl, R. and Muhler, M.
EUROPEAN JOURNAL OF INORGANIC CHEMISTRY. Volume: (2009) - 2009 • 26
Constrained optimal discrimination designs for Fourier regression models
Biedermann, S. and Dette, H. and Hoffmann, P.
ANNALS OF THE INSTITUTE OF STATISTICAL MATHEMATICS. Volume: 61 (2009) - 2009 • 25
The role of heterogeneous deformation on damage nucleation at grain boundaries in single phase metals
Bieler, T.R. and Eisenlohr, P. and Roters, F. and Kumar, D. and Mason, D.E. and Crimp, M.A. and Raabe, D.
INTERNATIONAL JOURNAL OF PLASTICITY. Volume: 25 (2009) - 2009 • 24
Multispectral photoacoustic coded excitation using orthogonal unipolar Golay codes
Mienkina, M.P. and Friedrich, C.-S. and Gerhardt, N.C. and Hofmann, M.R. and Schmitz, G.
IFMBE PROCEEDINGS. Volume: 25 (2009) - 2009 • 23
Kinetics and particle size effects in ethene hydrogenation over supported palladium catalysts at atmospheric pressure
Binder, A. and Seipenbusch, M. and Muhler, M. and Kasper, G.
JOURNAL OF CATALYSIS. Volume: 268 (2009) - 2009 • 22
Feasibility study of multispectral photoacoustic coded excitation using orthogonal unipolar golay codes
Mienkina, M.P. and Eder, A. and Schmitz, G. and Friedrich, C.-S. and Gerhardt, N.C. and Hofmann, M.R.
PROCEEDINGS - IEEE ULTRASONICS SYMPOSIUM. Volume: (2009) - 2009 • 21
A note on some random orthogonal polynomials on a compact interval
Birke, M. and Dette, H.
PROCEEDINGS OF THE AMERICAN MATHEMATICAL SOCIETY. Volume: 137 (2009) - 2009 • 20
Evaluation of Ferucarbotran (Resovist®) as a photoacoustic contrast agent
Mienkina, M.P. and Friedrich, C.-S. and Hensel, K. and Gerhardt, N.C. and Hofmann, M.R. and Schmitz, G.
BIOMEDIZINISCHE TECHNIK. Volume: 54 (2009) - 2009 • 19
Ellipsoidal InAs quantum dots observed by cross-sectional scanning tunneling microscopy
Blokland, J.H. and Bozkurt, M. and Ulloa, J.M. and Reuter, D. and Wieck, A.D. and Koenraad, P.M. and Christianen, P.C.M. and Maan, J.C.
APPLIED PHYSICS LETTERS. Volume: 94 (2009) - 2009 • 18
Capacitance-voltage analysis of ZrO2 thin films deposited by thermal MOCVD technique
Mih, T.A. and Paul, S. and Milanov, A.P. and Bhakta, R. and Devi, A.
ECS TRANSACTIONS. Volume: 25 (2009) - 2009 • 17
Stability of ultrafine-grained Cu to subgrain coarsening and recrystallization in annealing and deformation at elevated temperatures
Blum, W. and Li, Y. J. and Durst, K.
ACTA MATERIALIA. Volume: 57 (2009) - 2009 • 16
Rare-earth based oxide and nitride thin films employing volatile homoleptic guanidinate precursors
Milanov, A.P. and Thiede, T. and Hellwig, M. and Parala, H. and Bock, C. and Becker, H.-W. and Ngwashi, D.K. and Cross, R.B.M. and Paul, S. and Kunze, U. and Fischer, R.A. and Devi, A.
ECS TRANSACTIONS. Volume: 25 (2009) - 2009 • 15
Ab initio investigation of diamond coatings on steel
Böhner, A. and Janisch, R. and Hartmaier, A.
SCRIPTA MATERIALIA. Volume: 60 (2009) - 2009 • 14
Lanthanide oxide thin films by metalorganic chemical vapor deposition employing volatile guanidinate precursors
Milanov, A.P. and Toader, T. and Parala, H. and Barreca, D. and Gasparotto, A. and Bock, C. and Becker, H.-W. and Ngwashi, D.K. and Cross, R. and Paul, S. and Kunze, U. and Fischer, R.A. and Devi, A.
CHEMISTRY OF MATERIALS. Volume: 21 (2009) - 2009 • 13
Homoleptic gadolinium guanidinate: A single source precursor for metal-organic chemical vapor deposition of gadolinium nitride thin films
Milanov, A.P. and Thiede, T.B. and Devi, A. and Fischer, R.A.
JOURNAL OF THE AMERICAN CHEMICAL SOCIETY. Volume: 131 (2009) - 2009 • 12
Cycling properties of Sc- and Ce-doped NaAlH4 hydrogen storage materials prepared by the one-step direct synthesis method
Bogdanović, B. and Felderhoff, M. and Pommerin, A. and Schüth, F. and Spielkamp, N. and Stark, A.
JOURNAL OF ALLOYS AND COMPOUNDS. Volume: 471 (2009) - 2009 • 11
Effect of the addition ZrO2-Al2O3 on nanocrystalline hydroxyapatite bending strength and fracture toughness
Mobasherpour, I. and Solati Hashjin, M. and Razavi Toosi, S.S. and Darvishi Kamachali, R.
CERAMICS INTERNATIONAL. Volume: 35 (2009) - 2009 • 10
Prediction of cold rolling texture of steels using an Artificial Neural Network
Brahme, A. and Winning, M. and Raabe, D.
COMPUTATIONAL MATERIALS SCIENCE. Volume: 46 (2009) - 2009 • 9
Snapshot of a reaction intermediate: Analysis of benzoylformate decarboxylase in complex with a benzoylphosphonate inhibitor
Brandt, G.S. and Kneen, M.M. and Chakraborty, S. and Baykal, A.T. and Nemeria, N. and Yep, A. and Ruby, D.I. and Petsko, G.A. and Kenyon, G.L. and McLeish, M.J. and Jordan, F. and Ringe, D.
BIOCHEMISTRY. Volume: 48 (2009) - 2009 • 8
Isomerization reactions on single adsorbed molecules
Morgenstern, K.
ACCOUNTS OF CHEMICAL RESEARCH. Volume: 42 (2009) - 2009 • 7
The effect of carbon pore structure on the adsorption of cigarette smoke vapour phase compounds
Branton, P. and Lu, A.-H. and Schüth, F.
CARBON. Volume: 47 (2009) - 2009 • 6
Catalytic decomposition of alcohols over size-selected Pt nanoparticles supported on ZrO2: A study of activity, selectivity, and stability
Mostafa, S. and Croy, J.R. and Heinrich, H. and Cuenya, B.R.
APPLIED CATALYSIS A: GENERAL. Volume: 366 (2009) - 2009 • 5
Interactions between lattice dislocations and twin boundaries in tungsten: A comparative atomistic simulation study
Mrovec, M. and Elsässer, C. and Gumbsch, P.
PHILOSOPHICAL MAGAZINE. Volume: 89 (2009) - 2009 • 4
Prevention of damages to concrete gutters - Considerations on the desiqn of expansion joints
Breitenbücher, R. and Sievering, C.
BETON- UND STAHLBETONBAU. Volume: 104 (2009) - 2009 • 3
Schottky barriers at transition-metal/ SrTiO3 (001) interfaces
Mrovec, M. and Albina, J.-M. and Meyer, B. and Elsässer, C.
PHYSICAL REVIEW B - CONDENSED MATTER AND MATERIALS PHYSICS. Volume: 79 (2009) - 2009 • 2
Concrete attack in iron disulphidic soils
Breitenbücher, R. and Wisotzky, F. and Eisenberg, V. and Siebert, B.
BETON- UND STAHLBETONBAU. Volume: 104 (2009) - 2009 • 1
Semiconductor laser based THz generation and detection
Brenner, C. and Hoffmann, S. and Friedrich, C.-S. and Schlauch, T. and Klehr, A. and Erbert, G. and Tränkle, G. and Jördens, C. and Salhi, M. and Koch, M. and Hofmann, M.R.
PHYSICA STATUS SOLIDI (C) CURRENT TOPICS IN SOLID STATE PHYSICS. Volume: 6 (2009)